AI Voice Synthesis: The Early Days and Implications of Text-to-Speech
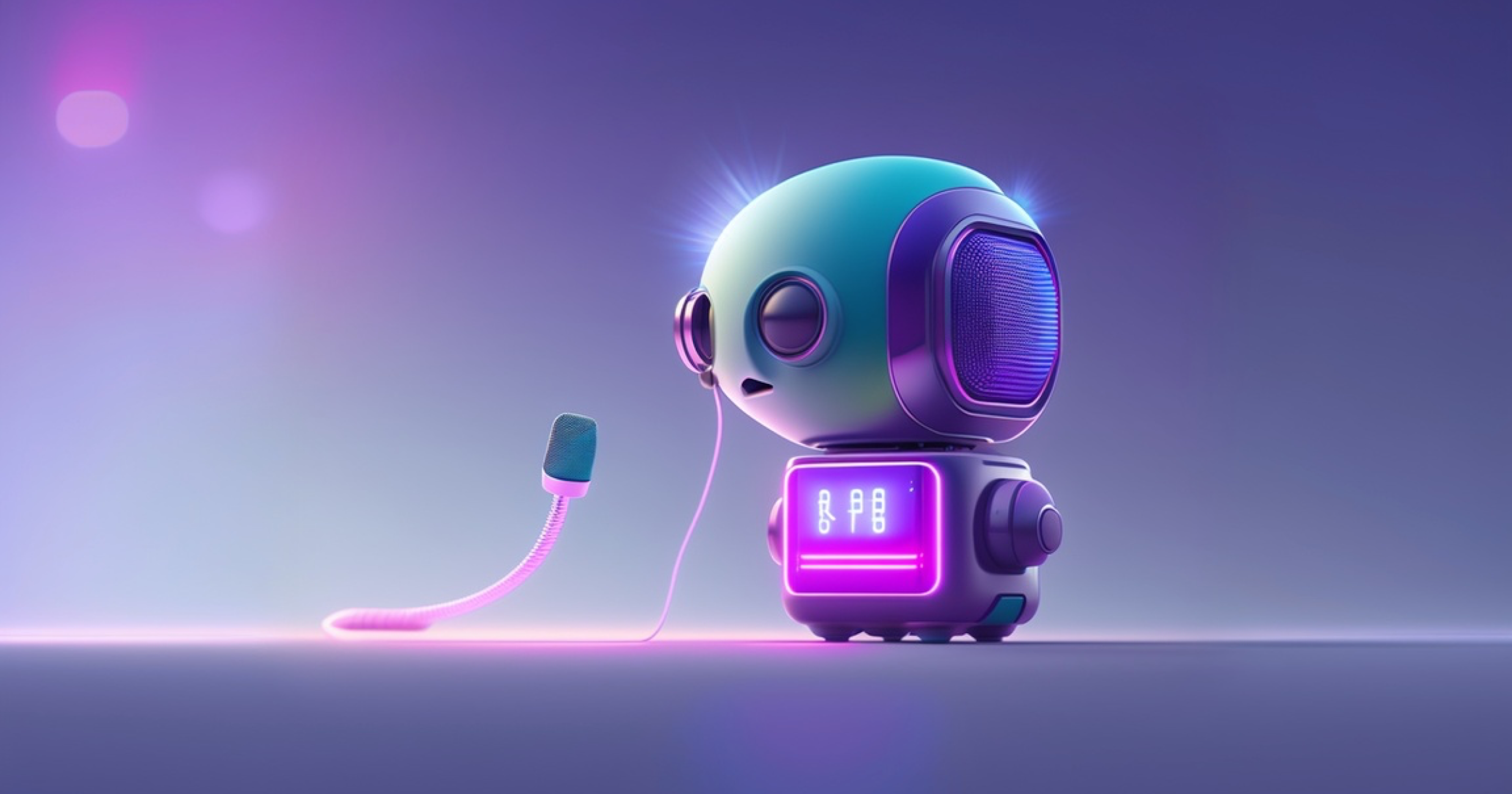
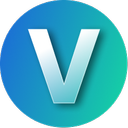
In the documentary “Roadrunner: A Film About Anthony Bourdain,” director Morgan Neville utilized older voice recordings of the acclaimed food critic and commissioned a software company to create an AI-generated version of Bourdain’s voice for three lines in his documentary. Neville’s decision brought the ire of Bourdain fans and also raised questions about the ethics of developing an AI voice based on a deceased person’s recordings. These ethical questions are just one of many surrounding plagiarism, copyright issues and more with the rapid development and improvement of generative AI.
A primary application of speech synthesis techniques is in text-to-speech systems, which convert written text into speech. Two of the earlier approaches of these text-to-speech systems are articulatory synthesis and concatenative synthesis, according to Deepgram research scientist Leminh Nyugen.
Early Text-to-Speech Systems
Articulatory synthesis synthesizes speech based on models of the vocal tract—the parts of your body involved in speaking. One such model is the source-filter model following the theory that the vocal tract filters the sound source, a movement of air, to produce different consonant and vowel sounds. The air is modeled differently to produce these different forms. Typically, linguistic experts are needed to help define this source-filter model. On the other hand, concatenative synthesis is a method to synthesize speech from smaller units, such as vowels and consonants, by sequencing them together. This form of synthesis requires a large database of speech unit recordings and the sequencing of these units produces speech that is less smooth and natural.
Ever used Siri? The first version of Siri was built utilizing concatenative synthesis. Live voice actors—Susan Bennett, in the case of Siri’s first voice—would record various sentences for hours, sometimes it was strings of numbers and other times it was sentences from Alice in Wonderland. A team of linguists would spend time analyzing these words and tagging them for a large database. Then, vowels and consonants from different sounds were combined together to form Siri’s responses.
A synthesis method that improved on both of these is statistical parametric speech synthesis. First, textual analysis occurs to generate linguistic features, such as the conversion of graphemes, the smallest unit of a writing system, to phonemes, smaller units of speech. Then, an acoustic model converts phonemes into acoustic features. In the final step of the pipeline, a vocoder converts acoustic features to a waveform, synthesizing the human voice signal. This method is more flexible in terms of adaptability and also more cost effective because it requires less expert input and entails less of the manual labor involved with recording a large corpus of speech units for a database.
More recent methods improve on this approach by reducing the number of modules involved. For example, WaveNet—released by Google in 2016—proposes a way to directly generate the waveform from linguistic features, thus removing the need for a vocoder. Other approaches can map text directly to waveform data, albeit with an occasional need for textual analysis. These new approaches result in a higher voice quality and require less feature engineering, so less expert knowledge is needed because there is a reduced number of modules.
However, as these text-to-speech systems become more advanced, what are some of the more deleterious effects of this?
Ethical Questions and Challenges Facing Synthetic Audio Today
While it might be entertaining to hear synthetic audio recordings forming a fake Drake song, these raise a myriad of ethical concerns relating to aesthetic value, copyright, creator compensation, and more. For example, Jay-Z’s company Roc Nation LLC sued YouTube to take down new songs made by programmers from Jay-Z’s existing tracks. The ability to produce new works of art without much effort and the global variation in intellectual property laws could erect future obstacles for artists who are looking to be compensated fairly for their effort.
Furthermore, voice synthesis can also directly impact the lives of ordinary people, through scammers who can extort money from individuals. A man called Regina, Saskatchewan resident Ruth Card, imitating her grandson, Brandon, and asked for money for bail. This is just one example of numerous people who have received calls from scammers imitating the voice of their loved ones. The FTC reported that 5100 incidents of impostor scams occurred through the phone resulting in $11 million worth of losses.
There are also security concerns with synthetic audio. In early 2020, a branch manager of a bank was duped into transferring $35 million worth of assets from the company to the hands of robbers because the robbers utilized a clone of the parent company director’s voice. Thus, voice synthesis calls into question the security of financial institutions. Synthetic audios could be potentially used to bypass voice authentication systems in financial institutions and like the situation with the bank’s branch manager, they could also be used to misdirect company executives to commit transfers to the wrong accounts.
Voice synthesis also has the potential to damage credible evidence in trials and law enforcement. During a custody dispute in the United Kingdom, a parent attempted to use a deepfake audio as evidence that the other parent had made violent threats, which was only discovered after analysis of the audio metadata.
With the potential for such widespread damage, what methods and detection systems have been implemented to detect synthetic audios?
Frontier Challenge: Detecting Synthetic Voices
Traditional detection methods focus on distinguishing between synthetic audios and real ones by focusing on specific features, such as how synthetic audios differ in their distribution across frequency bands. Google created their ASVspoof dataset in 2019. It contains synthetic speech produced by Google’s own deep learning models in 68 different voices from a variety of different accents to promote further research on synthetic speech detection. However, this dataset does not include the most recent iterations of text-to-speech systems, which sound much more human-like than their predecessors.
However, we are still quite far from a consistent form of synthetic voice detection, especially with how quickly synthetic audio development is advancing. There are also limited laws and regulations surrounding the development of synthetic audio: only three states address the potential implications of utilizing synthetic audio maliciously: Texas, Virginia and California.
For now, you may still be hearing Spongebob singing Creep in your social media feeds.
Unlock language AI at scale with an API call.
Get conversational intelligence with transcription and understanding on the world's best speech AI platform.