AI Minds #048 | Sumanyu Sharma, Co-Founder and CEO at Hamming AI
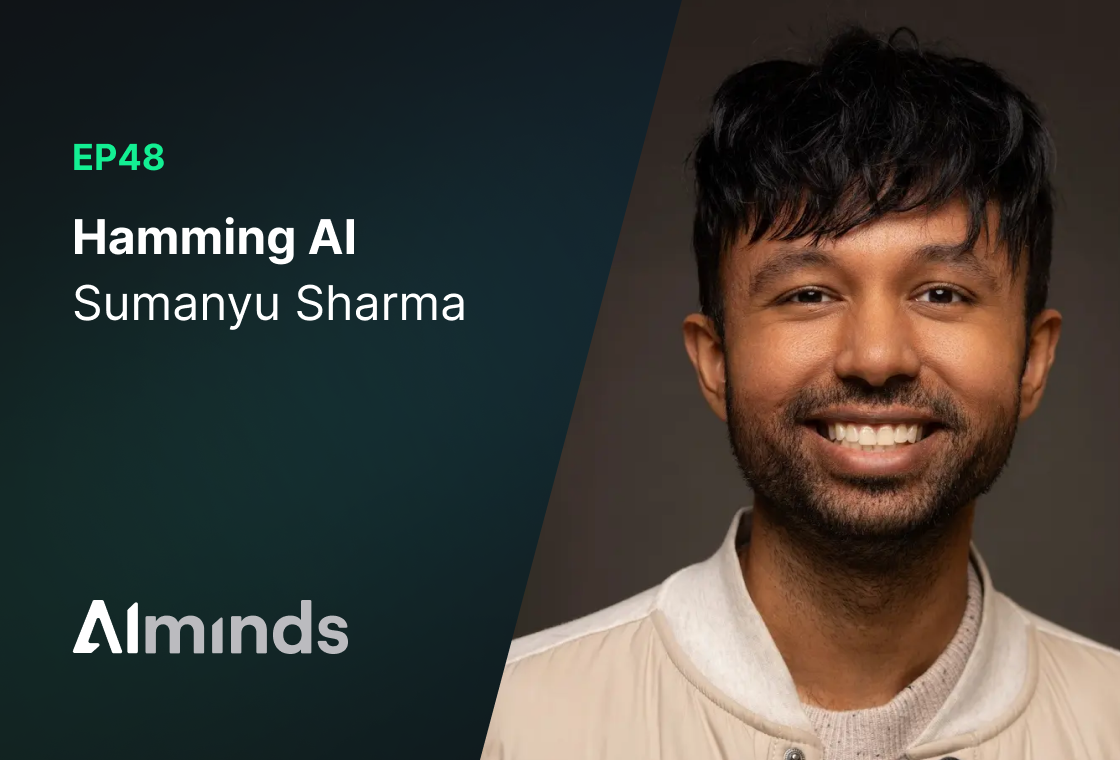
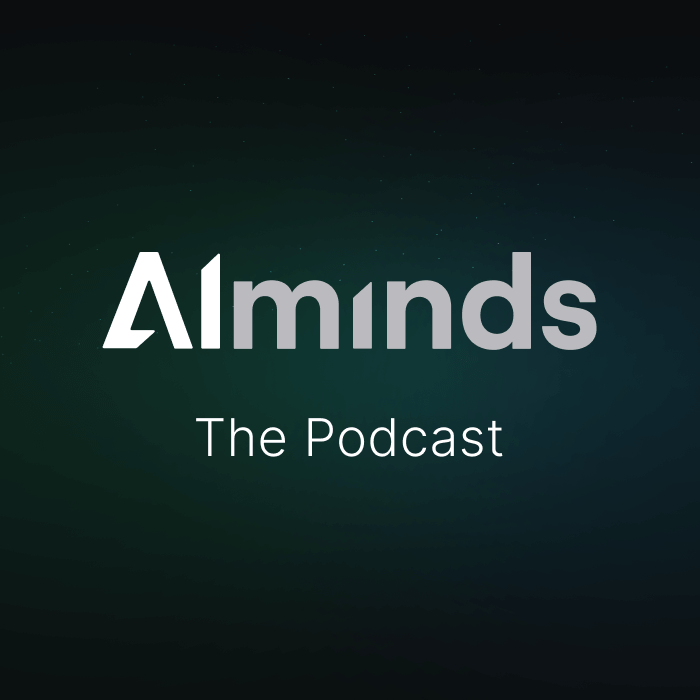
About this episode
Sumanyu Sharma, Co-Founder and CEO at Hamming AI; the platform automates AI voice agent testing, production call monitoring and governance for AI voice agents. The army of voice AI agents act like real people and place thousands of test calls simultaneously to find bugs, hallucinations and other issues often missed during manual testing.
Listen to the episode on Spotify, Apple Podcast, Podcast addicts, Castbox. You can also watch this episode on YouTube.
In this episode of AI Minds, Sumanyu Sharma, co-founder and CEO of Hamming, shares his inspiring journey—from a sheltered upbringing in India to finding himself intertwined with the future of AI-driven voice technology in Silicon Valley.
Sumanyu reflects on his transformative experiences at the University of Waterloo, pivotal internships, and his deep dive into the world of startups.
He delves into the challenges and breakthroughs in voice AI, highlighting the critical need for safety and reliability in systems designed for high-stakes industries like healthcare and customer service. Drawing from his time at Tesla and other leading organizations, Sumanyu shares how rigorous testing and real-world simulations are vital to preventing failures and ensuring accuracy in AI systems.
The conversation also explores the future of voice AI, its potential to surpass text interfaces, and how companies are integrating voice technology into innovative applications. Sumanyu envisions a world where voice AI becomes ubiquitous, transforming human-computer interactions while adhering to ethical and safety standards.
Tune in to learn how Hamming is at the forefront of voice AI innovation and discover the human-centered approach that drives Sumanyu’s mission.
Fun Fact: Sumanyu mentions how his time at the University of Waterloo significantly opened him up to the world of ambition and hard work. Initially finding the university challenging, he credits this experience as pivotal in broadening his perspective on technology and work ethic.
Show Notes:
00:00 Waterloo opened doors to ambition and discovery.
04:03 Machine learning's exponential progress fascinated me.
06:26 Citizen app provides real-time crime and safety alerts.
11:17 Voice agents struggle with poor audio and expectations.
15:11 Testing involves ensuring voice agents handle interruptions.
17:39 Analyzing interaction patterns, improving voice agent performance.
21:06 Voice will surpass text; focus on reliability.
More Quotes from Sumanyu:
Transcript:
Demetrios:
Welcome back to the AI Minds podcast. This is a podcast where we explore the companies of tomorrow being built AI first. I'm your host, Demetrios and this episode, like every episode, is brought to you by Deepgram. The number one speech to text and text to speech API on the Internet today. Trusted by the world's top conversational AI leaders, enterprises and startups like Spotify, Twilio, NASA and Citibank. I'm joined in this episode by the co founder and CEO of Hamming Sumanyu. Did I say it right?
Sumanyu Sharma:
Yep, you got it pretty close, Sumanyu.
Demetrios:
Almost.
Sumanyu Sharma:
Almost.
Demetrios:
So you spent some time in India, grow well, born there, moved to Canada early, went to Waterloo and then decided you wanted to get into the startup game. How did that fare for you? Because it seems like you've been at it for a while.
Sumanyu Sharma:
Yeah, Waterloo was quite special for me. I grew up, I would say, pretty sheltered. I didn't really know about tech in high school besides a few courses in HTML, css, you know, the basics. And Waterloo really opened up the door of what it means to be ambitious and what it means to work hard. You know, high school was pretty easy. Waterloo was not that easy. I struggled quite a bit for the first little bit, and I think I just discovered a whole new world I didn't even know existed.
Demetrios:
And did you start a company right after you left Waterloo?
Sumanyu Sharma:
I didn't. I tried starting. There was a project, I would say a hackathon at one of these, you know, hackathons were pretty big. I had a project idea in mind. I honestly didn't have the skills. I don't think the idea was that good. And I didn't have a great team to go build something great. And so I decided, hey, let's solve for these three things and then let's actually build something great instead of, you know, try to start a company just for the sake of it.
Demetrios:
And so that presumably brought you to California.
Sumanyu Sharma:
That's correct. The Waterloo, the phrase is cal, your bust. And so the goal was always to spend time in SF and level up. Because in Waterloo you spent maybe two years or so and you start saturating. Now you're looking for the next thing to level up. And Bay Area is the global kind of standard of excellence. At least that was true in my mind. I think it's still true.
Sumanyu Sharma:
And I tried really hard to get an internship in SF to learn as much as I can, and I got a lucky break in 2013.
Demetrios:
What happened?
Sumanyu Sharma:
I ended up interning at Future Advisor. It's a YC company. It looked really promising. I actually bombed the interview. Completely bombed it. And I hired you. They still did. I told them, hey, look, I know I bombed the interview, I'm aware.
Sumanyu Sharma:
But I will outwork anyone else and just give me a shot and I will make it work. I'll figure out a way to create value, learn really quickly, like whatever it takes to win. And so that's, that's been the mindset, I would say, since 2013 and it's still true today. Wow.
Demetrios:
There's a funny story I think that is you worth talking about and somebody that you were working with a Future Advisor then became your advisor.
Sumanyu Sharma:
He did, yeah. So I work with Bo Lu and John Xu. Both are co founders of Future Advisor and John was actually our group partner. We went through YC in summer 24, just a couple of months back and he happened to be one of our group partners, which was really awesome. I feel like John's been opening doors for us for me for now, 10 years straight. And so I owe a lot to John and both.
Demetrios:
Wow. So how did you get into AI?
Sumanyu Sharma:
Machine learning was pretty hot back in 2013, 2014, in one of the internships. I ended up doing a lot of machine learning and driving impact and I got hooked in the ability for systems to learn from data and actually become better the more data you have or the better the algorithm is. And I've just been double clicking on that since then. It's just a fascinating, I would say exponential rate of progress kind of since then. Ended up publishing a paper while I was at Waterloo in researching medical images and trying to make the lives of radiologists better by making it easy for them to pass in an X ray as an input and get back other clinically relevant X rays to make the diagnosis kind of easier. There's a few startups actually that have. Are building kind of around this similar concept. But you know, we were doing something really basic back then.
Demetrios:
Yeah. It's evolved quite a bit since then. Even just with the diffusion models, you can.
Sumanyu Sharma:
Absolutely.
Demetrios:
But still the same premise is there, that you want to make a radiologist or a doctor's life easier and make their diagnosis more confident.
Sumanyu Sharma:
That's correct. The tricky thing with that body of work and to be honest, voice agents right now is it's you want these systems to be accurate, you want these systems to be largely hallucination free as much as possible. And the tooling around this is very, very scarce, especially voice. I think in text, there's a Lot of companies that have innovated in helping other companies, other enterprises build reliable, you know, text style LLM or AI products. But this is missing in voice. And so the same challenges that we saw back in 2014 when I was a student, the same challenges continue to be true with voice. And so the theme of trust, safety, reliability has been pretty central for me.
Demetrios:
Yeah, so I feel like there was an insight that you mentioned to me before we hit record when you were at Citizen, working there and recognizing that audio is so important and there's this link between audio and safety data. Can you go into that?
Sumanyu Sharma:
Absolutely. The core insight for Citizen app, it's a consumer app here in the US it's quite popular in New York, LA. The core product sends you crime alerts, safety alerts, based on your location in real time to keep you safe and informed. And the insight behind that was realizing that all 911 data, as in phone calls, are actually public. And why not increase transparency and why not increase trust by having that information, transcribing it? It's like audio to text and then transcribing it, enriching it and then sending it out to users. And that would drive safety for people. And the same, I would say the same core insight we've been feeling and I guess synthesizing in the voice agent world where if you're talking to voice agents, you want them to not give medical advice. Right.
Sumanyu Sharma:
If you have a scheduler appointment, scheduler care coordinator, if someone's asking for medical advice, you can't really give that. And so it's AI safety, but it's the same core concept. So audio and safety really go well together. Audio is so it's a universal API for how we interact with the world and you just want that interface to be sacred and trusted as opposed to distrusted.
Demetrios:
Yeah, I feel like this idea of guardrails comes up a lot in the text based AI workflows. And that's probably because there's been so many companies that just slapped a chatbot on their website and then they got burnt for it. Like Air Canada, I think is one that is very obvious. There was another one that was like a Chevy dealer, right. And they were talking about how Teslas are better than Chevys. And you're thinking a Chevy dealer's chatbot is saying that Teslas are. And then it ended up selling a Chevy for $1 or something. So you see that there are problems with the tech space, but I don't hear it being as spoken about with voice agents.
Demetrios:
And having guardrails, keeping them on track and not allowing them to just answer whatever question the user asks. So that's, a fascinating insight and it feels like, I guess, what is the inspiration that you recognized? Wow. There's this insight that audio and AI can do everything or go anywhere at once if it's not tamed. We should probably build a company around that.
Sumanyu Sharma:
Yeah, I think in, retrospect it should have been obvious. I think it was maybe less obvious, you know, as, as you, as you, as you proceed forward and build something new. I think originally we were thinking of doing something in the tech space, but it just didn't feel of high consequence to us. It felt pretty saturated. There's 20 companies. It's sort of like pretty obvious. We always wanted to do voice, we just never found the right time. And so YC was the right time to kind of go all in and double click on voice and make sure these voice agents are stable.
Sumanyu Sharma:
So the next day or Canada incident or the next Chevy incident doesn't happen. And that's been our core focus. And I actually think, I think voice will actually end up being bigger than text. That's my personal prediction. And it's been largely a secret. There's lots of companies that are scaling very quickly with voice. I mean obviously DBRI included and it just feels like an open secret and now it's becoming more obvious. But when we were, I guess pioneering a couple of months back, it was very not obvious.
Sumanyu Sharma:
We're the only company that we're trying to make these agents reliable.
Demetrios:
Yeah. And there is another piece to that where you're thinking about, okay, Voice when it is looked at. Right now a lot of people are imagining replacing call centers with voice. I think that's about as far as our creativity goes. But there's so many other use cases and I wonder if you've seen some cool ones as you're talking with customers about how they're incorporating voice or even what their future vision of how voice will interact with their customers.
Sumanyu Sharma:
I think the most interesting one, I would say, or the most difficult ones to get right have been in the drive thru space. I think that's a really, really hard challenge. There's lots of companies that have tried to build voice ordering agents and reliability has to be in the number one problem. And so we focus pretty heavily in that space to make that reality, make that dream become reality.
Demetrios:
Why is it hard?
Sumanyu Sharma:
It's hard because in drive throughs the audio quality is actually quite poor. There's a lot of static people order very quickly. There's people use codes, I'll order the number one, the number seven. There's a lot of food allergies you have to take into account. And if you're building a voice agent that misses any allergies, I mean that's a lawsuit at some point or at least a very long delay for a human to go back and correct it. And so I think the most interesting to me are deployments in areas where there's high expectations for performance. Healthcare, banking services. Right.
Sumanyu Sharma:
If you're talking to, I mean, IVRs are pretty hard to traverse, but if you're, if you're trying to get something done and the agent promises, yes, I will go ahead and do that for you. And it doesn't do that. That's quite problematic. On the creativity front, a lot of interesting use cases around training humans. There's a few companies we're working with who are actually training, who are doing simulations to train humans. And we're helping train the simulations that then help train humans. So it's a little recursive, but that's the funniest, I would say.
Demetrios:
And what are the trainings for just training people how to speak to customers? Like sales training for people. That's correct.
Sumanyu Sharma:
Improving quality, improving their competency, having a safe way for them to practice different scenarios. You know, it's kind of hard to find a bunch of people who have all these super rare accents. But if you're using Deepgram or other, TTS style providers or SAT providers, it's a lot easier for us to help simulate the real world. We can have background noise, we can have some kids crying in the back. And so that helps train the humans on handling these kind of cases.
Demetrios:
Yeah, I can imagine. The drive through one has got to be so difficult because the slang that's used also at every drive through and the different dialects and if someone doesn't realize that they are speaking to an AI and you just speak normal, sometimes it will be okay and then other times it won't be because I think it's not quite at the point with all of the noise where you can pick up everything. And like you said, if the hardware isn't there and it's not picking up the sound in a high quality manner, the. That just makes it so much more difficult for everything downstream.
Sumanyu Sharma:
That's correct. And testing these systems is super, super painful because, are you really gonna, buy like a blow dryer or like try to Add noise, physical noise. And so it makes a lot more sense for us to virtualize these environments and kind of like Tesla, when I was at Tesla back in the day, we had an internal simulation, rig that would allow the FSD team to make changes to the system and test it before launching to prod and having issues. Let's actually simulate and test out hey, how does it actually perform kind of in dev. And those testing cycles are so much faster by having it kind of with you than trying to kind of ship in prod. And so we're productizing, I would say the core insights from Tesla, FSD team and the Citizen, observations including Anduril as well around like safety and reliability.
Demetrios:
And what are you doing exactly for the simulations with is it on someone's like an enterprise comes to you and says hey, I've got this model or I've got this voice agent. Can you stress test it? Can you tell me where it's weak, where it's strong? Is that the idea?
Sumanyu Sharma:
That's the idea. And I think testing it's. There's different styles of it, there's a lot of nuances. So testing could be regression testing where there's clear paths and states that are allowed for your voice agent that you want to make sure are handled like your agent's handling them correctly. Those are happy about testing. I think the adversarial testing ends up being more funny and more interesting where we'll often just have our agents just interrupt aggressively, just start interrupting and the voice agent gets sort of really confused or frustrated or even restarts the loop where it starts. Hey, how can I help you? So there's lots of different edge cases that we've now discovered across customers that are very not obvious. Adding an elevator ding or adding some background noise forces some voice agents to just like pause because they think someone's talking and so you end up just like waiting for each other to talk.
Sumanyu Sharma:
And so that ends up being a lot of the work we do initially. But as we help make these voice agents reliable and give visibility into hey, how good are they really? Are they really ready to go live or not? Then the challenge becomes preventing regressions and monitoring real world behavior and connecting it back to the testing piece. So really monitoring in the drive thru case it's looking at every order at actual human calls and transcripts and analyzing them and finding errors and then proactively creating test cases for them. So you have a self kind of improving optimization loop very similar to the FSD objective internally.
Demetrios:
There's a string I want to pull on and I'm not quite sure that I'm going to be able to fully form the or articulate this thought that I have. But I had a friend that worked at Amazon with Alexa and he talked about how a lot of times they wouldn't know when Alexa failed because it's like a silent failure. Somebody says hey Alexa. And then it doesn't respond. You don't know that it just failed. So I get the feeling that you also are encountering that type of thing. How do you deal with it? How do you. It's like these silent failures that potentially can slip under the rug.
Sumanyu Sharma:
Totally. That's kind of where a lot of the production call analytics comes in. And deeply understanding what was the interaction pattern between a human in the voice agent and looking for all the error and failure modes that the voice agent made and looking across patterns not just within one conversation, but across conversations to give product ENG Ops teams insights into what they should be improving. I think that's one part of the equation. The other is there's one thing we discovered that was surprisingly helpful for our customers, which is production health checks where a human perhaps may stumble upon an edge case in a low frequency way. Maybe it's not every single true action that's failing. We can stress test the production systems, not just the dev systems, but the production systems live kind of like in a chaos monkey style. Netflix had a chaos monkey just testing prod.
Sumanyu Sharma:
So we can do that as well. And that's a much more deterministic way for us to define these kind of silent errors and make them loud.
Demetrios:
In the product, how does it work? I've. You find errors and then do you go back and you retrain a model or are you just recognizing that like how do you fix these errors when they come about?
Sumanyu Sharma:
Yeah, step one for us that we're extremely. It's a hard problem. We haven't solved it completely. The first step is to detect errors and to observe. That's the first step. I think we're still there. The next step is at the moment humans take that information and then go make changes to the system in order to improve it. The ideal version is for having to go and fix these agents proactively based on the errors that are coming.
Sumanyu Sharma:
We're not there yet and it's still an already fairly difficult problem. We haven't quite cracked it, but are always looking for engineers if they're interested in working on self healing, self deporting. You know, voice agents, you know, please DM me. That's the. The holy grail for us is to not need, you know, a human loop. Maybe a human can review and approve things, but we want actually a fully closed loop optimized system as much as we can. That would be the best.
Demetrios:
Yeah, I can see that vision where a human is just getting flagged. Something like a series of calls or transcripts and there is a next best action being presented to the human. Do you want us to go retrain? Just click okay and boom. It will do what it needs to do to ideally improve and then test and simulate it. Like you were saying before. It brings it and it pushes that to prod.
Sumanyu Sharma:
Exactly. To know what to improve. We first have the test and so we're in phase one. I think of the master plan. We still have to solve phase one. We haven't solved phase one yet.
Demetrios:
The voice agent field is fascinating to me and I get the feeling that it's what humans want when they interact with technology. It is the dream that we've had in the sci fi sphere for ages. You don't look at old sci fi videos and see humans clanking away at a keyboard. You see them talking directly to computers.
Sumanyu Sharma:
I think voice generally is going to have much more PMF and be much more pervasive than text. That's my personal prediction. I think this year folks are building a lot of prototypes and MVPs. I think 2025, everyone is going to be focused on reliability, especially enterprises will be really, really focused on reliability, governance, having clear guardrails to make sure these deployments don't become like Air Canada 2.0 or the Chevy 2.0. And so our vision is to help give that peace of mind at all layers of the stack. And so we're extremely excited to make these agents reliable and trustworthy.