Deepgram Completes $72M Series B Round to Define the Future of Speech Understanding
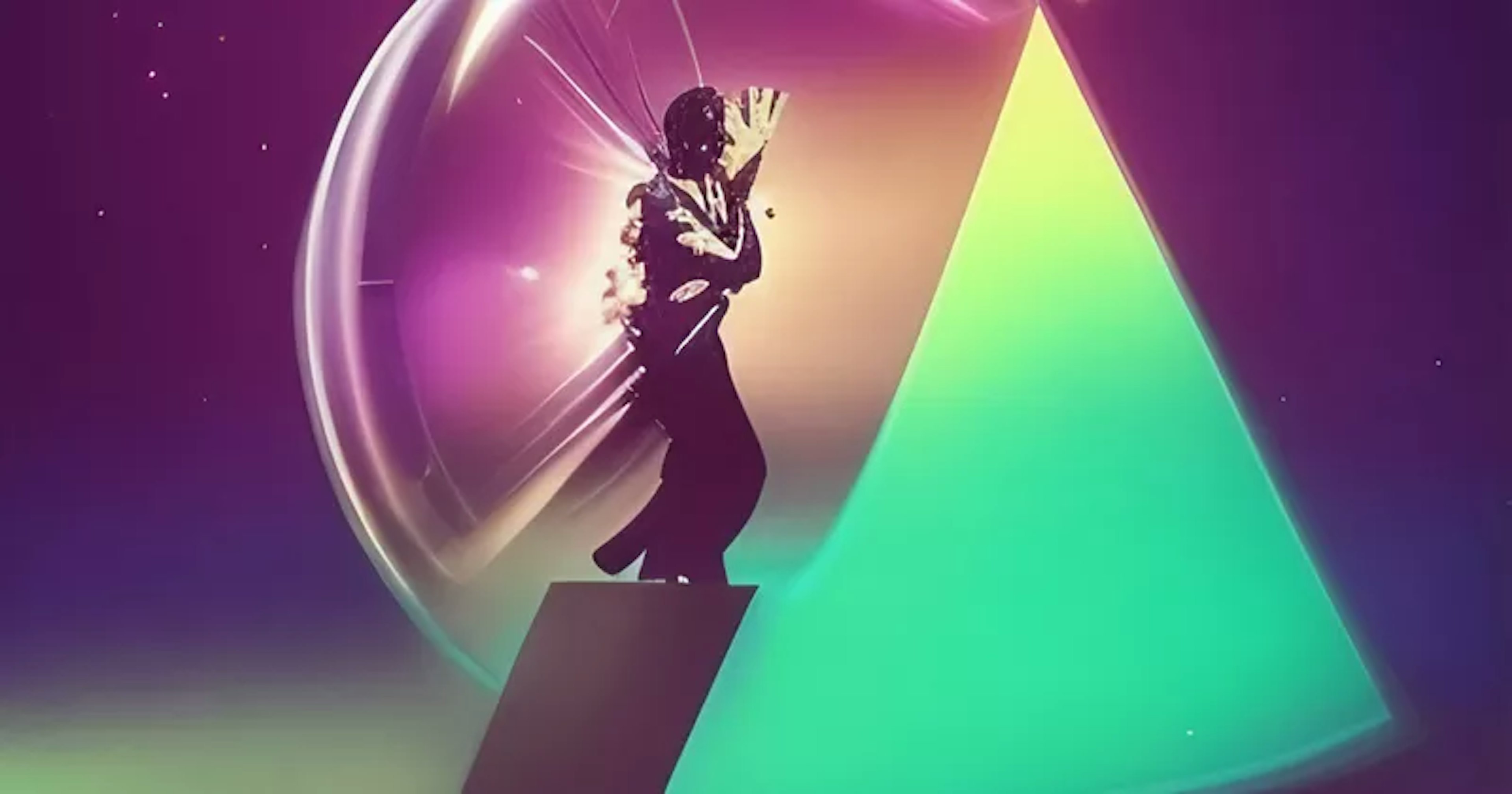
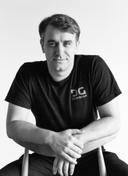
“Life moves pretty fast. If you don’t stop and look around once in a while, you could miss it.” That quote from Ferris Bueller's Day Off (1986) is a favorite here at Deepgram. We use that clip as an example file in our onboarding flow, so there’s a pretty good chance that that clip was the first thing you transcribed with our speech AI.
But there’s a reason I opened with that movie quote: it’s instructive. Deepgram, as a startup, moves very fast. It’s easy to get distracted by the thousand little things that happen every day. So in addition to announcing some new funding, I also wanted to take this opportunity to reflect on where Deepgram is today, and share a bit more about where we’re putting our focus next.
About the raise
Drumroll please…
Deepgram is thrilled to share that we’ve raised $47 million to close out our Series B round, the first tranche of which was announced back in February 2021. This brings our total Series B raise to $72 million, which makes it the largest Series B round raised by a speech AI company.
We’re looking forward to working with Karan Mehandru and the rest of the team at Madrona, who led this round of funding. Karan has been a friend of the company since before he joined as Madrona’s managing director, and we couldn’t have picked a better partner to work with as Deepgram hits its next phase.
We’re proud to say that Alkeon Capital Management provided a significant portion of the capital for this round as well. The firm’s sophisticated perspective on capital markets and commercializing deep tech innovation will be instrumental in Deepgram’s next chapter. But you don’t have to take our word for it.
Additional thanks go to returning investors, such as Blackrock, Tiger Global, Wing VC, Citi Ventures, SAP.io, InQTel, Nvidia, and Y Combinator, among others.
As a Foundational AI company, we’ll use this funding to expand our research and engineering teams to define the future of AI speech understanding.
That’s the raise. Now, let’s stop and look around.
Phase changes at Deepgram
We’ve been building Deepgram since 2015. There are many ways to think about the evolutionary phases that we’ve gone through.
There’s the startup growth cycle of “three folks and some GPUs in a basement,” to seed-funded, to “becoming a real company with real customers,” to where we’re at today: the product leader with so much room to grow—both in terms of technological capabilities and our business.
There’s also the technical story, about how Deepgram is on the fourth iteration of its speech engine, and the many considerations that went into architecting our systems over the years.
But, in general, it might be best to frame Deepgram’s progress in terms of phases:
Phase 0: We invented a way to structure and train an end-to-end deep learning model to process voice data.
Phase 1: We improved the system to produce transcripts with near-human accuracy using AI. (In certain respects, this is always a work in progress, but it’s a largely solved problem across many of the languages we work with.)
Phase 2: We’ve made those AI-generated transcripts more legible, to both humans and machines. That starts with formatting options around numbers and punctuation, and includes the ability to split conversations by speaker with diarization and capture the cadence of natural speech with utterance detection and formatting. (You can try out these features in the demo Missions of the Deepgram Console. No credit card required.)
At each phase of Deepgram’s development, we’ve made significant progress toward the goal of making every voice heard and understood. And that’s why Deepgram’s Phase 3 will be all about understanding and transforming speech at scale with end-to-end deep learning models. What does that mean?
Here’s what’s next: Phase 3
The goal is to give our users the most comprehensive understanding of what was said, how it was said, and who said it, which can hint at the bigger Why behind the What and the How.
Think about what most folks can do in conversation. That’s what we’re enabling with AI. We’ve got some exciting new speech understanding features in the pipeline and in production:
Language detection and translation will enable developers and enterprises to capture and make use of voice data from a much broader spectrum of human language.
Sentiment analysis using both semantic and tonal signals reveals the emotions expressed in an interaction, which has applications in customer service, content moderation, and elsewhere.
Automatic summarization saves on review time by pulling out the most salient points from a piece of audio, accelerating any decision-making process based on that conversation.
Speaker identification uses unique features of a speaker’s voice to make them easier to track across multiple separate interactions, automating what used to be a tedious labeling process. Speaker ID can be used to register speakers' profiles and identify unique speaker across multiple files.
Topic detection surfaces the “matter” of conversations, helping to make a corpus of speech data more structured and queryable.
Some of these features are currently available in beta, while others are still not quite ready for public testing (but will be soon). Expect a more complete rollout over the next few months. Don’t blink or you’ll miss it.
Beyond the first trillion words
Voice is the dark matter of enterprise data. Trust me on this… I have a PhD in particle physics and worked two miles underground architecting systems to detect dark matter with deep neural networks. And I left particle physics to tackle what feels like a bigger and more tangible problem: transforming dark data into something both humans and computers can understand.
Looking at legacy workflows, it’s easy to understand why voice data remains a dark pool of value for most enterprises. Human-powered transcription is very expensive to do, both in terms of time and money, even at small scale. And you can still expect a baseline human error rate between 3-5%. Use one of the legacy speech recognition solutions and you’re saddled with even more error-ridden transcripts which require a painstaking review-and-revise cycle to correct. This is decidedly not the way.
We already offer the fastest, most accurate, and cost-effective speech AI models on the market. Now we're extending past transcription that tells you what was said, to understanding that tells you why it was said. And, in classic Deepgram fashion, we want to engineer it properly so that we remain the market's first and best choice for speech AI. That means delivering additional, computable speech understanding metadata built into transcripts all while being 100x less expensive than human transcription and at least half the cost of any alternative, including open source speech AI.
Since launch, Deepgram has transcribed over 1 trillion words. We know there are many trillions more that are waiting to be pulled from the noise and understood with unprecedented accuracy and contextual dimensionality. Voice intelligence is out there, and we’re here to help any developer at any company discover its value with just an API call. You bring your voice. Deepgram delivers the intelligence.
If you have any feedback about this post, or anything else around Deepgram, we'd love to hear from you. Please let us know in our GitHub discussions .
Unlock language AI at scale with an API call.
Get conversational intelligence with transcription and understanding on the world's best speech AI platform.