Google vs. NVIDIA: Losing the AI Innovation Competition
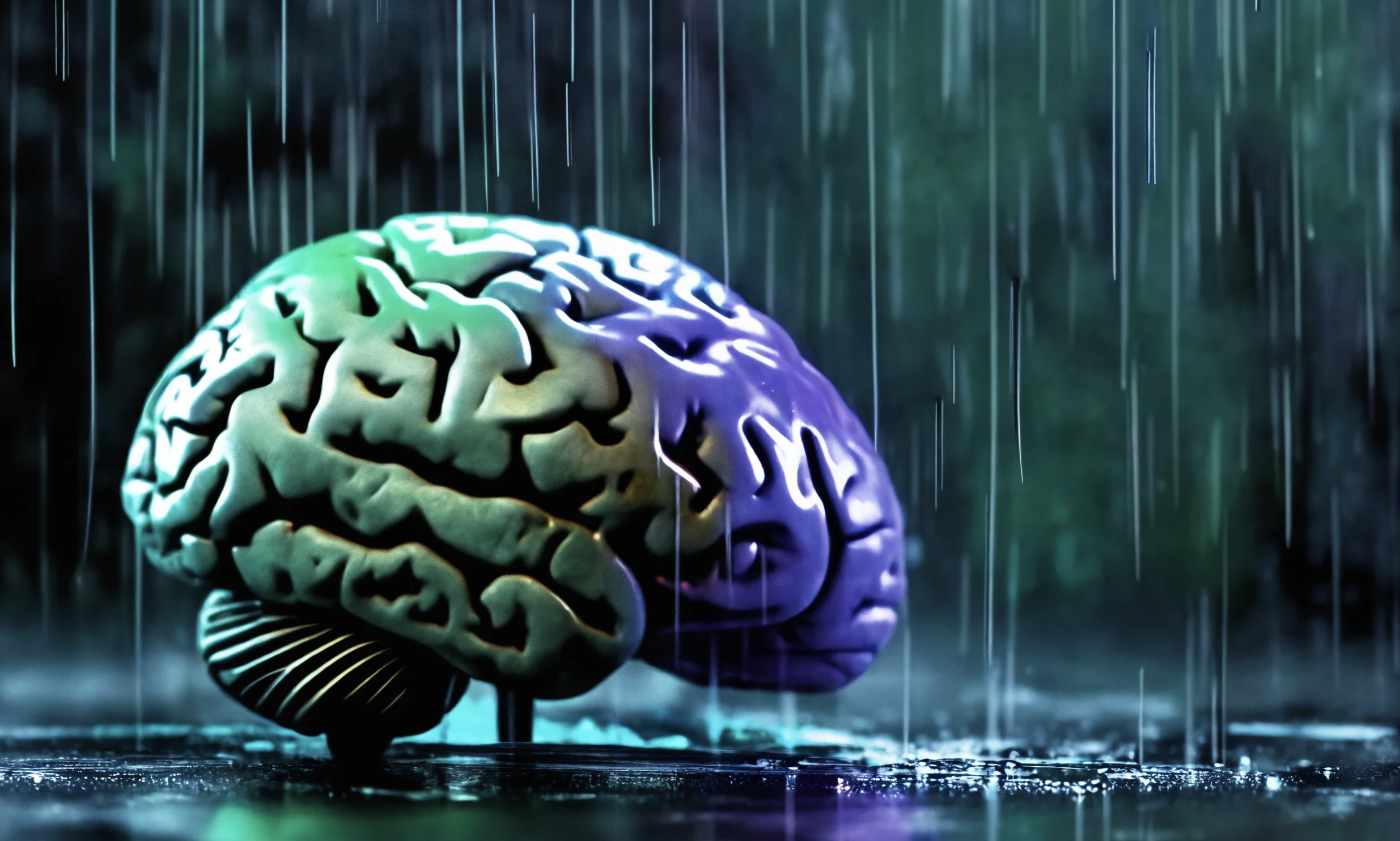
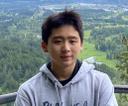
On June 11th, 2024, NVIDIA became the most valuable publicly traded company in the world. Though its shares have slipped down afterwards, without a doubt, NVIDIA has been one of the fastest growing companies in the entire world, being accelerated by the boom of AI research and development with the chipmaker being the foundation of nearly all AI-related computers.
NVIDIA’s market cap now sits at a little over $3 trillion dollars, nearly double what it started with in the beginning of 2024, less than half a year ago. As the competition in AI heats up, big tech companies, not just chipmaker competitors such as Intel or AMD, but Microsoft, Google, and Meta, are all attempting to catch the “AI hype”.
Notably, compared to other competitors along with its track record, Google seems to be falling behind, at least in terms of valuation. Of course, a company’s value doesn’t speak the entire story and unlike NVIDIA, Google has a much broader market that isn’t solely focused on AI.
Although Google, a company with an amazing track record for revolutionizing both AI research and hardware, may be falling behind amidst the rise of AI. The fact that LLM developments from recent years by Google trails behind nearly all other popular models from large tech companies doesn’t help the case either.
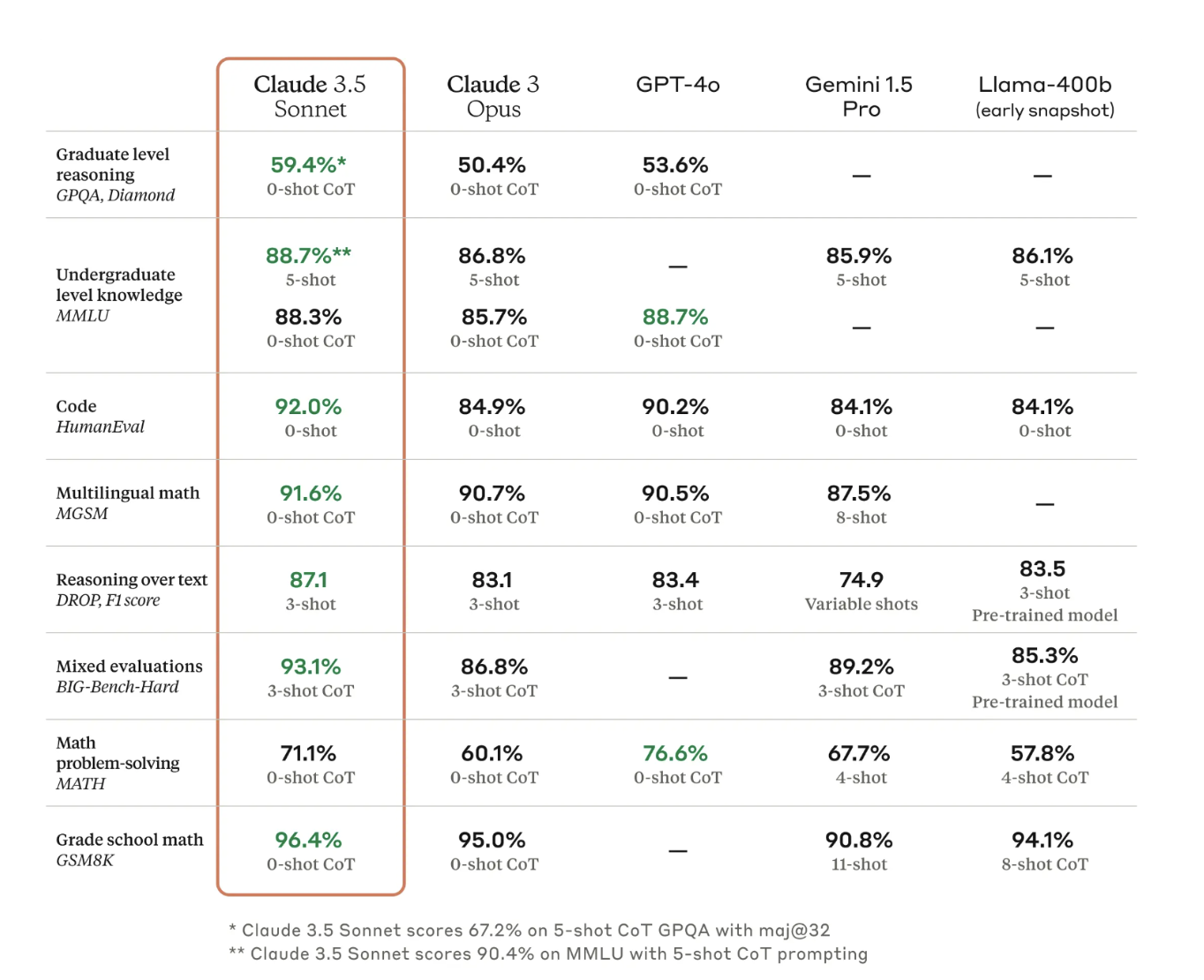
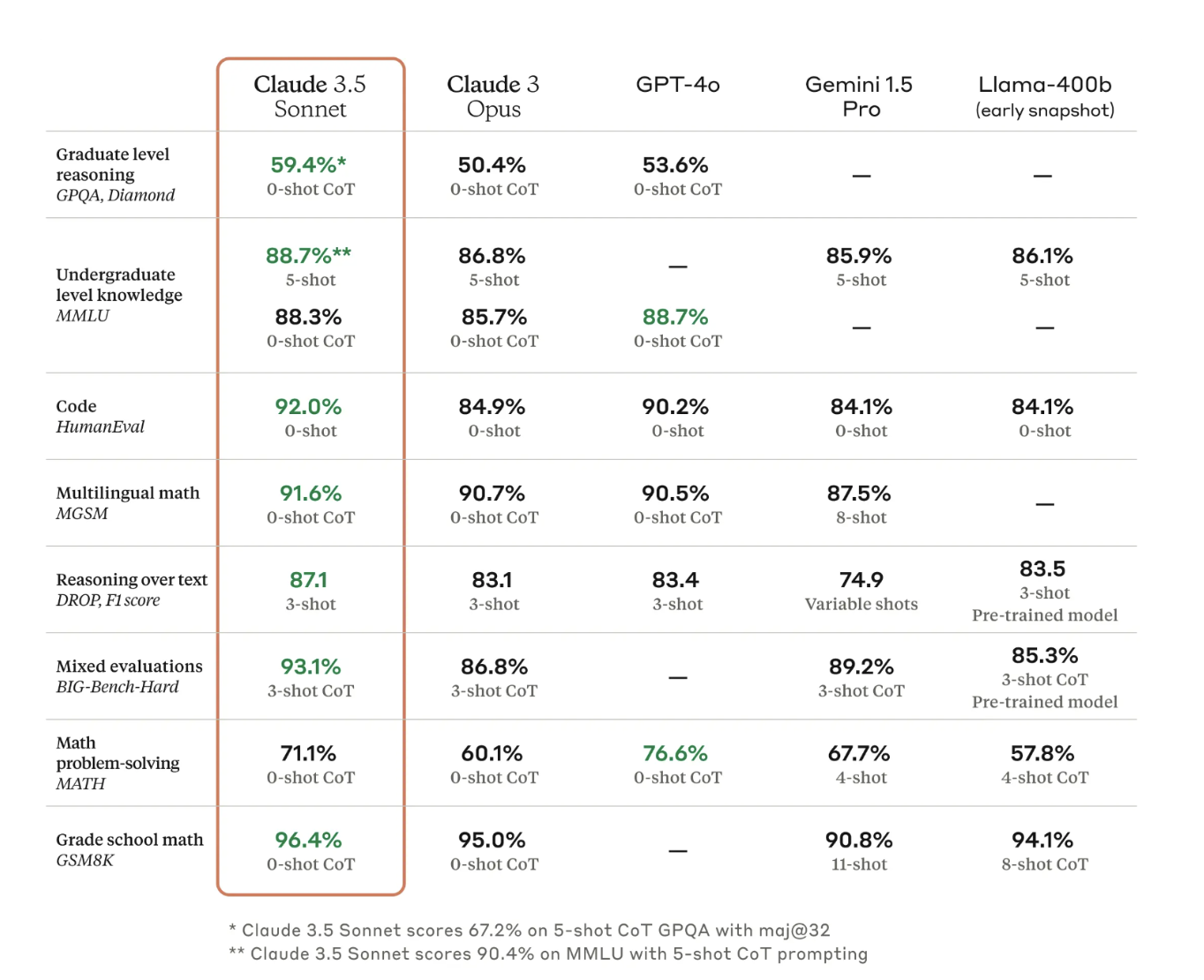
However, the AI race is not just about market valuation and benchmarks; they alone cannot tell the entire story. Google, with its expansive reach and diverse portfolio, has invested heavily in AI research and development through its Tensor Processing Unit (TPU) technology and projects led by Google Brain and DeepMind. Despite these efforts, questions remain about whether Google can keep pace with NVIDIA’s rapid advancements in AI hardware, particularly its GPUs that are setting new benchmarks in AI compute power.
While Google and NVIDIA are competitors, NVIDIA almost single-handedly supports the entire AI infrastructure, supplying GPUs to nearly every major tech company, including Google. Within this competition, there’s also a layer of partnership between the two giants.
NVIDIA’s strategic moves, including developing custom AI chips for major tech giants and launching powerful GPUs like the H100, have positioned it at the forefront of AI innovation.
Meanwhile, Google’s TPU technology, though impressive, has primarily powered its services and cloud infrastructure. The latest version, TPU v5p, has shown significant performance and efficiency improvements but faces stiff competition from NVIDIA’s H100 GPUs.
This article delves into the technological advancements, strategic initiatives, and competitive dynamics between NVIDIA and Google in the AI domain. By examining specific details of their AI hardware and research projects, we aim to provide a comprehensive analysis of whether Google is truly losing to NVIDIA from a research and technology perspective.
Nvidia’s Technological Edge
Nvidia’s rise in the AI landscape is fueled by its rapid advancements in GPU architecture, particularly with the Hopper architecture and the powerful H100 GPU. The H100 offers up to four times the performance of its predecessor, the A100, addressing the computational demands of AI training and inference with enhanced power, memory bandwidth, and efficiency
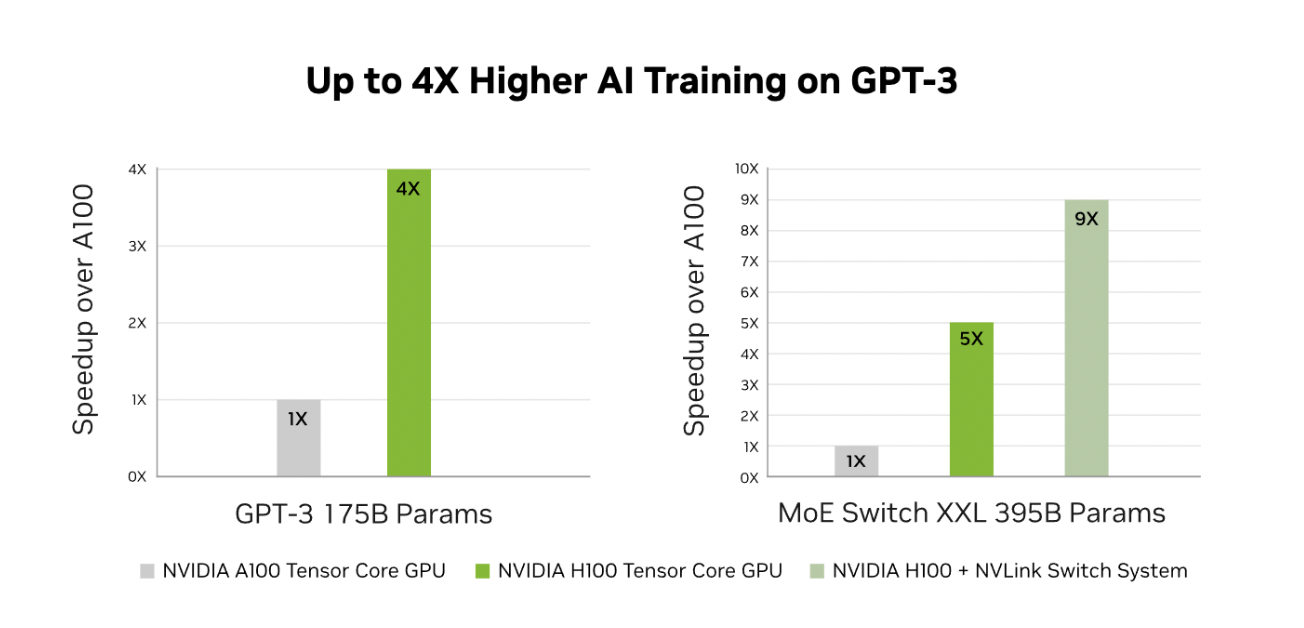
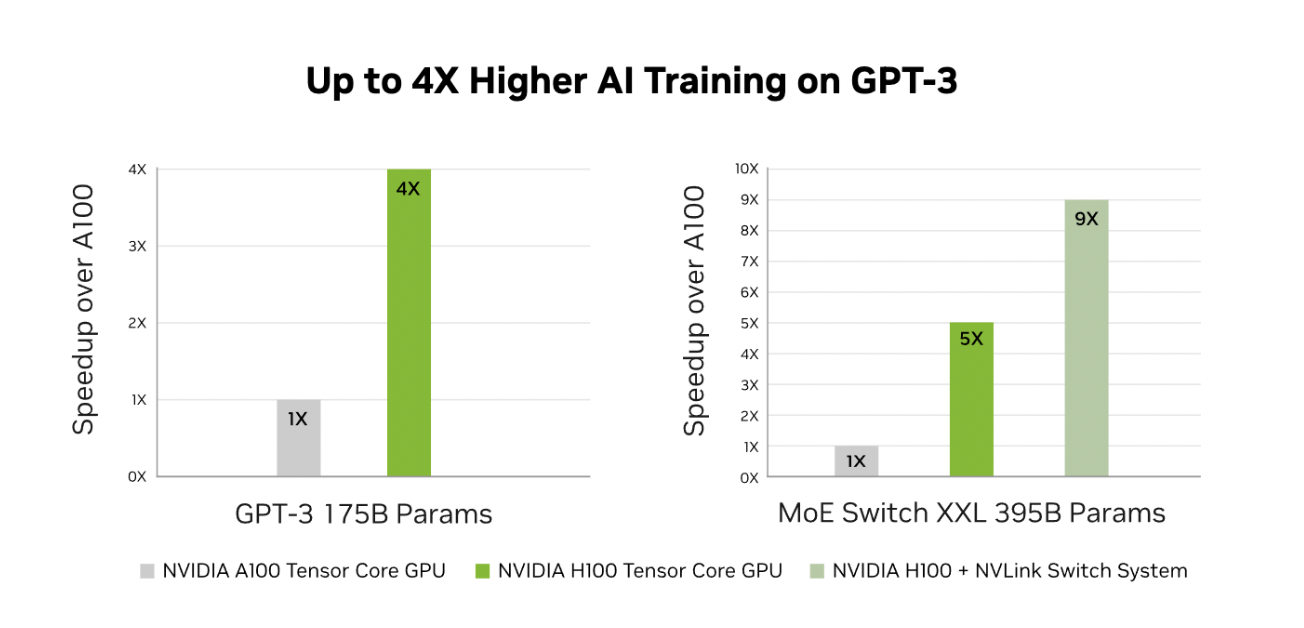
Accelerated Product Cycles
Up until 2021, NVIDIA has always followed a 2 year product cycle in its GPU production, if not longer. The most popular consumer grade GPU series, the GeForce RTX lineup, had a 4 year gap between the release of the 20 series and the 30 series. On the other hand, its data center GPUs specifically designed for heavy computation refreshes every 2 years, with the V100, A100, and the H100 being introduced every other year.
In a strategic move to stay ahead in the competitive AI and computing markets, Nvidia has accelerated its product development cycle from two years to one year with the CEO Jensen Huang announcing that “After Blackwell, there’s another chip. We’re on a one-year rhythm”, referring to the new architecture that will be the successor of Hopper.
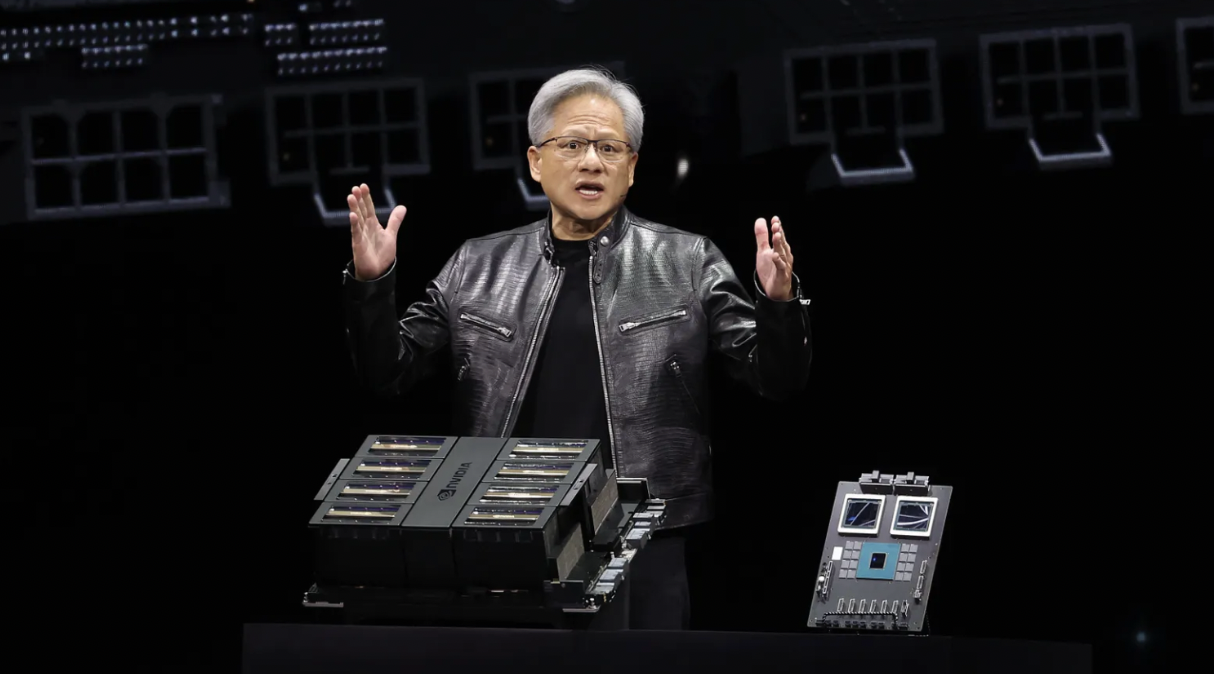
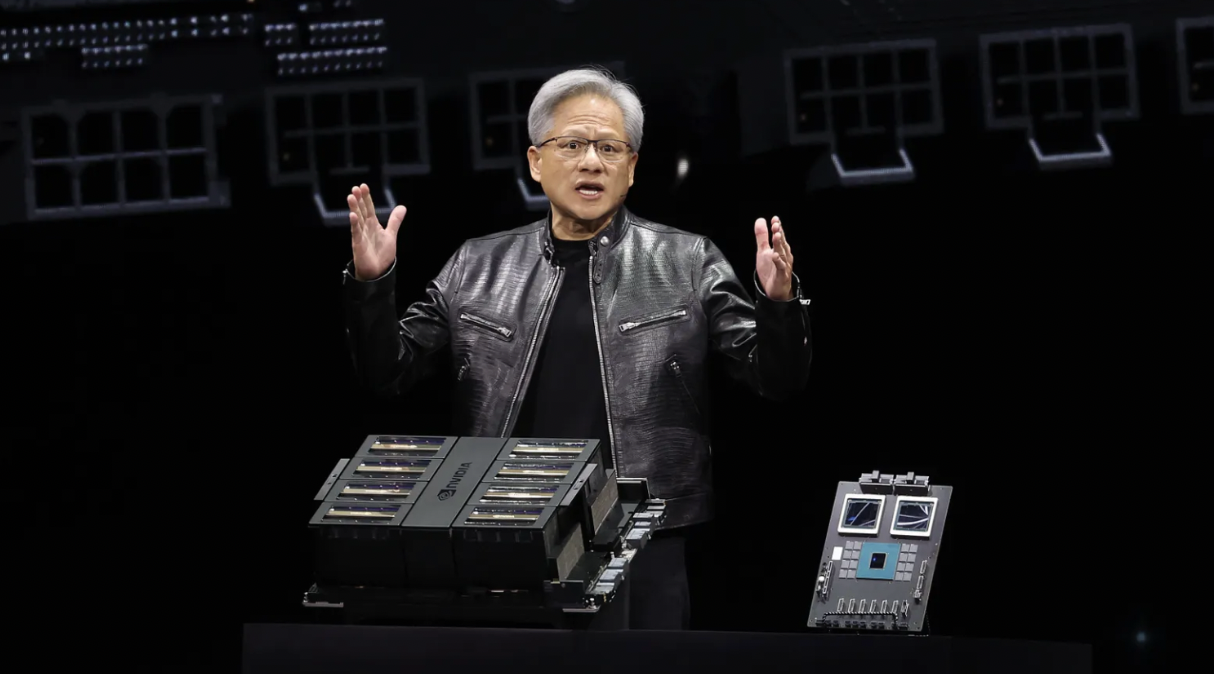
The Blackwell architecture is still on schedule to be released in 2024, sticking to the two-year cycle with the Hopper architecture in 2022. However, Huang revealed in his keynote at COMPUTEX 2024 the next-generation GPU architecture, dubbed “Rubin”, on track to be released in 2025, merely 3 months after the announcement of Blackwell.
On the consumer graphic cards side, the RTX 50-series is also expected to launch by the end of 2024, rumored to have much larger memory bandwidth and capacity with GDDR7 memory compared to the previous 40-series, which uses GDDR6.
Additionally, similar to the previous generation Grace CPU, NVIDIA will also be dipping its toes in the CPU space with an anticipated ARM-based CPU called “Vera”, combined with the Rubin GPU in the Vera Rubin accelerator.
It’s clear that NVIDIA is keeping pace with the increasing demand of AI chips, with these announcements only driving more anticipation and excitement.
NVIDIA’s Specialized Architectures
One of the key ingredients to success for NVIDIA is identifying the current and future trends in computing and capitalizing on those gains. The H100 GPU released in 2023 contains modules specifically designed for LLM inference, boasting a 12x performance increase for GPT-175B compared to A100s.
With the explosion of LLM development from universities, research labs, and tech companies worldwide, and the widespread use of the Transformer architecture, NVIDIA’s new Blackwell lineup features a second-generation Transformer engine, supporting double the compute and model sizes.
It’s clear that NVIDIA is focusing on the popularization of Transformer-based models. Considering the company has a 98% market share in data center GPUs, it’s likely that this push for specialized GPUs will create a positive feedback loop: the Blackwell and future GPU architectures will encourage increased usage of Transformer-based models, which in turn will drive NVIDIA to release even better, faster specialized chips.
In addition to the standard B100 and 200 that will be released as part of the Blackwell architecture, NVIDIA also plans to produce the GB200 NVL72, a supercluster of 72 Blackwell GPUs capable of running trillion parameter LLMs in real time. There’s no doubt that NVIDIA is ahead of the game compared to any chipmaker, not just Google.
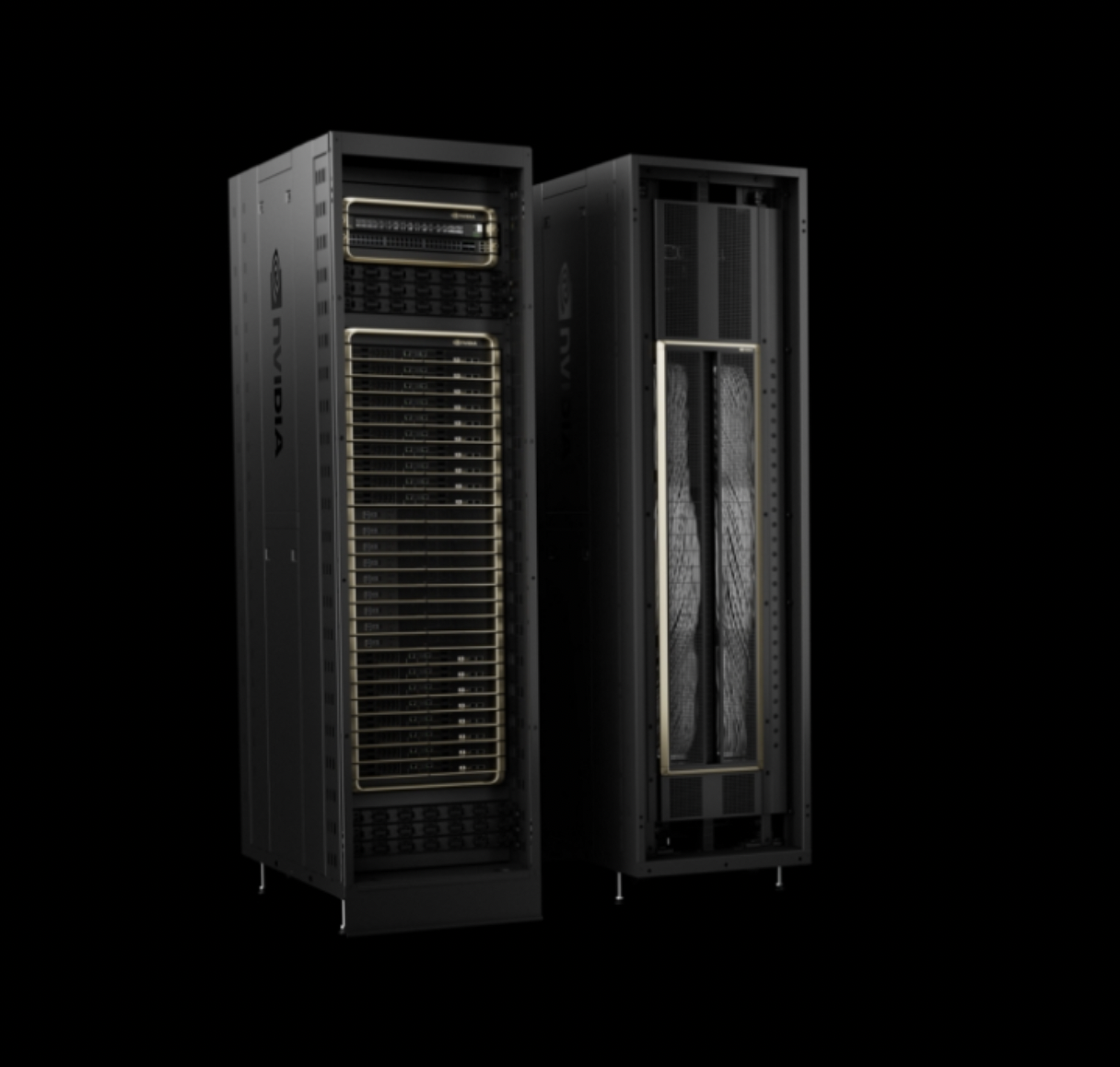
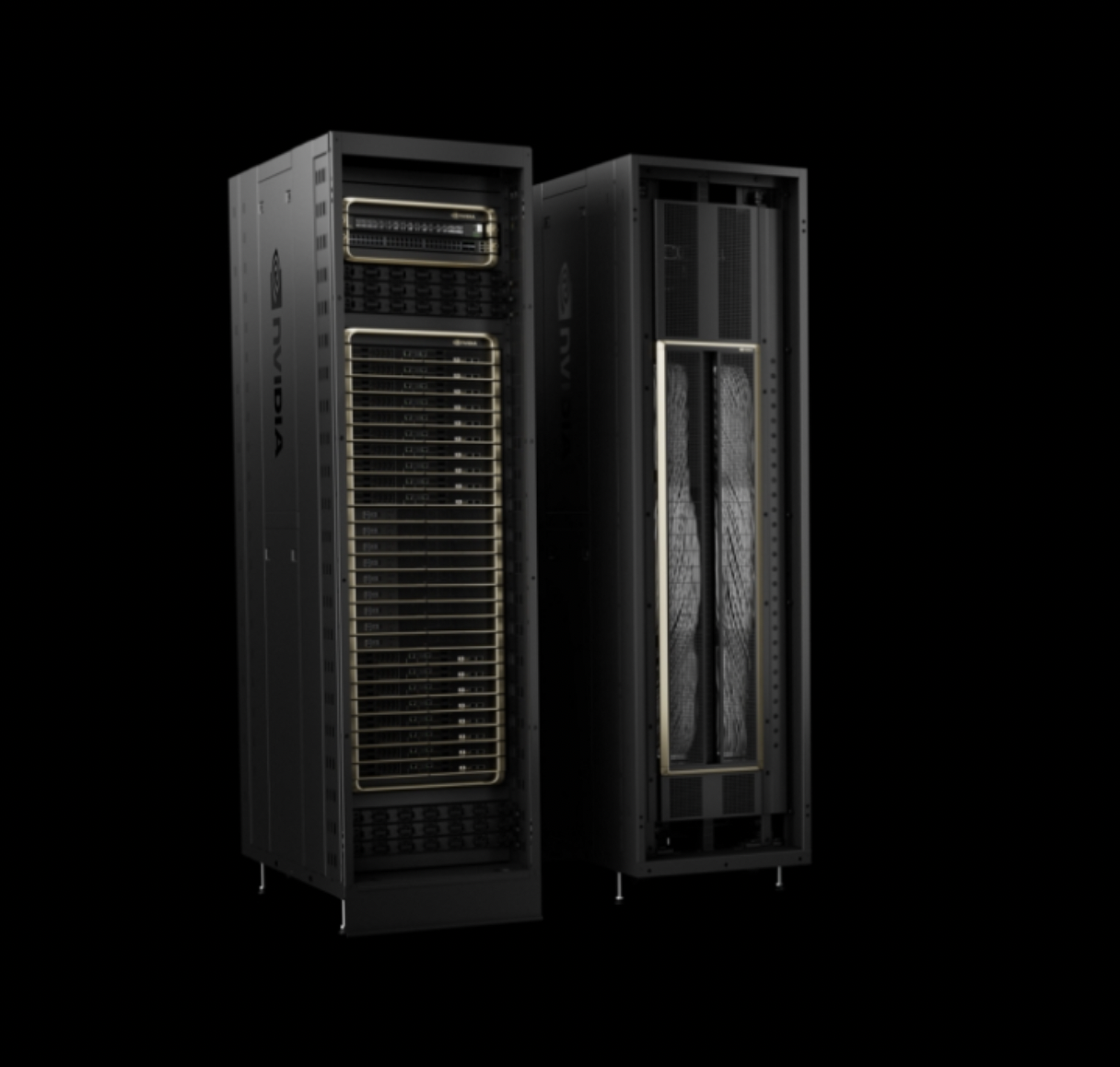
NVIDIA’s Software Ecosystem
While Nvidia is renowned for its hardware, its software ecosystem plays an equally vital role in its dominance in the AI market. The CUDA-X suite of libraries and tools enhances GPU-accelerated applications across various sectors like deep learning and high-performance computing. NVIDIA AI Enterprise delivers enterprise-grade AI tools and frameworks optimized for data centers and cloud environments, ensuring scalable and secure deployment. The NGC Catalog serves as a hub for GPU-optimized software, offering pre-trained models, SDKs, and application frameworks, catering to AI, HPC, and visualization needs.
Though these softwares may not be used by day-to-day consumers, technologies such as CUDA remain as the foundation for not only AI but accelerated computing, and more likely than not will still be in use even if NVIDIA falls short on the hardware side in the future.
Google’s Research Advancements
On the other side of the story, unlike NVIDIA that started as a graphics card company, Google’s origins remain on the software side with its search engine and cloud services.
But this is not to say that Google is nothing less of a pioneer in the AI industry. Google DeepMind combines two of the brightest AI research labs: Google Brain and DeepMind. DeepMind was co-founded by Demis Hassabis, Shane Legg, and Mustafa Suleyman in 2010 with its headquarters located in England.
Prior to the acquisition by Google in 2014, DeepMind found success in Deep Reinforcement Learning, the subfield of machine learning that combines Reinforcement Learning and Deep Learning with breakthroughs such as the Deep Q Network.
Throughout the decade, DeepMind was behind some of the most famous AI breakthroughs prior to the explosion of LLM. This includes the undefeated Go agent AlphaGo and many of its successors such as AlphaZero, MuZero and the later AlphaStar, an AI agent capable of defeating top professional players in the video game StarCraft II.
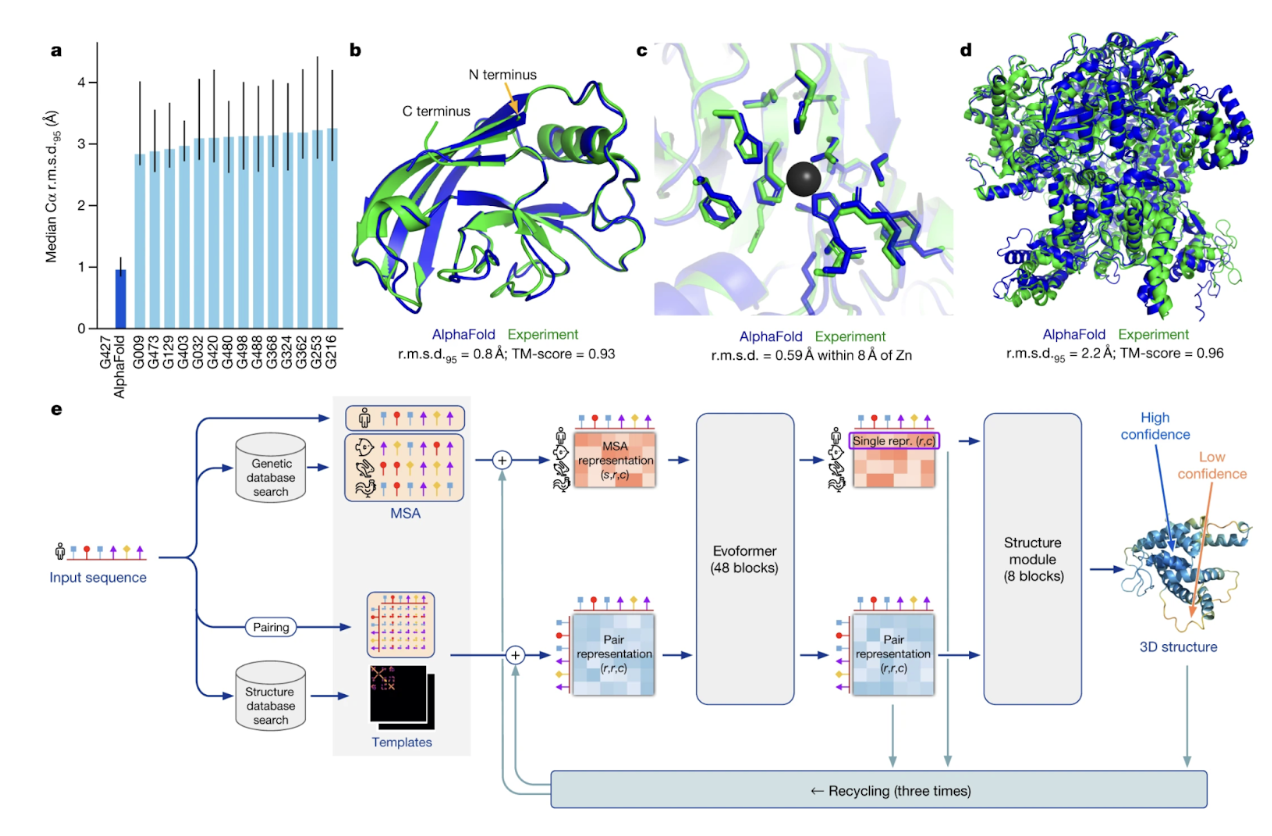
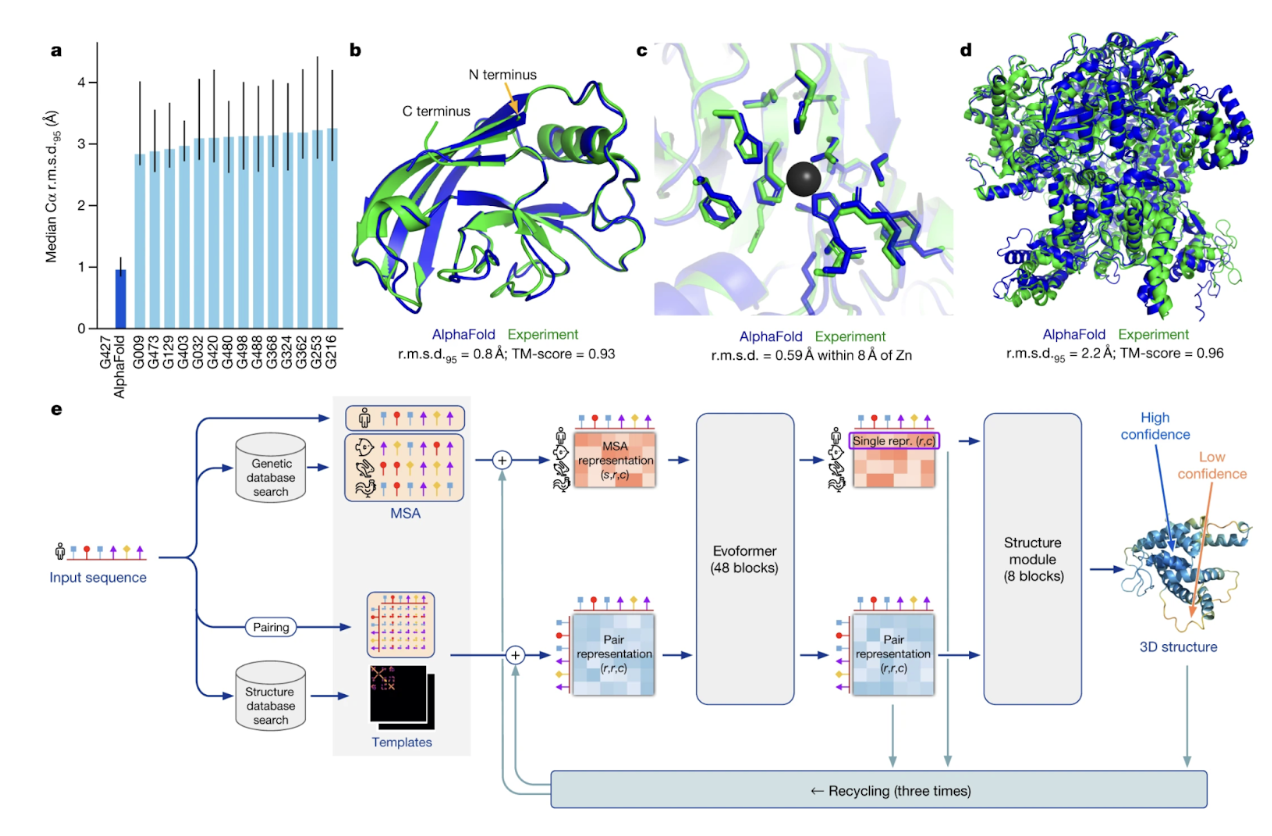
DeepMind was also the team behind AlphaFold, the world-renowned AI system capable of predicting 3D protein structures with unprecedented accuracy. The model has not only opened up new ideas and techniques in terms of AI research but significantly accelerated research in nearly all fields of Biology.
Google Brain began in 2010 with the goal of improving Google products and services through AI but has evolved to contribute towards the field of machine learning in general on both research and software technologies.
Years later, many machine learning systems run on the infrastructure built by Google Brain, including the open-source frameworks JAX and Tensorflow with the latter being one of the most widely used frameworks for general-purpose machine learning.
Finally, and perhaps the most well-known research development from Google Brain: the Transformer architecture. Its first debut in 2017 was merely targeted towards sequence-to-sequence modeling, marking a massive leap compared to traditional methods such as RNN and LSTM.
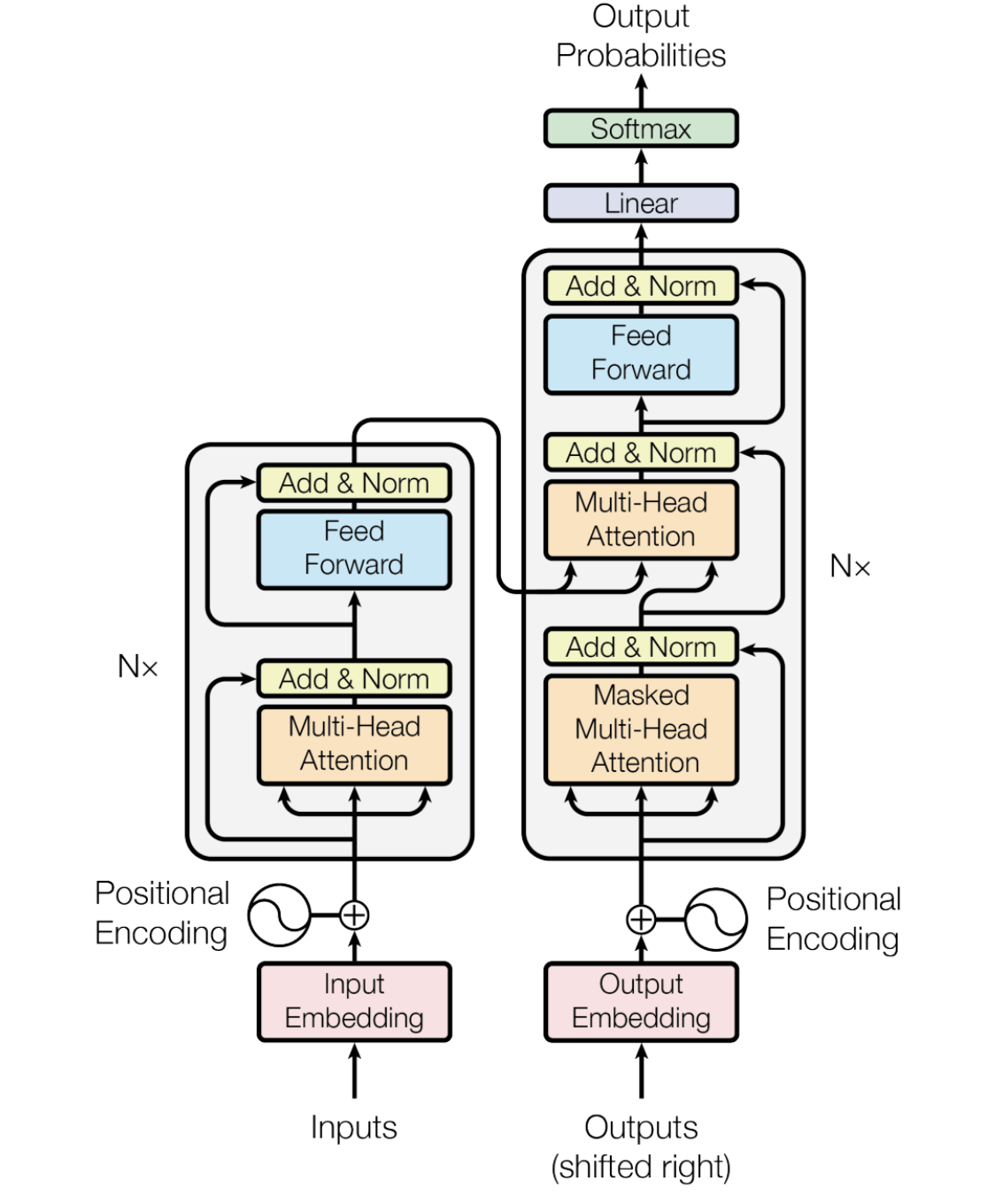
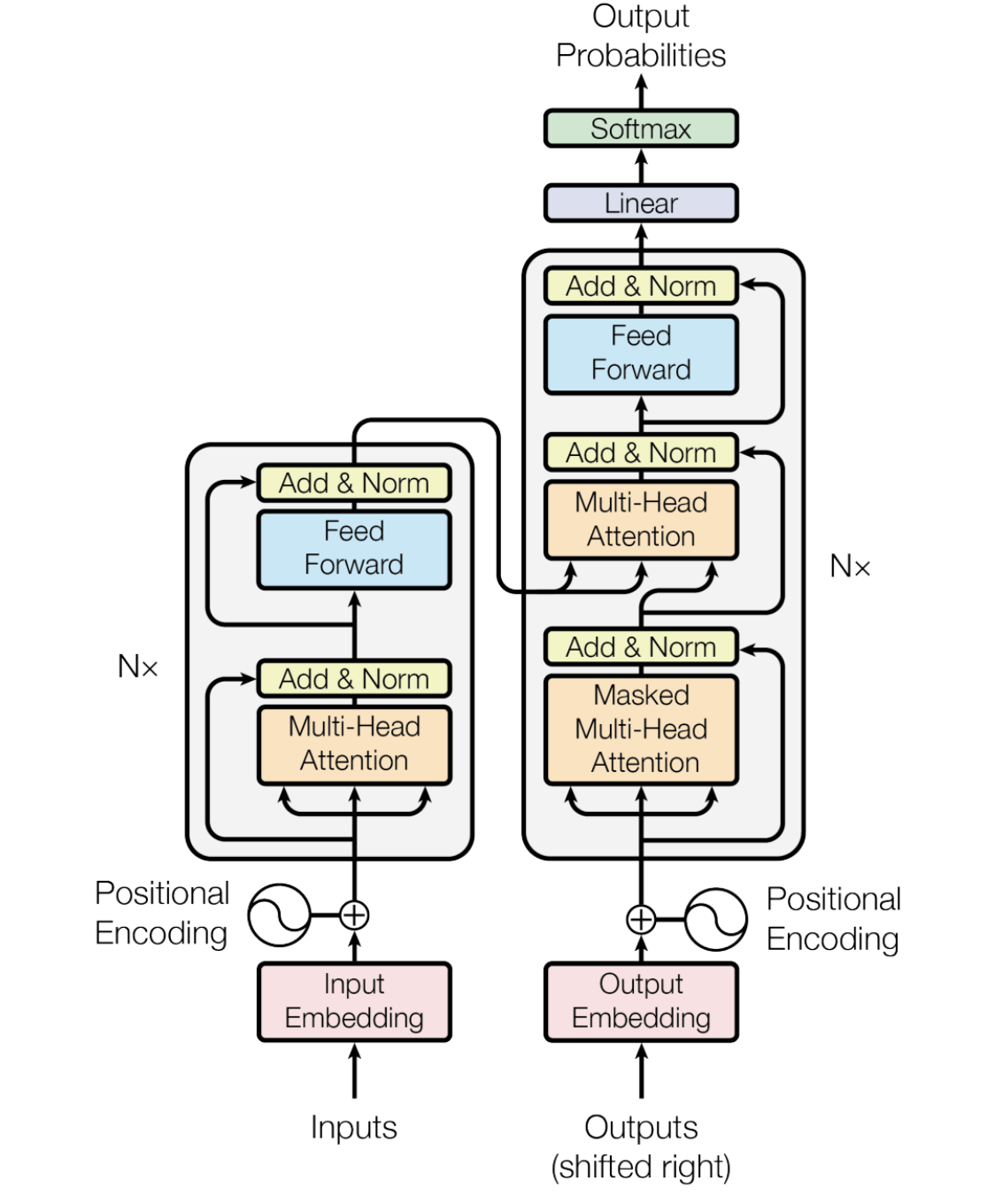
However, as time went by, researchers discovered that the Transformer architecture, along with its novel self-attention mechanism, is an excellent choice for nearly all forms of data modalities and tasks as demonstrated by models like the Vision Transformer(Also developed by Google!).
Today, Large Language Models (LLMs) have pushed the Transformer architecture to its full potential, dominating the field of machine learning as a whole. In a way, without the advancements from Google Brain, none of the financial and hardware successes of NVIDIA would have been possible. There wouldn’t be a dedicated module for executing Large Language Models if the Transformer architecture was never invented.
Google’s Hardware Developments
Not to be outdone by NVIDIA, Google can also be considered a leader in AI-specific hardware development. In fact, Google’s specialized AI chips, the Tensor Processing Unit (TPU), are among the oldest dedicated hardware for AI. Introduced in 2015, TPUs were initially developed to accelerate Google’s own machine learning models. By 2018, they were made available to the public through Google Cloud, marking a significant step in democratizing access to high-performance AI hardware.
The evolution of TPUs highlights Google’s continuous innovation in AI hardware. The TPU v4, for instance, was estimated to be between 1.2 and 1.7 times faster than Nvidia’s A100 GPU, providing significant performance advantages for AI workloads. The latest TPU v5p, introduced alongside Google’s Gemini AI models, is even more powerful. With 8,960 chips per pod, a throughput of 4,800 Gbps, and 95GB of high-bandwidth memory, the TPU v5p is designed to handle the most demanding AI training and inference tasks. This new TPU is reportedly 2.8 times faster at training large language models compared to its predecessor, the TPU v4, and offers a 2.1-times improvement in value-for-money.
Although Google’s TPUs aren’t as versatile as NVIDIA’s graphic cards, they definitely offer better bang for the buck.
Despite these advancements, Google’s TPUs have faced challenges. The TPU v6, while offering impressive improvements in energy efficiency and performance, has been criticized for its high cost and limited availability.
Who Has the Upper Hand?
From a bare-bones numbers comparison, NVIDIA most definitely tops Google in terms of financial metrics, with its market cap comfortably sitting above Google’s by about three-quarters of a trillion as of June 2024. Some analysts even believe that the chip maker's stock will top $1000 post-split.
On the research side, there is no question that Google dominates NVIDIA, publishing hundreds of papers each year. Some of these, such as “Attention is All You Need,” have cemented themselves in AI history as foundational works.
However, determining whether Google is losing to NVIDIA is much more complex and nuanced. NVIDIA and Google are fundamentally different companies, engaging in different parts of the technology sector, and only certain portions of their operations overlap competitively.
NVIDIA is currently benefiting from the increasing demand for graphics cards for both personal use and data centers. However, its laser-focused strategy and expanding specialized chip production for specific machine learning models come with risks.
The dotcom bubble burst still haunts the technology sector as markets skyrocket on the excitement and anticipation of Artificial Intelligence. Some speculate that, much like in the 2000s, the “AI bubble” may also burst due to overvalued anticipation and underwhelming results.
An economic collapse in the technology sector wouldn’t serve well for either NVIDIA or Google, but in such a case, NVIDIA may suffer more damage than Google. Beyond its AI-focused research and products, Google has a broader influence with its search engine, cloud services, and many other technologies that are core components of today’s internet and unrelated to Artificial Intelligence. In other words, NVIDIA’s short-term success may not translate to long-term longevity.
In terms of AI development, Google and NVIDIA share a somewhat symbiotic relationship: without NVIDIA’s hardware advancements, Google’s research wouldn’t have popularized the machine learning field, while without research, NVIDIA’s hardware would primarily serve the gaming industry. However, Google does have an upper hand as they produce hardware on par with NVIDIA’s data center GPUs.
At the end of the day, there’s no definitive answer that deems either company a “loser” in the AI revolution. While some may speculate that Google is falling behind for a company that initiated many industry practices and technologies, NVIDIA’s short-term success isn’t everything either.
Unlock language AI at scale with an API call.
Get conversational intelligence with transcription and understanding on the world's best speech AI platform.