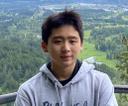
In the bustling realm of artificial intelligence (AI), small startups are often seen as the birthplaces of fresh, innovative ideas. But they face a big challenge: defensibility. Unlike large tech companies, these smaller players often lack the resources to defend their market position. Big names like Google, Amazon, and Microsoft have vast amounts of data, money, and established reputations that naturally defend their market spot. They can attract top-notch talent and have the financial strength to either build or buy innovative AI solutions. This scenario creates a steep hill for small AI startups to climb if they aim to carve out and defend their own space in the AI market.
Lack of Resources
Aside from many of the business and connection perspectives of an AI startup, attempting to match big companies’ technical compute resources is near impossible. For example, the training of GPT-4 cost more than $100 million. For any smaller startups trying to dip their toes in the LLM space, the competition that they are facing is beyond what most small companies can handle. It’s difficult to get investors excited without results, but without funds for training large scale models, there never will be results.
Aside from compute resources, in the field of Machine Learning, the data is as important, if not more, than the model itself. Many larger tech companies have their own proprietary datasets or spend large sums of money to license datasets from third-party providers. The sheer scale of resources that large companies like Google, Amazon, and Microsoft possess enables them to tackle complex AI projects with an ease that is unattainable for startups. The training of advanced models such as GPT-4 is a testament to the financial muscle these corporations flex. The vast datasets that these companies have accrued over the years provide a bedrock for training sophisticated machine learning models, further sharpening their competitive edge. For instance, Google’s access to vast amounts of data through its search engine, YouTube, and other platforms gives it a distinct advantage in training AI models.
Imagine a group of skilled and ambitious sailors who have meticulously planned a voyage to discover new lands. They have the maps, the knowledge, the vision, and the fervor to embark on this daring journey. However, they lack a robust ship and the necessary provisions to undertake the voyage. They find themselves on the shores, gazing at the vast ocean that holds promise, but are stranded without the means to set sail.
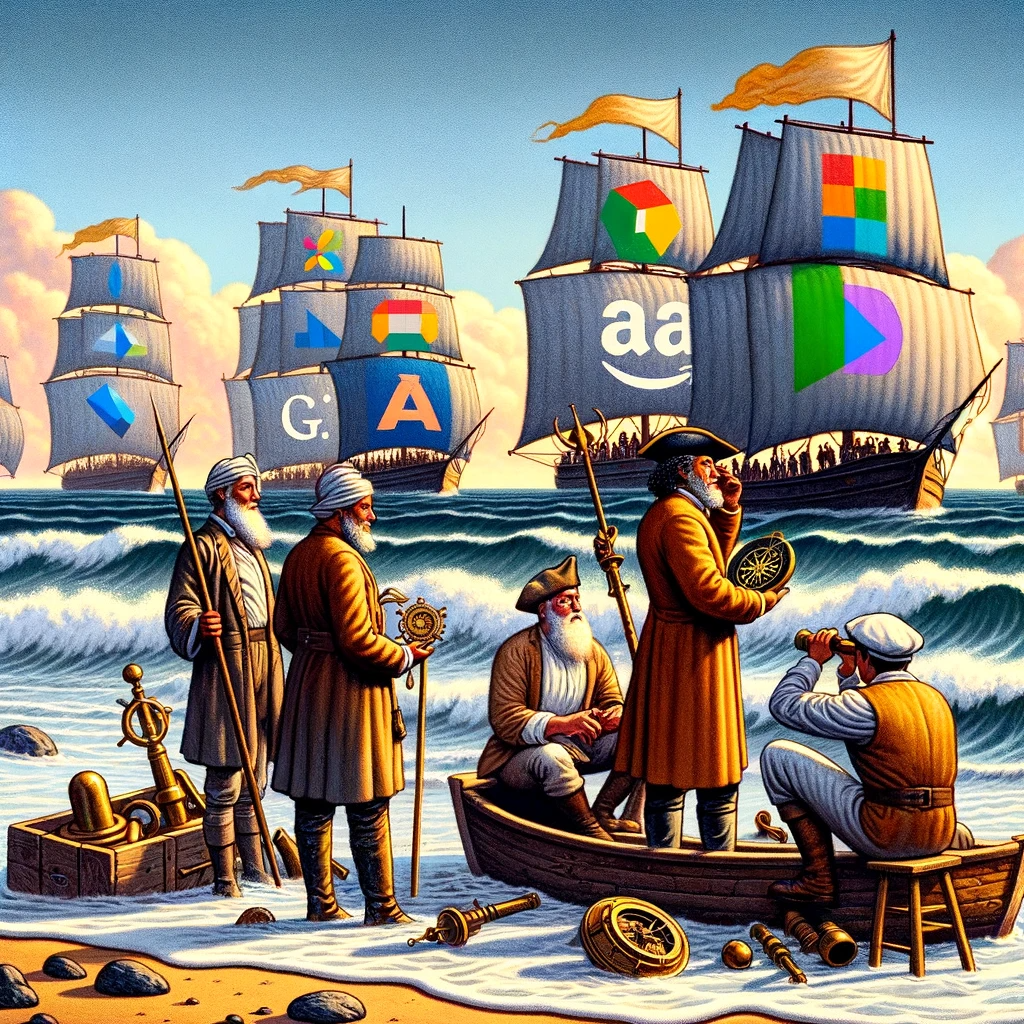
Source: DALL-E
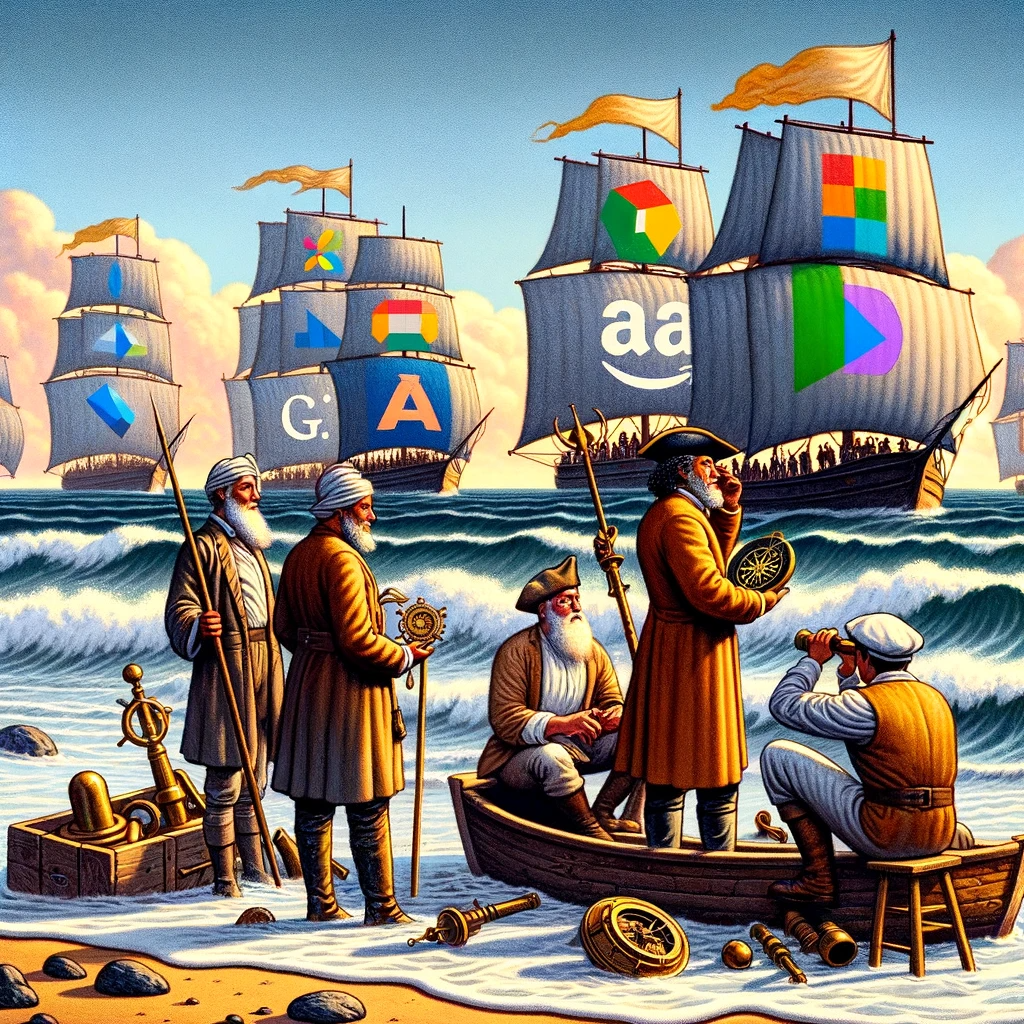
Source: DALL-E
In a similar vein, AI startups often house brilliant minds, brimming with innovative ideas and the drive to explore the unchartered territories of artificial intelligence. They hold the maps to potential breakthroughs and have a clear vision of the destination they want to reach. Yet, the lack of resources—akin to the sturdy ship and essential provisions in our metaphor—hinders their journey into the vast, promising yet turbulent seas of the AI realm. Without the necessary data to train their models (the food and water for their journey), and the computational resources (the sturdy, well-equipped ship), they find themselves stranded on the shore, able to see the boundless potential that lies ahead but unable to move towards it. Meanwhile, the large tech companies with their colossal ships and abundant provisions are already well on their way, charting the course and claiming the new lands.
A Turnaround for Startups
However, this is not to say startups have absolutely no chance of competing with larger companies from a technical standpoint. There are many public datasets available to use. For example, AlphaFold, the pinnacle of automated protein structure prediction, was mainly trained on two large public datasets: the Protein Data Bank (PDB) and UniProt. Moreover, startups can leverage datasets like ImageNet or UCI Machine Learning Repository, which are openly accessible and provide a vast array of data from diverse domains. This accessibility to public datasets can be a game changer for startups, leveling the playing field to some extent.
Furthermore, while large companies have the advantage in terms of data and compute resources, startups often have the upper hand when it comes to agility, innovation, and focused expertise. Their smaller size allows for quicker decision-making processes, less bureaucratic inertia, and a closer relationship with their customer base. This enables a faster response to market changes and customer feedback, which is crucial in the rapidly evolving field of AI.
Startups often have a closer rapport with their customers, which can be leveraged to co-create solutions and gain invaluable market insights. This close interaction enables a better understanding of market needs and a quicker response to customer feedback, which is crucial for product development in the AI domain.
The narrower focus of startups often translates to a deeper level of expertise in their chosen domain. This focused expertise can lead to superior solutions and a better understanding of their target market’s pain points. ElevenLabs, for instance, has pioneered text to speech AI, with their models revolutionizing the text to speech space: their models generating voices nearly indistinguishable from authentic audio along with emotions and sentiment.
Traditional Challenges
Other than the lack of resources in the AI space, traditional challenges that tech startups face applies the same to AI startups, if not further amplified. With the AI hype growing at an unprecedented rate, many startups are hoping to hop on the hype train and snatch a piece of the pie.
Talent Acquisition and Retention
Acquiring top-tier talent is a difficult task for AI startups, especially when competing against the allure of big tech companies. For instance, Google, with its deep pockets, managed to hire Geoffrey Hinton, a pioneer in deep learning, as a Google Fellow. This not only bolstered Google’s AI expertise but also showcased its ability to attract world-renowned talent. AI startups, lacking similar financial prowess, may find it challenging to attract such high-caliber professionals. Furthermore, the retention of talent can also be problematic. Big tech companies often poach skilled individuals from successful startups, offering them more lucrative packages and a platform with vast resources.
There are startups, for instance, like Fixie, founded upon talents from big tech companies and thus do not face problems. Other startups attract talents through offering a more generous pay than larger companies would for positions of the same level. However, their offer typically comes with great risk and job instability. Although by raising the pay, startups are able to grab the attention of some people, others still stick with bigger corporations for its stability.
Then again, the recent tech job market definitely isn’t at its peak, with major layoffs in large tech companies starting from 2022, continuing to this day. Startups can take this opportunity to attract talent that may be disillusioned with the instability of larger firms. Individuals affected by layoffs, especially those with a penchant for innovation and risk-taking, may find the dynamic and impact-driven environment of startups more appealing.
In this shifting landscape, startups have the chance to showcase the benefits of a flatter organizational structure, a more significant potential for individual impact, and the flexibility that larger companies often lack. By emphasizing these aspects and offering equity stakes or profit sharing, startups can turn the tide to their favor, not just as a stopgap for these professionals but as a long-term career destination that aligns with their personal growth and values.
Market Dynamics and Competition
The market dynamics heavily favor established players. A pertinent example is Amazon’s foray into the grocery sector with the acquisition of Whole Foods, which immediately posed a threat to smaller startups in the sector due to Amazon’s established distribution network and customer base. Similarly, in the AI domain, when big tech companies decide to venture into a niche area, their vast resources and brand recognition can overshadow startups. For instance, if a startup is developing a novel AI-driven healthcare solution, and Google decides to enter the same space, the competition can become overwhelmingly skewed.
Additionally, companies such as NVIDIA and H2O.AI has a well established reputation
in both the amateur and professional Artificial Intelligence community. NVIDIA’s Kaggle Grandmasters team, composed of top data scientists, competes in challenges that push technological boundaries, such as a recent American Sign Language recognition contest won by team member Christof Henkel, with the prize money supporting charitable causes. These achievements underscore NVIDIA’s commitment to societal impact through AI and enhance its market position by attracting top talent and demonstrating thought leadership in AI development.
This strategic engagement in community-driven AI competitions serves as both a marketing tool and an incubator for innovation, allowing companies like NVIDIA to showcase their capabilities and commit to solving real-world problems, which in turn reinforces their reputations as employers of choice and leaders in AI technology.
Strategic Missteps
Strategic missteps can be particularly detrimental for startups. For instance, choosing the wrong market segment or pricing strategy can lead to a startup’s demise. On the other hand, big tech companies have the financial cushion to recover from such missteps. They have the resources to reiterate and relaunch products, something that Quibi, a startup in the streaming space, didn’t have when faced with challenges post-launch. Their failure to gain traction led to a swift downfall, while big tech companies like Netflix have weathered numerous strategic missteps over the years, adjusting their strategies based on market feedback and continuing to thrive.
Lack of Diverse Skill Set
The need for a diverse skill set is paramount in navigating the complex challenges of AI. Big tech companies often have teams with a mix of skills including data scientists, engineers, UX designers, and domain experts. In contrast, a startup may have a limited team, possibly lacking in domain expertise or user experience design. For example, a startup focusing on AI-driven financial solutions may have a team of excellent engineers and data scientists but lack the necessary regulatory or financial domain expertise, which a big tech company like IBM or Google could easily onboard due to their resources.
Defensibility Strategies
This is not to say all AI startups are bound to fail, there are various defensibility strategies that startups can tackle in order to stand a chance against larger corporations.
Niche Specialization: Startups can carve out their niche by specializing in a specific industry or problem set where they can apply AI in a way that big tech companies have not fully addressed.
Agile Product Development: AI startups should leverage their agility to iterate quickly, tailor their offerings to the market’s needs, and adapt to feedback faster than their larger counterparts.
Collaborations and Partnerships: Establishing partnerships can help startups overcome resource limitations. For example, collaborating with academic institutions for research and development can be a cost-effective strategy for innovation
Customer-Centric Solutions: Small AI startups can focus on customer-centric solutions and capitalize on their ability to closely engage with customers for co-creation and customization of products.-
Competing in AI-native Product Categories: Focusing on product categories that are inherently AI-driven can provide a competitive edge. AI-native products like search engines, recommendation systems, and specialized legal or medical technology tools can significantly benefit from generative AI innovation.
Feature Density Differentiation: Startups can distinguish themselves by offering a rich set of features rapidly, exploiting the speed of innovation in AI technology. The focus on feature density and quick time-to-market can set them apart from larger companies that may be slower due to compliance constraints and bureaucratic processes.
Owning New Product Categories: By identifying and solving emerging problems, startups can establish themselves as leaders in new product categories. This proactive approach can position them ahead of larger players who may not be as quick to recognize and act on these emerging needs.
Use of Open Source and Public Datasets: Leveraging open-source software and publicly available datasets, such as the Protein Data Bank or the UCI Machine Learning Repository, can help startups bypass some of the high costs associated with proprietary data and tools.
Innovative Monetization Models: Exploring alternative monetization strategies, such as freemium models, subscription services, or pay-per-use, can attract customers who are not well-served by the pricing models of larger companies.
Focus on Talent Recruitment and Retention: With the recent layoffs in big tech companies, AI startups have the opportunity to attract top talent who may prefer the culture and dynamism of smaller, innovative companies.
The Future of AI Startups
In the dynamic world of AI, the battlefield isn’t reserved for giants alone. AI startups, while smaller, pack a punch with their swift moves and bold innovations. They don’t just survive; they can thrive by playing their cards right — focusing on unique strengths, staying close to their customers, and turning the market’s volatility to their advantage. Their journey may be tougher, with resources slim and the competition fierce, but it’s their agility and creative edge that could write them into the annals of AI history. Like nimble boats amidst industry titans, they can find their own path to success, proving that in the AI odyssey, ingenuity and adaptability are as valuable as size and strength.
Unlock language AI at scale with an API call.
Get conversational intelligence with transcription and understanding on the world's best speech AI platform.