AI vs. Toxicity: Battling Online Harm with Automated Moderation

Tife Sanusi
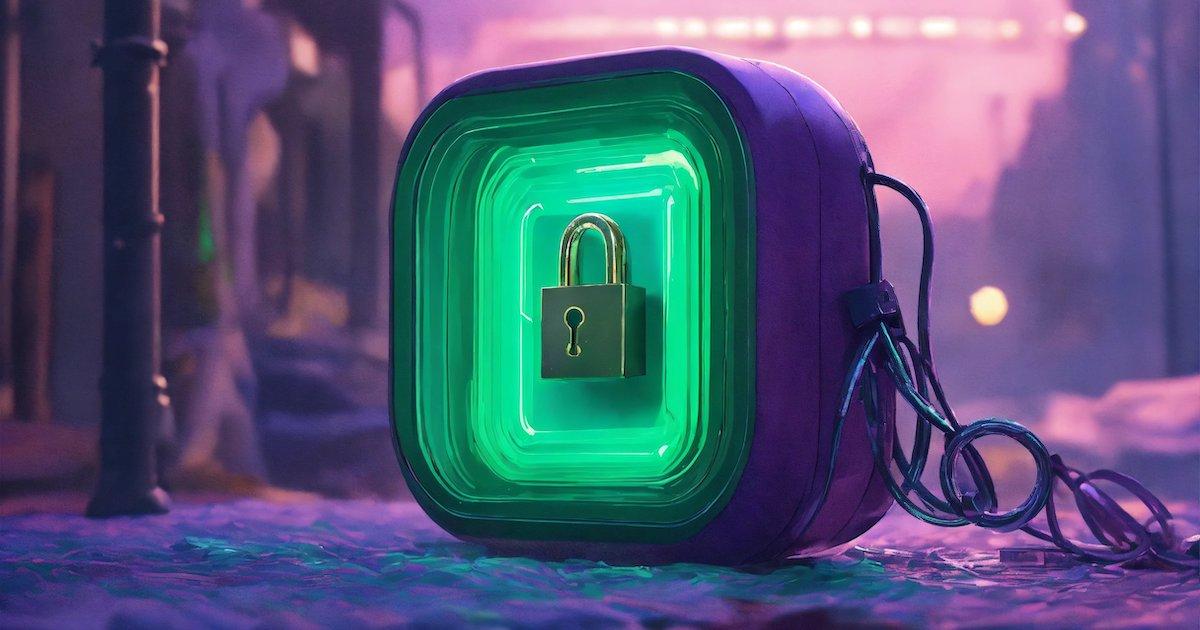
In 2019, Facebook faced a lot of heat for its failures with content moderation. The Cambridge Analytica scandal and the subsequent lawsuit had taken place the previous year, and a terrorist had just live streamed a massacre on the platform. In a bid to control this problem, Facebook ramped up its use of AI-powered content moderation, which now accounts for 95% of flagged content on the platform. More recently, the spread of COVID misinformation has highlighted the importance of moderation and the inadequacy of human moderators, as Facebook currently has over 15,000 content moderators.
As social media companies continue to grow, there is a greater emphasis on content moderation and how it can be done efficiently and ethically. Most large companies have thousands of people at any given time combing through their platforms to identify extreme and dangerous speech, harassment, and harmful videos and images. As the impacts of these platforms have skyrocketed over the past couple of years (for example, social media sites like Twitter and Facebook have been instrumental in elections and politics worldwide), so have the consequences of online harm, even beyond the platform that it exists on.
In order to maintain a relatively safe online environment, content moderators have to look through content containing graphic violence, hate speech, sexually explicit material, and violence on a daily basis. This, combined with the fact that this type of content is being produced and uploaded on these platforms at increasing rates, has made it necessary to look for an alternative solution to human content moderators. Enter AI. Some companies are now introducing AI-powered content moderation to curb some of the issues with traditional content moderation and provide a consistent approach to moderation on their platforms.
How it Works
Autonomous content moderation systems use machine learning algorithms trained on large datasets to analyze and recognize patterns in language and classify content, making them a fitting solution for automated content moderation. After the content moderation machine learning model calculates the features and outputs a score using these features, the user must first create or upload the content for automatic moderation to take place. Features are generated based on the likelihood that the content contains graphic violence, hate speech, or any other flagged content. The content is flagged as a possible rule break if the score is above a minimum threshold.
Content Moderation in Audio and Video
When elements of audio and video are added, this becomes more complicated. For audio files or content, a transcription API is first needed for speech-to-text conversion and to identify possible rule breaks. This also includes a layer of context analysis for things like tone, slang, accents, and cross-cultural differences in word usage. AI-powered content moderation requires the ability to consider all of this and proper speech-to-text transcriptions when identifying toxic content in audio files. Being able to do so will result in either overpenalization or adequate moderation. Using voice analysis (which includes speech-to-text conversion, NLP, contextual AI, and sentiment analysis) for audio moderation helps decipher the context in an audio file and identify any rule breaks.
Video moderation follows a similar routine as audio moderation, with the introduction of computer vision and generative adversarial networks (GANs) to identify banned images and manipulate content such as deepfakes, nudity, or weapons. Another tool used for video moderation is optical character recognition (OCR), which helps analyze text in images to make it machine-readable. As with audio moderation, audio elements in the video file can also be converted to text, which is then passed through a text-based moderation process.
Sentiment Analysis in Content Moderation
Sentiment analysis analyzes a given piece of content to determine if its overall emotion is positive or negative. This process is important in content moderation since, more often than not, the emotion behind the content is a better indicator of the toxicity of the content than just keyword analysis. This is usually done using a sentiment lexicon to quantify the text’s degree of positivity or negativity. The most popular lexicon, SentiWordNet, assigns three scores to a synset: positivity, negativity, and objectivity. Another commonly used lexicon, Afinn, comprises 3,382 words rated between -5 (for maximum negativity) and =5 (for maximum positivity).
This type of analysis is advantageous in cases where nuance and context are essential, which is one of the weaknesses of automated content moderation. Of course, there are some limitations with sentiment analysis, especially given the complexity of human language and how things like tone and sarcasm are subjective and differ from region to region. However, a good sentiment analysis model should be able to decipher nuances in tone and context and arrive at a score equivalent to the content being analyzed. Other limitations, like multilingual sentiment analysis, would have to be solved by training the model in each language.
Societal Implications
If there was ever a job that AI should take over, content moderation would be it, in my opinion. Many content moderators report mental health issues, including insomnia and depression, due to spending hours looking through images, videos, and descriptions of graphic violence, sexual harassment, abuse, and extreme hate crimes. Most of these moderators are also not compensated well, although it is difficult to think of sufficient compensation for this type of work. With the introduction of AI-powered content moderation, moderation teams would be able to take a break from consuming this type of content. Since this type of moderation is not yet entirely accurate, there would still be a need for human supervision, especially in complex cases.
As with all AI systems, there is a risk of replicating real-life biases and discrimination with AI-powered moderation models, ironically harming the communities that moderation is supposed to protect. This can look like the over-penalization of marginalized groups like women, the LGBTQ community, and other minority groups due to linguistic variations, sampling bias, or modeling decisions. Conducting regular audits, adding channels for community feedback, and creating more ethical keywords for bias evaluation can all help to solve this.
AI-powered content moderation could solve many problems with traditional moderation by helping with fast and standardized moderation and lifting some of the burden from human moderators. There is obviously still a need for more research and collaboration to create systems that model the guidelines and principles of the company without any bias or harm to marginalized communities.