Listening to Forests with Machine Learning & Algorithms
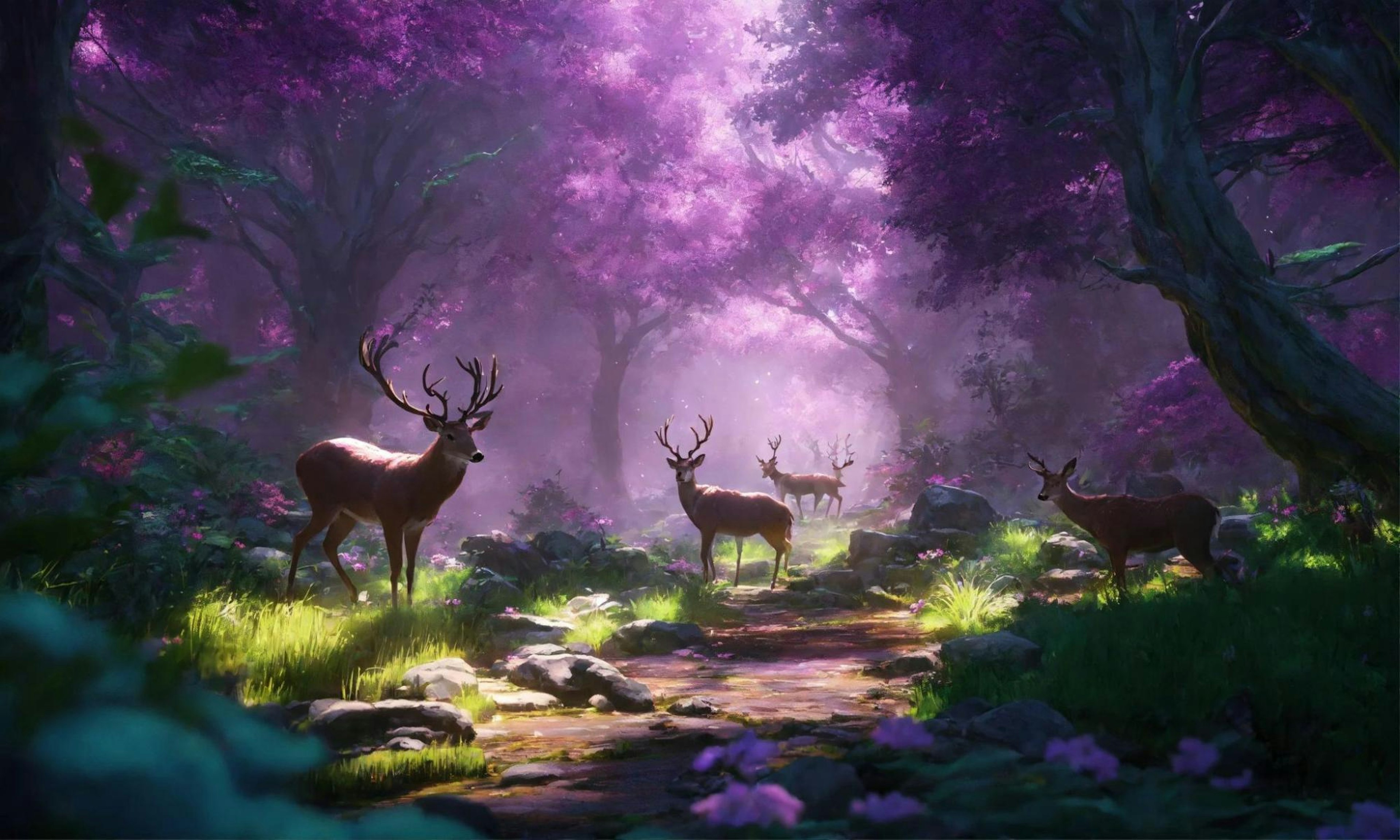
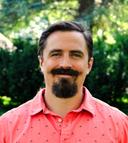
If you stroll deep into an undisturbed forest, plop yourself down somewhere comfy, and open your ears, you can learn a lot.
It may take a few tries, but, eventually, you'll notice Mother Nature's organisms—ranging from her insects like cicadas, crickets, and katydids, to frogs and toads, to songbirds, to mammals like squirrels, monkeys, African forest elephants, and more—all contribute to a wild orchestra.
Once you develop an ear for a forest's cadences and frequencies, you might also notice that, upon obnoxiously intruding into some new thicket, a sudden, almost eerie silence replaces that forest's vibrant song (if you enter slow and stealthy, the forest might not clam up).
When a Forest's Music Fades
This effect is so pronounced that the United States military (and I assume most militaries) teaches its soldiers to listen to forests for life-saving clues when they patrol through the woods. (This practice, Stop-Look-Listen-Smell, is like a sensory-based mindfulness exercise that an entire unit partakes in to, ideally, detect when something is amiss.)
If you're presence triggers a forest to turn down its sound dial, its baseline symphony gradually returns if you just sit still for around five to ten minutes. Once those sounds resume, you can interpret subsequent changes in the forest's baseline noise as indicative of activity elsewhere. An enemy patrol nearing your position, for example, is apt to spook the closeby bugs, frogs, birds, and mammals back into silence again (a predator, say a tiger, in proximity might induce the same forest-silencing effect that humans do). This means that in thick foliage, you'll often hear clues of danger before seeing any.
The same holds for our forest conservation efforts. Bioacoustics, the study of individual organisms' use of sound, and ecoacoustics, the study of entire ecological “soundscapes,” are increasingly helping researchers better understand the health of forests and their inhabitants by "listening" to forests at scale (in addition to other biomes).
Narrow vs. Broad Listening
Bioacoustics has been applied to goals as diverse as decoding Killer Whales' complex language, monitoring elusive African forest elephants (which we'll look at later), and testing whether embryonic sea turtles coordinate their hatching times via sound (some evidence suggests they do). Ecoacoustics has been applied to goals ranging from monitoring noise pollution in cities to quantifying forest recovery efforts (we'll also learn about this later) to measuring biodiversity in restored ponds. Both of these fields shine in limited-visibility environments like deep oceans and thick forests, where cameras' and satellites' contributions are limited.
Though bioacoustics and ecoacoustics differ along their scope of inquiry—bioacoustics generally studies individual species's sounds, whereas ecoacoustics generally studies all the sounds emanating from an entire ecosystem (usually numerous species at once)—these two field's subjects of study and their tools and techniques largely blend together.
Both fields, for example, often employ passive acoustic monitoring devices (i.e., listening devises), spectrograms (visualizations of sound frequencies across time), and machine learning algorithms to study organisms' and ecosystems' sounds. Since both fields are strongly intertwined but listen to forests with slightly different goals in mind, let's look at a bioacoustics and an ecoacoustics study aimed at saving our dwindling forests and their inhabitants.
Tallying Up African Forest Elephants with Bioacoustics
Let's start narrow, exploring a bioacoustics study aimed at a single forest-dwelling species—the African forest elephant.
Because they live in dense forests, satellite imagery, drone footage, and tree-mounted cameras are of little use for monitoring African forest elephants. You might think trained observers dispatched into the forests where these elephants live could be useful, but, despite their size, African forest elephants are surprisingly good at evading human researchers (plus, you can't deploy enough trained human observers to continuously monitor an entire rainforest, and even if you could, that many humans tromping around would seriously disrupt that forest's organisms).
To overcome these challenges, pre-bioacoustics researchers attempting to monitor African forest elephant populations often relied on extrapolating from visual clues that weren't exactly easy to spot—like elephant dung piles scattered around the forest floor.
Extrapolating Population Size from Dung Piles
This old-school forest-elephant-census-by-counting-dung-piles method was wrought with challenges. Because it wasn’t feasible to scour an entire forest floor for elephant dung, researchers limited their search to a subset of the forest and extrapolated from that. Adding to this implementation challenge, African forest elephant dung piles are found at a higher density the further away you get from human roads, and the math needed to account for this is messy enough that it's often overlooked.
To overcome these elephant counting woes, director of the Elephant Listening Project and Cornell behavioral ecologist Peter Wrege implemented a more effective method than counting piles of poo—an acoustic census.
Beyond an Elephant's Reach: Hanging Listening Devices High Up
Here’s how they did it. First, Wrege’s team hung about 50 recording devices from trees about 10 meters up (beyond an elephant's feeding range and a poacher’s gaze), spaced apart about one per 5 square miles across 580 square miles in the Republic of Congo's Nouabalé-Ndoki National Park. Then, three months later, they hacked their way back through the forest to retrieve the listening devices' memory cards, harvesting around 100,000 hours of forest audio data.
If deploying and fetching acoustic sensors from the dense forest wasn't strenuous enough, Wrege faced a follow-on challenge common to many modern machine learning problems—parsing the signal from the noise (in this case, the signal Wrege sought was elephant vocalizations and the "noise" was everything else—other organisms, wind and rain, passing airplanes, humans, gun shots, vehicles, etc.). Sparse elephant vocalizations could be hidden anywhere within the trove of recordings Wrege collected, and 100,000 hours is a lot of audio to sift through manually—even if Wrege enjoyed an army of research assistants with ears finely tuned to detect African forest elephant vocalizations.
Spectrograms, Human-Labeled Data, and Deep Learning to the Rescue
So Wrege's team turned to spectrograms and deep learning, both staples of bioacoustic research. Spectrograms are visualizations of sound frequencies across time. An African forest elephant's deep "rumbles" mapped to a spectrogram, for example, look like the following:
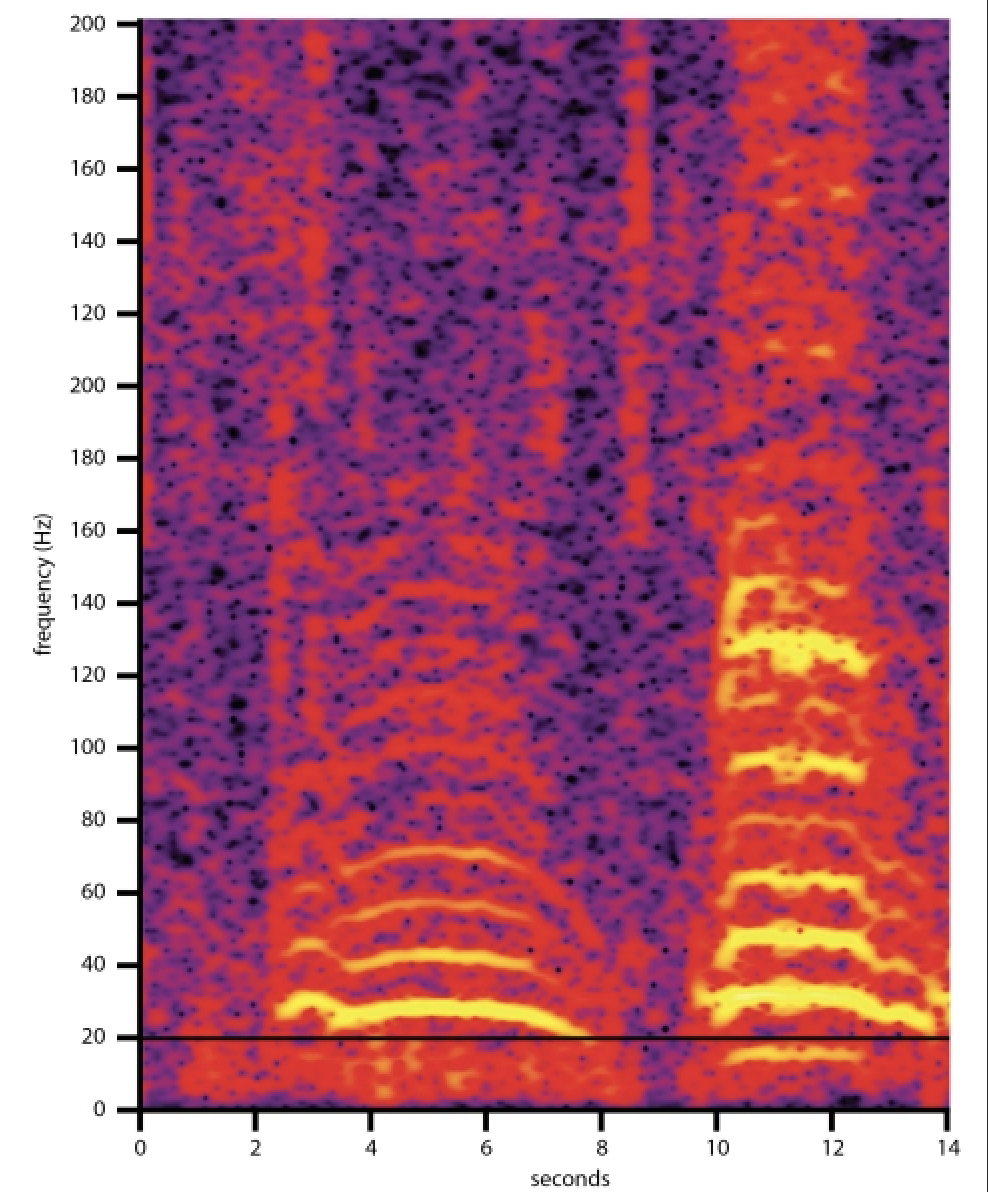
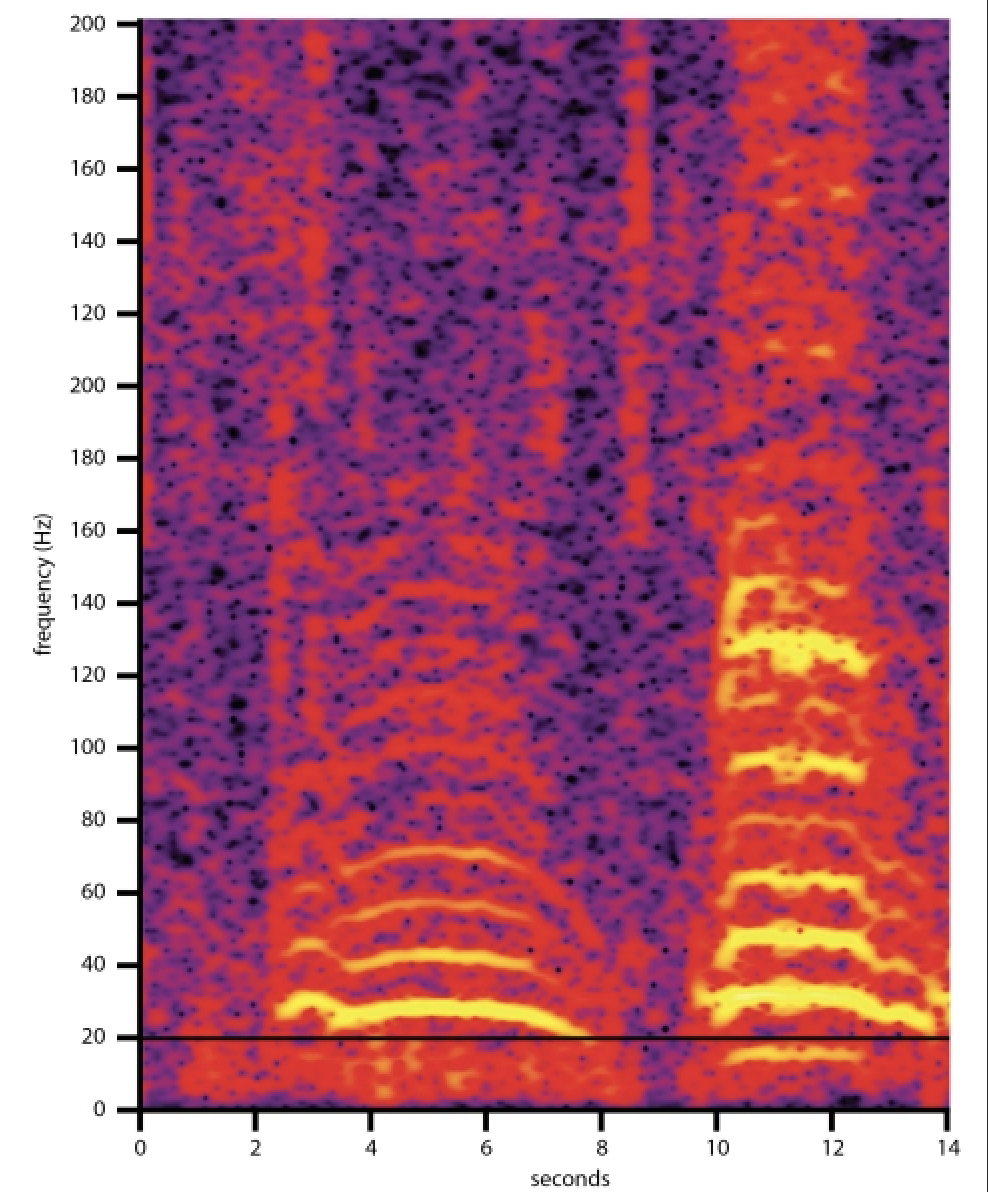
Spectrograms are often crucial in bioacoustics because different species' sounds (and even different sounds from the same species), when mapped to a spectrograph, often exhibit signature patterns that humans and computer vision algorithms can easily identify. Each sound’s unique appearance on a spectrogram improves the accuracy and speeds up the process of classifying recording segments as specific calls from specific species (e.g., spectrograms of an African forest elephant's "scream," "snort," or "trumpet" appear different from the forest elephant's “rumble” above, and all of these would look different from an orangutan "kiss squeak").
Video Source: The Elephant Listening Project (Video of a African forest elephant screaming and its corresponding spectrogram)
Collaborating with bird researcher and Conservation Metrics CEO Matt McKown, Wrege's team converted all their forest audio recordings into spectrographs and trained a neural network on a human-labeled dataset. This was a binary, elephant-sound-or-no-elephant-sound classification task, but even labeling sufficient data to train a neural network on took significant time since humans first needed to be trained to accurately identify elephant sounds before they could accurately label audio clips as "elephant" or "no-elephant." Once trained, however, this neural network elephant sound classifier saved the researchers a lot of time, allowing them to analyze the 100,000 hours of forest recordings within 22 days (this was all back in 2017, so it would be much faster on current hardware).
Inferring Elephant Behavior from Sound
With a usable elephant-sound classifier, Wrege's team accurately parsed elephant vocalizations from multiple recorders' data, even triangulating elephants' locations when enough monitoring devices picked up the same elephant call.
The combination of sound and location data allowed Wrege et al. to infer several African forest elephant patterns of life, like how many elephants occupied specific swaths of the forest and where they roamed across time, a non-trivial contribution given how tough observing African forest elephants is.
Finding a Gunshot in a Haystack (of Recording Data)
What's more, Wrege's team noticed gunshot sounds in their recordings. Within the entire 100,000-hour collection, gunshots were (thankfully) even more sparsely distributed and shorter in duration than African forest elephant vocalizations, making hand-labeling gunshot recordings for the entire recording body impractical. Instead, Wrege et al. trained a gunshot detection algorithm using a collection of known gunshot recordings and then tested this detector on a sample of 2400 hours of recording data where humans did verify all gunshots. Their gunshot detector identified 94% of the actual gunshots within the verification sample.
Satisfied with its accuracy, Wrege’s team ran their gunshot detection algorithm on all the recordings. Then, by combining this gunshot data with known anti-poaching patrol data, Wrege et al. discovered that poachers were more active in certain areas than previously thought, which helped park rangers adjust their foot patrols for more effective coverage.
When Logging Frequencies and Elephant Frequencies Clash
Wrege's team also found that sounds emanating from logging operations hampered African forest elephants' ability to communicate (i.e., "communication masking") when the elephants were over 15 meters apart and within 800 meters from a logging site, a concerning finding given how heavily African forest elephants rely on sound to find each other in the dense forest. Researchers and policymakers can use these types of findings, along with elephant location data, to guide where they’ll issue future logging permits.
The Need for Speed (and for On-Device Detection)
As much progress as Wrege and follow-on researchers made in tallying up African forest elephants and inferring their behavior with the help of bioacoustics, researchers still face significant challenges. One is developing machine learning models simple enough to run locally on the recording devices. Another hurdle is making the data analyses intuitive enough for conservation and anti-poaching practitioners to quickly interpret and react to.
Existing elephant sound detection methods are slow and compute-intensive. Conservationists and park rangers can't, however, exactly twiddle their thumbs, waiting around for sound recordings to finish processing in a far-off lab if they want to use that information to, for example, respond promptly to sounds of gunshots or sounds of elephants nearing a village (preventing human-elephant conflict is a big piece to the elephant conservation puzzle too). With the rapid progress of machine learning models and hardware, on-device models that can quickly broadcast detection events seem inevitable, but, especially for endangered species, we need them ASAP.
Monitoring Entire Forests with Ecoacoustics
Applying bioacoustics to count African forest elephants and study their behavior was a complex undertaking. Considering that Wrege et al.’s study only focused on a single species, how can an ecoacoustics approach possibly hope to listen to a forest's many vocal organisms all at once?
To pull this off, ecoacoustics focuses its inquiry on entire "soundscapes," recordings of all an environment's sounds. In Wrege et al.’s African forest elephant study, the soundscape would be the raw 100,000 hours of recordings before researchers applied elephant or gunshot sound detection algorithms to parse out specific sounds.
Sound Categories
Soundscapes typically include the following three sound categories:
Anthrophony: human-derived sounds like vehicles, music, talking, chainsaws, or gunshots
Geophony: earth or weather-derived sounds like rustling leaves, the trickle of a running brook, or the patter of rain drops
Bioophony: non-human, organism-derived sounds from the many forest-dwelling invertebrates and vertebrates whose stridulations and vocalizations many of us recognize
Ecoacoustics approaches consider all of these types of sounds important because, among other things, they might:
indicate poaching or illegal logging activity
identify endangered or invasive species
denote forest recovery (or lack of it)
measure biodiversity
suggest weather's impact on specific organisms
help count a species' populations
To get a better idea of how ecoacoustics illuminates what goes on within forests, let's take a look at a recent study that quantified forest recovery. First, though, let’s address why the popular method of counting trees to measure forests’ health doesn’t quite hack it.
Misguided Tree Tracking Obsession
Because it's increasingly apparent that human activities like logging, mining, and agriculture are threatening our forests, one of the earth's best carbon capture technologies, researchers and organizations are launching herculean efforts at forest preservation and restoration. Much of this effort (understandably) obsesses over trees.
Focusing reforestation efforts strictly on carbon capture goals without monitoring biodiversity can, however, incentivize monoculture plantations; planting rows upon rows of the same type of tree might help capture carbon but does little to restore a degraded forest's biodiversity.
Thanks to satellite imagery and the fact that trees are stationary, quantifying how quickly trees regenerate during forest recovery programs is quite straightforward. What's less clear (and often overlooked) is how to measure biodiversity recovery, an important metric given that forests brim with life (especially the dense tropical forests that house 62% of our world's land-based vertebrates).
Sounds of Stress
When a forest suffers, since its inhabitants suffer along with it, that forest’s soundscape leaves us with useful acoustic clues. If we listen to these, we can gauge both the damage done to a forest and its recovery.
For example, so many forest organisms normally sound off together at dusk and dawn that a healthy forest's chorus "blends" together, making it tough to distinguish between species. This phenomenon, “spectral saturation," however, diminishes in weakened forests. Additionally, recovering forests' insect soundscape saturation rises at night (perhaps because some insects' predators aren't as numerous as they once were, leading to more insects in damaged forests than healthy ones). Yet another indicator of stressed biodiversity is that some male songbirds stop singing in proportion to increased logging activity, suggesting they have less food and fewer mates post-logging than they did pre-logging.
If we consider that these are just a small sample of the many acoustic hints forests can reveal to us (if we listen), it becomes obvious that measuring a forest’s recovery solely by counting trees neglects many vital indicators of that forest’s health.
The Ecoacoustics’ Toolbox
Ecoacoustics offers researchers several approaches to track dips and rises in forests' biodiversity. Ecoacoustics’ most specific approach is tracking individual species' vocalizations. Ecoacousticians might use these species-specific methods to study, for example, an endangered species (e.g., African forest elephants) or to monitor invasive species (e.g., cane toads in Australia). This is a key area where bioacoustics and ecoacoustics blend together.
Where the two fields diverge is in ecoacoustics’ broader tools. The broadest is the "acoustic index," a mathematical formula often designed to benchmark how biodiverse a sound sample is by considering all sounds in a forest (the insects, amphibians, birds, bats, mammals, humans, running rivers, rustling leaves, etc.). Acoustic indices quantify biodiversity by measuring soundscape parameters, like:
signal-to-noise ratios (the strength of a target acoustic signal compared to the background noise)
frequency variations (how many frequency types are present in an audio sample)
sound complexity (how many different sounds exist within an audio sample)
entropy (the declining variety of sounds in audio samples)
In between the narrow species-level analysis and broad whole-forest-level analysis is "soundscape partitioning." By harnessing assumptions of the "acoustic niche hypothesis," researchers often imperfectly divvy up frequency ranges present in a soundscape into the broad taxonomic groups that utilize them (e.g., in this study, birds’ and insects’ frequency ranges had clean boundaries while amphibians’ and mammals' frequency ranges overlapped each other).
A drawback of this method is that it requires expert knowledge of what frequency ranges various organisms use, a luxury we often lack. Undiscovered species and known species, for example, both create sounds that we're not yet familiar with. Guerrero et al. recently helped overcome this conundrum by handing this placing-organisms-into-their-respective-frequency-buckets process entirely over to machines. To do this, they used an algorithm designed for multivariate sound analysis, the LAMBDA 3pi algorithm, which clustered numerous species into groups, accurately recognizing species across four test datasets 75% to 96% of the time. This approach offers a biodiversity assessment similar to conventional acoustic indices while bypassing the need for human experts to divide the sound spectrum into taxonomic groups.
Though imperfect, soundscape partitioning approaches often prove useful. They have, for example, noticed that logged forests are more likely to have stronger insect vocalizations at night and less synchronized bird vocalizations at dawn and dusk than unlogged forests are.
Quantifying Biodiversity in Recovering Forests
To address the often overlooked acoustic aspect of forest recovery, Müller et al. recently tested if ecoacoustic tools could measure biodiversity in an Ecuadorian forest recovery project. To do this, they recorded soundscapes (with recording devices) and sampled insects (with light traps) in 43 land plots of the following types:
active cacao agriculture plots (with few, if any, native trees remaining)
secondary forests (1 to 34-year-old recovering forests reclaiming old agricultural plots)
OG forests (Old Growth forests with original trees—the baseline of forest health for this experiment)
From these recordings, Müller et al. manually labeled 183 bird, 3 mammal, and 41 amphibian species' vocalizations and calculated 5 acoustic indices over 2 weeks worth of recordings.
A downside of acoustic indices is that no single acoustic index has yet been developed that universally denotes biodiversity (perhaps unsurprising given how different ecosystems exist on our planet). Existing acoustic indices are flawed enough that some researchers argue they're not yet ready to serve as independent proxies of biodiversity. Alcocer et al., for example, recommend caution toward relying solely on acoustic indices, advising instead to pair them with other biodiversity metrics.
Following Alcocer et al.'s recommendation, Müller’s team deployed insect light traps, collecting insect DNA to better understand insect community composition and augment their sound data. They also used Convolutional Neural Networks (CNN) to identify 73 individual bird species within their forest recordings. Their CNN-derived bird community composition correlated well with the bird species that human experts identified in the recordings.
Müller et al. then compared the insect DNA data and organisms' sound data with the known stages of recovery of the different forest plots. They found that the combined acoustic indices and the CNN were a good way to show how far along in recovery a forest was (a mixture of acoustic indices was better correlated with biodiversity than any single acoustic index). Overall, Müller et al. found that ecoacoustics’ automated monitoring tools can indeed help track recovering forest’s biodiversity.
By quantifying the rate at which different species inch their way back into a recovering forest (i.e., biodiversity), soundscapes help us overcome weak proxies for biodiversity—like counting new tree growth. Perhaps it’s unsurprising that multimodal approaches (e.g., soundscapes + satellite imagery + insect traps) paint a fuller picture of what’s going on beneath the canopy than single modalities, but Müller et al.’s study gives us solid evidence that this is indeed the case.
Current Challenges in Ecoacoustics
For all its promise, though, applying ecoacoustics in forests is tough for several reasons. One barrier that Müller et al. point out is that soundscape approaches to monitoring forest recovery must be calibrated regionally (every forest is unique). To speed up the process of validating such models, Müller et al. recommend establishing a global sound bank of non-bird vocalizations (ample bird data already exists, but non-bird recordings are less plentiful).
The next difficulty is that forests are noisy. Ecoacousticians want noise, of course, but when numerous species are singing, chirping, and bellowing over one another, it's tough—even for highly trained humans—to accurately label data for deep learning models to train on, so that we can, for example, then use machines to zero in on specific bird or insect sounds within an entire soundscape recording.
Who's Speaking?
Labeling what organism is “speaking” and when they’re doing so is a challenge similar to "speaker diarization," the process of identifying and labeling different speakers within human speech recordings. We have algorithms that automatically perform accurate speaker diarization in human speech, thanks, in part, to the fairly standard conversational "turn taking" that humans typically stick to.
An entire forest's soundscape, however, doesn't exactly exhibit the turn-taking common in human conversation (though we might consider different species' tendencies to harness different segments of the frequency range, the "acoustic niche hypothesis," as a type of “turn-taking”). Unsupervised learning approaches like Guerrero et al.’s LAMBDA 3pi algorithm might assist us here, but even if only to verify unsupervised models’ performance, we still need accurately labeled sound recordings (and we probably won’t shed our dependence on supervised learning approaches anytime soon).
Variability and Mimicry
Complicating things further, some species enjoy a crazy vocal repertoire. Birds, in general, stand out in this regard, but some birds are on another level. The Brown Thrasher, for example, can belt out over 1,100 different songs.
And then there's mimicry to contend with. For example, how do we get machines to distinguish between when a Brown Thrasher mimics another bird like the Northern Flicker or White-eyed Vireo and when one of these birds sings its own tune?
Worse, bird songs and calls vary significantly between species and sometimes vary amongst the same species along geographic locations; the same bird might even sing the same song slightly differently on different occasions. Mix together numerous individuals of numerous bird species, all overlapping one another, and you can appreciate how complex a soundscape can get—just from birds alone (then mix in mammals, amphibians, and bugs).
Diversity and Quantity of Insects
Likely because backboned organisms tend to be easier to spot and listen to, the bulk of bioacoustics and ecoacoustics forest studies focus on birds, amphibians, and mammals. But if we really want to gauge a forest's health, we can't ignore the other type of organism that forests teem with—invertebrates.
Deep learning models can identify insect species by the sounds they make remarkably well. Some models, for example, can accurately detect and classify mosquitoes by their wingbeats.
This is wonderful because each insect species, for example, contributes to a forest's soundscape and biodiversity, but consider the sheer quantity of chirps, buzzes, trills, and other sounds that invertebrates produce.
To the human ear (and often to machines), these sounds often blend together into a cohesive tapestry of background noise. Given that the number of invertebrate species significantly outnumbers vertebrates, properly including insects in ecoacoustics analyses remains challenging.
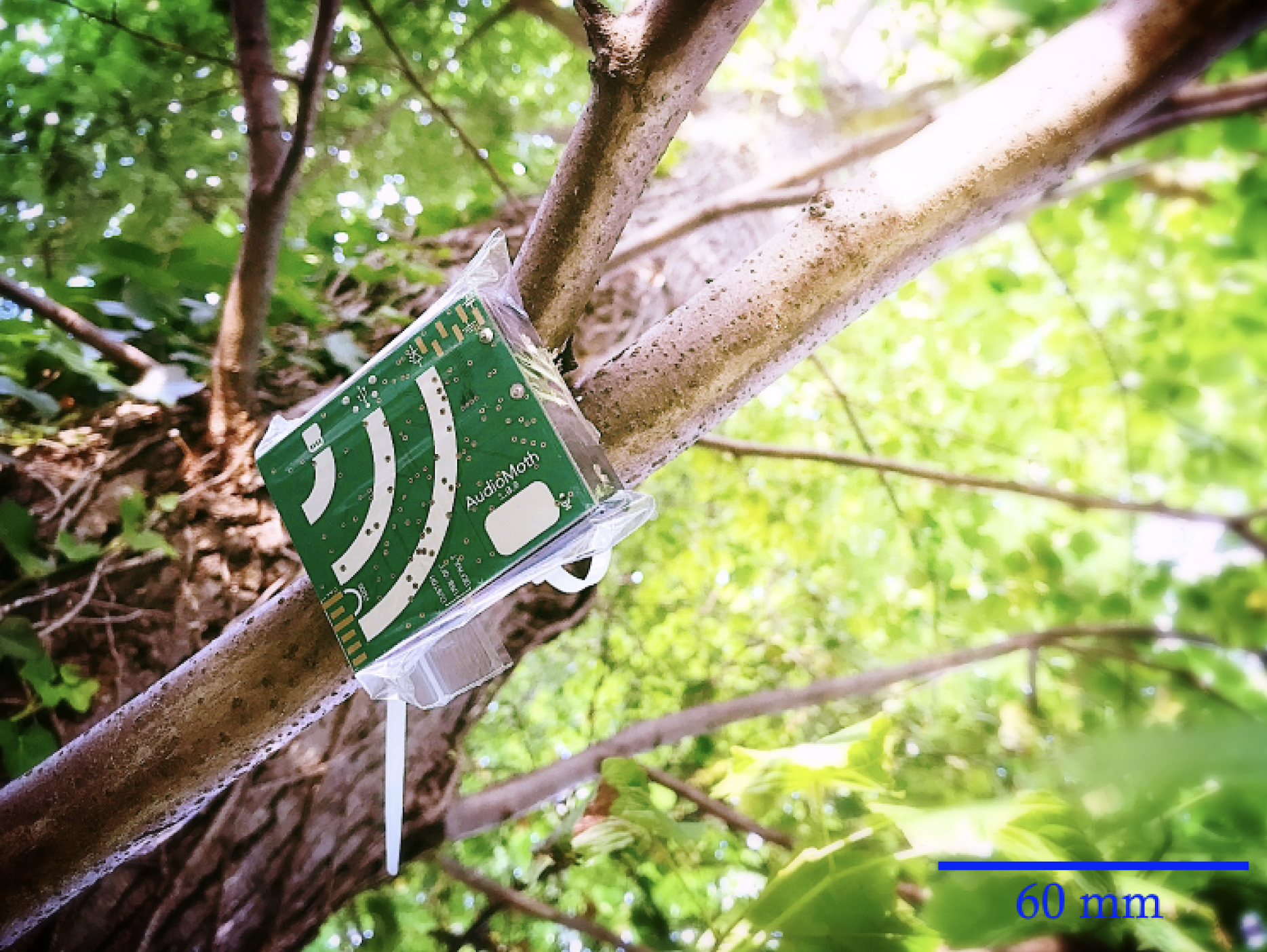
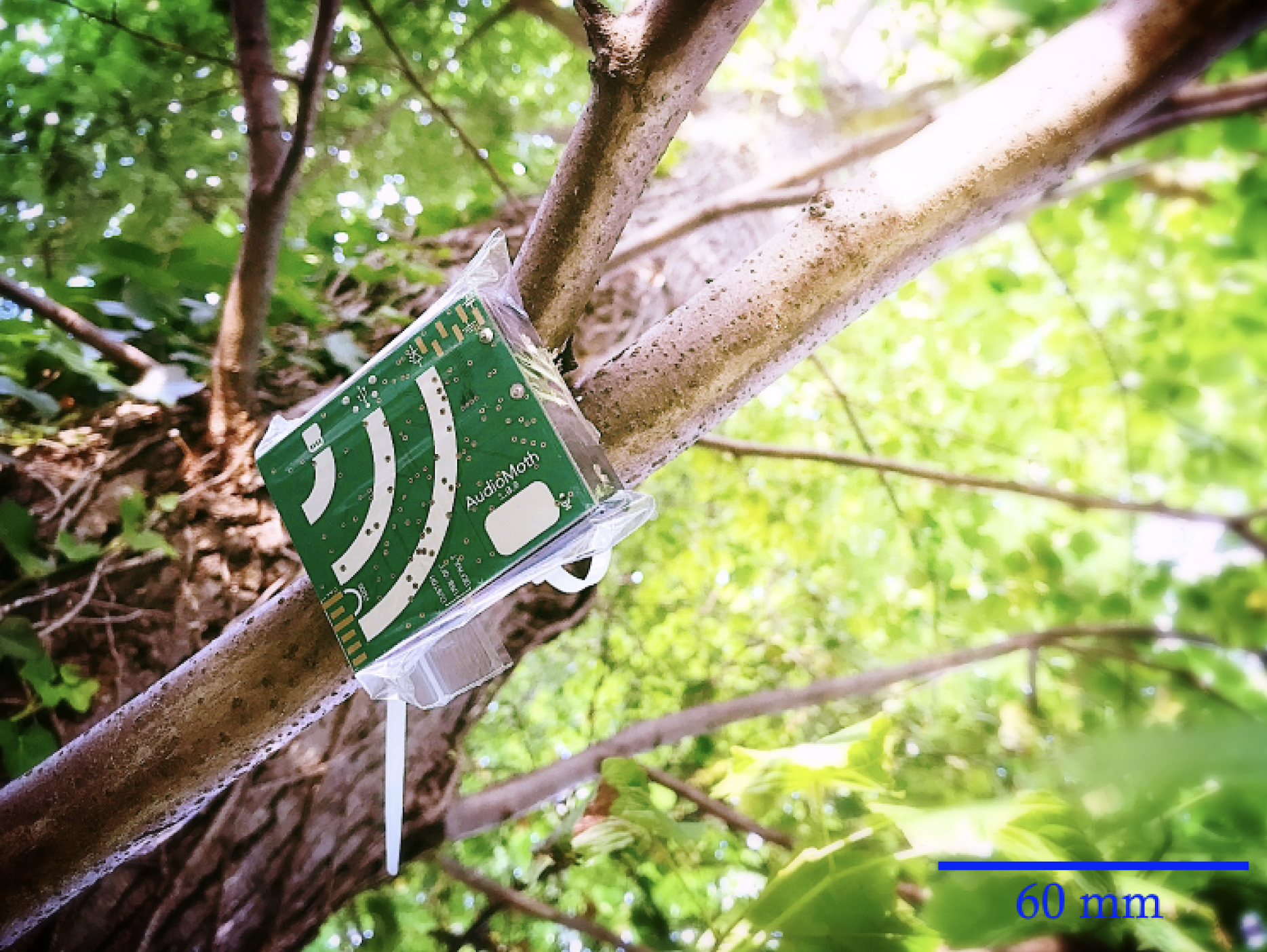
The Tales that Forests Tell
Forests are more than vast tracts of trees; they’re living, breathing communities or organisms, each with its own story to tell. And an epic tale emerges when we combine all these stories. Despite some significant challenges that bioacoustics and ecoacoustics need to overcome, hopefully you've gained a sense of the advantages they offer us for studying forests’ epic tales and their creatures’ individual stories. Both fields help us study visually cryptic yet vocal species' behaviors, reduce observer bias, can be scaled to large areas and run continuously, and help us quantify conservation efficacy. Even more powerful is combining numerous data modalities—like satellite or drone imagery, dung pile counts, DNA from bug traps, and more—with sound to create "multimodal" forest data. As we further unlock our forests’ acoustic signals, we’ll discover new chapters in the story of our planet's forests, gaining insights crucial for their preservation and recovery.
Note: If you like this content and would like to learn more, click here! If you want to see a completely comprehensive AI Glossary, click here.
Unlock language AI at scale with an API call.
Get conversational intelligence with transcription and understanding on the world's best speech AI platform.