AI emits Carbon, but how much value do we get in return?
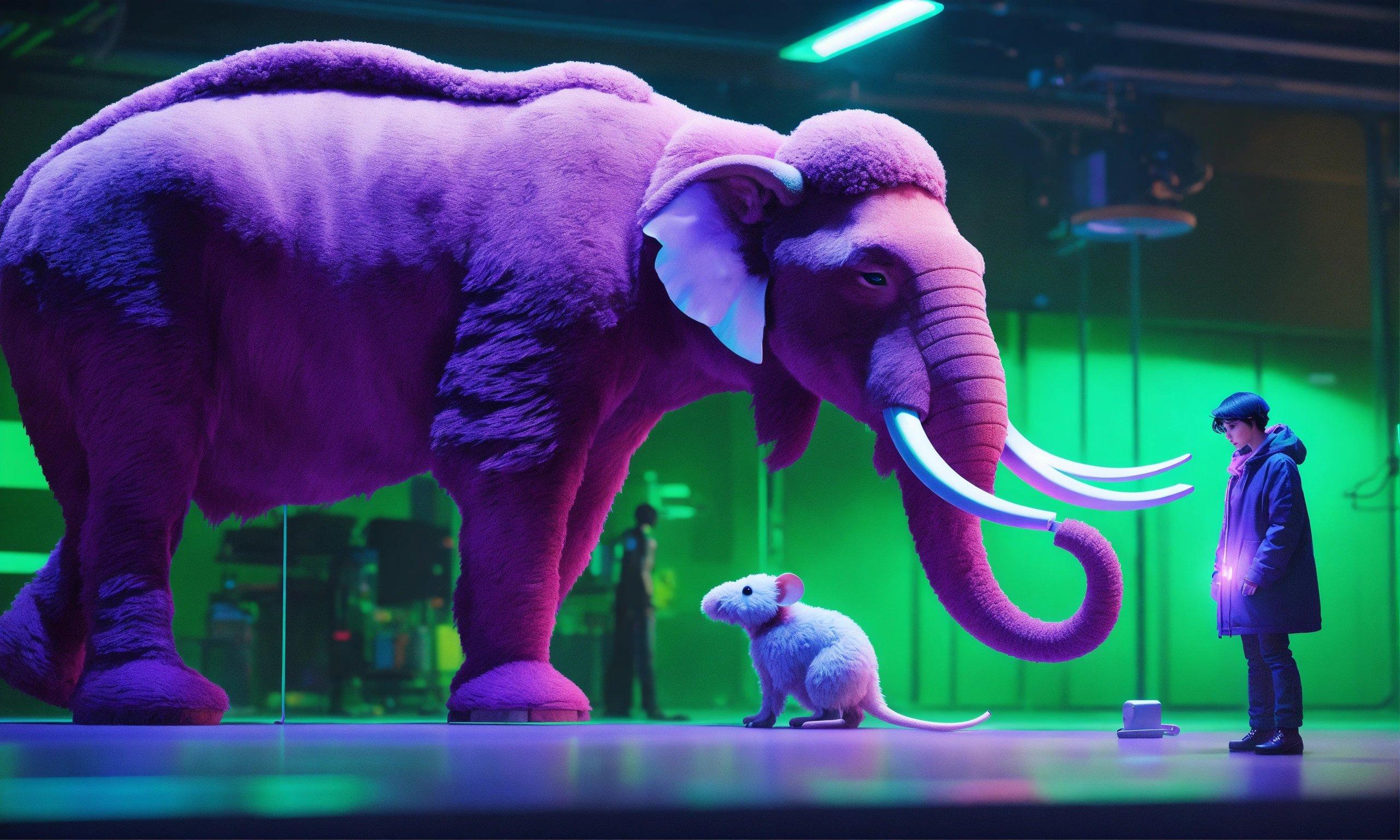
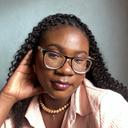
AI is revolutionizing not only the tech industry but also public and private industries in all around the world. We’re creating chatbots that perform as well as (and sometimes better than) humans at standardized tests, predicting renewable energy availability in advance to improve energy utilization, and maybe enabling self-driving cars. If we’re to believe some AI experts, it is possible that in a decade, the world we live in then might look vastly different from our reality now, with AI being embedded into our everyday lives. However, all of this development comes at a cost, a carbon cost, to be exact—especially with the most popular type of AI right now, generative models.
Some model developers opt to say the quiet part out loud. Case in point: Meta disclosed their estimates for training the herd of Llama language models. As stated in the paper announcing Llama 1, released in February 2023, about 300 tons of CO2 was generated as a result of training the model. Llama 2, announced in July, took roughly 3.31 million hours of GPU time across all available model weights, resulting in 539 tons of CO2 emissions, according to the Llama 2 paper. The Facebook parent company aims to be carbon neutral by 2030.
It is also estimated that training GPT-3 took 1.287 gigawatt hours and emitted 552 tons of CO2. For context, this is about as much electricity as 130 homes in the U.S. would consume in a year.
However, a quote from the 78-page Llama-2 paper itself reads “100% of these emissions are directly offset by Meta’s sustainability program.” Or, in other words, Meta contends that their internal environmental efforts fully compensate for any emissions created by the training, finetuning, and testing of Llama-2.
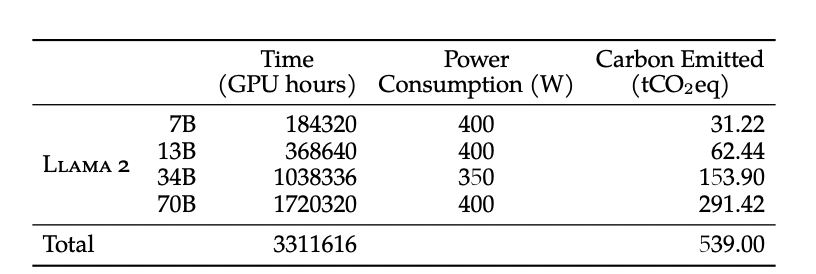
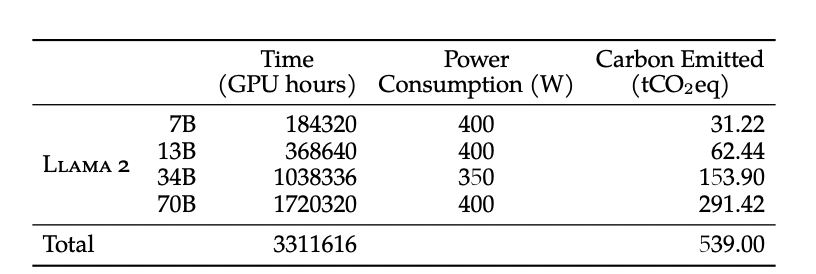
Naturally, as large language models grow larger, so do their computing and energy demands. Most of the data we have concerning the carbon footprint of AI models also does not include the energy required to run inference (which is usually when the most energy is spent) and the total lifecycle carbon footprint of the model, meaning that most of this data is roughly calculated. While the total amount of energy is overestimated in many cases, the amount of energy consumed by these models is still considerable.
🌍 So, What Does All That Carbon Get Us?
Compared to AI models that detect cancer cells or predict tornadoes, it is easy to see that generative models serve a very different purpose. Most of the allure of these models, especially ChatGPT, is the promise of efficiency and productivity. Artists can use them to streamline their creative process and as a sounding board for ideas. Salespeople can also use it to draft cold emails and generate potential sales strategies, and these are just a few use cases.
Although generative AI companies talk about the future applications of these models in contributing to an AI utopia (even though the reality is that they are very far away from achieving genuine artificial general intelligence, or AGI), I think that they should be able to stand as they are right now. When discussing the energy cost of generative models, it is therefore essential to first figure out if generative models (and their potential uses) are significant enough to warrant the amount of energy that goes into building and running them.
🎹 Putting it into Perspective: The Carbon Cost of Entertainment
With the growing mainstream popularity of generative AI models, it is impossible to deny that a lot of people around the world are not at least fascinated by them, especially since ChatGPT broke records by crossing one million users only five days after its release, surpassing social media platforms like Facebook, Instagram, and TikTok. By January 2023, just two months after its launch, it had reportedly reached 100 million active users. No doubt, there are many, many people who use tools like ChatGPT as part of their work, but the entertainment value of interacting with large language models cannot be discounted.
With these numbers, we know that ChatGPT alone serves at least tens of millions of users every month, maybe enough to justify its carbon footprint, especially compared to other forms of entertainment, according to Screen New Deal, a report on the film industry’s environmental impact, the average big budget film production produces 2,840 metric tons of CO2—over 5x the estimated carbon emissions of training GPT-3. Other popular forms of entertainment, like theme parks and significant sporting events, also have shockingly high carbon footprints. While it can be argued that they are not exactly the same as generative models, they all entertain millions of people worldwide.
👣 Is it Worth the Footprint?
There could also be a case made for the potential of generative models. While we don’t believe that they are intelligent enough to pose a severe threat to people’s livelihoods or careers, we think that they can be helpful as long as we are all aware of their limitations. ChatGPT, when used properly, would serve as an excellent teacher's or writer’s assistant, automating tasks and workflows while helping with writer’s block and serving as a sounding board for creative ideas. These types of tasks could help millions of people do their work more efficiently, increasing productivity and relative job satisfaction.
That is not to say that these companies should not be questioned or criticized for their energy consumption and emissions. As interest and funding in this field increase, so will the capacity of generative models and, in turn, their carbon footprint. A great example is the newly announced GPT-4, which OpenAI claims outperforms the previous model in every test. Because there will always be a newer, better model to be made, it is important to start considering the environmental impacts of these technologies. This calls for transparency from AI companies and an investment in minimizing the environmental cost of AI. It also requires these companies to take the pledges they’ve made (Google, Microsoft, and OpenAI have all made promises to improve energy efficiency or pursue net zero emissions) seriously and work together towards fulfilling them.
Unlock language AI at scale with an API call.
Get conversational intelligence with transcription and understanding on the world's best speech AI platform.