Introducing Nova-2: The Fastest, Most Accurate Speech-to-Text API

Josh Fox
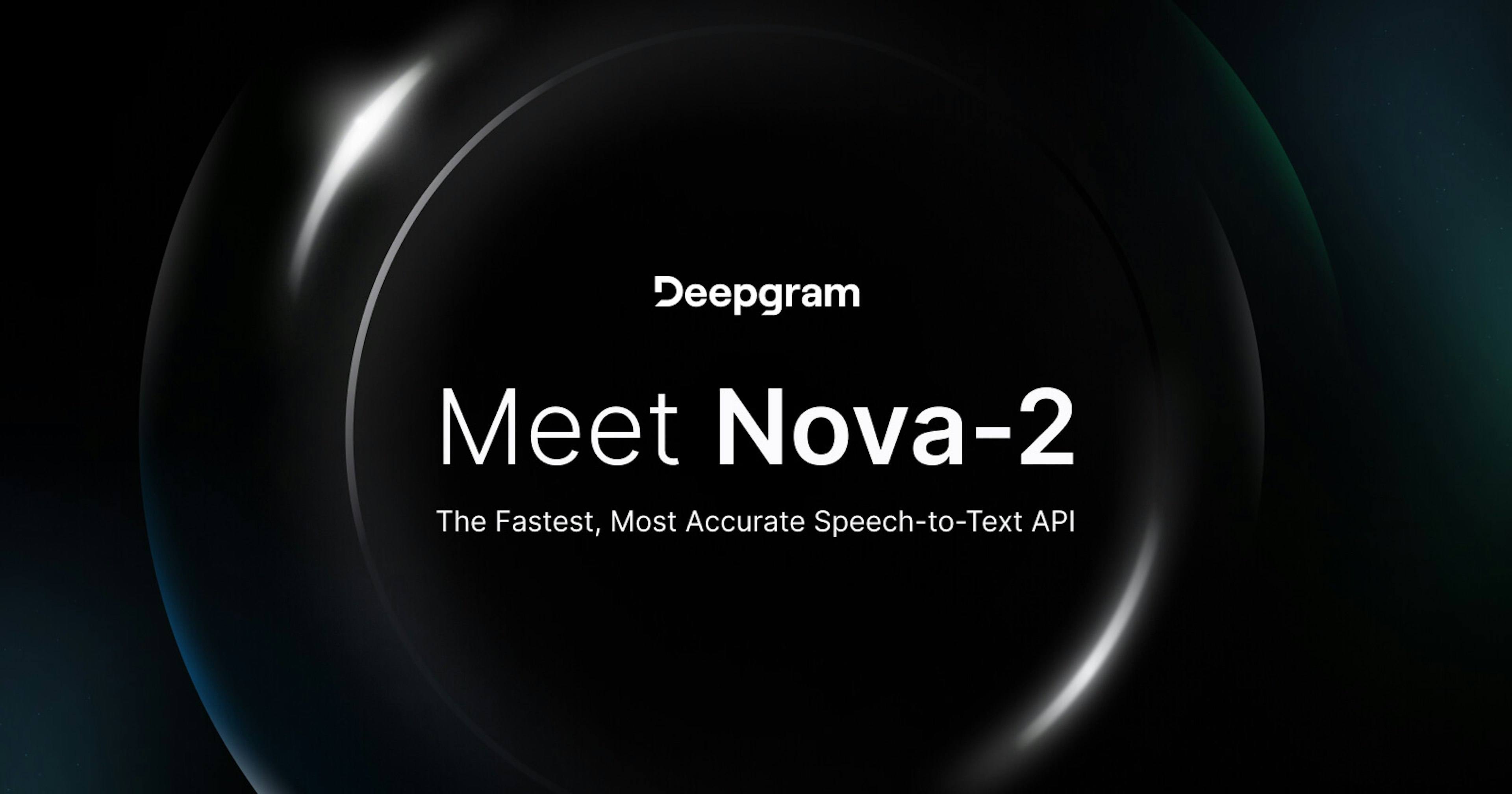
tl;dr:
Our next-gen speech-to-text model, Nova-2, outperforms all alternatives in terms of accuracy, speed, and cost (starting at $0.0043/min), and we have the benchmarks to prove it.
Nova-2 is 18% more accurate than our previous Nova model and offers a 36% relative WER improvement over OpenAI Whisper (large).
Contact us for early access to Nova-2 today or you can immediately try out all of our models and features in our API Playground!
Meet Deepgram Nova-2: Raising the Bar for Speech-to-Text
We’re excited to announce Deepgram Nova-2, the most powerful speech-to-text (STT) model in the world, is now available in English (both pre-recorded and streaming audio) for early access customers. Compared to leading alternatives, Nova-2 delivers:
An average 30% reduction in word error rate (WER)[1] over competitors for both pre-recorded and real-time transcription
5-40x faster pre-recorded inference time
The same low price as Nova (starting at only $0.0043/min for pre-recorded audio) with 3-5x lower cost than the competition
Since the launch of our initial Nova model (Nova-1) earlier this year, we have been dedicated to delivering enhanced capabilities. These new features encompass improved speaker diarization, smart formatting, filler words support, and our inaugural domain-specific language model for summarization. These additions not only elevate the value we provide to our customers but also underline our commitment to advancing the forefront of language AI.
Furthermore, our model research team has maintained an exceptional level of productivity, upholding our longstanding tradition of relentless improvement in the quest for flawless speech-to-text accuracy and even superhuman transcription performance (refer to Fig. 1). With Nova-2’s word error rates consistently below 10% across domains, we proudly announce the realization of this monumental achievement.
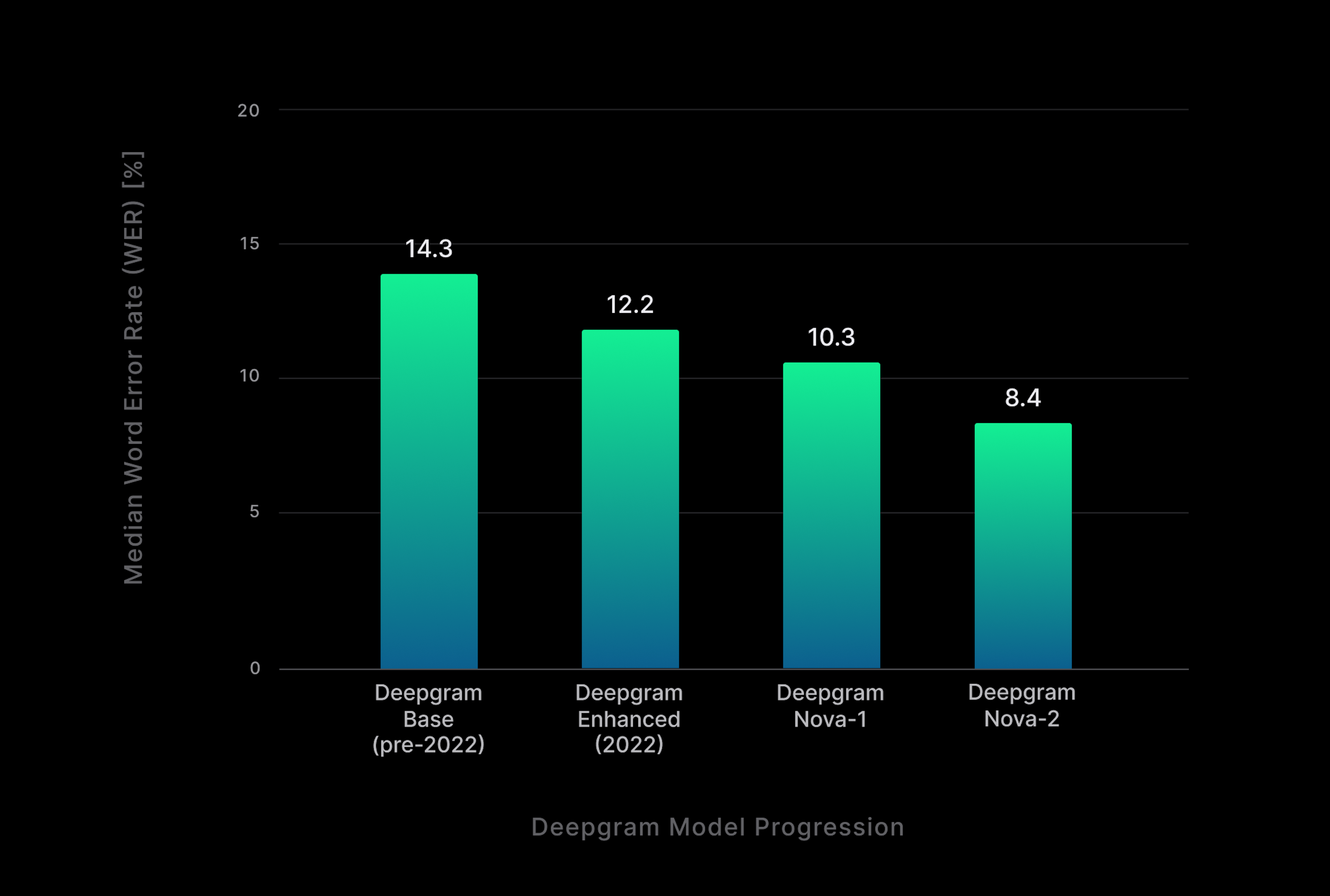
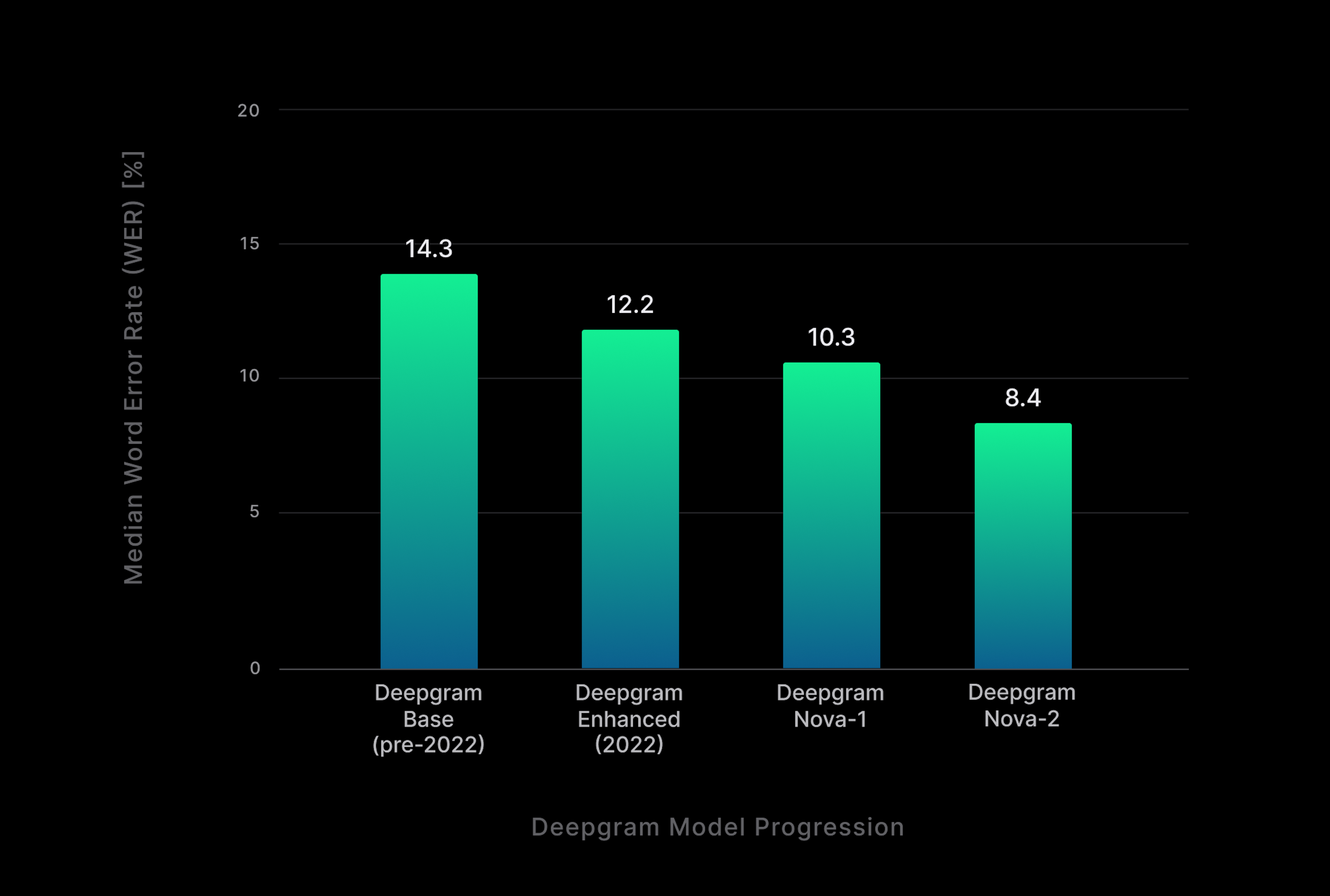
Figure 1: Deepgram model evolution over time. Over the last two years, Deepgram’s word error rate has decreased by more than 40%.
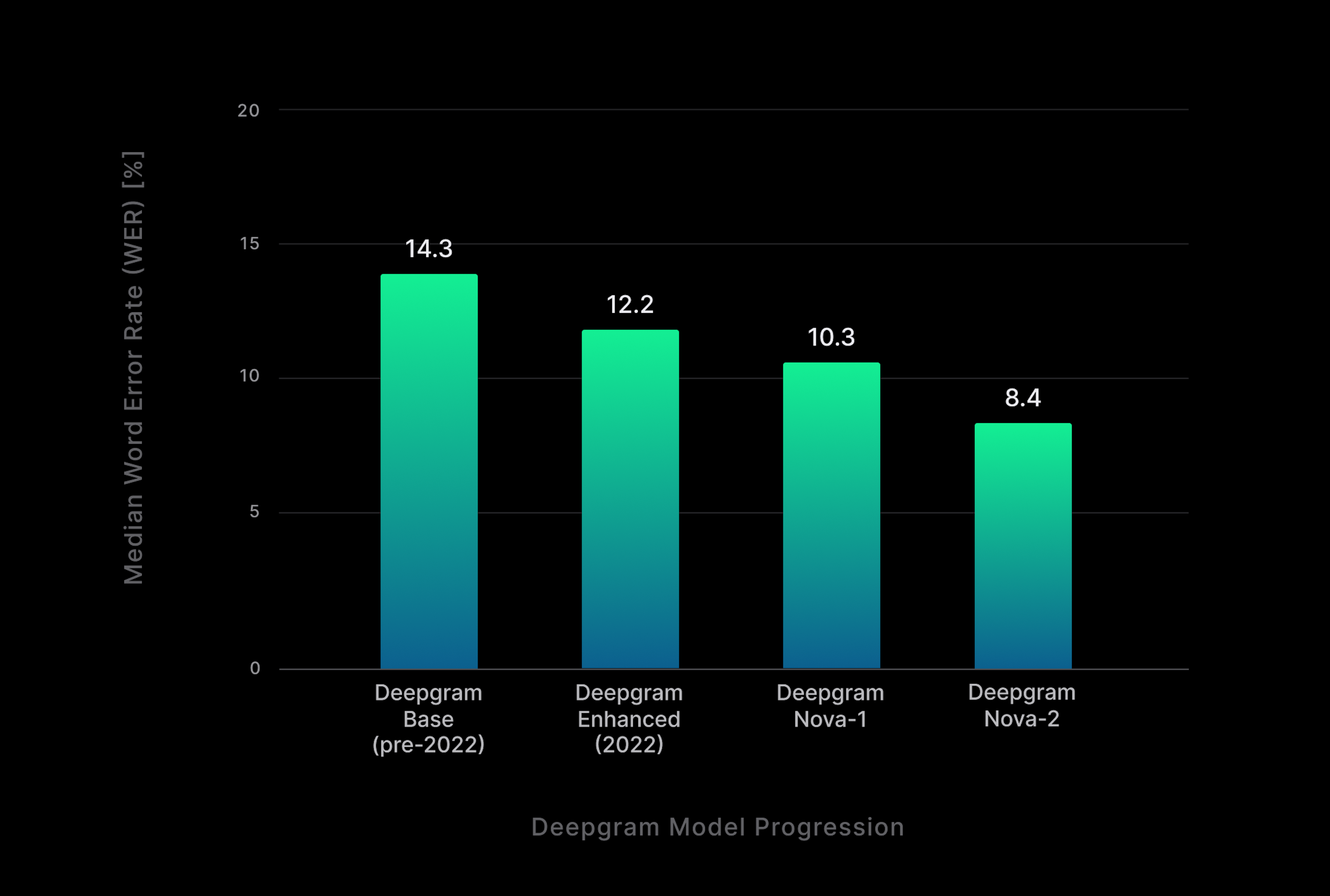
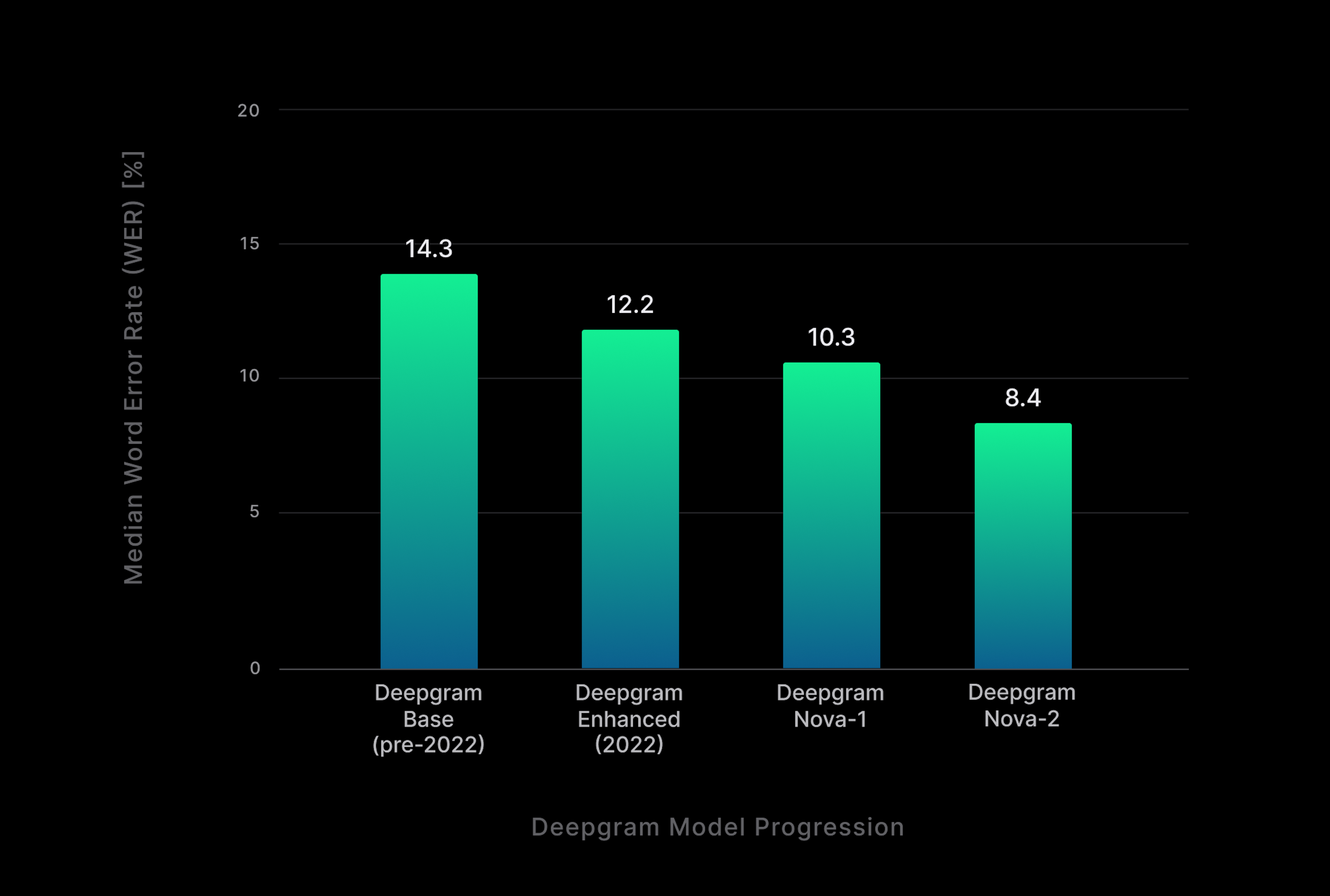
Figure 1: Deepgram model evolution over time. Over the last two years, Deepgram’s word error rate has decreased by more than 40%.
While Nova-1 resulted in a significant improvement in accuracy from prior model architectures, Nova-2 extends those advancements even further across diverse audio domains due to three key factors:
Speech-specific optimizations to the underlying Transformer architecture.
Utilization of advanced data curation techniques, skillfully executed by Deepgram's in-house DataOps team.
Rigorous implementation of a multi-stage training methodology, leveraging a substantial real-world conversational audio dataset.
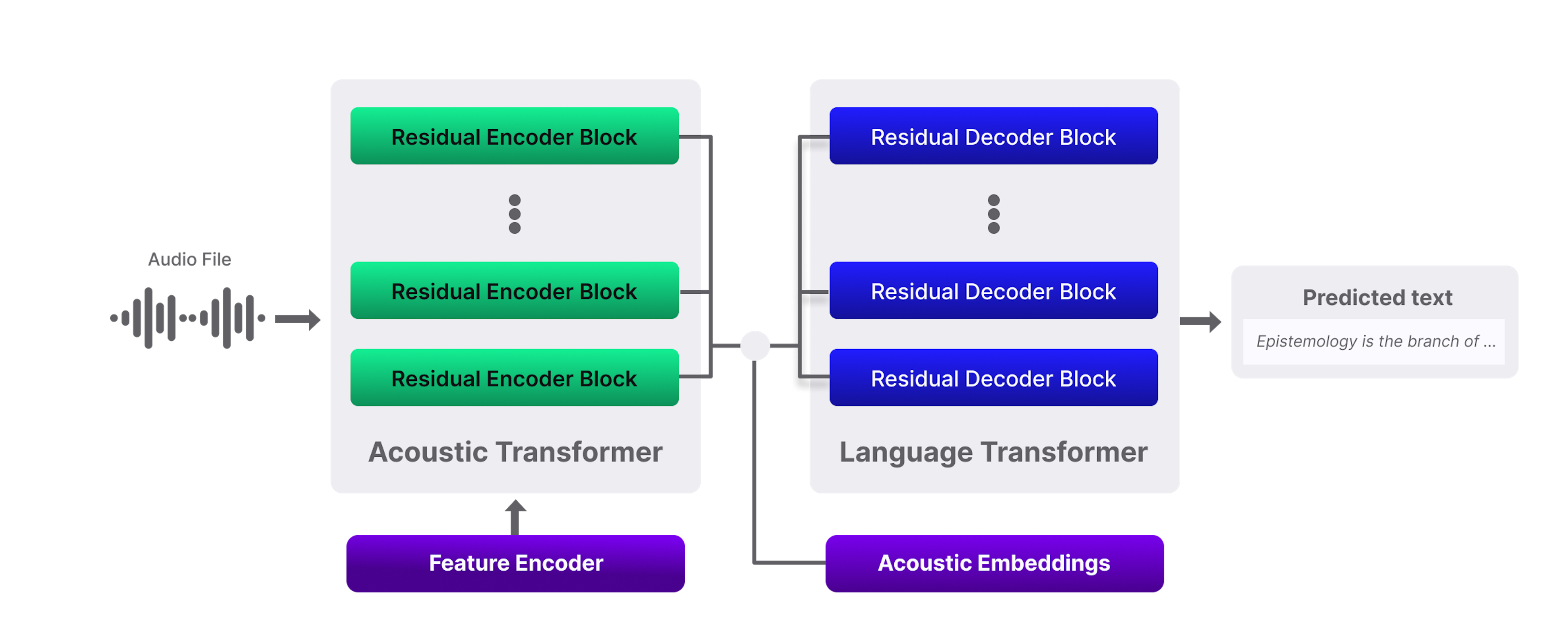
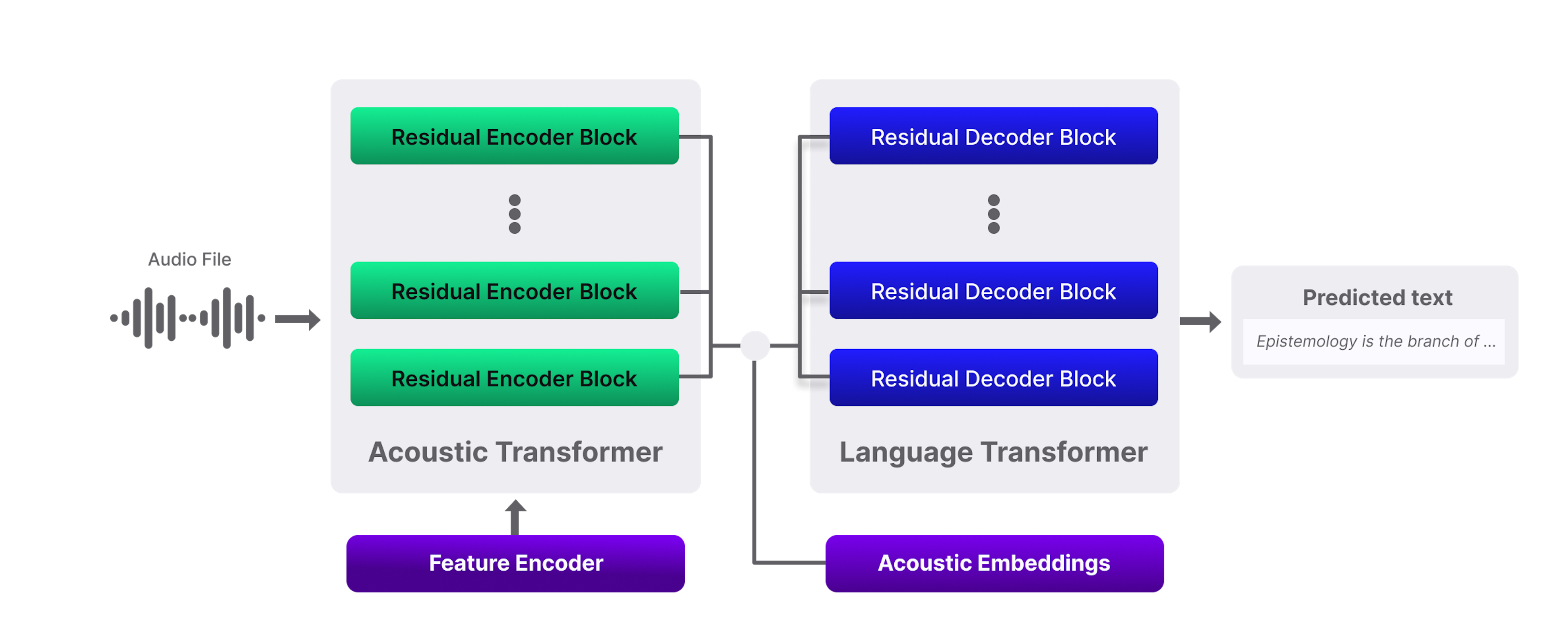
Figure 2: Deepgram Nova-2’s Transformer-based model has been optimized using proprietary architecture characterization algorithms to boost transcription accuracy without compromising inference speed.
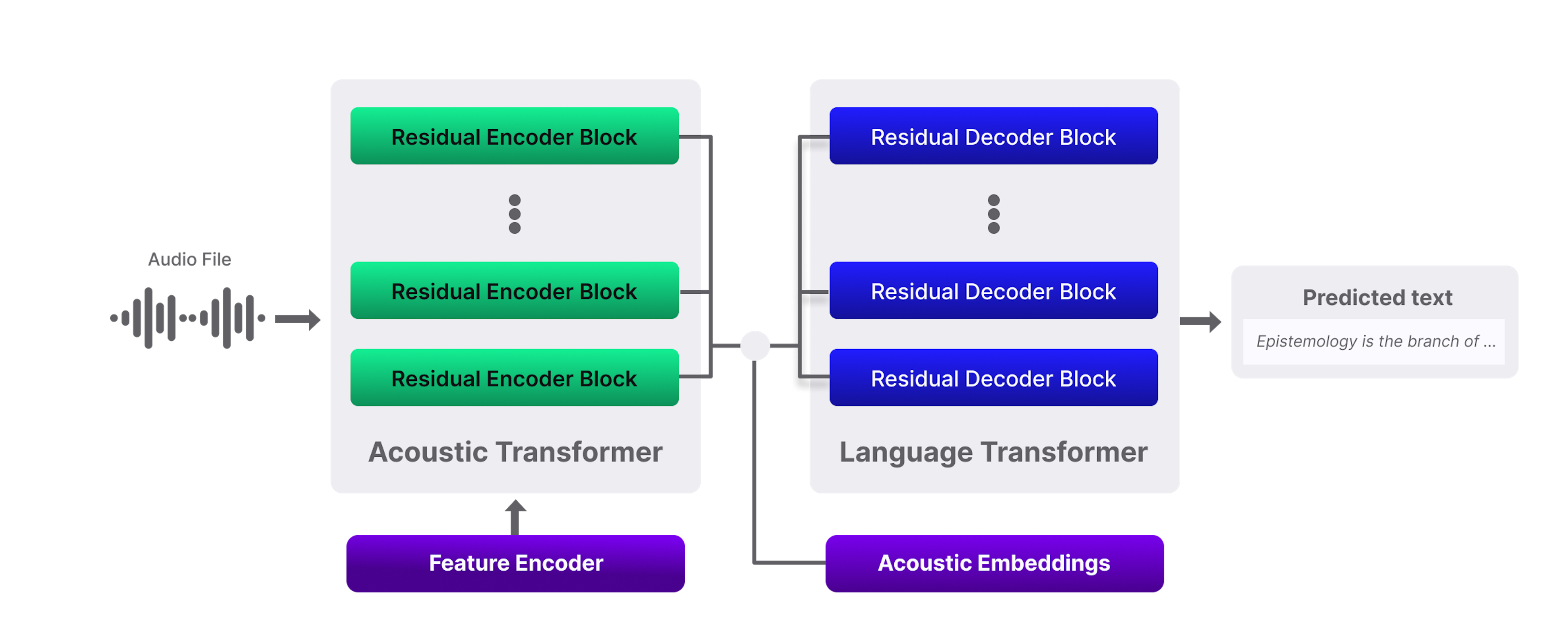
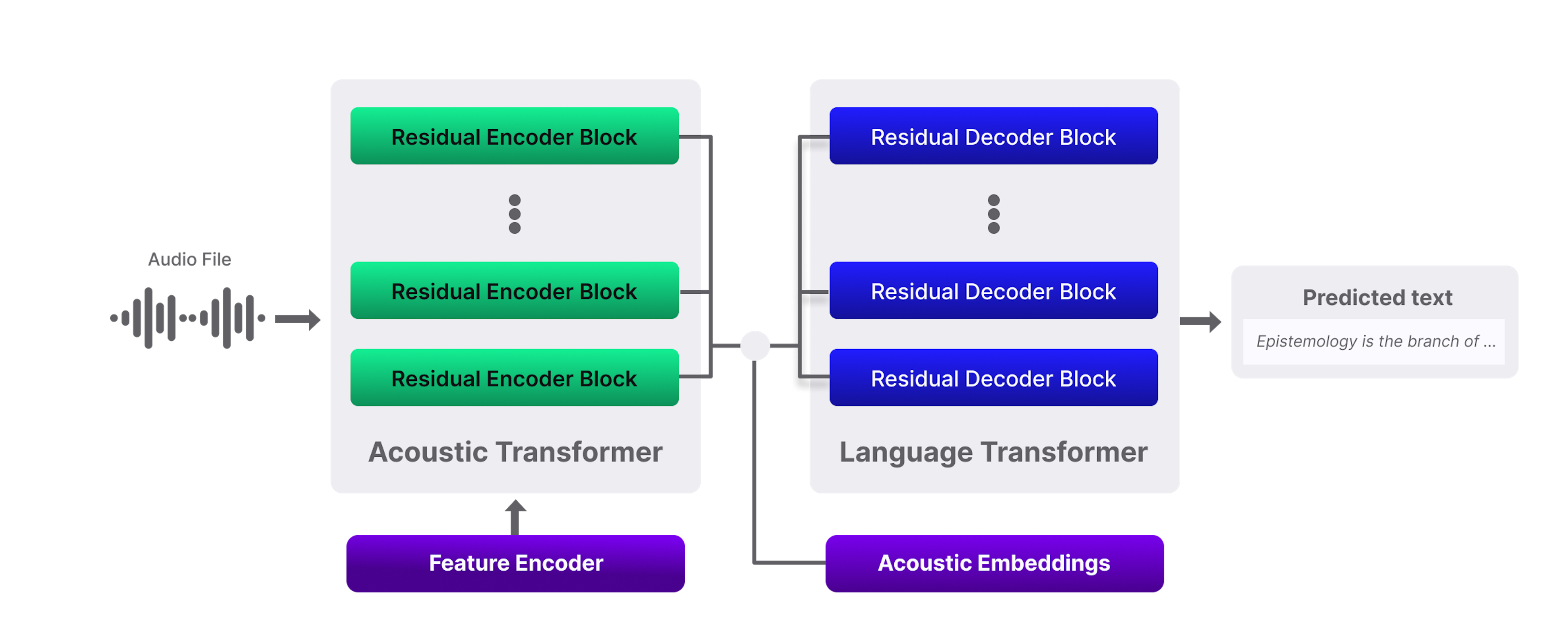
Figure 2: Deepgram Nova-2’s Transformer-based model has been optimized using proprietary architecture characterization algorithms to boost transcription accuracy without compromising inference speed.
Nova-2 employs a novel Transformer-based architecture that offers significant improvements over its predecessor. The result is an 18.4% reduction in word error rate (WER) from Nova-1. Additionally, Nova-2’s architectural enhancements significantly boost accuracy for both pre-recorded and streaming transcription of entities (i.e. proper nouns, alphanumerics, etc.), punctuation, and capitalization.
Smart entity handling is an important aspect of speech-to-text for many applications, improving readability and utility in downstream tasks that depend on accurate formatting of account numbers, company names, and dates/times to support workflow automation. In this context, Nova-2 delivers:
Improved entity accuracy with a 15% relative reduction in entity error rate from Nova-1 on entities overall
22.6% relative improvement in punctuation accuracy over Nova-1
31.4% relative improvement in capitalization error rate compared to Nova-1
Extending upon Nova's groundbreaking training, which spanned over 100 domains and 47 billion tokens, Nova-2 continues to be the deepest-trained automatic speech recognition (ASR) model in the market. Nova-2 was trained in a 2-stage curriculum starting from the largest, most diverse dataset in Deepgram’s history, curated from nearly 6 million resources and incorporating an extensive library of high quality human transcriptions. The result is a new state-of-the-art model capable of superhuman transcription performance that consistently outperforms any other STT model in the market today across a wide range of speech application domains (see benchmarks below).
In simple terms, Nova-2 establishes a new gold standard for ASR performance. Its extensive training on diverse datasets positions it as the most dependable and versatile model in the market. This makes it the perfect choice for a wide range of voice applications that demand both exceptional accuracy and speed across various contexts. Nova-2 also serves as a robust foundation for further customization in specific domains and use cases, allowing for more precise tailoring when necessary.
Comparing Nova-2 Accuracy, Speed, and Cost
Let’s take a detailed look at how Deepgram Nova-2 compares with other speech-to-text models.
Accuracy: 30% lower error rate (WER) than the competition
Our benchmarking methodology for Nova-2 builds upon our previous test suite for Nova. In this iteration, we expanded our assessment by pitting Nova-2 against the latest models for a broader spectrum of competitors, utilizing over 50 hours of human-annotated audio across more than 250 files extracted from real-life scenarios. This encompassed a wide range of audio lengths, diverse accents, varying environments, and subjects.
This stands in contrast to other benchmarks that rely on meticulously curated, pre-cleaned audio data from a limited set of sources, carefully controlled to narrow the scope of test results. This distinction is of paramount importance because the true value of a competitive benchmark lies in its ability to accurately mirror real-world performance, making it more relevant and reliable for assessing practical applications.
Using these datasets, we calculated Nova-2's Word Error Rate (WER) in pre-recorded inference mode and compared it to other prominent models. The results show Nova-2 achieves a median WER of 8.4% for all domains/files tested overall, representing a 16.8% relative error rate improvement over the nearest provider (see Fig. 3) and besting the performance of all tested competitors by an average of 30%. Of particular note is Nova-2’s sizable relative WER improvement (36.4%) over OpenAI’s popular, and most performant, Whisper (large) model.
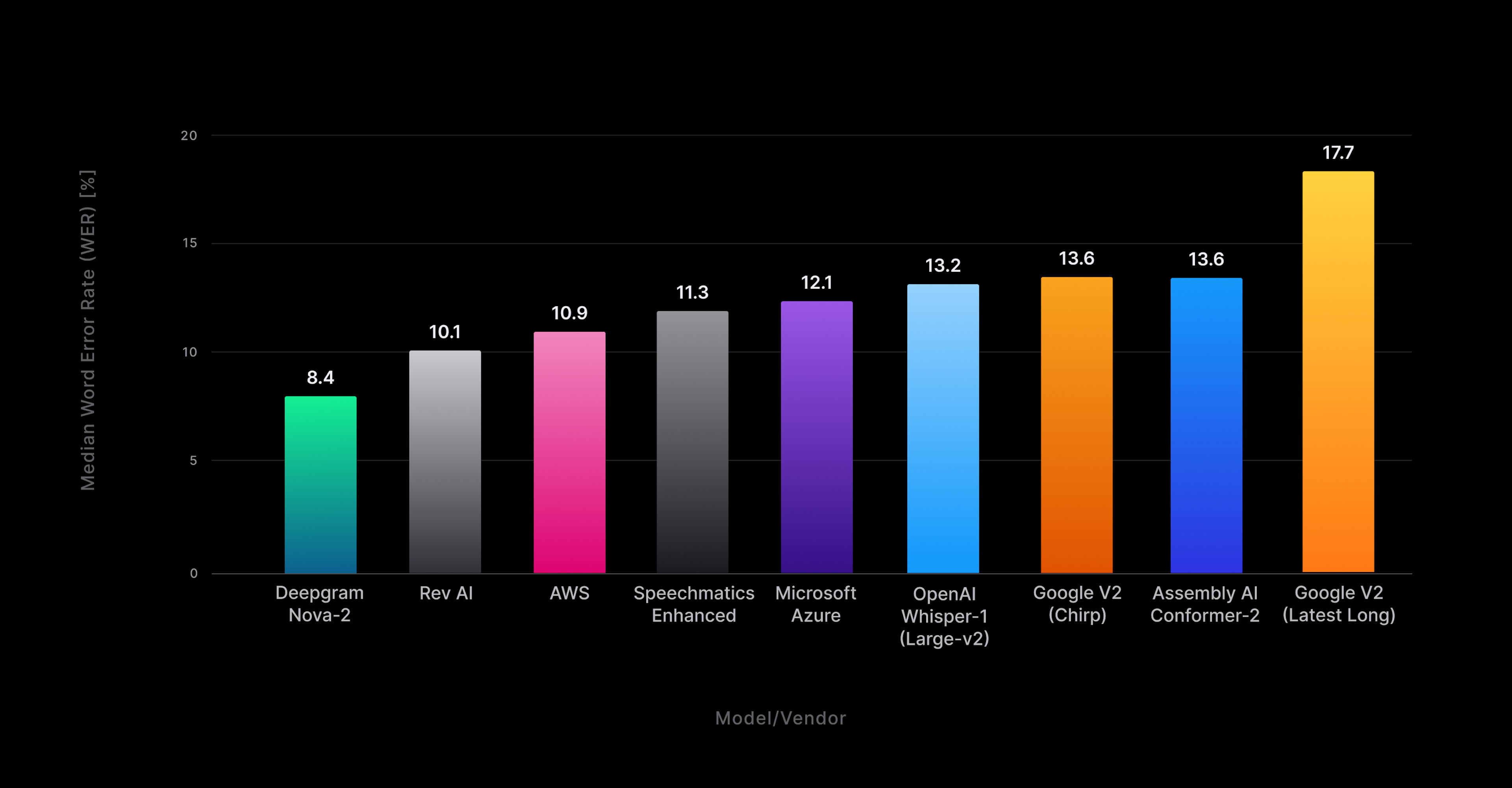
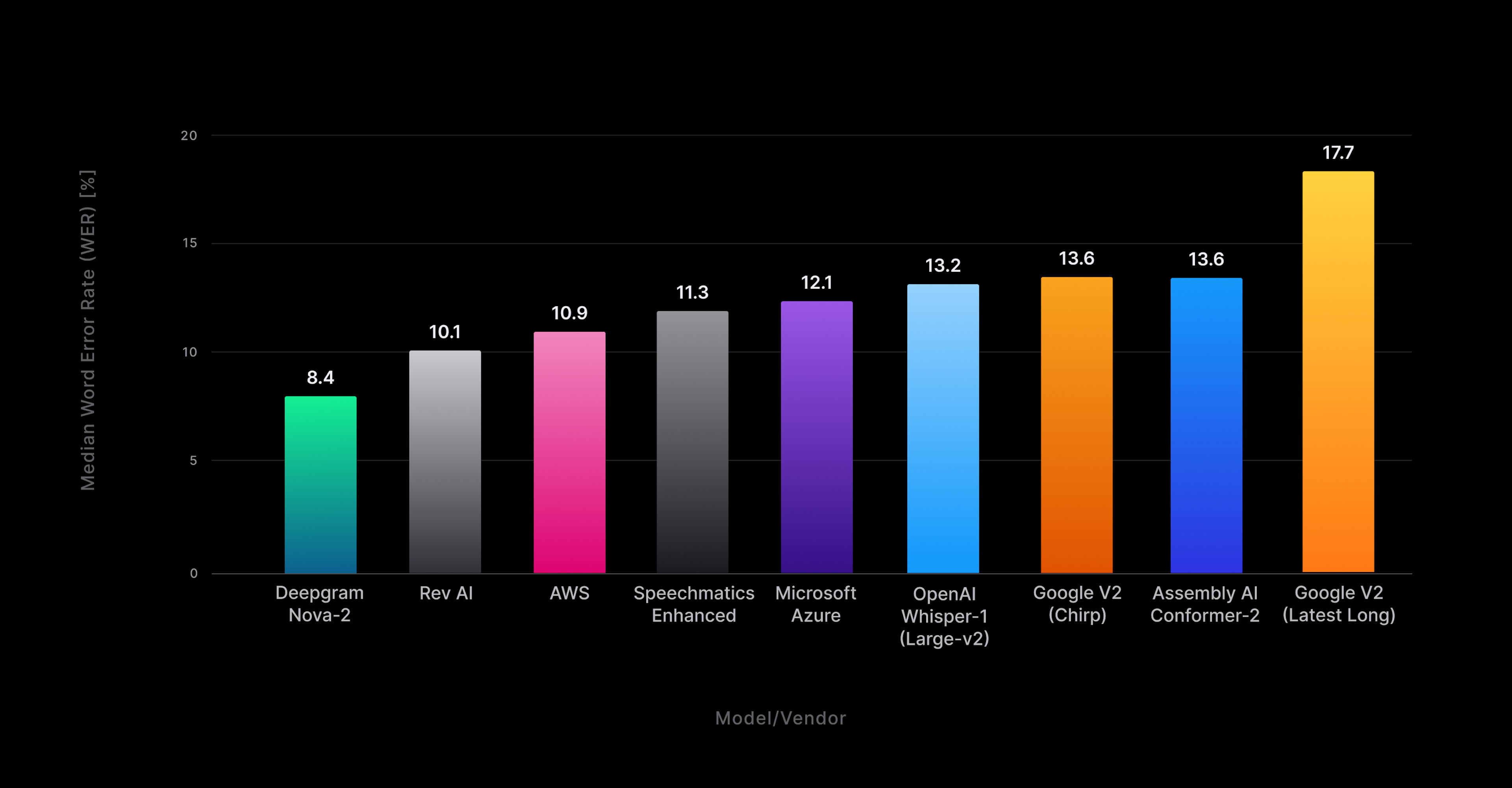
Figure 3: Median file word error rate (WER) for overall aggregate pre-recorded transcription across all audio domains.
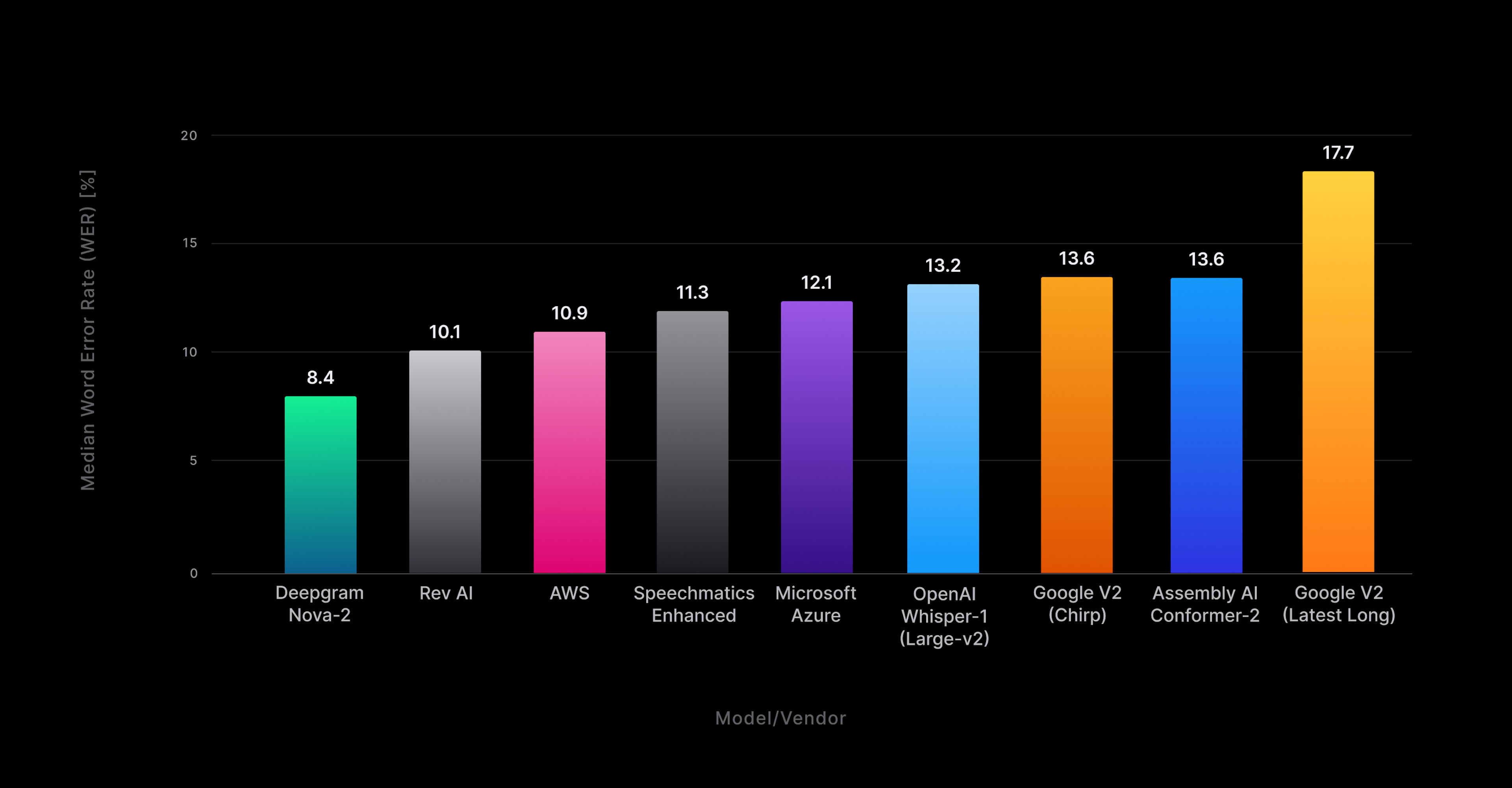
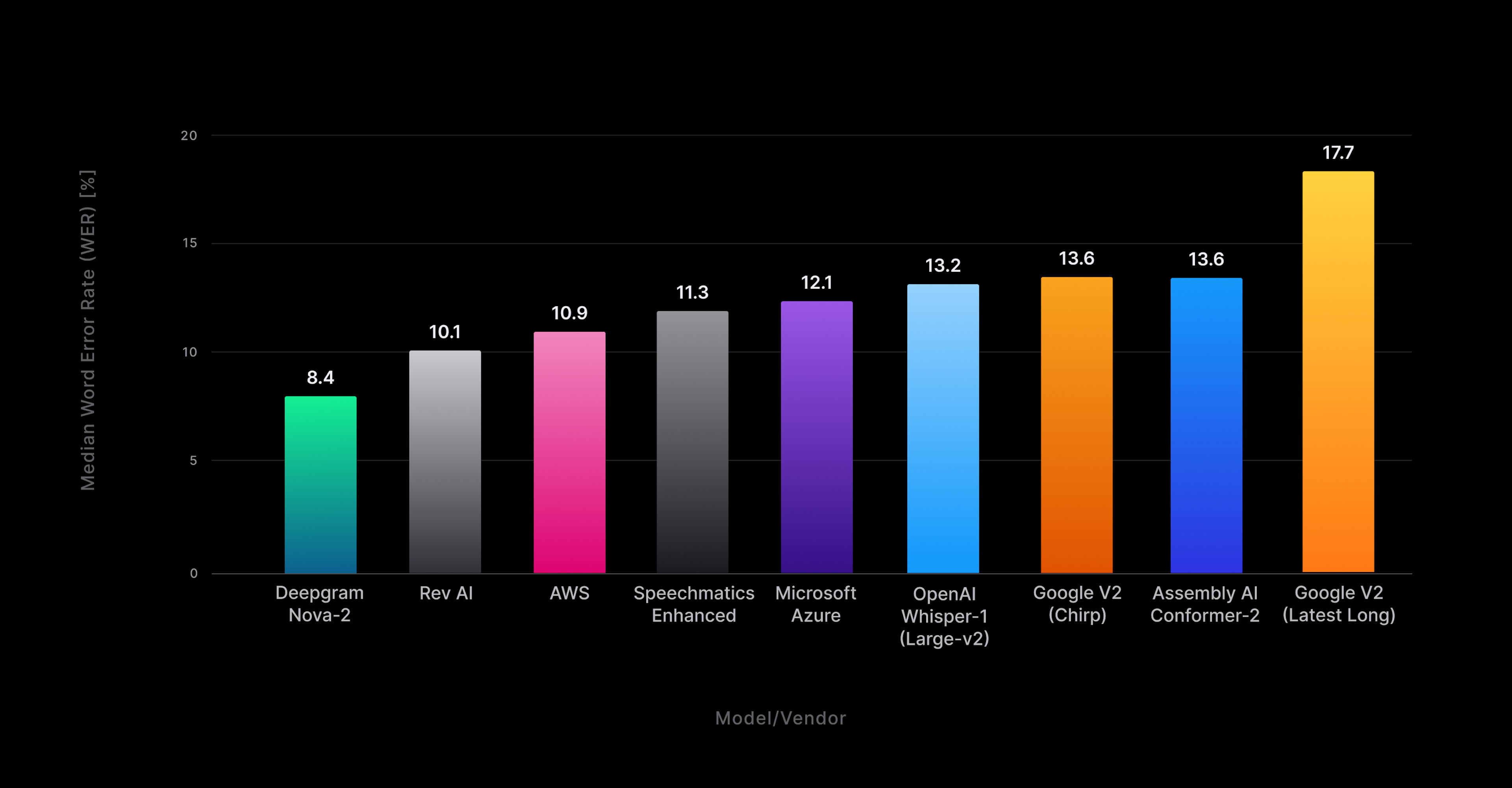
Figure 3: Median file word error rate (WER) for overall aggregate pre-recorded transcription across all audio domains.
More specifically, our benchmarking test set was equally split across four predominant audio domains in the transcription world: podcast, video/media, meeting, and phone call. In domain-specific tests, Nova-2 outperformed all commercial ASR vendors and open-source alternatives, demonstrating exceptional overall accuracy and emerging as the winner within each individual audio domain.
As seen in Fig. 4, Nova-2 displays lower variance than competing offerings as well, with a tighter spread in test results that corresponds with more consistently accurate transcripts in real-world implementations.
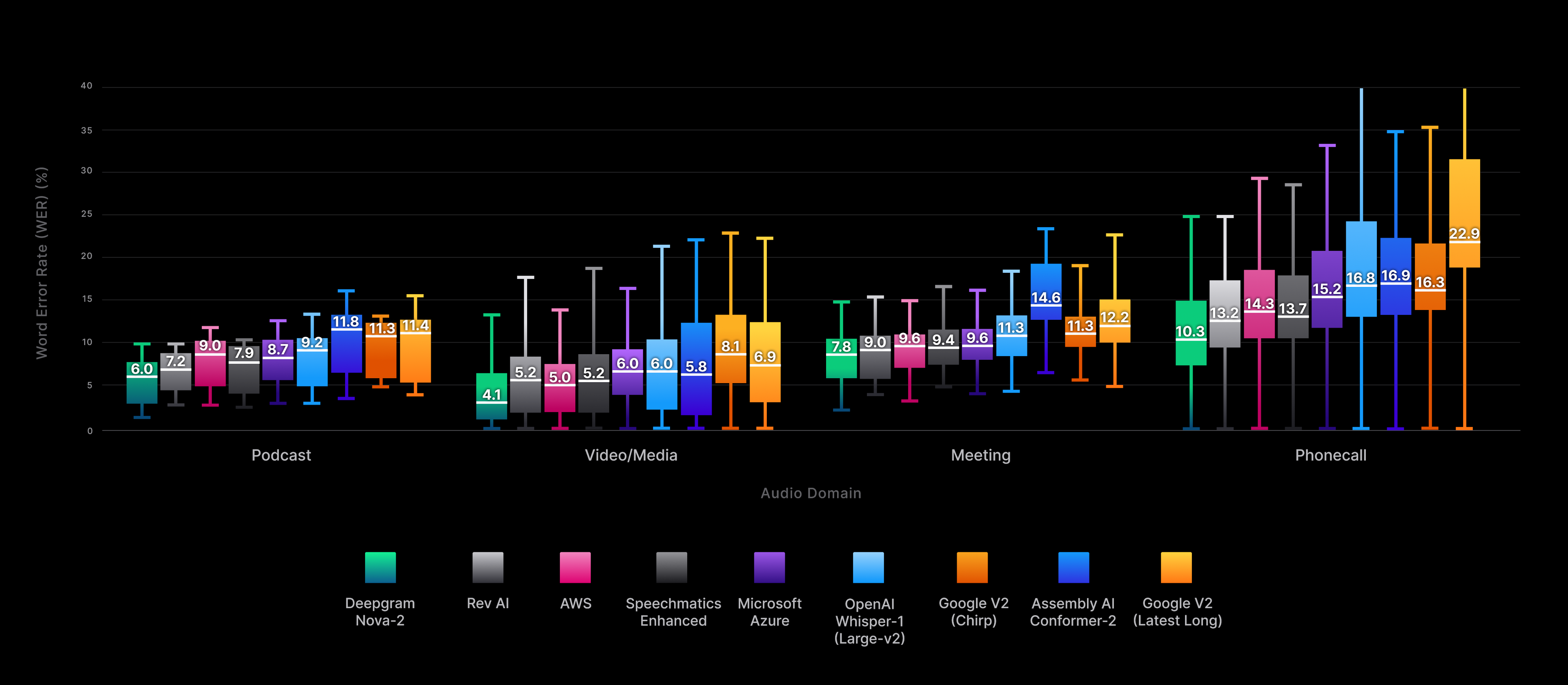
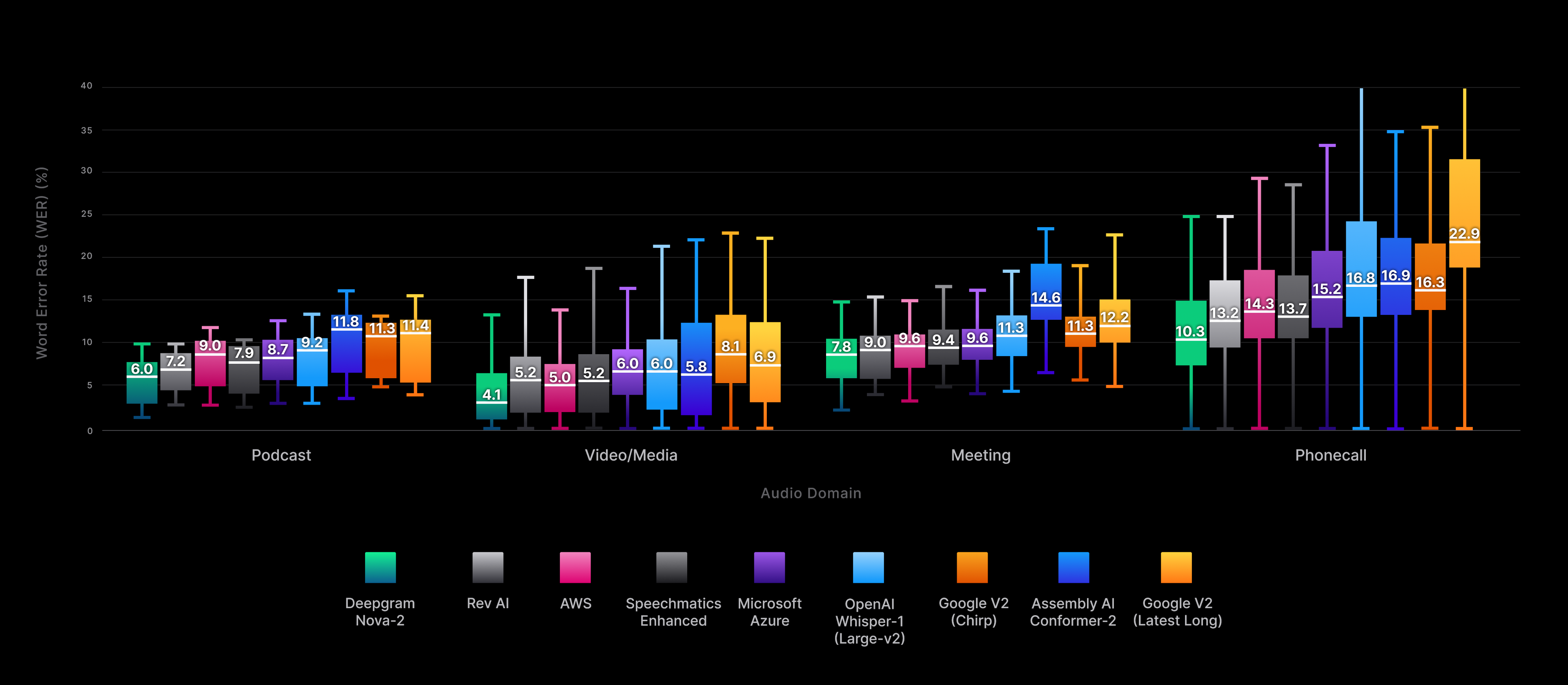
Figure 4: The figure above compares the average Word Error Rate (WER) of our Nova-2 model with other popular models across four audio domains: video/media, podcast, meeting, and phone call. It uses a boxplot chart, which is a type of chart often used to visually show the distribution of numerical data and skewness. The chart displays the five-number summary of each dataset, including the minimum value, first quartile (median of the lower half), median, third quartile (median of the upper half), and maximum value.
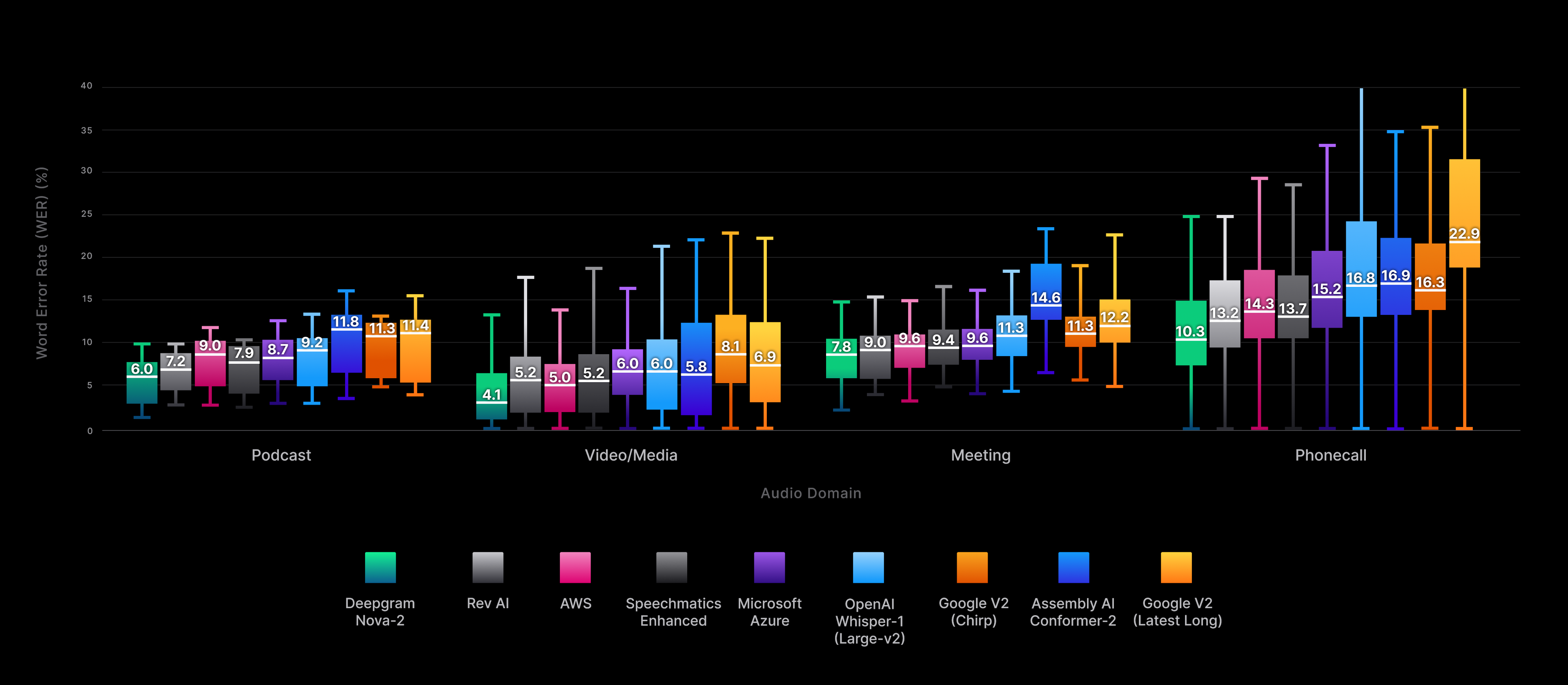
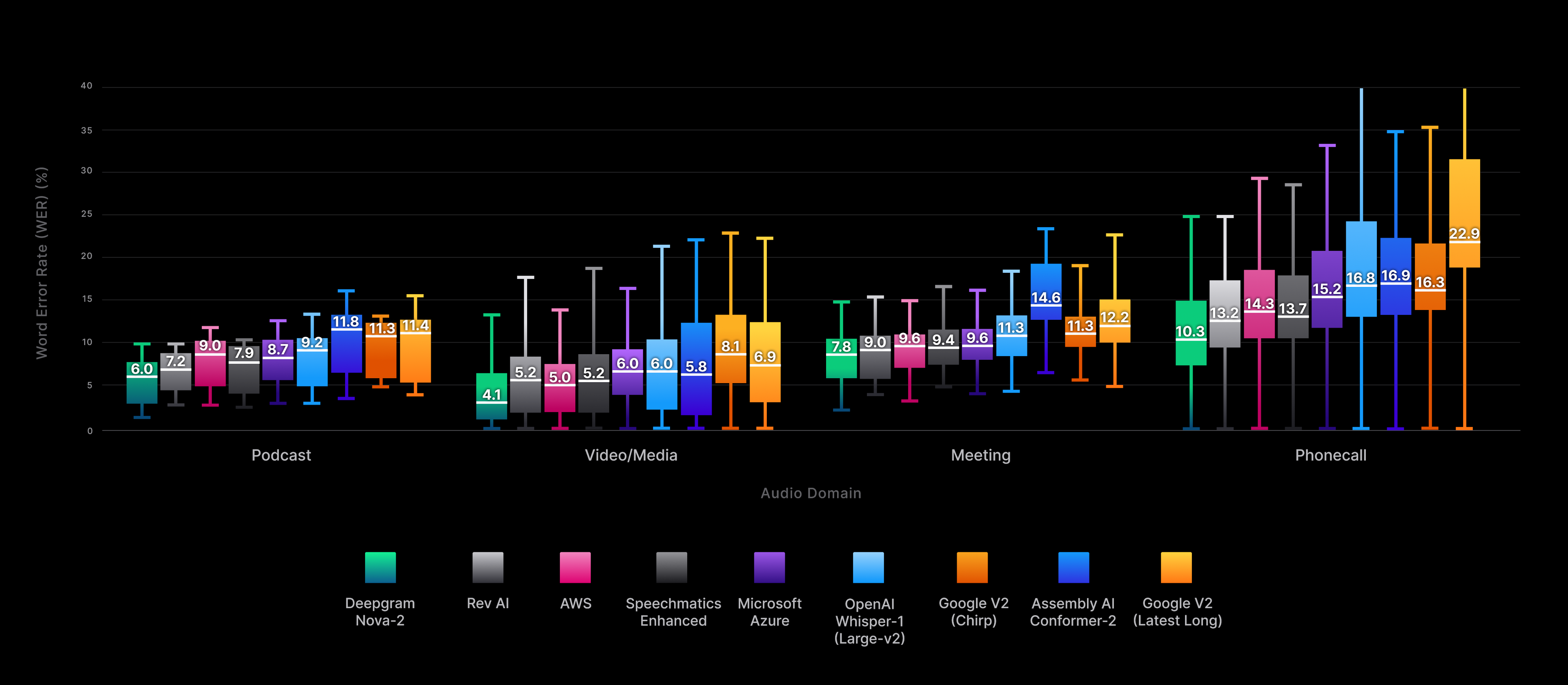
Figure 4: The figure above compares the average Word Error Rate (WER) of our Nova-2 model with other popular models across four audio domains: video/media, podcast, meeting, and phone call. It uses a boxplot chart, which is a type of chart often used to visually show the distribution of numerical data and skewness. The chart displays the five-number summary of each dataset, including the minimum value, first quartile (median of the lower half), median, third quartile (median of the upper half), and maximum value.
The undisputed leader in real-time accuracy
Modern speech applications–such as real-time agent assist, live captioning of streaming video, and automated food ordering systems–depend on real-time transcriptions to automate interactions with end users and deliver a good customer experience. However, limited options exist for true real-time speech-to-text.
Several providers included in our pre-recorded audio tests lack a native streaming model, such as OpenAI Whisper, so our real-time benchmarking was restricted to a smaller set of alternatives. As the results of our evaluation show in Fig. 5, Nova-2 handily outperforms the field with an average relative reduction in WER of 30% across all domains and 12% lower error rate than the nearest competitor.
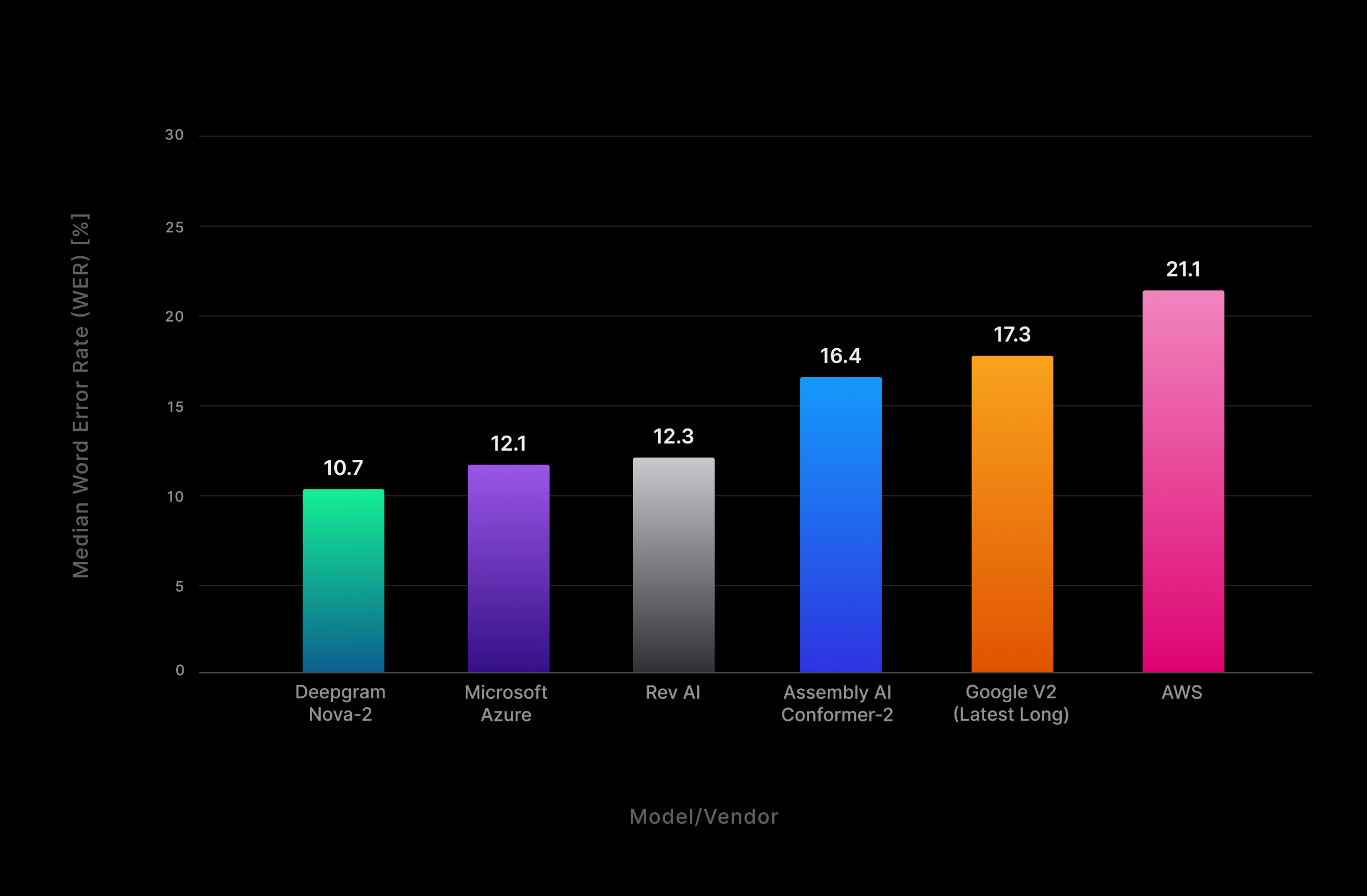
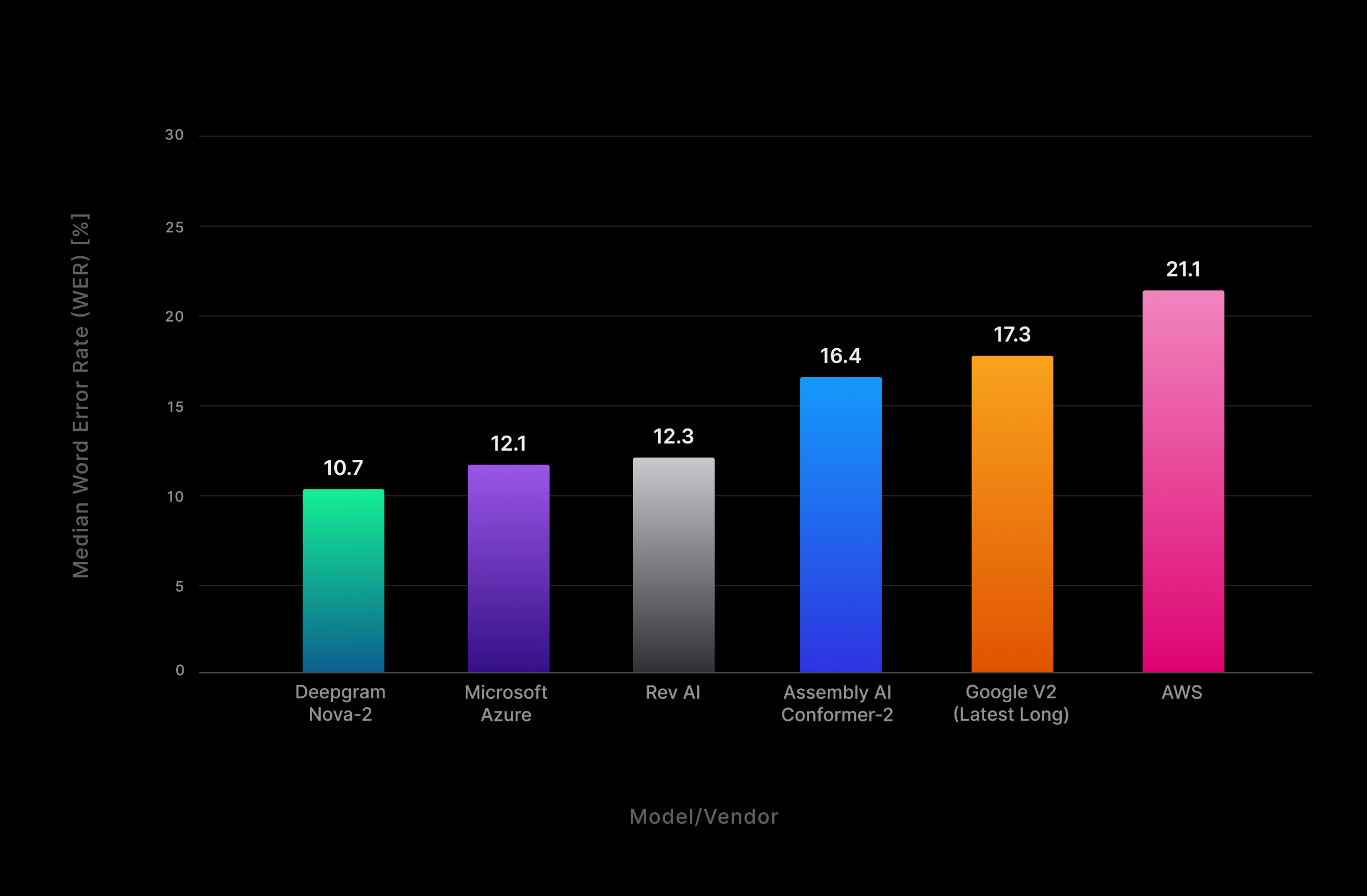
Figure 5: Median file word error rate (WER) for overall aggregate real-time transcription across all audio domains.
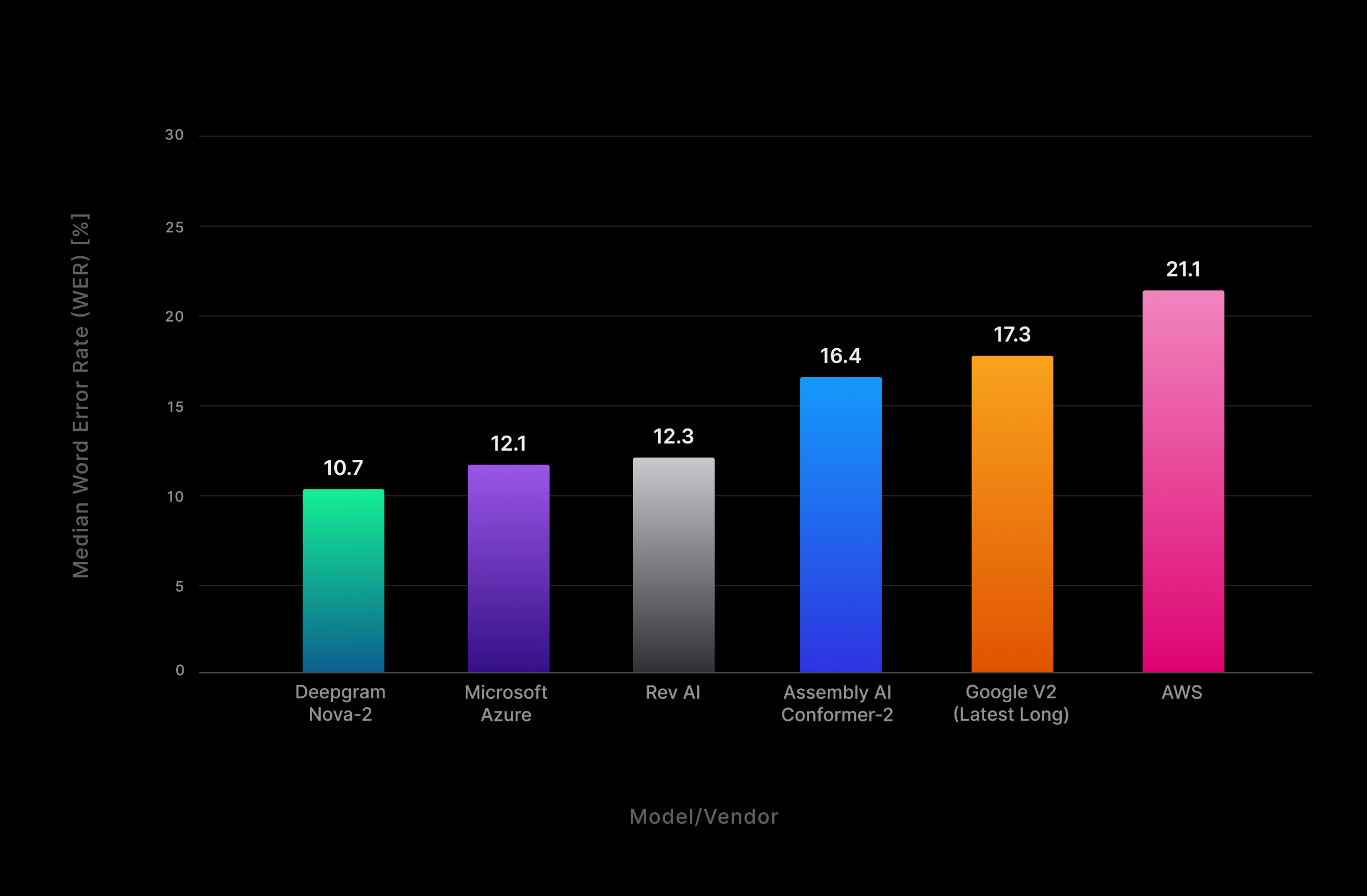
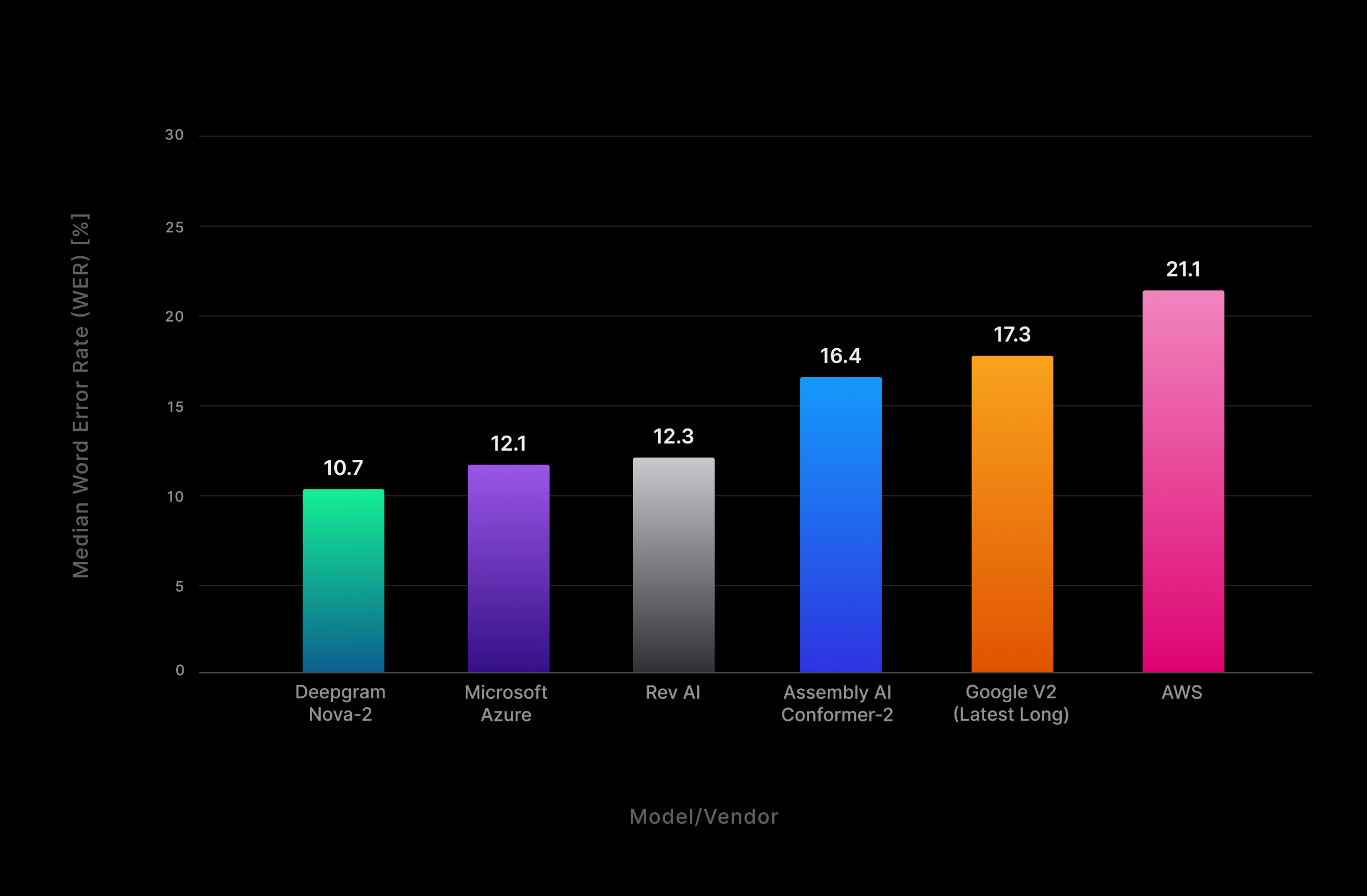
Figure 5: Median file word error rate (WER) for overall aggregate real-time transcription across all audio domains.
Speed: Hands-down the fastest model
Fast inference speed is a critical feature for many use cases. To evaluate Nova-2's competitive performance, we conducted multiple inference trials across each model/vendor for comparison. Speed was measured as the total turn-around time (TAT) starting from just before the transcription request was sent to just after the transcription response was returned. These speed assessments were carried out by sending the same 15-minute[2] audio file 10 times through each model/vendor, with speaker diarization[3] included, to ensure a fair and direct comparison.
From the resulting turn-around times (TAT), we compiled aggregate inference speed statistics and compared them to Nova-2's performance under the same parameters. Our findings revealed that Nova-2 surpassed all other speech-to-text models, achieving an impressive median inference time of 29.8 seconds per hour of diarized audio. This represents a significant speed advantage, ranging from 5 to 40 times faster than comparable vendors offering diarization.
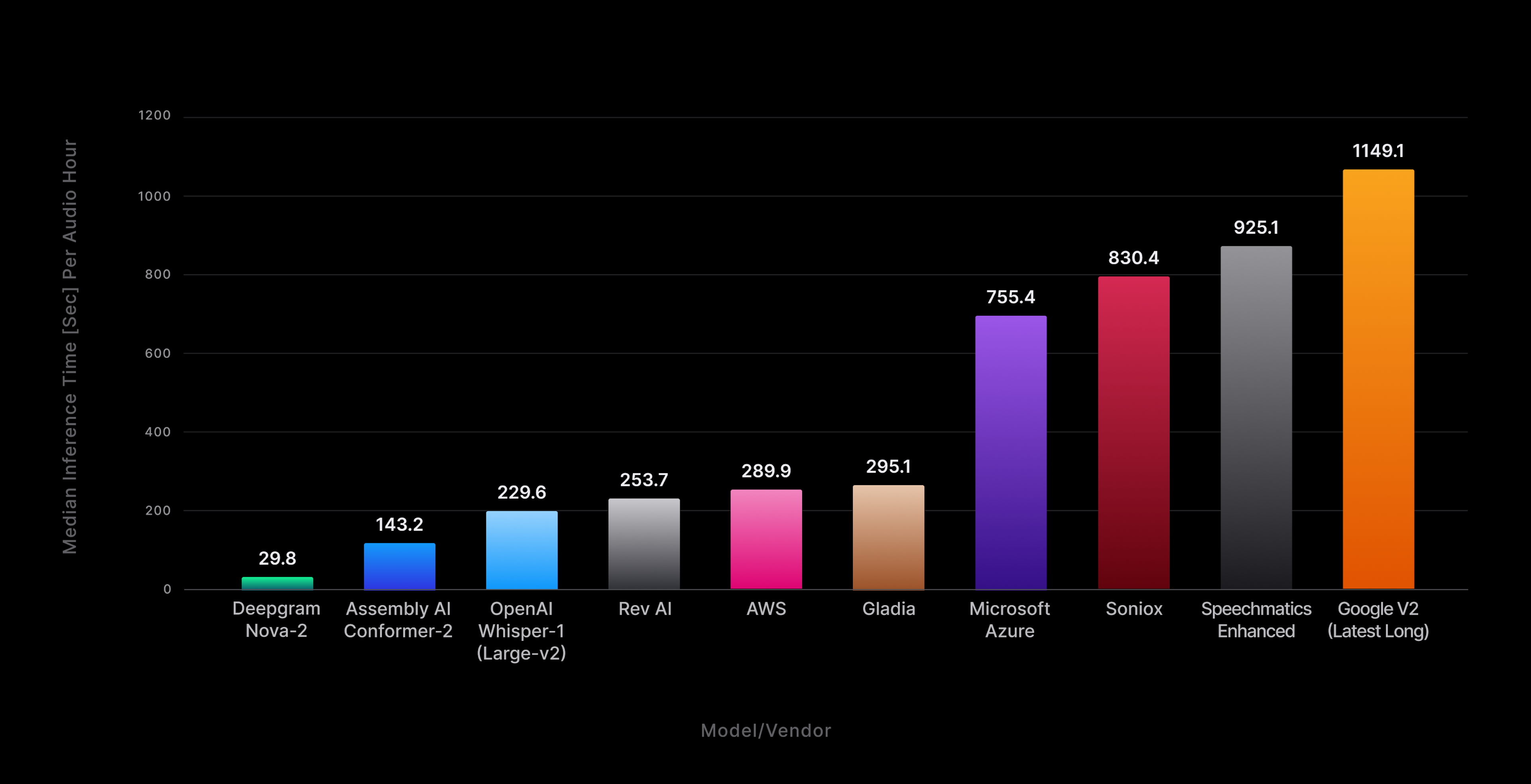
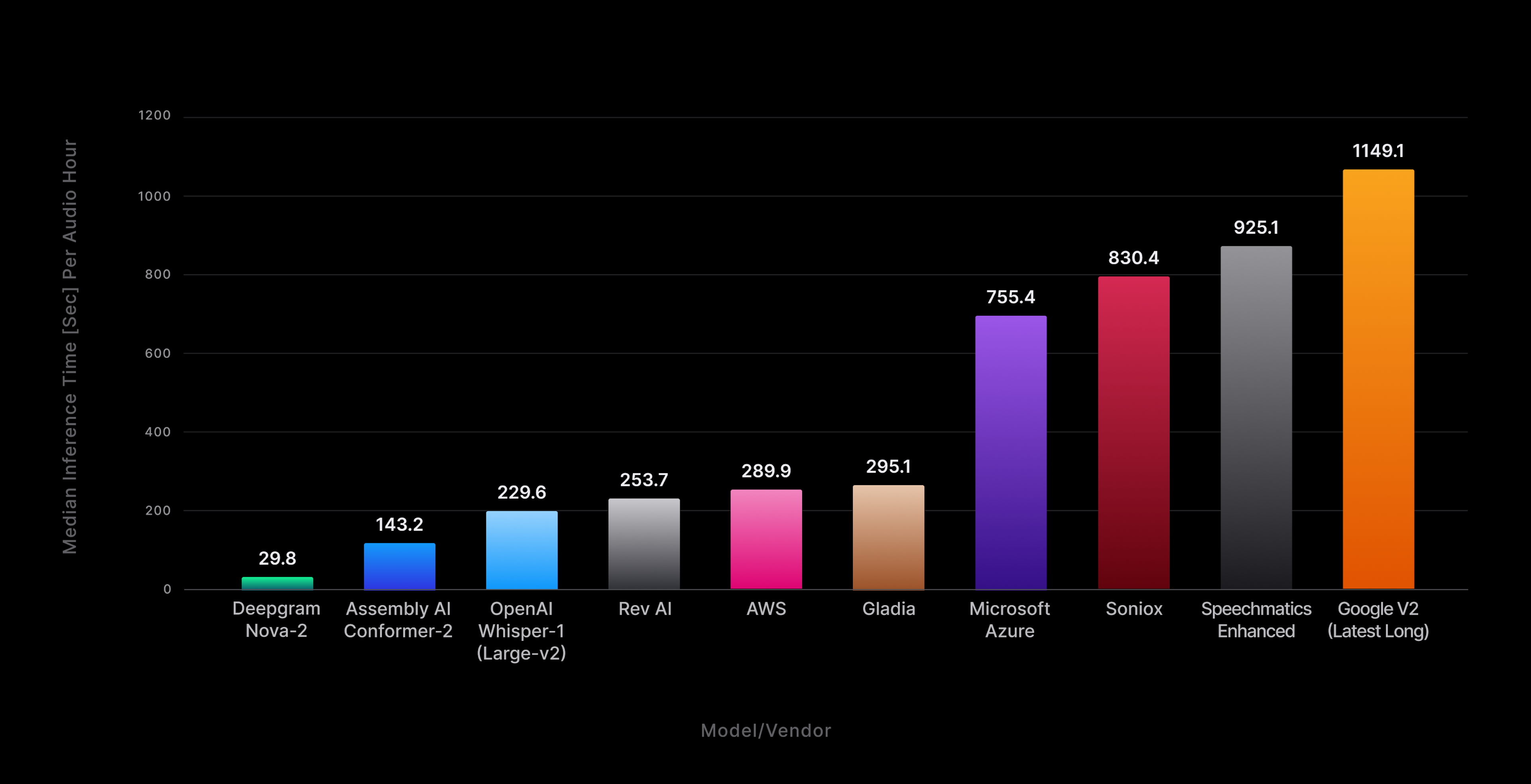
Figure 6: The median inference time per audio hour was determined using longer files, and diarization was included where applicable. To ensure a fair comparison, results are based on numerous trials of the same file for each vendor, giving them a fair opportunity to showcase their performance.
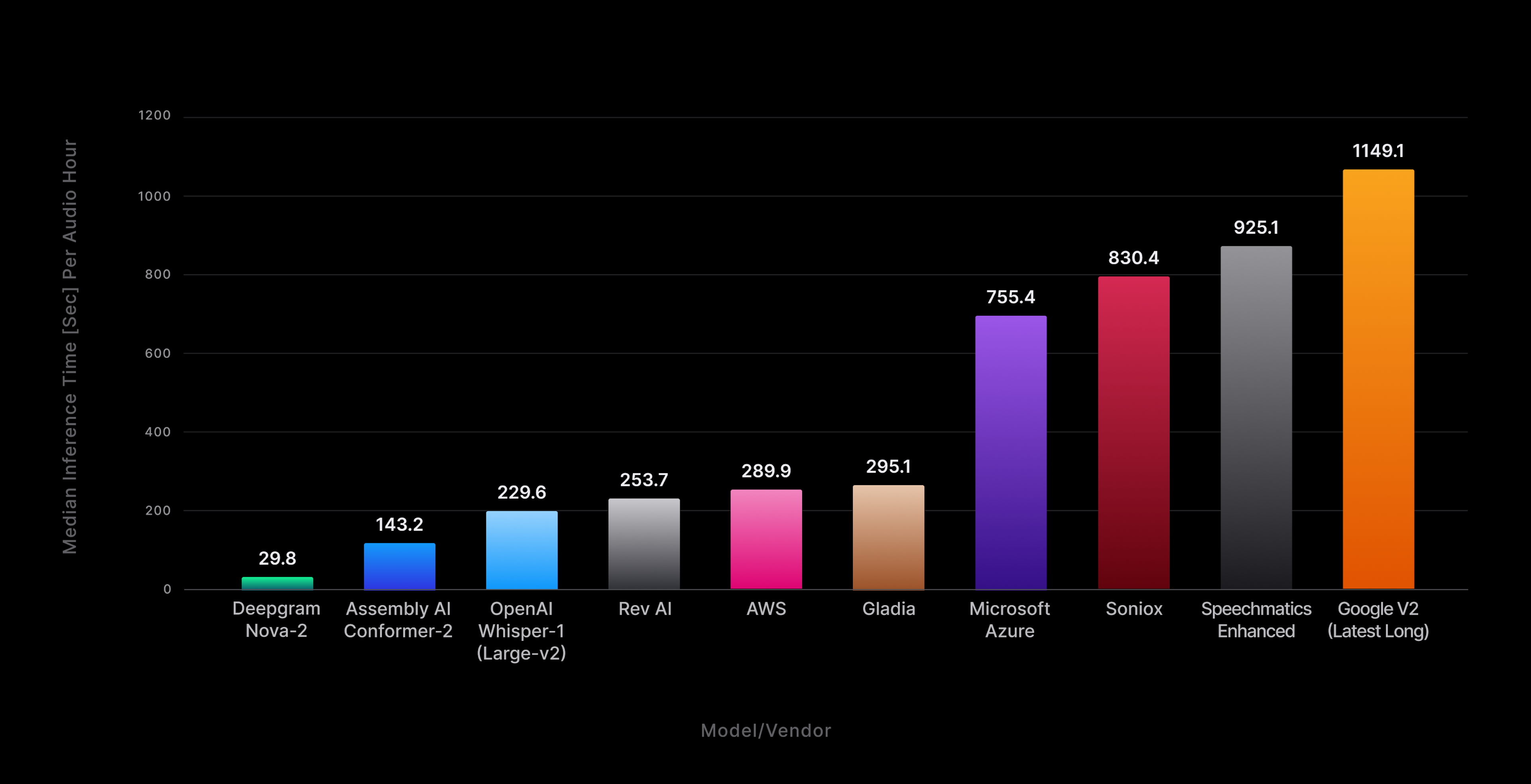
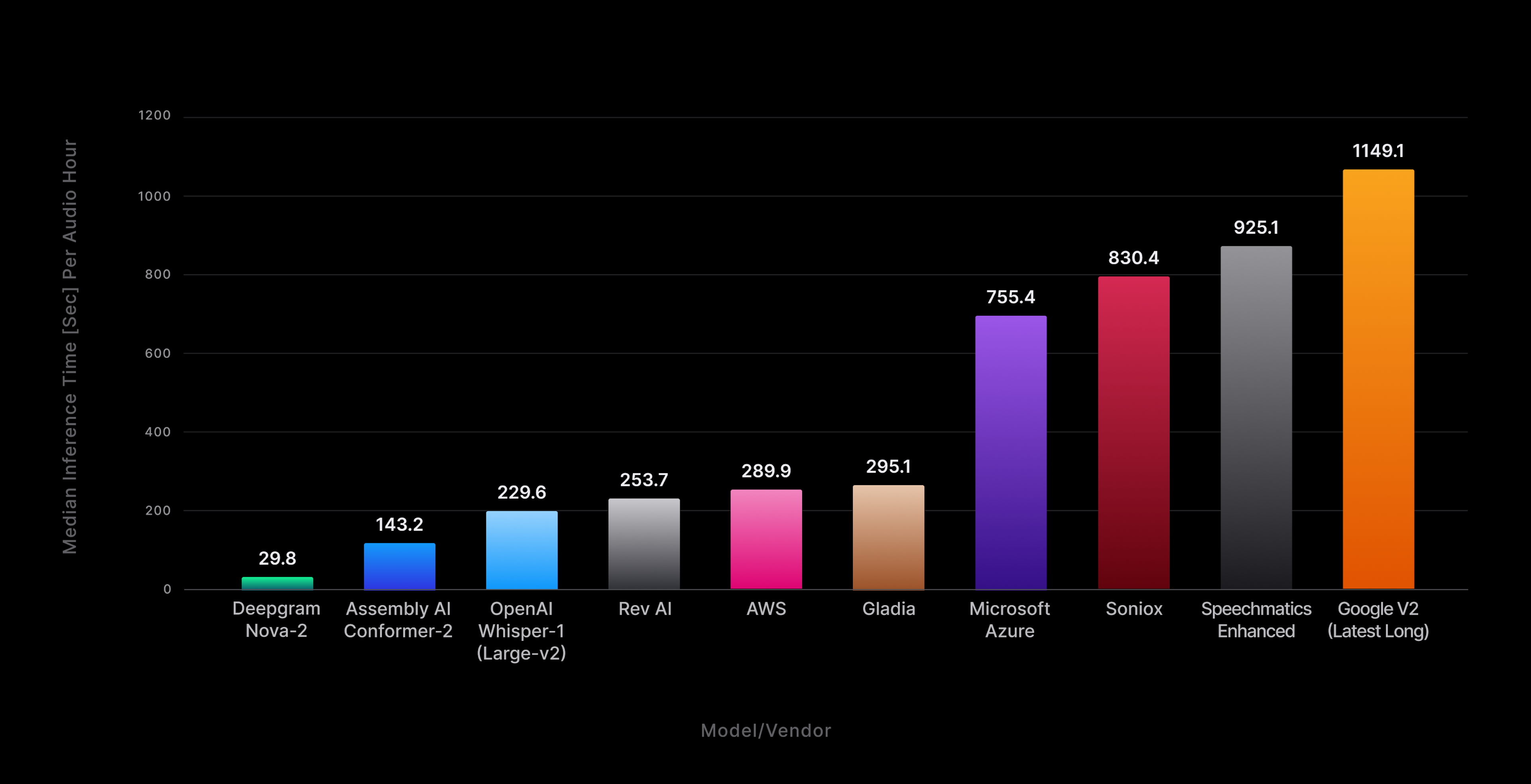
Figure 6: The median inference time per audio hour was determined using longer files, and diarization was included where applicable. To ensure a fair comparison, results are based on numerous trials of the same file for each vendor, giving them a fair opportunity to showcase their performance.
Cost: The most affordable ASR model in the market
Deepgram Nova-2 isn't just exceptionally fast and remarkably accurate. Like its predecessor, it's also designed to reduce your expenses. Our cutting-edge, next-generation models push the boundaries of efficiency, resulting in tangible cost reductions for your operations.
Nova-2 maintains the same starting price as Nova at just $0.0043 per minute of pre-recorded audio, nearly 3-5x more affordable than any other full-functionality provider (based on currently listed pricing) in the market.
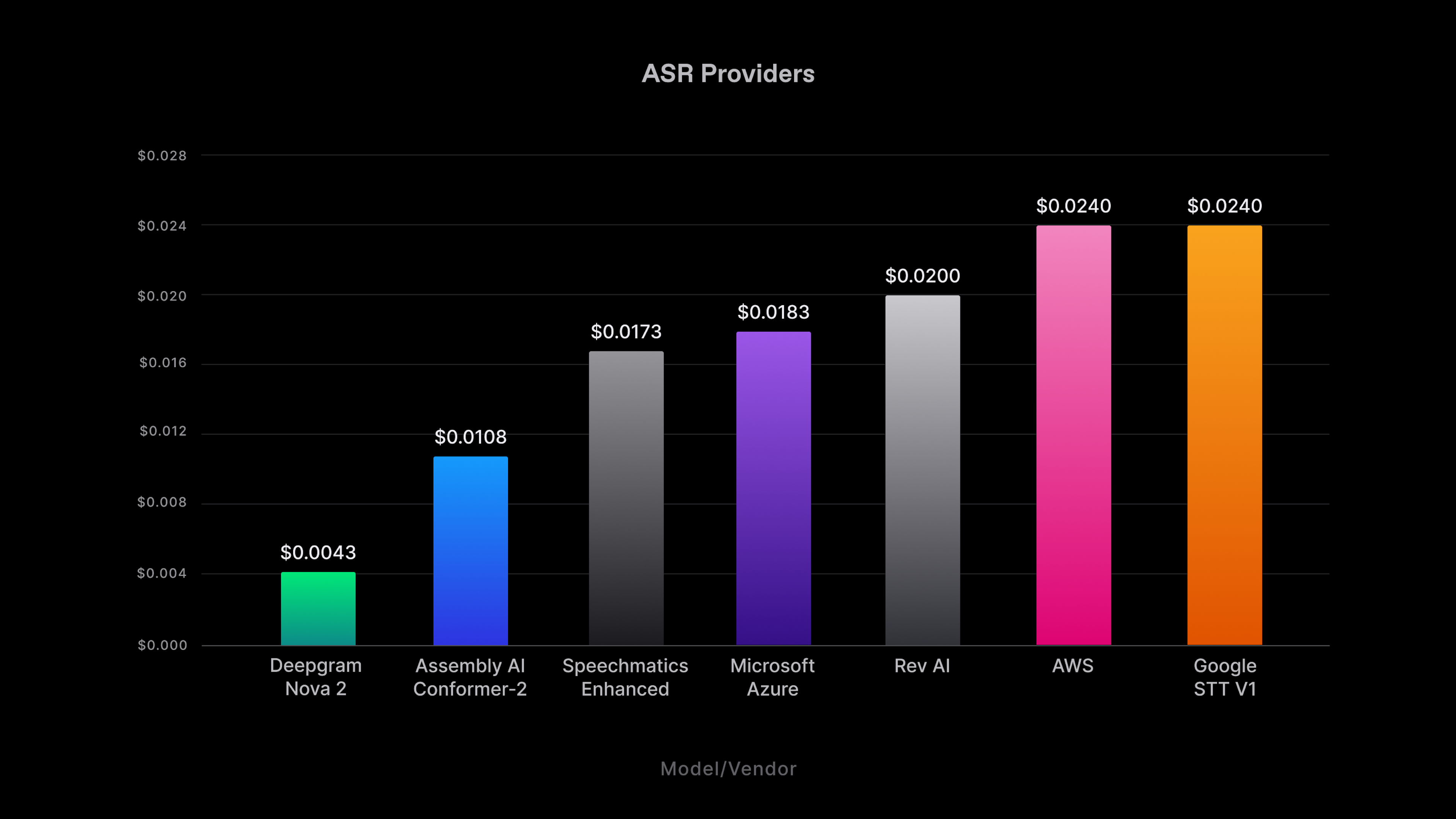
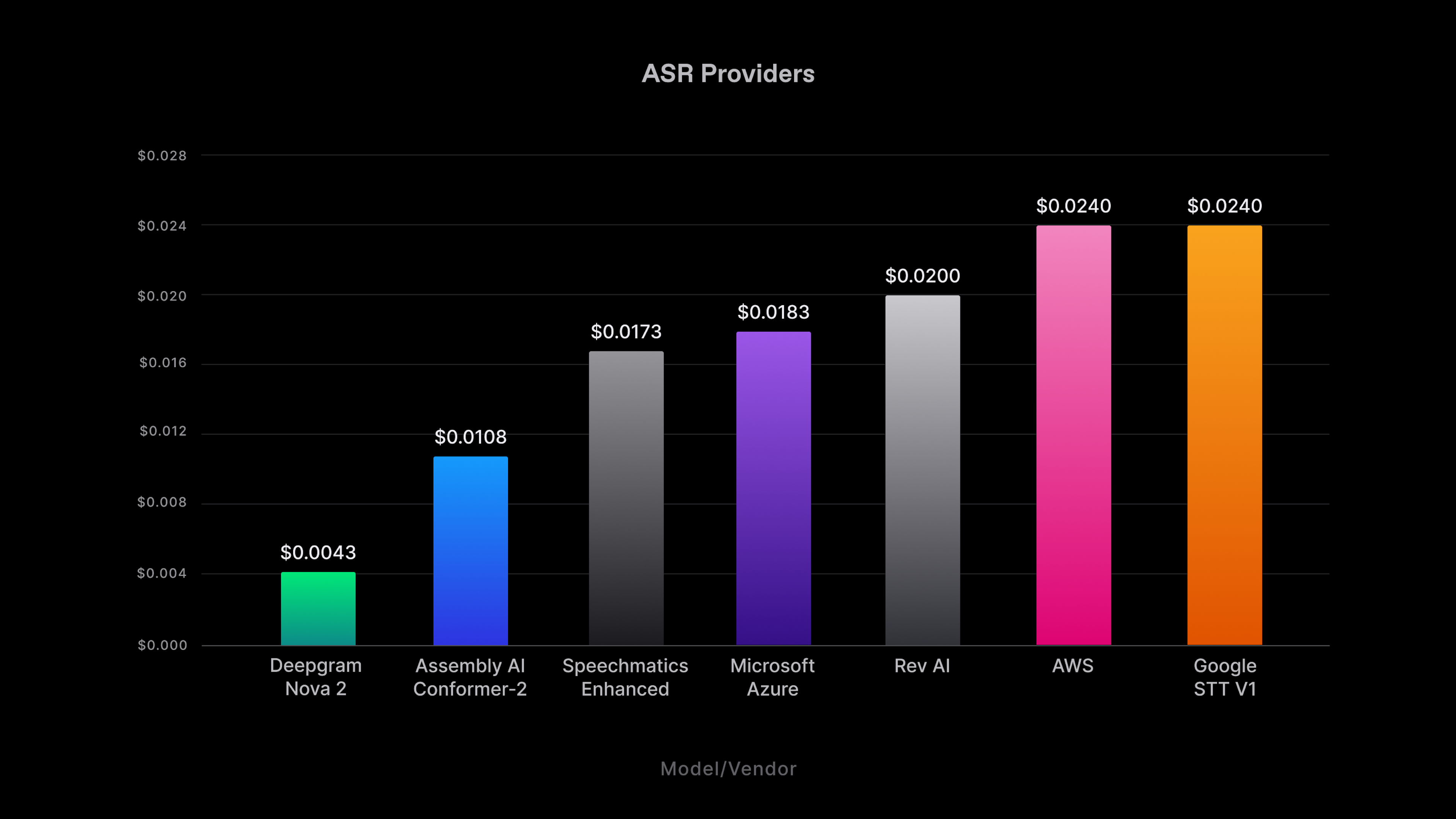
Figure 7: Pre-recorded transcription pricing.
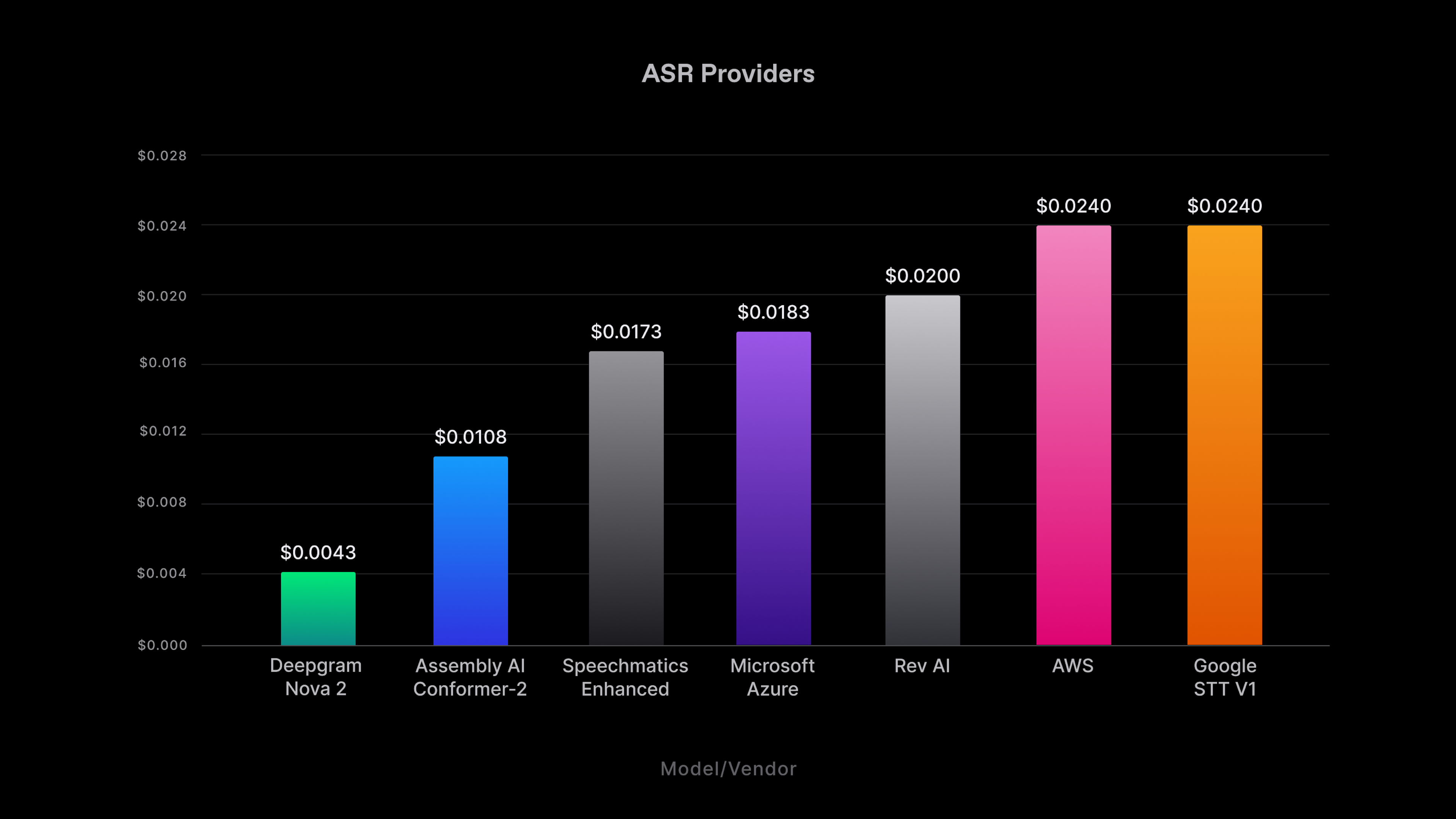
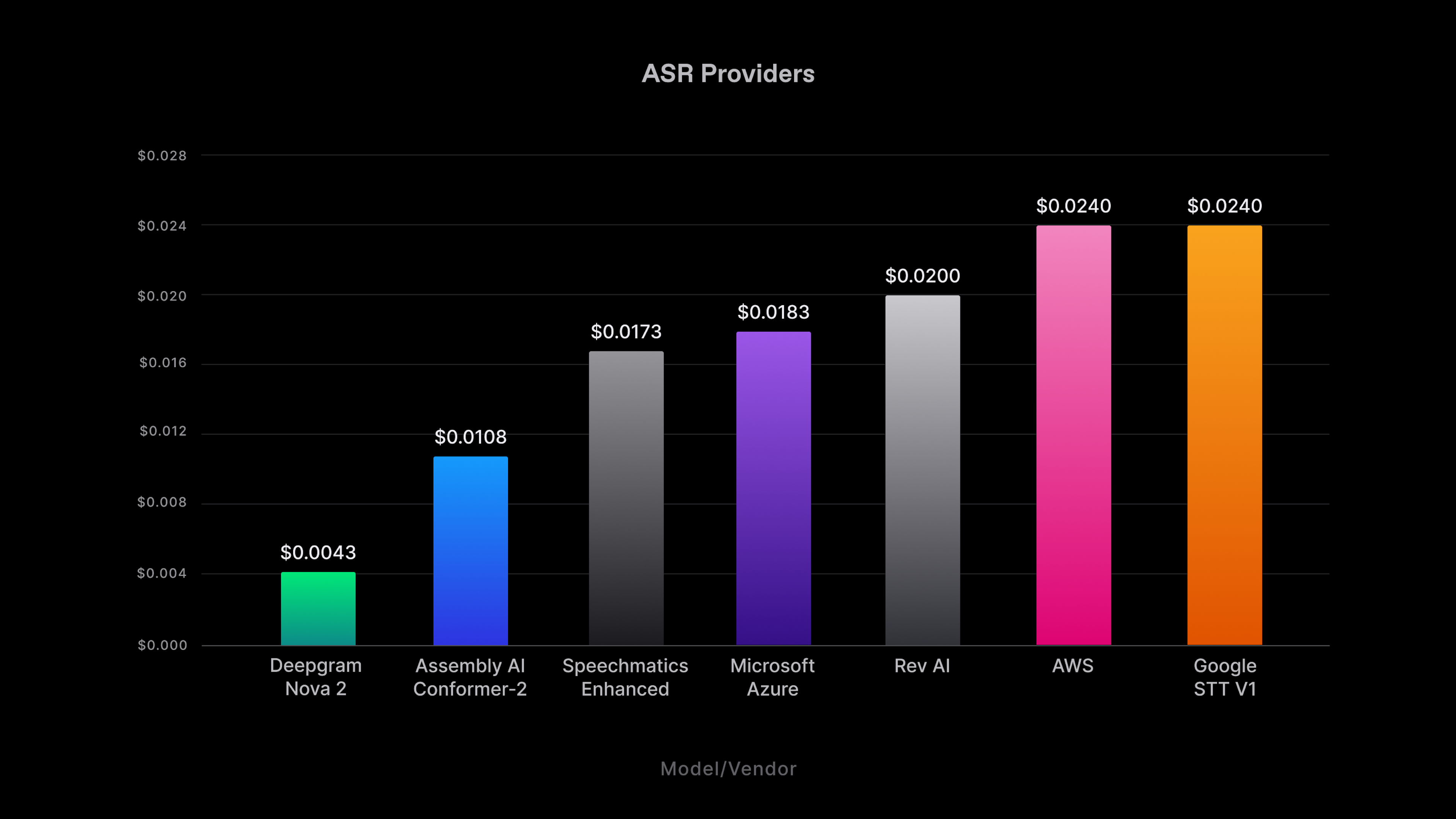
Figure 7: Pre-recorded transcription pricing.
Getting Started
It’s easy to get started with Nova-2, as all new signups and existing Pay-as-you-Go and Growth customers will automatically be granted access. Current customers under contract can request access here.
To access the model, simply use model=nova-2-ea in your API calls. To enable entity formatting, use model=nova-2-ea&smart_format=true. If you want to use the model/tier syntax, use model=2-ea&tier=nova in your API call.
Our early access model is limited to English audio only for now, but we are hard at work training additional languages and use case models for our upcoming general availability (GA) release. To learn more, please visit our API Documentation.
What’s Ahead: Building the Future of Language AI
The landscape of language AI is in a perpetual state of evolution. Here at Deepgram, we're enthusiastic about the ongoing advancements that are making automatic speech recognition increasingly practical for addressing real-world challenges. Our primary objective is to facilitate the seamless integration of language AI into your applications through our APIs.
We invite you to become an early access user or visit our API Playground to explore and assess Deepgram Nova-2 firsthand. Evaluate its performance and make your own comparisons in terms of accuracy and speed with any of the models featured in our benchmarks while also carefully considering the cost implications aligned with your specific application requirements. What compromises, if any, are you willing to make between accuracy, speed, and cost? Or do you find yourself in a position where no tradeoffs can be made at all?
As pioneers in AI-driven communication, Deepgram is unwavering in its commitment to reshape the way we interact with technology and with each other. We firmly believe that language is the key that unlocks the full potential of AI, forging a future where natural language serves as the cornerstone of human-computer interaction. With industry-leading ASR provided by models like Deepgram Nova-2, we're one step closer to realizing this future.
However, we're far from reaching the end of our journey. If the past six months are any indication, we have an array of exciting announcements in store. Stay tuned for more updates coming your way soon!
Footnotes
[1] To compare speech-to-text models accurately and obtain an objective score that corresponds to people's preferences, we first ensured that the output of each model followed the same formatting rules by running it through Deepgram's text and style guide normalizer.
[2] We attempted to standardize speed tests for all Automatic Speech Recognition (ASR) vendors by using files with durations longer than 10 minutes. However, OpenAI's file size limitations prevented us from submitting longer files. Therefore, the turnaround times shown for OpenAI in this analysis are based on the types of shorter audio files that can be successfully submitted to their API.
[3] Features like diarization were enabled where possible for consistency across vendors under evaluation when making pre-recorded audio transcription calls, however neither OpenAI Whisper nor Google V2 API support diarization. This gives those vendors an advantage in the pre-recorded speed comparisons with results that are inflated compared to those that would occur with such feature support added.
If you have any feedback about this post, or anything else around Deepgram, we'd love to hear from you. Please let us know in our GitHub discussions or contact us to talk to one of our product experts for more information today.