Human vs. Machine: A Comparative Study on Creativity in the Age of AI
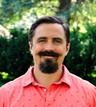
Brad Nikkel
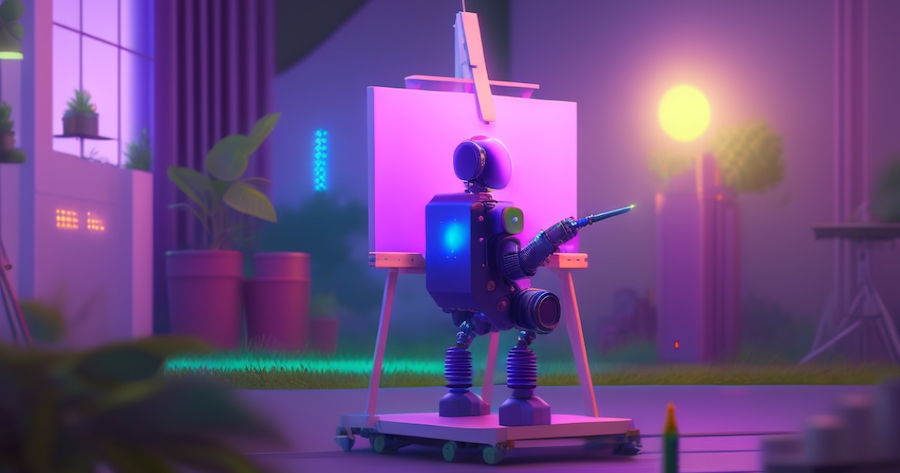
Artificial Intelligence (AI) has shattered many assumptions—even amongst the field’s old hands. Douglas Hofstadter—himself a skeptic of machine intelligence whose work inspired many AI researchers—mistakenly speculated that chess would remain beyond AI’s competency when he wrote Gödel, Escher, Bach. Given recent developments in AI-generated pop songs, you might say that Hofstadter also incorrectly speculated that musical capacity would remain beyond AI’s grasp in the same book. While we can forgive Hofstadter’s 1979 mispredictions of future AI capabilities, how should we view the underlying assumption that machines aren’t currently (or possibly won’t ever be) creative the way that we believe humans to be.
While plenty of people beyond Hofstadter likely hold similar assumptions, other folks are increasingly comfortable ascribing emergent properties to Generative AI (GAI) chatbots, including theory-of-mind, meta-learning, and even, lo and behold, creativity (though Schaeffer et al. recently demonstrated that some so-called emergent properties vanish when swapping non-linear for linear performance metrics). Before OpenAI claimed that their GPT-4 was “more creative and collaborative than ever before,” a pair of researchers tested the assumption that GAI chatbots cannot be creative. To do so, Humboldt University research assistant Jennifer Haase and University of Essex psychology lecturer Dr. Paul Hanel compared human and chatbot-generated ideas’ creativity. Given chatbots’ hallucination propensities, Haase and Hanel believe that chatbots are likely more useful for creative tasks than tasks grounded in reality, seeing chatbots as potential partners in human idea creation.
Scientifically Testing Creativity (in Humans and Machines)
How, exactly, does one go about testing whether someone (or some machine) is creative? To start, researchers don’t typically investigate creativity in binary terms (i.e., creative/uncreative). Rather, they study facets of creativity like “problem formulation, idea generation, idea selection, and potential idea implementation.” So, Haase and Hanel steered away from the less useful (and probably intractable) question of “are chatbots creative or not” by administering a creativity test that scientists often administer to humans—the Alternate Use Task (AUT)—to chatbots. To understand why they chose AUTs (we’ll dig into those in a bit), we should first hash out how Haase and Hanel view creativity.
Importantly, Haase and Hanel delineate creative tasks by scope into little-c (“everyday creativity”) and Big-C (“far reaching”) creativity. We won’t all paint a Mona Lisa, but plenty of our routine tasks often involve creativity; weaving together conversations, deriving quicker routes home, planning an optimal order of errands, or whipping up a meal from a nearly empty fridge, for example, all harness little-c creativity. Since chatbots aren’t yet composing concertos or architecting skyscrapers on their own, if they’re creative at all, Haase and Hanel believe chatbots qualify as little-c creative. Since their study focuses on little-c creativity, Haase and Hanel define creativity simply as “creating something new and useful,” arguing this definition adheres to our common-sense notion of creativity.
Alternative Use Task: Brainstorming New Uses for Everyday Objects
Now, back to Alternate Use Tasks. AUTs—still debated but widely appreciated for their predictive validity toward broader creative capabilities—involve generating as many uncommon uses for common objects as possible within some time limit. If some researcher asked you to take an AUT, they might, for example, ask you to list as many novel uses for an umbrella as you could muster within five minutes, tallying up any use other than keeping oneself dry from the rain.
Haase and Hanel gave a standard Alternative Use Test (AUT) to 100 human and five chatbot (Alpa.ai, Copy.ai, ChatGPT3, Studio, and YouChat) study participants, asking each participant to generate novel uses for the following five items:
ball
tire
fork
toothbrush
pair of pants.
Humans were given three minutes per object to write as many novel uses as they could. Since a three minute time limit would be less meaningful toward computationally powerful chatbots, they were instead asked, “What can you do with [one of the five objects]?” and then asked, “What else?” up to three times to get follow-on results (the chatbots often gave numerous results per prompt). Haase and Hanel randomized prompt orders and used separate chat sessions per prompt to deny chatbots knowledge of prior prompts.
Then, six human raters and, for good measure, an AI model trained to judge AUTs—oblivious to whether AI or humans conjured the uncommon uses for common objects—judged the human and chatbot study participants’ responses via the “Consensual Assessment Technique” (CAT). Less fancy than it may sound, the “Consensual Assessment Technique” consists of a handful of experts (in some specific creative domain) independently assessing some potentially creative output. In Haase and Hanel’s study, the six human judges scored individual participant responses’ originality and fluency, and then, since some participants generated numerous responses per prompt, averaged together each participant’s response scores per prompt. Haase and Hanel were more interested in measuring originality than fluency since chatbots can easily pump out volumes of text (fluency).
Experiment Results
Like beauty, though, isn’t creativity largely in the eye of the beholder? Can we really expect six humans and a machine judge to agree on responses’ originality and fluency? To assess consensus amongst the six human raters’ originality scores, Haase and Hanel computed intraclass correlations, finding human raters’ creativity assessments agreed between 85 and 94 percent of the time. Similarly, averaging the human raters’ scores and correlating these with the AI judge’s scores showed human-machine agreement 78 to 94 percent of the time. The same intraclass correlation method also showed a strong correlation between machine and human raters’ fluency judgments, which agreed 98 to 100 percent of the time. In the two charts below, you can see this solid human-machine consensus.
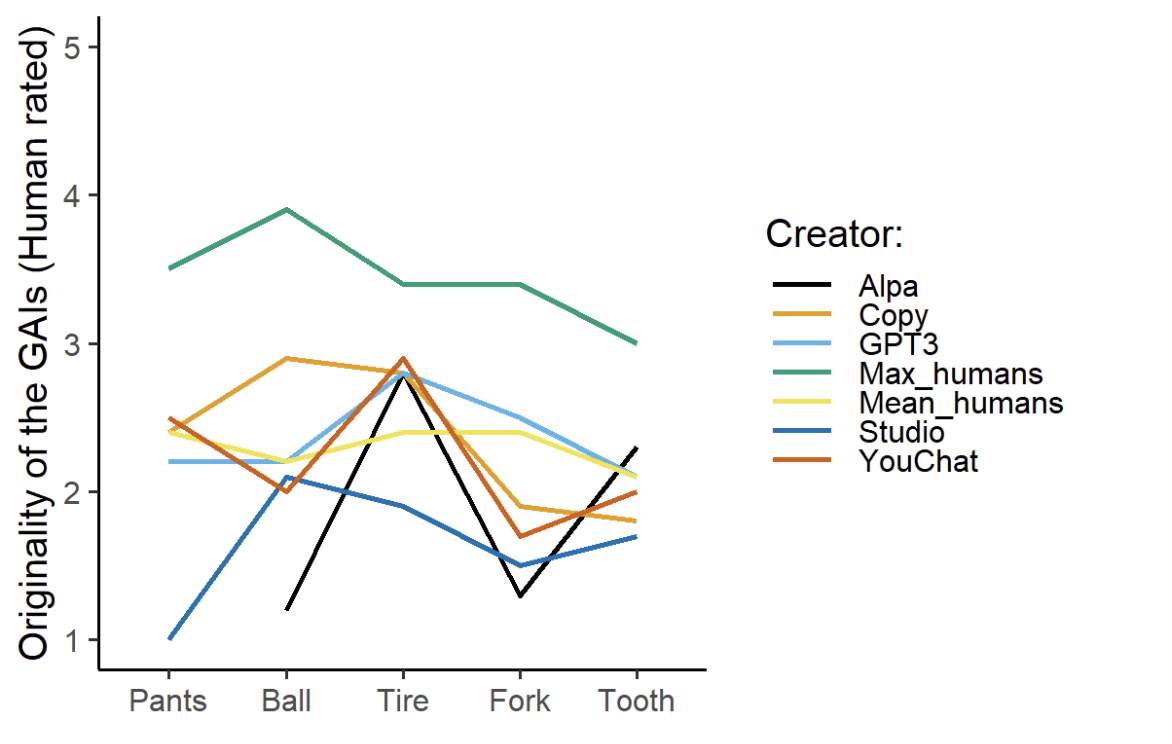
Human-rated originality scores for each generative artificial intelligence (GAI), including the average score from humans and the score of the most creative human.png.png
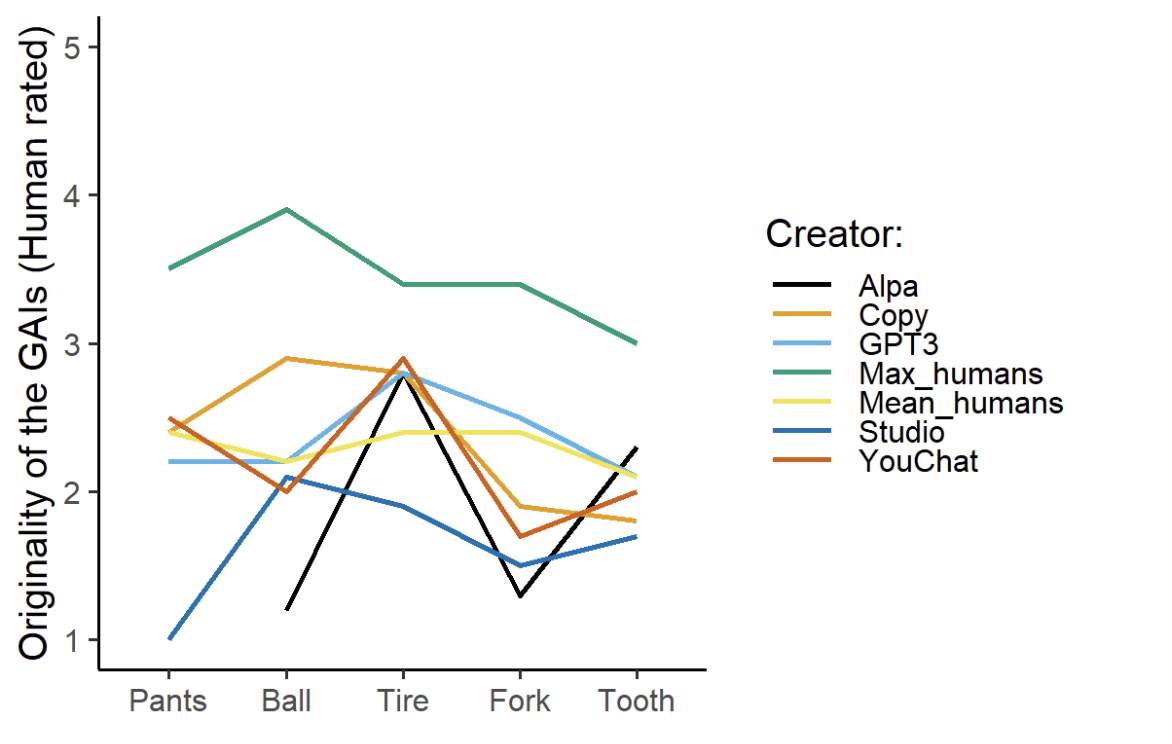
Human-rated originality scores for each generative artificial intelligence (GAI), including the average score from humans and the score of the most creative human.png.png
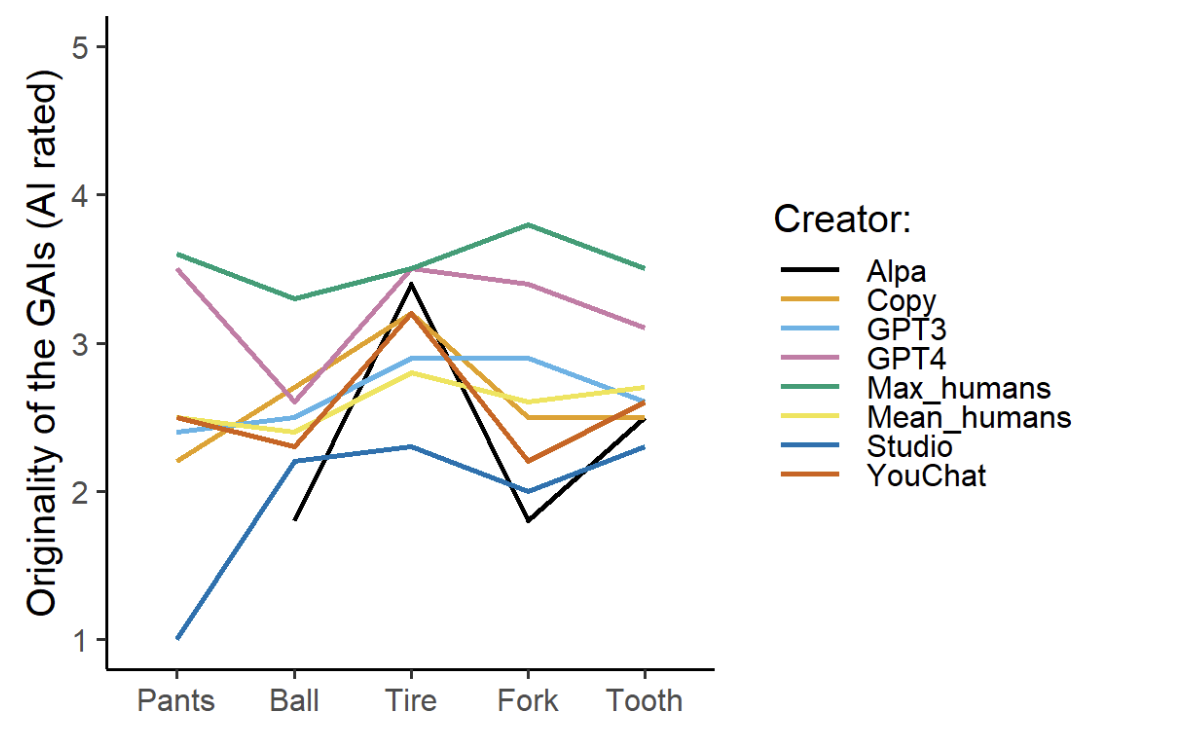
AI-rated originality scores for each generative artificial intelligence (GAI), including the average score from humans and the score of the most creative human.png
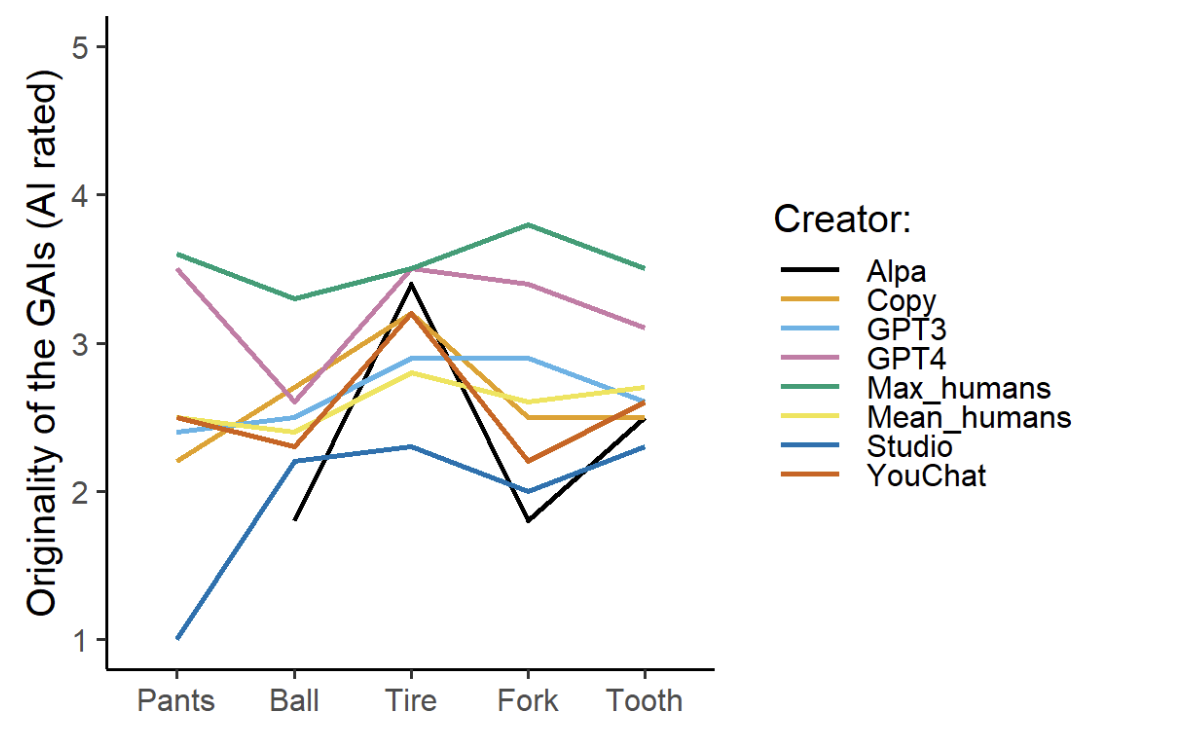
AI-rated originality scores for each generative artificial intelligence (GAI), including the average score from humans and the score of the most creative human.png
Interestingly, Haase and Hanel found no significant difference in originality among the five chatbots tested. While on average 32.8 humans were more original than the most original GAI chatbot, Haase and Hanel found that, overall, the chatbots tested were about as creative (or uncreative) as most humans (at these specific AUTs).
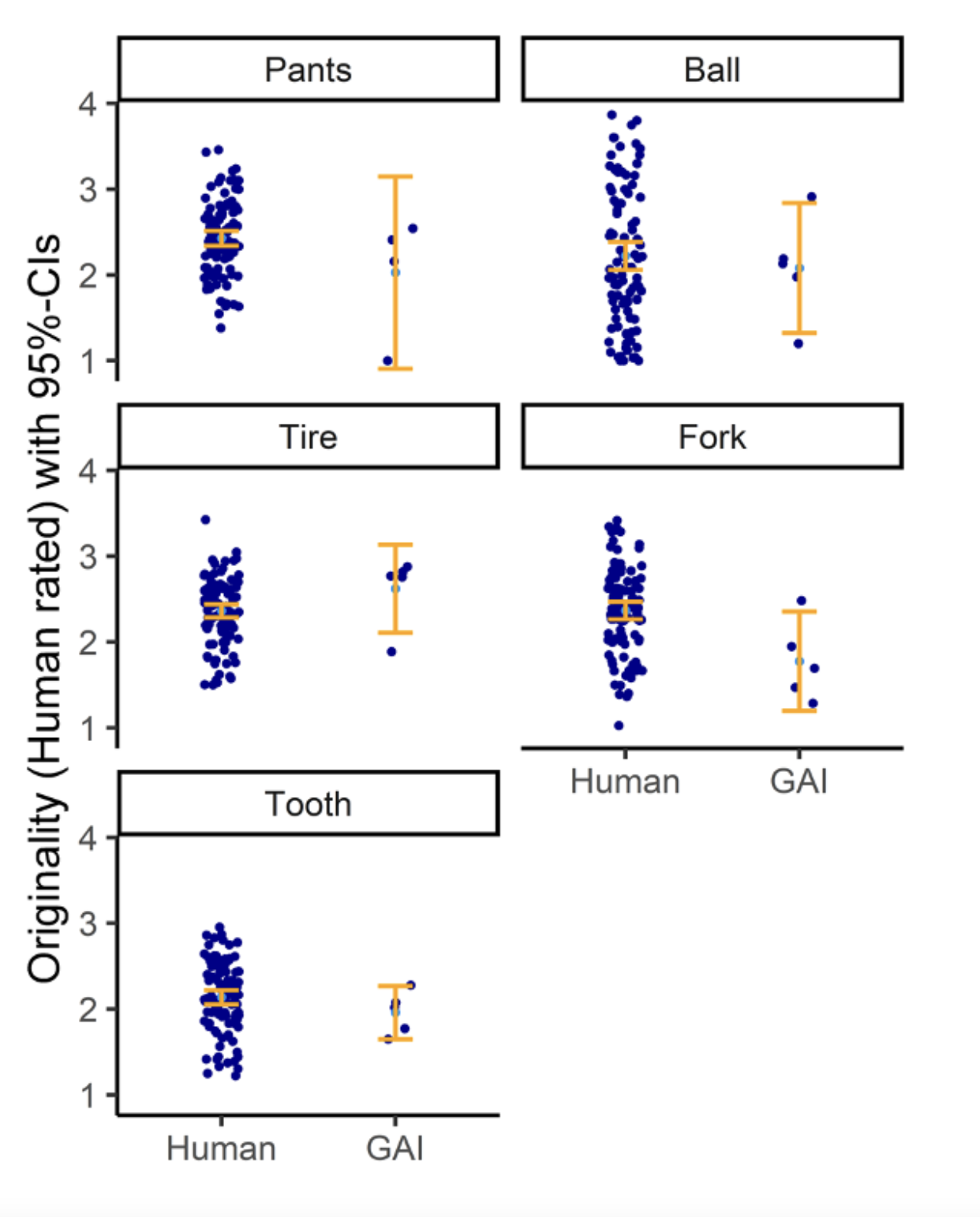
Human-rated levels of originality for human and GAI-generated ideas
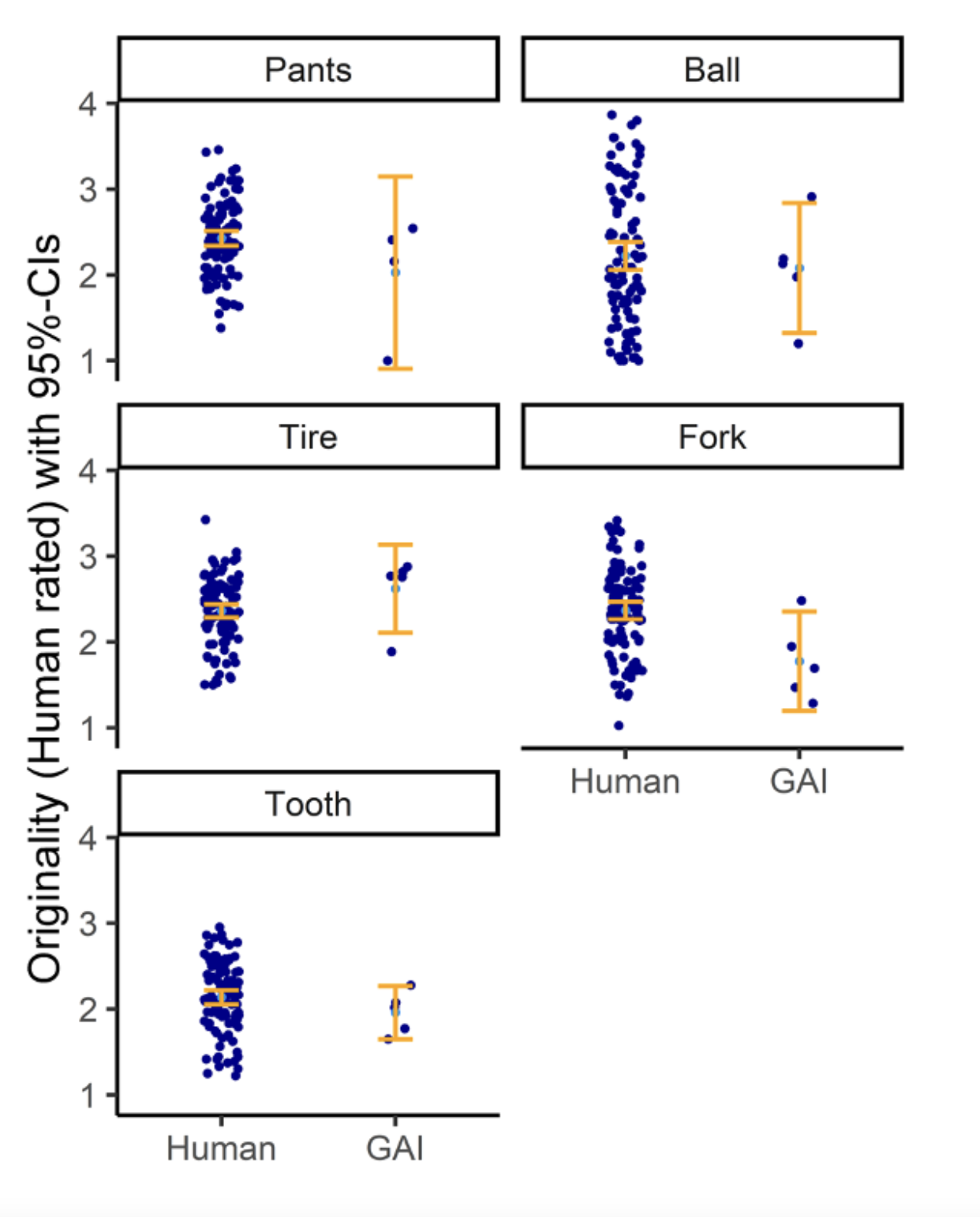
Human-rated levels of originality for human and GAI-generated ideas
A Few Shortcomings
Haase and Hanel admit a few experimental issues. First, they couldn’t rule out the possibility that the chatbots they tested saw AUTs in their training data. Given that Haase and Hanel used everyday objects (the point of the test), it indeed seems likely that the chatbots they tested might have encountered solutions to existing AUTs in their training data, possibly even AUTs using the same objects that Haase and Hanel tested on. It’s not immediately clear, though, how Haase and Hanel might have ruled this possibility out other than by first inventing fictional everyday objects, describing those objects to human and chatbot study participants, and then asking the participants to create novel uses for the fictional everyday object.
A second issue was that GPT-4 was not yet released when Haase and Hanel tested chatbots’ creative potential. Since springing additional tests on the human raters might make them suspicious that the additional tests might have something to do with GPT4, Haase and Hanel opted to instead only compare GPT4’s performance with other chatbots’ performance. Lending some credence to OpenAI’s claims, GPT4 surpassed the other chatbot GAIs at all tests except the ball test, where it came in second place.
Why Does Ascribing Creativity to ChatBots Feel so Off?
Despite Haase and Hanel’s results, it still seems odd to claim GAI chatbots are creative, even if only at little-c creative tasks. But why? Haase and Hanel address several common criticisms of chatbot creativity claims. The main argument that critics launch is that since chatbots simply stitch together existing ideas, they’re not creating anything novel and, thus, are not creative. Given that GAI chatbots are largely complex statistical models with a dash of stochasticity mixed in, this intuitively seems like a fine argument.
But we can launch the same argument against paragons of human literary, artistic, musical, and engineering creativity; rarely, if ever, is a human idea born in a complete vacuum. Instead, ideas often build on, permute, or recombine existing ideas. At least a thread connects even the most original ideas to some web of non-original ideas. From Adolphe Sax’s desire to mesh woodwinds’ dexterity with brass instruments’ sound, the saxophone was born. Jazz partly inspired abstract expressionists’ painted depictions of motion. Toss a dart anywhere at the map of human creative endeavors and it’ll be connected to some other creative endeavor—somehow.
Beyond ideas begetting ideas, some humans many consider creative quite literally permuted, recombined, and stitched together existing words, sentences, and phrases. Eighteenth century composers experimented with “musical dice,” slicing sheet music into chunks (e.g., measures), mixing them up, and then rolling dice to determine these musical segments’ order. The 1920’s Dada movement applied a similar “cut-up” technique to literature, which later authors like William Burroughs borrowed. Riffing off this tactic, David Bowie also cut out words and phrases, mixed them up, and stitched them together for lyrical inspiration, and eventually asked a friend to write a computer program that did this at scale. It seems we don’t have much ground to stand on in the stitching-together-existing-ideas-ain’t-creativity argument without also belittling human creativity.
The “Why” Behind Creativity
Maybe the problem is chatbots’ lack of motivation to create. For now, we must ask chatbots to generate some potentially creative output; otherwise, they do nothing. Typically, no one commands humans to be creative; it’s something they almost feel urged toward for various reasons. Stemming back to modern computing’s genesis, there’s a persistent notion that since humans must give computers instructions, we can’t consider them creative. Alan Turing dubbed this “Lady Lovelace’s objection,” because Ada Lovelace, one of the original conceivers of programmable computers, saw computers as unable to conjure their own ideas, excelling only at executing human-defined commands.
When a human, on the other hand, creates something we deem creative, we often care about their motive to do so. Knowing that a window view from an asylum he spent nearly a year in inspired Van Gogh’s The Starry Night might affect your opinion about that piece’s creativity. Likewise, knowing of his imprisonment by the Nazi’s in Dresden while the Allied forces firebombed that city might inform you about Kurt Vonnegut’s dark humor and, in turn, your appraisal of Slaughterhouse-Five’s creativity. In other words, we often place significant weight in the why behind human creativity.
It seems creative humans’ algorithms (i.e., their reward functions) are often some mixture of internal and external motivations, whereas creative chatbots’ reward functions are strictly bound to what humans find useful, appropriate, interesting, etc. Perhaps this is why it feels off to call a machine, on its own, creative, despite some chatbots now performing close to humans at AUTs.