Neural Networks, Hieroglyphs, and Speech AI: A History of Transcription
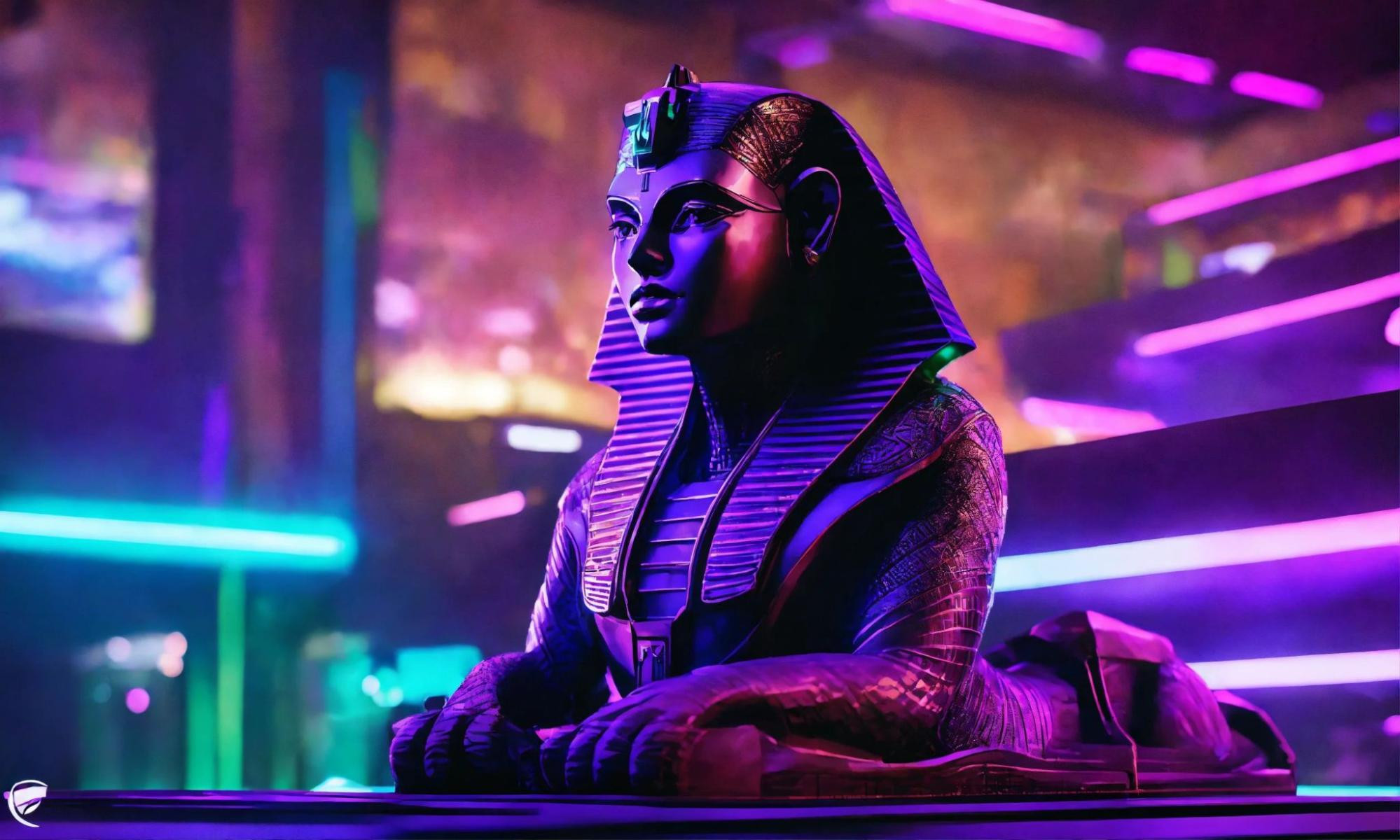
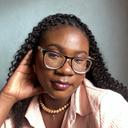
Speech transcription has always existed in one form or another for most of human existence, from hieroglyphics in ancient Egypt to cuneiform writing in ancient Near East. These days, we have speech recognition apps that can hear, understand, and transcribe speech available to millions of people. This is powered by speech recognition, or speech-to-text, technology, a field dating back to the 1950s when the first speech recognition tool was developed. Here is a more comprehensive history of speech transcription.
Early methods of speech transcription
Different types of writing have been used as a form of documentation since as early as 3400 BC. In Ancient Egypt, hieroglyphics were used to document words, events, and customs preserving them for thousands of years. Elsewhere around the world in ancient Greece, poems like Homer’s Iliad and Odyssey were transcribed from their original oral composition into text in the late 8th or 7th century BC. Like many early transcriptions, both hieroglyphics and Homeric Greek, the form of Greek used in writing at that time, have long since fallen out of use and been replaced by modern forms of writing. In the 4th century BC, the first transcription system was established by the Library of Alexandria in order to collect all the books in the world.
Manual speech transcription
In the early years, transcriptions were handwritten by dedicated transcriptionists and scribes who would spend hours painstakingly transcribing information for future use. Transcriptions were in demand by different professionals who needed to keep records, take notes, and document events. This included legal practitioners, businessmen, and physicians, until one of the latter created the modern English shorthand. The introduction of shorthand in the 17th century was considered revolutionary since it shortened the time and energy needed to transcribe and take notes. Secretaries, who mostly consisted of women, began to take dedicated shorthand classes as part of their secretarial training.
When the typewriter was invented in 1868, it quickly became indispensable in offices as a more efficient solution to handwritten transcription. The increasing number of women entering the workforce as secretaries and typists meant that early typewriters were marketed towards women and intentionally modeled to look similar to sewing machines. According to a 1900s census, 94% of stenographers and typists were women. Stenographer keyboards invented in the 19th century also helped to make transcribing easier for stenographers and are still in use in courtrooms today. By the 1990s, dictation machines like the Dictaphone had been invented and were being used to record speech for eventual transcription.
The phonograph, which was invented in 1877 by Thomas Edison, used sound vibration waveforms to record and reproduce sound, the first step in speech transcription. Although the phonograph was invented as yet another tool to assist office transcriptionists, it would go on to be one of the first machine transcription tools.
Introduction of speech recognition tools
In 1953, three scientists at Bell Labs, Stephen Balashek, R Biddulph, and K.H. Davis built a system, the Automatic Digit Recognizer or AUDREY, that could recognize the sound of numbers (0 - 9) when spoken by Davis. This was a huge accomplishment at the time even though it could only accurately recognize Davis’ voice and so was not useful commercially. Most research into speech recognition at the time was focused on numbers which worked to serve telephone systems. Meanwhile, IBM was working on its Shoebox machine which could understand 16 English words. The Shoebox machine was showcased in 1962 at the 1962 World Fair. During this time, labs in the US and other parts of the world were also carrying out research into speech recognition. MIT Lincoln lab built a 10-vowel recognizer and researchers at Kyoto University in Japan were able to build a phoneme recognizer, the first use of a speech segmenter for speech recognition and analysis.
In the late 1960s, Itakura Fumitada and Shuto Saito individually created the basic concept of Linear Predictive Coding, setting the foundation for pattern recognition and speech recognition technologies still used today. In 1969, John Pierce, an influential engineer at Bell labs wrote an open letter criticizing speech recognition research. This caused a lull in Bell labs research as funding for speech recognition dried up.
In the early 70s, Tom Martin, a scientist, founded the very first speech recognition company called Threshold Technology and developed VIP-100 which was used by manufacturing firms for quality control. The success of the VIP-100 system influenced the US Department of Defense’s research arm, DARPA, to fund a Speech Understanding Research program for five years. During this time, Carnegie Mellon University successfully built Harpy, a system that was able to recognize speech with a vocabulary of 1011 words. Apart from Harpy, other systems built during the SUR program were Carnegie Mellon University’s Hearsay and BBN’s Hear What I Say.
By the 1980s, there was a shift towards the use of Hidden Markov Models (HMMs) for speech recognition. HMM is a more statistical modeling framework and was originally developed in the 1960s at the Institute for Defense Analysis in Princeton. The introduction of HMM to speech recognition has been useful in the accuracy of speaker independence and large-vocabulary speech recognition tasks. This research led to the development of practical speech recognition tools in the 90s including Dragon Dictate, the first consumer speech recognition product.
Machine Translation in the 2000s
Speech-to-text research in the 2000s was mostly sponsored by the US Department of State’s DARPA. Under DARPA sponsorship, two programs: Effective Affordable Reusable Speech-to-Text (EARS) and Global Autonomous Language Exploitation (GALE). The EARS program was made up of four teams and was able to collect over 260 hours of conversation from more than 500 people while the GALE program was focused on extracting information from Mandarin and Arabic news sources and translating it to English. Other government agencies also began to make use of speech recognition technology for keyword spotting and other government uses.
One of the major turning points for speech recognition was the introduction of Google’s voice service, GOOG-411 in 2007. With GOOG-411, a user could call in and get basic information free of charge. The service which allowed Google to build a large amount of voice data is the foundation of Google’s current speech system including Google Voice Search.
By the late 2000s, the tide was shifting away from the use of HMMs and towards deep learning methods. In 2009, Geofrey Hinton, a professor at the University of Toronto, and Li Deng, a researcher at Microsoft, proposed the use of deep feedforward networks for acoustic modelling in speech recognition. Since then, other forms of deep learning techniques have been used including the use of transformers, a type of deep learning network.
2010s till now
Since the introduction of Dragon Dictate in 1990, speech recognition systems had to be trained to recognize the speaker’s voice (Dragon Dictate itself had to be trained for 45 minutes), a chore that took time and energy. However, by the early 2010s most systems were speaker independent meaning that they could respond to words regardless of who is speaking. This was an important development for speech recognition research and a major progress for industrial speech recognition systems.
End-to-end automatic speech recognition also rose to popularity in the early 2010s and by 2014, there was considerable amount of research done by researchers from Google Deepmind and the University of Toronto. End-to-end automatic speech recognition is a system that directly maps a sequence of input acoustic features into a sequence of grapheme or words simplifying the process of traditional speech recognition. Attention based models are currently the most popular type of end-to-end ASR since it can learn all the parts of a speech recognition model directly.
In 2018, Google Deepmind introduced their Deep Audio-Visual Speech Recognition research which would be able to recognize phrases and sentences spoken by a talking face even without audio. This research focused on lip reading and launched the release of an audio-visual speech recognition dataset. The year after in 2019, Amazon launched their transcription service for medical practitioners, Amazon Transcribe Medical.
In 2020, Meta launched wav2letter@anywhere, an open source framework that can be used to perform online speech recognition like live video captioning and on device transcriptions. More recently, they introduced their Massively Multilingual Speech (MMS) project, a dataset with labeled data for over 1000 languages and unlabelled data for over 4000 languages.
The future of machine transcription
Speech transcription has come a long way from typewriters and shorthand used by typists and secretaries in the early years. Today, machine transcription has created a faster and more efficient way to transcribe making it so that transcribing is no longer gendered. However there is still work to be done especially with the transcription of other languages aside from English. Currently, labeled data is available for only about 14% of the 7000 languages spoken across the globe. This means that machine translation is still not possible for the majority of the world’s languages.
Although speech recognition word error rates on popular benchmarks were shown to have surpassed human word error rates in 2017, humans still generally understand speech better than machines. There is an opportunity to learn from human speech recognition especially in the aspect of context and nuance research.
Note: If you like this content and would like to learn more, click here! If you want to see a completely comprehensive AI Glossary, click here.
Unlock language AI at scale with an API call.
Get conversational intelligence with transcription and understanding on the world's best speech AI platform.