How good, really, is AI at predicting the stock market?
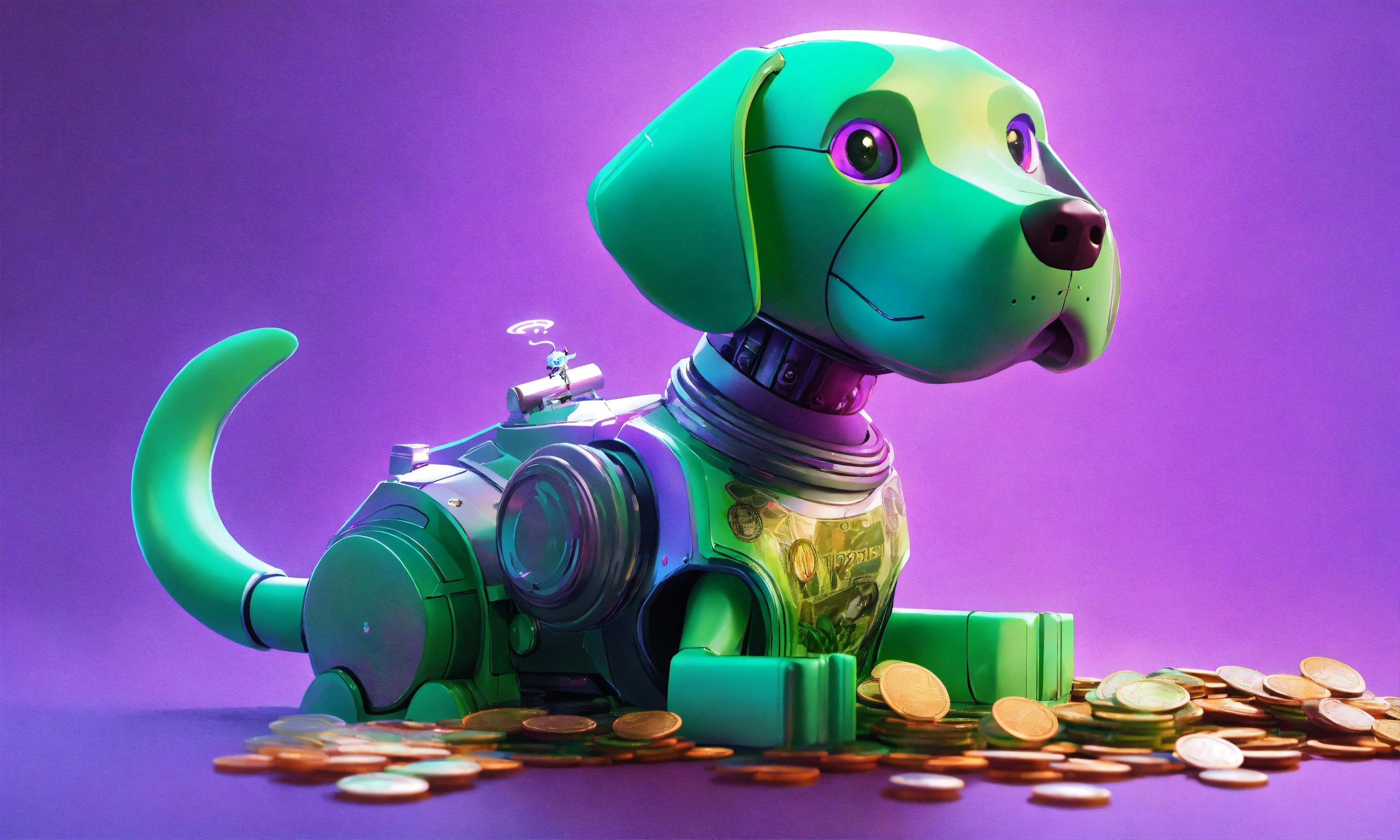
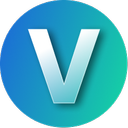
For the past year or two, ChatGPT has dominated media headlines, covering everything from its potential effect on the creative writing industry to the rise of jobs related to prompt engineering. A less-covered topic is the potential use of ChatGPT in financial analysis, specifically predicting stock market movements. Researchers from University of Florida (Go Gators!) discovered that ChatGPT was successful in predicting next-day stock performance based on over 50,000 financial news headlines, with a strong positive correlation between higher scores from ChatGPT and better returns. Recently, Bloomberg itself joined in on the wave with its creation of Bloomberg GPT, an LLM trained on a wide range of financial data that intends to support NLP tasks within the financial industry.
Despite this recent influx of news, NLP is not new to the financial analysis world. One of the earliest uses of NLP in financial report analysis was a methodology developed to evaluate non-quantitative data, or narrative data, in financial disclosures and other financial texts to improve accounting research in 1984. Later on, a daily stock market forecast was developed from textual web data in 1998. There was a general lack of development during the 2000s because NLP techniques at the time, like bag-of-words, required texts to be free of “noise” and to be more structured. Furthermore, word order is not considered in bag-of-words; for example, “Meta has gaining advantages on Amazon” gives the same result as “Amazon has gaining advantages on Amazon
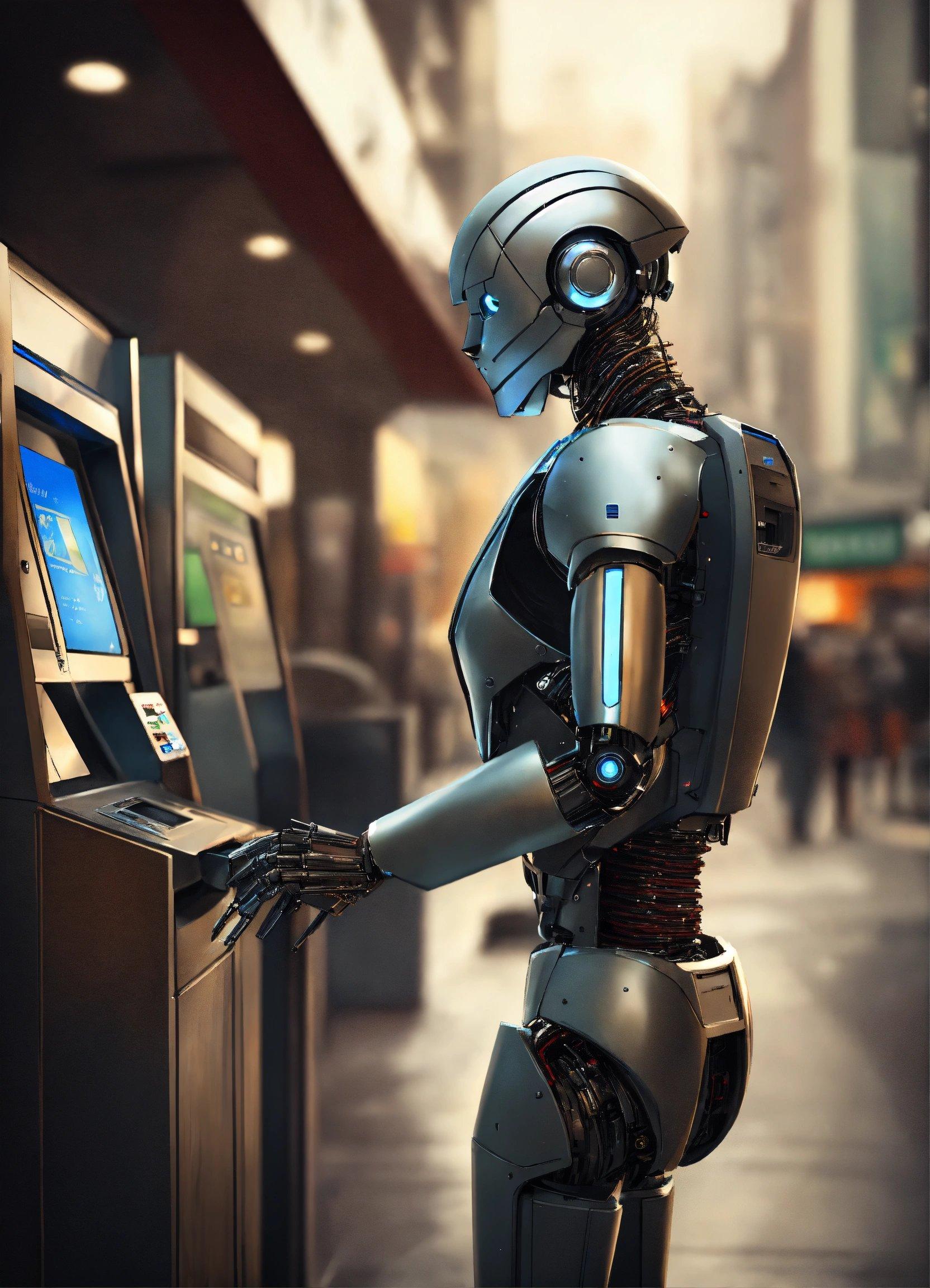
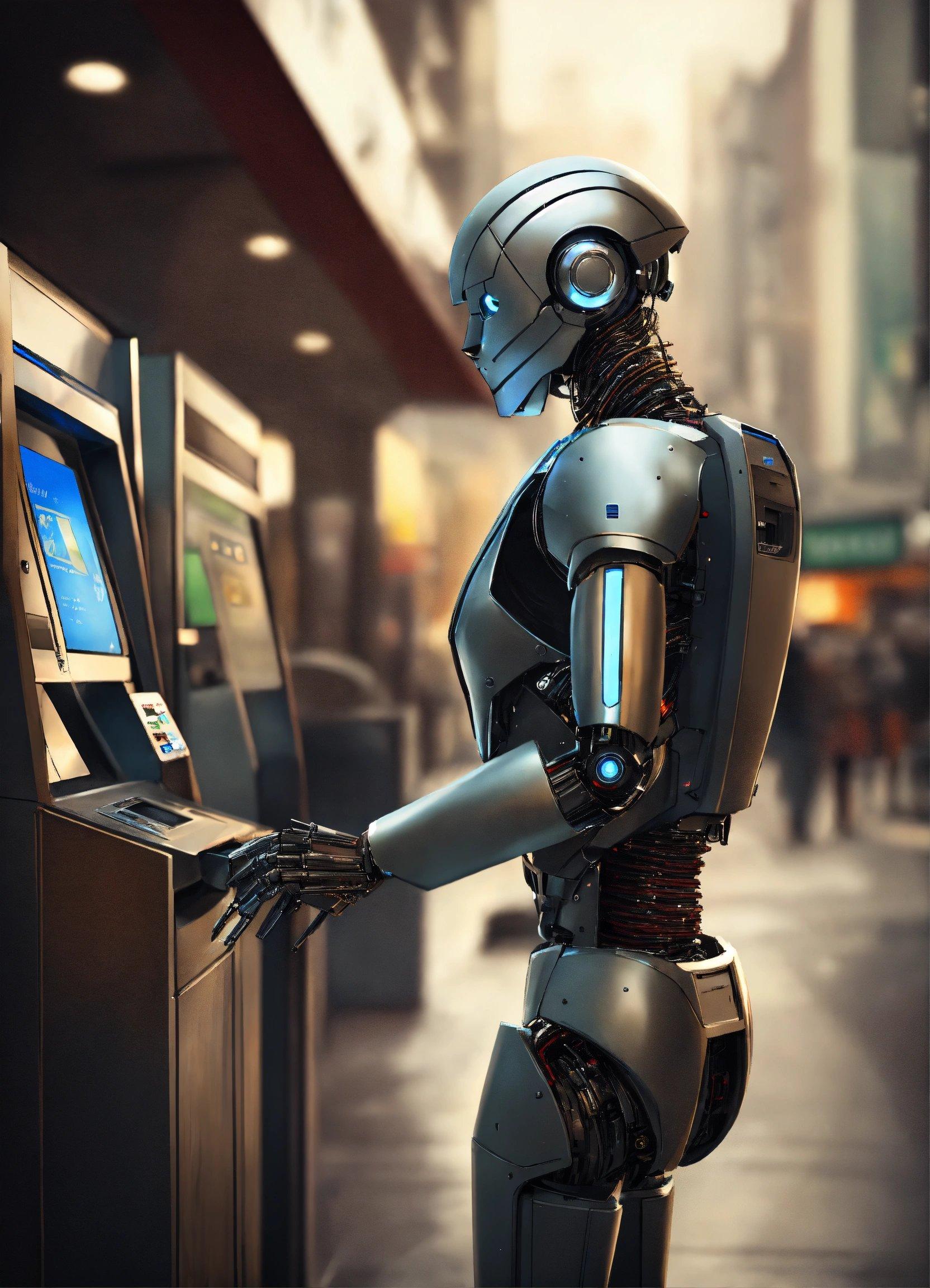
However, in the early 2010s, there was a large influx in NLP analysis on financial markets due to the popularity of social media. Social media came with much more real-time user data and the content itself was rather limited, an example would be the character limits on Twitters. Researchers had a large focus on developing new methods to analyze this new data which brought about more sophisticated techniques that processed words at a human-level accuracy.
Now, sentiment analysis, which includes smaller NLP subtasks like subjectivity and sarcasm detection, plays a large role in many current NLP algorithms. Sentiment analysis is an NLP technique used to classify text by identifying if the author has negative or positive emotions about the subject of the text. For example, a model could be trained on negative and positive Amazon reviews.
There are three highly popular approaches currently that use NLP in portfolio construction and risk modeling. One of them, Loughran-McDonald, continues to use bag-of-words in its analysis. The technique utilizes sentiment-labeled words to identify the tone of a financial text; this was developed after University of Notre Dame researchers Tim Loughran and Bill McDonald recognized that a majority of the words identified by the Harvard dictionary as negative were not negative within financial contexts. The dictionary they use is continually updated as new words enter finance. Another approach is Google’s FinBERT. FinBERT is based on a filtered version of the Reuters TRC2 financial corpus which contains 1.8 million articles published between 2008 and 2010 and is trained for sentiment on a Financial PhraseBank, with words labeled by finance professionals based on how they thought such words would affect stock market prices.
The final approach is Alexandria Technology whose original functionality classified DNA sequences. Alexandria’s language model determines which words appear together in the text and translates these combinations into larger concepts. After the language model is built up, it is then trained for sentiment analysis with sentences from earning calls.
Financial forecasting itself covers a wide range of topics, yet a large amount of studies tend to focus on stock market and foreign exchange rate prediction due to the complexity of most financial data and the difficulty of obtaining information such as corporate financial statements. As evident by the datasets the three approaches mentioned above use, much data originates from financial periodicals and news.
Because of the complexity of financial data and the amount of noise in the data, skeptics debate whether or not much of financial forecasting can be accurately modeled. In general language, there are clear grammar structures and general rules for phrasing but there are no formal rules for how stocks can interact with each other; for example, a pandemic that could plummet stock prices cannot be predicted by a forecasting model because humans themselves cannot accurately predict the date of a pandemic.
Furthermore, the field is still in its infancy. While there is an improvement in using expert-picked or hand-picked keywords to associate emotions with specific financial terms, these still possess biases pending the perspective of the financial expert themselves. Most of the work in financial forecasting does not occur in academia but rather in financial firms themselves who will rarely publish their work. For example, Renaissance Technologies generated an annual return of 66% from 1988 to 2018, outcompeting others like Warren Buffett, but it is uncertain how they did so.
With continued fine tuning and improved dataset labeling by a variety of financial experts, it is likely that NLP can play a large role in stock market predictions and international foreign exchange rates especially within financial firms due to the large amount of public data available. Furthermore trading floors are already automated to an extent; thus, it is natural for chatbots to continue playing a role within this space. However, it is unclear if these models can “break into” other aspects of the financial sector, such as VC investing in early-stage startups because of lack of data and the amount of factors that would have to be considered.
Unlock language AI at scale with an API call.
Get conversational intelligence with transcription and understanding on the world's best speech AI platform.