AIMinds #024 | Liz Tsai, CEO and Founder at Hi Operator
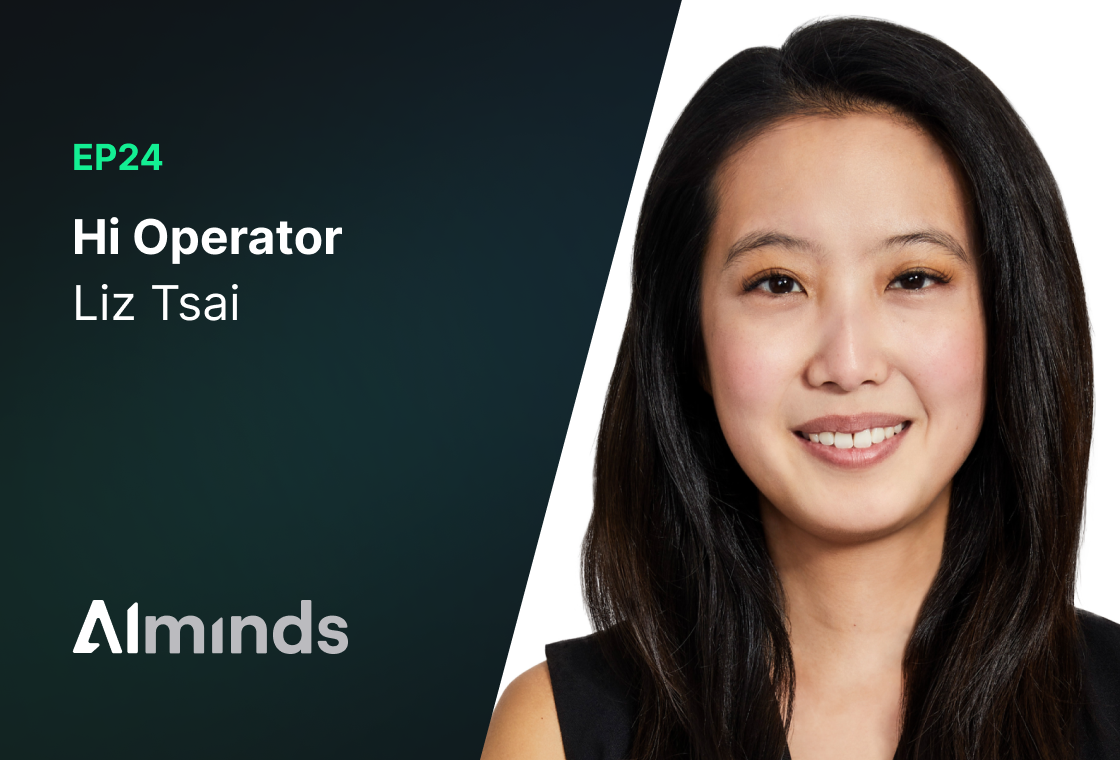
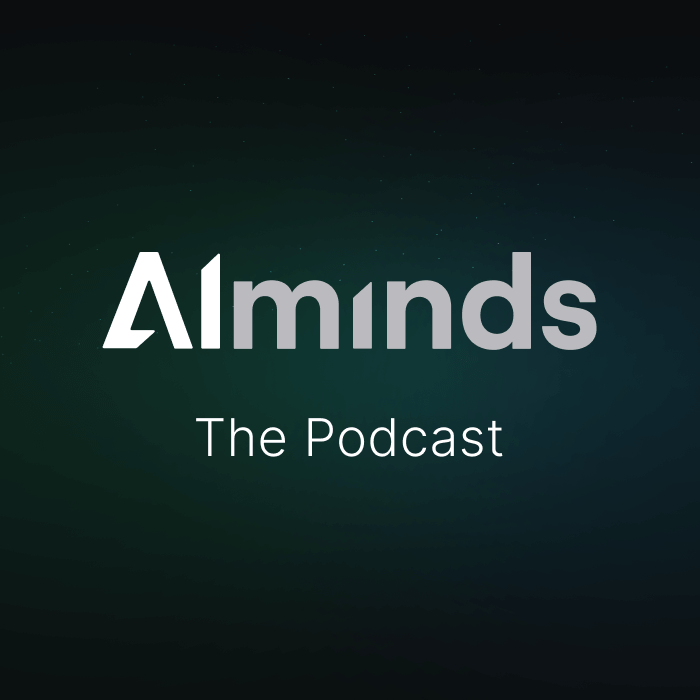
About this episode
Liz Tsai is a systems-engineer with over a decade building technology companies. She is the CEO and founder at HiOperator: an automated, customer support platform that uses generative AI to provide personalized customer responses to tackle the most complex customer issues.
Before founding HiOperator, Liz joined a YC-backed parking analytics startup in Silicon Valley. Prior to that, she was a physical commodities trader in Switzerland and Singapore.
Liz attended MIT at age 15 where she double-majored and double-minored, as well as achieved a master's degree at the MIT Media Lab.
Listen to the episode on Spotify, Apple Podcast, Podcast addicts, Castbox. You can also watch this episode on YouTube.
In this episode of the AI Minds podcast, Demetrios speaks with Liz Tsai, founder of HiQ, to discuss the role of AI in enhancing customer support. Tsai shares her journey from commodities trading to the tech world, eventually leading to her founding of HiQ. The discussion elaborates on HiQ’s pivot from its initial focus on conversational AI to refining back-end workflow automation, driven by the realization of the early limitations in conversational AI's effectiveness.
A significant portion of the episode details HiQ's strategy of learning from real operations within a contact center to deeply understand and improve customer support using AI. Tsai emphasizes the importance of AI in monitoring and improving the accuracy of automated customer interactions, ensuring they align with company policies and increase customer satisfaction. She highlights the use of large language models (LLMs) and deterministic task automation to achieve a balance between automation efficiency and reliable service quality.
The podcast concludes with insights into how AI not only automates but also oversees the quality of customer interactions, stressing the role of AI in risk management and the optimal integration of human oversight to enhance service delivery. Liz Tsai’s experience illustrates a proactive approach in leveraging AI to sustain and elevate the efficacy of customer support systems.
Show Notes:
00:00 Rediscovery of NLP and journey into customer support.
04:39 Revisiting thesis led to systematic automation. Management outdated.
08:07 Customer support processes divided into front-end, back-end.
12:58 Focus on current data, identify gaps, integrate.
15:38 Automating role-based processes with black-and-white models.
18:35 Issues arise when AI interacts with users.
23:19 Automating tasks to optimize human agents' time.
25:02 Human involvement has a threshold for effectiveness.
More Quotes from [Speaker]:
Transcript:
Demetrios:
Welcome back to the AI Minds podcast. This is a podcast where we explore the companies of tomorrow built with AI. Top of mind. I am your host, Demetrios, and this episode is brought to you by Deepgram, the number one text to speech and speech to text API on the Internet today. Trusted by the world's top conversational AI leaders, startups, and enterprises like Twilio, Spotify, NASA, and Citibank. We are joined in this episode by Liz, the founder of HiQ. How are you doing today, Demetrios?
Liz Tsai:
Thank you for having me doing well. What about yourself?
Demetrios:
I am great. I love this energy that you bring into the conversation. I know that we just talked at length about what you've been up to at HIQ, and I want to get into the inspiration behind the product, the product itself. But you have a bit of a backstory that I will do a little bit of a TLDR on and get people up to speed so that they know you were at. Well, born and raised in Texas, and then went to MIT, then said, all right, MIT was great, but I'm going to go travel the world a little bit. You were doing commodities trading, is that it?
Liz Tsai:
Yep, physical commodities trading. Applied for the job in New York. They offered it to me in Geneva, Switzerland, and I said, yeah, let's go. Let's go see what it's all about.
Demetrios:
Not a bad gig. I could see how that could be fun. And then went to Singapore and did a little bit more of that. But you stopped at some point doing the commodities trading. What made you pivot out of that?
Liz Tsai:
So one of my closest friends had just started a startup out in San Francisco. We went to MIT together, and he said, come out and work with me. And I loved going from a company of 10,000 people, a large trading company, a company of ten people, and that kind of kicked off the startup bug for me. Right. I mean, a lot of people will say, you're in customer support automation. Now, what does that to do with physical commodities trading? And the fact is, fiscal, commodities trading is like 10% macroeconomics, 90% process optimization, shipping metal around the world, selling it for a profit. And that actually very much informs the way that we think about customer support.
Demetrios:
Oh, fascinating. Okay, so you got the startup bug, then you applied to YC, and you got into YC in 2017 and started doing something that I think a lot of people were doing at the time, but it fizzled out. Right. There was the year of. It was the last time we had the year of the chatbot.
Liz Tsai:
What happened I think people had rediscovered NLP, and people were going around saying, well, why can we hit with the NLP hammer? And I think customer support is always very tempting because of the sheer amount of data, text and voice that you generate there. And we looked at that and we said, okay, we do believe that automation is very much part of the future of customer support. But 2017 conversational AI is not ready for prime time. So what can you do? What's the backend workflow automation that you can build? And how do we learn about that? I mean, we were a bunch of MIT nerds who went through IC who didn't know a lot about customer support. And so we ended up going on this journey over the next five years where we essentially built like a really big outsourced contact center, like hundreds of seats out in western New York, and really learning from the floor up, what does it mean to actually run customer support programs at scale.
Demetrios:
So you went through the tech accelerator YC, and then you said, which I've heard they tell you to go and talk to customers. You said, all right, you know what's better than talking to customer? Becoming our own customer.
Liz Tsai:
Exactly.
Demetrios:
Oh, that is classic. And so what did you learn over those years of building this company?
Liz Tsai:
We learned a lot. Right? Because customer support is often where edge cases happen. Customer support, by nature, is a problem with a lot of service area, because, you know what? If the customer went through everything perfectly, they wouldn't have ended up in customer support generally. Maybe there's some proactive outreach things. And so we said, you know what? If the goal is to learn all about customer support and automate that, and that involves scaling a big call center, fine, let's do it. Let's learn from that. And then 2021 2022 hit, and we kind of looked up and we said, you know what? Conversational AI, we all saw this with chat GPT is kind of getting ready for primetime.
Liz Tsai:
So we went back, revisited our thesis, and we ended up starting to automate, very systematically our entire customer support outsourcing function. And what that looked like was breaking apart all the customer support processes into literal processes, and then going through and saying, what can we automate? What can we not automate in a very systematic fashion? And then when we started to automate customer support fractionally, and then in some cases entirely, we started to realize that all of the sort of management and quality infrastructure we built up when we were hundreds of agents didn't work anymore. So when you have hundreds of customer service agents, you have team leads, you have managers, and you also have like QA analysts, right. Their job is to every week look and go and grade somewhere between one to 5% of last week's contacts. So you can give feedback to your agents and help your agents improve and also report back and say, what is the quality of support that all my agents are providing? And it's slow, it's delayed, it's a very manual audit process. Right. Between that and you might also ask your customers how you're doing. Like I said, a CSAT survey between these two, you get maybe five to 15% of data coverage out of that.
Liz Tsai:
And it's not ideal to have data on a weekly delayed basis. But when you're managing big teams of humans, humans that are smart, that you've hired for attitude, humans can figure it out. Right. It's okay to be a little bit slow, but we realized that when we started to actually automate customer support, you needed much faster ways to get instant feedback on quality monitoring and observability. So you sort of see in real time, I've got all these automations going, are they going off the rails or are they not? And so the first version of what is now HIQ was really built for our own customer support team as a way to say, how can we use AI? Not just to directly automate customer support, but to provide real time visibility and data information back to CX leaders.
Demetrios:
So it's, it's that monitoring layer. And I do really like that because if things are going off the rails, you want to know as fast as possible so you can course correct. Yeah, yeah, exactly. Before your customers know. Ideally because they shouldn't have to be the ones that are reporting it to you. But I do think they're taking a step back and going to these, the processes part and recognizing what can we automate, what is not automatable? Is that a word? And for some reason, when I said it's slow, I don't know if it actually is a word. But the part that I wanted to ask was around what were some pieces of these building blocks that you realized this is really not, we cannot bring automation to this part of the process.
Liz Tsai:
You know, it's hard to say. We're going from zero to one, like no automation to fully automated. Right. But when you start to really take a systematic, process, process oriented approach to what actually goes into a customer service conversation, you start to realize that there are a lot of pieces that actually make maybe more sense to automate than to necessarily have a human do. And we approached this from the perspective of, look automation is not the goal, right? No one's giving you a gold star for how much automation you're using. What you care about are your ultimate business metrics. That might be, that might be first response time, that might be quality, that might be CSAT, whatever that is, that's what matters. And so whether you use automation or not really goes towards supporting that.
Liz Tsai:
And so when we first started, what we did was we broke down a lot of, customer support interactions into the front end, generally the conversational layer, and then the back end, like the workflow automation layer, right? So we've all been e commerce customers. So here's a really simple example, right? Imagine, you know, you are emailing in a customer service team and saying, hey, I bought this, it's broken. Please honor the warranty, right. The conversational layer is the company understanding that, hey, that's what the customer is asking for, right? The backend layer is then going and say, can we hook into the e commerce system? Can we look up the customer? Can we locate their order? Is it within the warranty? Oh, they sent us a photo. Is that photo in fact, of a broken item processing the replacement and then sending that back to the conversational aspect. And so when we look at that, we say, okay, well, the conversational aspect is kind of 10% of the work the company needs to do, but it's 90% of the customer's experience. Right? So that's where, especially when it's customer facing, we want to be extra, extra careful and have really strong guardrails that any sort of automation we use. But the backend processes, looking things up, determining whether it's qualified that automate.
Liz Tsai:
Humans are not great at clicking around, doing math, all of that stuff. But we do use a lot of both generative models as well as other smaller ML machine learning models in our system. And there, once something's customer facing, we sort of think about this matrix of whether we can automate and how much to automate. Because you can do many things with models. You can feed it all of your support documents and policies and say, please answer these questions, or you can use them more as sort of a final filter. In the warranty case, what we would be doing is doing all the backend actions and collecting essentially a list of what was done. And then you're asking a generative model to, hey, don't make things up from scratch. This is the statement of facts.
Demetrios:
Reframe it for us and reframe it so that you can give it to the customer in a way. Or is it reframe it just to make sure that everything that was done was correctly done.
Liz Tsai:
Ah, great question. Two things, right? So you both want to think about automating directly. But then we sort of went automation first, or we started automating the reply, for example. So here's what the customer wrote. Here's the statement of facts that we did. Please generate a really empathetic reply back to the customer. Right, so we want automation first. But then as we started to hit this tipping point of automation, we realized, wait, before you automate anymore, we actually need to stop and start thinking about quality.
Liz Tsai:
And then that's where we started building out additional, almost like validator models throughout our workflows that would then take that and say, okay, well, let's just double check. This is the reply that either the automation or the agent wants to send. Please make sure that it's relevant based on what was said. It's compliant with the policy and processes. And then if the message is saying a replacement was generated, tap the shopify integration and make sure a replacement was actually generated. So a little bit of both. Our current view is that the quality monitoring is almost step zero. Before you start to think about using a bunch of different automation solutions, you really want to make sure that your entire customer support program is instrumented for real time visibility.
Demetrios:
So I do like this step zero of before you do anything, before you're even touching and trying to bring automation to it, let's just monitor what's happening right now. What are the baseline metrics that we can go off of? And then you can say, all right, well, this could be better. It seems like there's bottlenecks here. It seems like there is things that continue to happen in this part of the business, or whatever it may be, or with this task specifically, that's where we can try and bring some kind of better methodology, whether that's automation or just having another human in the loop or having something better happening there so you're able to see the blind spots. And I do think that one of the key things that you said is it doesn't really matter how you fix things. You just want to make sure that you're paying attention to the metrics and that you're fixing them or you're getting whatever can help you to hit those metrics, whether it's automation or bringing more firepower into the equation with humans. Then use that right and figure out a way to make that work. So what does this like layer of just monitoring look like on the business and specifically on the customer support side of things.
Demetrios:
How do you go about monitoring and then how do you go about suggesting upgrades?
Liz Tsai:
I love that. And it's really that what, everything you said was just this focus first on understanding, right, the current data of the CX program, the layout, all the different pieces, because then that allows you to one zoom out and say, okay, where are my current gaps? If I'm going to roll out an automation or AI solution, where is it going to have the most impact? And then because you have all the instrumentation, once you roll it out, it also allows you to monitor it and see what's happening. What does that mean tactically? Which I think is your question here is we essentially integrate, HiQ integrates in directly with customers support CRM systems like Zendesk, customers salesforce, gladly gorgeous. And we essentially write along. So you integrate your CRM and then we write along. And as customers write in or contact the company, we're categorizing what the customers are writing in about. And then as the brand sends replies, whether they're automated or human replies, we are essentially monitoring the quality on that, both of the conversational layers. So you, you know, as a generally, as a general human, is this a good quality conversation? But then also on a policy and compliance level, is what's being said in line with company policies? And is what's being said actually being actioned in the backend systems? And then we also predict in real time customer satisfaction.
Liz Tsai:
Right. So what your customers are writing in about the quality of replies the company's producing back and then how the customers feel about that. And when we talk to companies that are starting to think about what do we automate first in our customer support plan. This also sort of lays that groundwork because it allows you to have visibility into what are the biggest contact categories that maybe we are currently really good at or could improve on that are maybe either making our customers really happy or really upset because that then allows you to map, well, what are my biggest categories where there's a lot of room for improvement and then we can do the deep dive of, of those big categories that need improvement. Where are steps that you can systematically automate and then also use this as a tool to then make sure that you're accomplishing your quality and satisfaction goal.
Demetrios:
And it does feel like when you are monitoring and you're going for that understanding first, what you're going to encounter with customers is that not one system looks the same. And so you have to understand the system first before you can actually make recommendations. Have you seen that you can productize these upgrades, if you will, or is it something that each one you have to go in and work with the specific system to make a very custom solution?
Liz Tsai:
So we found that we can actually productize this pretty well because any sort of automation we find is sort of a combination of role based black and white automation and then models that you could pull in when things are a little bit fuzzy. A good example of maybe a productizable, scalable version of this is you might have a conversation where the customer is asking for a refund and the company is saying, yes, we price adjusted for you. Here's a refund that you can use an LLM to understand. What is it asking for? It's asking for a refund. And then you're tapping a sort of black and white integration that you've built into shopify to say, okay, well, was a refund also processed? So for us, it's sort of the black and white of building out all the integrations, building out all the hooks. Right. And then allowing models and LLMs and some generative aspects to help navigate and make sense of that.
Demetrios:
Oh, fascinating. Yeah. So I think I see and understand what you're saying is that I imagine a lot of times just having the integrations might be an upgrade. But then if you throw LLMs and you throw generative models into the mix, they're understanding much more as this data is flowing from point a to point b, and they can help monitor that and potentially intercept or make it better, upgrade it as it goes across.
Liz Tsai:
I mean, the other bit here, which I think you touch on here, is also that we think a lot about risk management and sort of the ROI versus the risk. Because one way of perhaps building customer service automation is to integrate everything in, feed the model, your support docs, and say, go for it. Customers saying this, you have the ability to process a refund and shopify or replacement or whatever, go for it. That's a high risk application. A lot of things like price adjustments and whether or not a customer qualifies for them, that's a very deterministic thing. So using a probabilistic model to do that is not only a waste of compute, but also kind of scary, because the amount of price adjustment a customer needs is not probabilistic, it's deterministic. But when you're thinking about something like observability in QA, right. Much, much safer, you can let that model go live because you're not actioning things.
Liz Tsai:
You're really using it to give you real time visibility. In a way where instead of trying to prevent downside when you're trying to, when you set an automation live on your customer, you're kind of trying to prevent bad things from happening here, you're leveraging a lot of those same models and access to instead catch things and look for opportunities to improve. So you can be a lot more, I think, liberal with what you allow a model to do because it's functioning in observability rather than in direct customer actions.
Demetrios:
And this may be getting a bit into the weeds too much, but I had to ask, because I was thinking about that, the deterministic versus non deterministic modes that you get where you can have a big problem like the one that we've been seeing, it's like companies end up in the headlines for all the wrong reasons, whether it is Air Canada not giving refunds, or it's the Chevy dealer who's selling Chevy for $1 or talking about how much better Tesla is than Chevy. Those are reasons that you don't want to have AI interacting with the end user, right?
Liz Tsai:
Yep.
Demetrios:
So it is good to be thinking about that. But there are, as you were saying, times when it's not like there's a gray area. And so having these generative models produce the response to the customer or to anybody, it's not the best, it's not the hammer that you need, or it's not the tool that you need to make that happen. And so are you using things like knowledge graphs in those areas? Are you trying to just give, like a relational database answer that is pulled out? And how does that look?
Liz Tsai:
So the way that our workflows are structured is we have a lot of nodes that are deterministic, nodes that tap other tools, right? So, I mean, I guess the canonical example here is maybe that LLMs are really bad at arithmetic, right?
Demetrios:
Yeah.
Liz Tsai:
Right. Because arithmetic is a deterministic task, not a probabilistic task. What you actually needed to do is recognize that what you're trying to do here is a deterministic task and like, tap the calculator node, right. And then accept that back in as part of your broader action. So we think a lot about it in that sense. Right. The step, the task is not solve current task using any sort of model. The task is actually solve the current task by breaking it apart into its constituents.
Liz Tsai:
Components. Figure out which components are deterministic, which ones are fuzzy. Fuzzy nodes are nodes where there are many correct answers and then route them to the correct tools. That tends to be how we think about it. So calculating a price adjustment deterministic task, that's a calculator, but then communicating that back to the customer or determining whether or not it was a good communication. There are many acceptable ways to tell a customer that they got a price adjustment.
Demetrios:
Oh, fascinating. Okay, I like it. And now going back to the monitoring piece, because I do really like this idea of, you get to know before the customer knows when the automations aren't working as well. How does that work out? How do you flag things? And, like, do you shut down the system so that if there is any doubt that things are going off the rails, you. You don't let it go off the rails first?
Liz Tsai:
Yeah. So we built haiku for ourselves. We essentially had that. So maybe to riff off the example you gave there, where if for whatever reason, you traverse the workflow and you've generated a reply that doesn't follow policy, that's, I don't know, selling cars for a dollar. Right. We would have an additional validation node between that and then actually sending the reply back through Zendesk that basically says, hey, this is a reply that our body sent. Please make sure it's in compliance with our policies. Right.
Liz Tsai:
And if no, that's a great opportunity to, like, flush it back and send it to a human for review before you send it out for HIQ. When we work with outside clients, it does still go out, but as soon as the reply is sent out, HIQ runs and we'll check to make sure it's a good conversation and also grade it on policy and compliance. So it's sort of like having an AI QA analyst sitting next to each one of your agents to make sure they're doing a good job. So you can catch things as soon as they go out if it's not a compliant reply.
Demetrios:
Yeah, because I imagine that there is a bit of a trade off between getting something out, getting out that response, and speaking to someone as quickly as possible or appeasing their whatever their complaint is when they're coming into the company versus making sure that it is the highest quality and it is following everything that it needs to follow.
Liz Tsai:
Yeah, that's fair. And I think that's what we sort of think about as the trade off between QA and customer satisfaction at times. Right. So we actually very much predict both because QA and CSAT don't necessarily move in sync. Right. You can, like, hand our refunds left and right. You won't have a very good QA score, but you might have a really great satisfaction score. And vice versa.
Liz Tsai:
But when it comes to that trade off, as teams start to integrate more and more automation solutions, that allows them to already greatly speed up the speed at which they're sending replies out. And so that then brings the opportunity of, okay, well, given that we can have the ability to go so quickly, where do we put our human agents to make the most of their time and ability? Right. It's like we have 50 customer service agents. Where is their time best spent? It's probably not best spent processing routine back office tasks. It's probably best spent when a model flags it up and goes, hey, this conversation is starting to go south, or hey, this conversation is getting kind of hairy. Can you, can you plop in there? Right. So, yes, there's that trade off of time, but I think it's much more about if you treat your customer support agents, really, as experts, where can you best leverage their time?
Demetrios:
Yeah, I've been fascinated with this question for years, and I think that is probably one of the most interesting pieces, is if you are going to be automating things, and if you are going to have the ability to, let's just say, let AI do the whole workflow end to end, where are you putting the human in the loop to make sure that the quality is high? And to, and I do like this other piece where you're saying, well, you know, if you can automate many different parts of this, then you're compressing the total time down. And so it's okay if you put a human in two or three times in this loop, because they're still going to be doing something faster than if a human were to do the whole thing.
Liz Tsai:
Definitely. And to add onto that is there's also, we realized this firsthand, but humans are very human, so you might assume that it's always better to have a human in the loop at a specific node. But what we found was that there's actually like an error rate below which it's better to not have the human. So imagine you're a human and you're supervising this one automation node, right? If that node requires you to intervene 50% of the time, great, that's a great use of you. Right? But if the automation is good enough that you only need to intervene 5% of the time, you're not going to catch that 5%. You'll actually get so used to just rubber stamping it and clicking approve that you don't catch the 5% error. So we realized as we went through that there's actually like a threshold where if, like, less than 10% is actually wrong. You need to introduce a second layer of automation because you can't present that to a human.
Liz Tsai:
They will just rubber stamp it.
Demetrios:
Wow, that is such a cool learning. And I see myself in that human category. If I'm getting 95% of the things that I'm seeing are going to just be, yeah, okay, looks good to me. Then I probably am going to say, everything looks good to me.
Liz Tsai:
Yeah, you're human, right? You just zone out a little bit. And that's where I think generative AI tends to be best, because they don't have things like empathy and approval fatigue.
Demetrios:
Yeah. Well, Liz, this has been absolutely fascinating talking to you. I appreciate you coming on here and really going deep into the weeds on what you're doing at Hiq and how you're leveraging AI to monitor. You're also making sure that in these contact centers and in these, like, customer support use cases, your customers are getting the most out of each interaction. And so it's cool to see I'm rooting for you and want to make sure that next time I reach out to a company, I hope they are using your tools so that it can ensure a good experience from my end.
Liz Tsai:
Well, Demetrios, thank you so much for having me. And yeah, we're definitely excited. It's all about QA at the speed of automation.
Demetrios:
There we go. So if anybody wants to get in touch or start using HiQ, where should they go to find you?
Liz Tsai:
Hiq, CX, reach out. There's a demo video on there, or you can reach out to our team. We would love to, love to chat and hear more about how you're automating and thinking about automating QA.
Demetrios:
And I will just mention this for anybody that is an avid podcast listener. You all do a podcast weekly on LinkedIn?
Liz Tsai:
Yep. Every other Wednesday on LinkedIn we do a plain speak where we talk to mostly CX leaders, not so much at just the conceptual level, but really deep down into the weeds, how they're leveraging AI immediately to make a difference for their teams and their customers. So join us. You have a few minutes on Wednesdays.
Demetrios:
Excellent. Yeah, we'll leave a link to that in the show notes. And this has been awesome. Thank you.
Liz Tsai:
Thank you so much.