How the AI Business World Actually Works: A Startup’s War
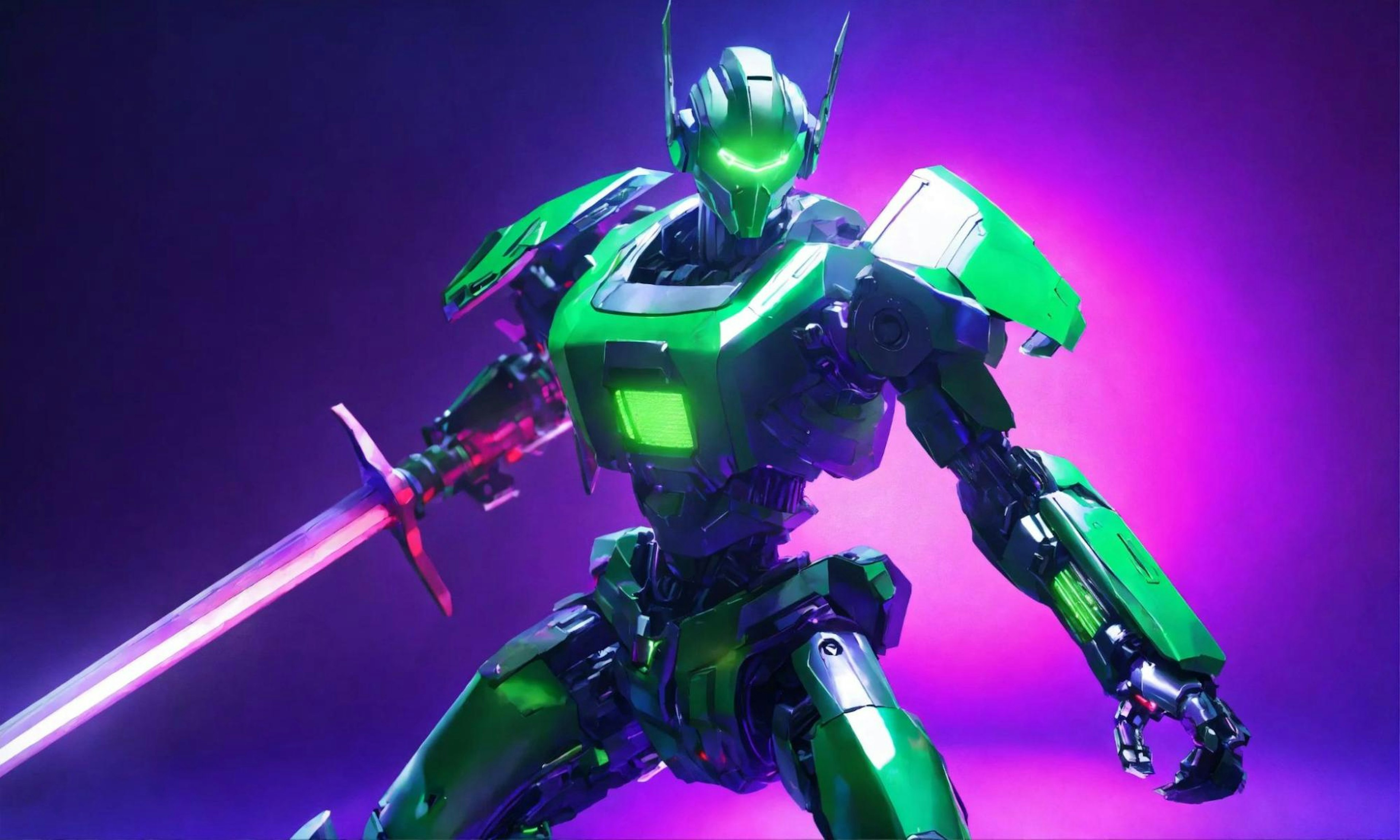
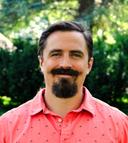
In May 2023, a leaked memo, penned by an anonymous Google engineer, spread around the internet; its author opined that neither Google nor OpenAI enjoyed a competitive moat relative to open-source Language Models (LMs). The memo’s key conclusion begs an important question: if Google—a gargantuan company with many of its products infused with Artificial Intelligence (AI)—is fretting about open LMs scaling over their rampart walls, shouldn’t AI startups be even more sketched out? Aren’t their moats paper thin compared to Google’s?
About a month after it leaked, Google’s DeepMind CEO, Demis Hassabis, ceded that the widely-discussed memo likely originated from Google while dismissing its main conclusions. Hassabis believes that Google still enjoys many competitive advantages that the memo overlooked.
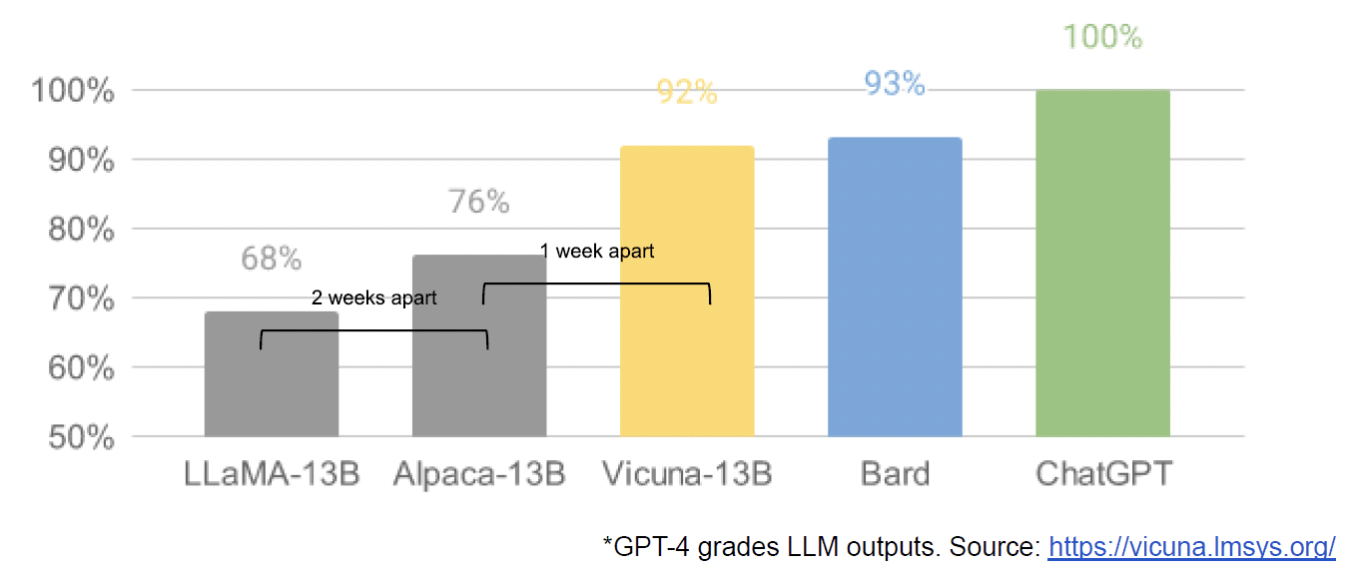
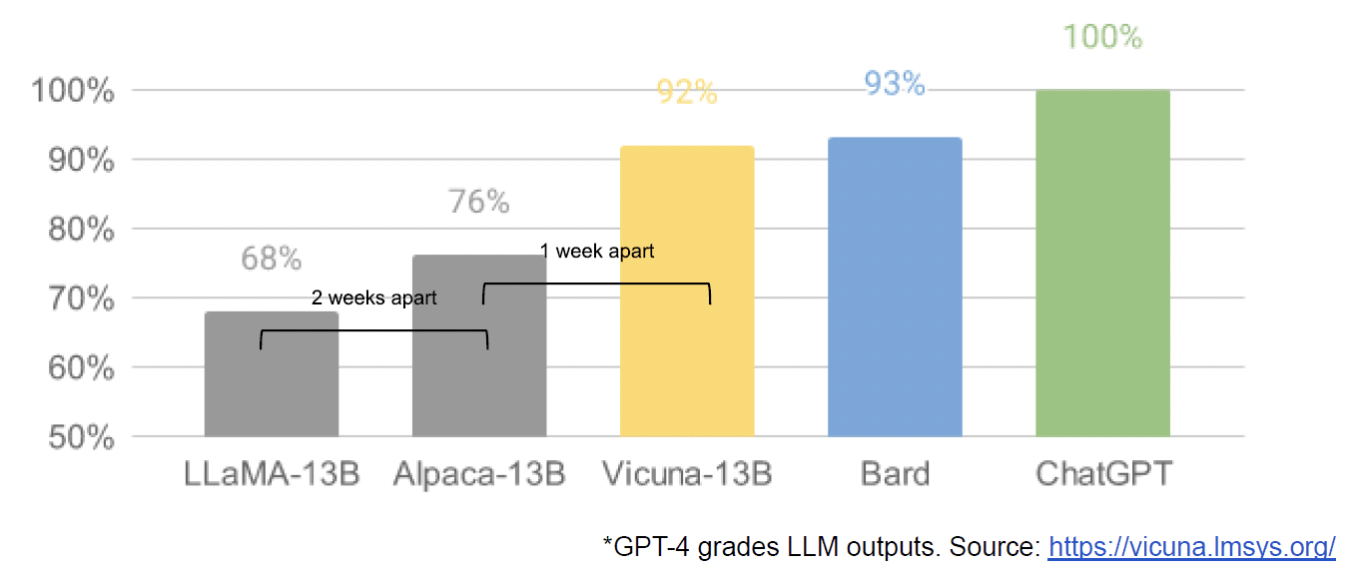
So, who’s correct? The anon engineer or DeepMind’s CEO?
Should Big Tech be scared of unassuming, scrappy AI startups and open-source projects with little to lose and a lot to prove? Are lumbering Big Tech incumbents (the currently dominant firms) too cumbersome to fend off swarms of agile startups, each biting away at their market share like fleas gnawing a dog?
Or are AI startups and open-source projects largely under the heel of Big Tech behemoths’ formidable talent, financial, and compute resources? Can startups protect themselves from regulatory capture, acquisitions, and other strategies that steamroll competition?
Business Rhymes with War
Perhaps both the juggernauts and AI startups will suffer death by a thousand cuts (à la open-source models). Only time will tell, but the leaked Google memo surfaces many important questions about defensibility.
Business isn’t war, but both share enough dynamics and lingo (all this moat and defensibility talk) that we can say they rhyme.
In “The Art of War,” Sun Tzu advised understanding your enemy and yourself if you seek ideal battlefield outcomes (i.e., winning); the second best option is only knowing yourself or only knowing your enemy; the worst option is understanding neither. Whatever “side” you’re on—startups, open-source, Big Tech, or some combo of these (they’re not mutually exclusive)—let’s heed Sun Tzu’s advice (swapping enemy for competitor) and consider all sides’ strengths and vulnerabilities to grapple with this startup versus incumbent thing.
Who Cares About Moats? Can’t We Just Build Cool Stuff?
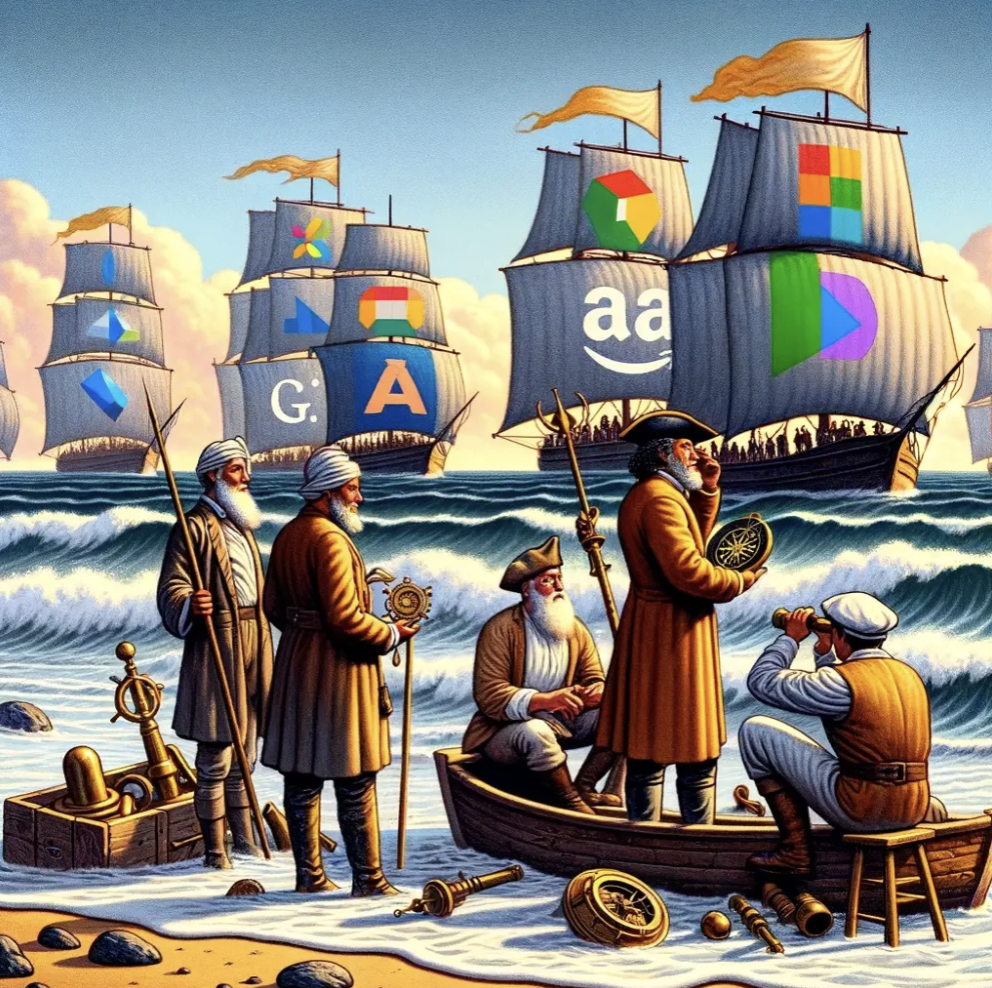
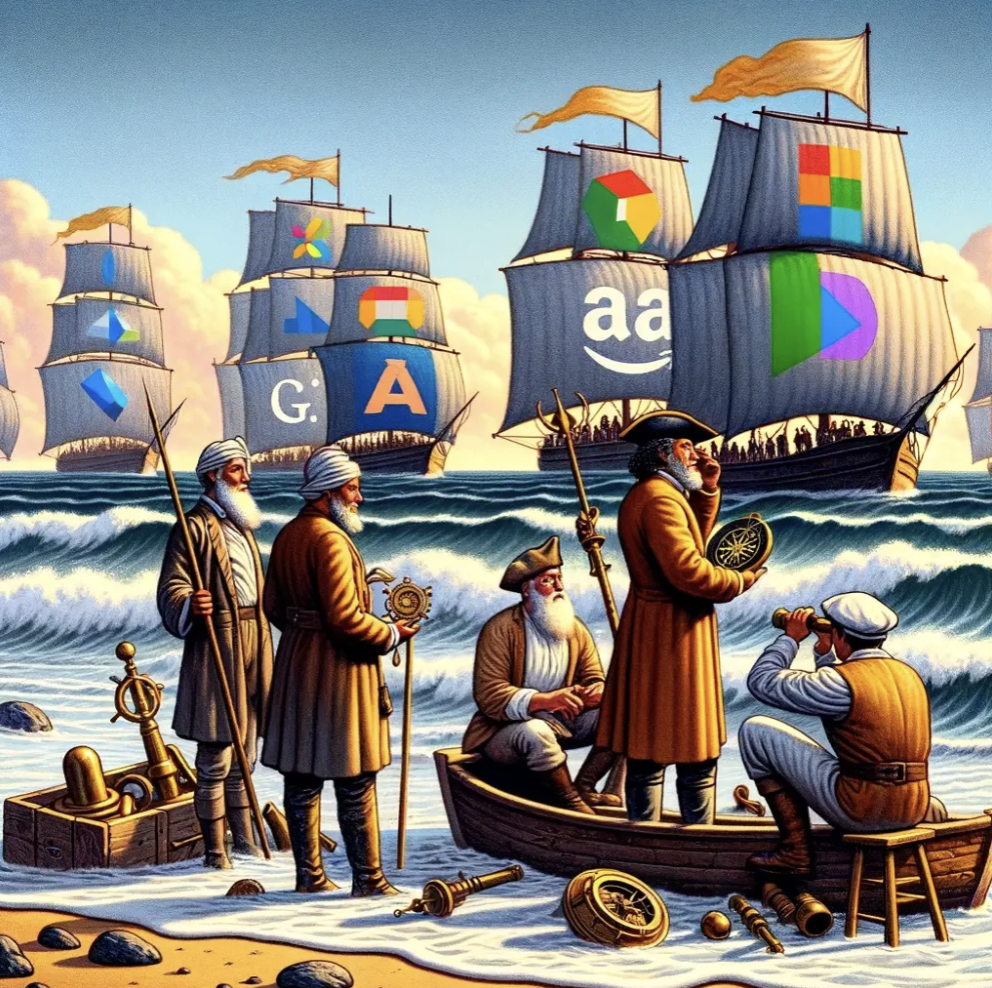
If you surveyed a hundred Venture Capitalists (VCs) about the most important competitive strengths and weaknesses of AI incumbents relative to their challengers (startups) and vice versa, good luck finding agreement; some zero in on data, some focus on the founders, some fixate on network effects, and the list goes on.
You will find significant consensus on this, though—that AI startups’ need defensibility to remain competitive for the long haul. Defensibility is a VC obsession.
Here’s why.
If an AI startup’s competing incumbents haven’t already added AI to their products, they’re likely to do so eventually—assuming the market reveals that a startup’s product is valuable. When this happens, how can that startup compete? What differentiates them from their competition and can they prevent the incumbent from catching up to them?
A startup’s value proposition doesn’t have to be better algorithms or models; sometimes something simpler, like better customer support or better design, can create a moat. But every startup needs something to serve as a barrier that the incumbent can’t easily breach.
Every startup needs a moat, but NFX's general partner James Currier points out that defensibility is even more vital in the digital world than the physical world because switching to a digital competitor often only requires a few clicks or taps (later, we’ll learn about an exception to this called switching costs). This especially holds true for customers using AI APIs; swapping APIs isn’t terribly tough to do, so AI startups that offer “AI as a service” should think hard about defensibility—just not right off the starting blocks.
Premature Defensibility Obsession
VCs want to bet on the right horse, so their scrutinizing moats is understandable. If you’re an early-stage startup—while it never hurts to strategize—stressing about moats too early might be counterproductive.
Investor and tech entrepreneur Elad Gil argues that nascent startups—by default—are easily replicable because it typically only takes a handful of people and less than a year to build them. In a similar vein, author and software engineer Matt Rickard aptly points out that “‘What if Open AI builds this?’ became the new ‘What if Google builds this’”, both oft-repeated questions equally unhelpful for early-stage startups (despite VCs’ insistence on asking them).
When numerous startups launch "chat with your personal data" products, incumbents like Google, Amazon, or Microsoft are about as bothered as a lion is by a few jackals probing its perimeter for some carcass scraps. Incumbents rarely pay much attention to startups until after they gain some traction and market share (usually between 4 and 7 years). Once this happens, though, incumbents will jump in the ring with startups. If this happens, then, yeah, you’ll want a moat.
We saw this with OpenAI. It was after ChatGPT’s meteoric rise to the mainstream that Microsoft transformed Bing into a “generative search engine” and Google released Bard in a bid to not be outshined.
Uncertainty as a Moat
According to author and investor Packy McCormick, early-stage AI startups have a strategic advantage over established ones because Big Tech rivals don't notice them until they have a clear product-market fit and then try to copy, destroy, or acquire them. Beyond being relatively obscure, AI companies that start up during bear markets and their coinciding economic pessimism also enjoy increased uncertainty.
Referring to this phase as “coverage,” McCormick contends that the balance between product development and moat-building shifts as certainty increases, modeling his ideal moat at any given startup stage as:
"Depth of Moat Needed = How Obviously Good Your Idea Is - How Hard it is to Build"
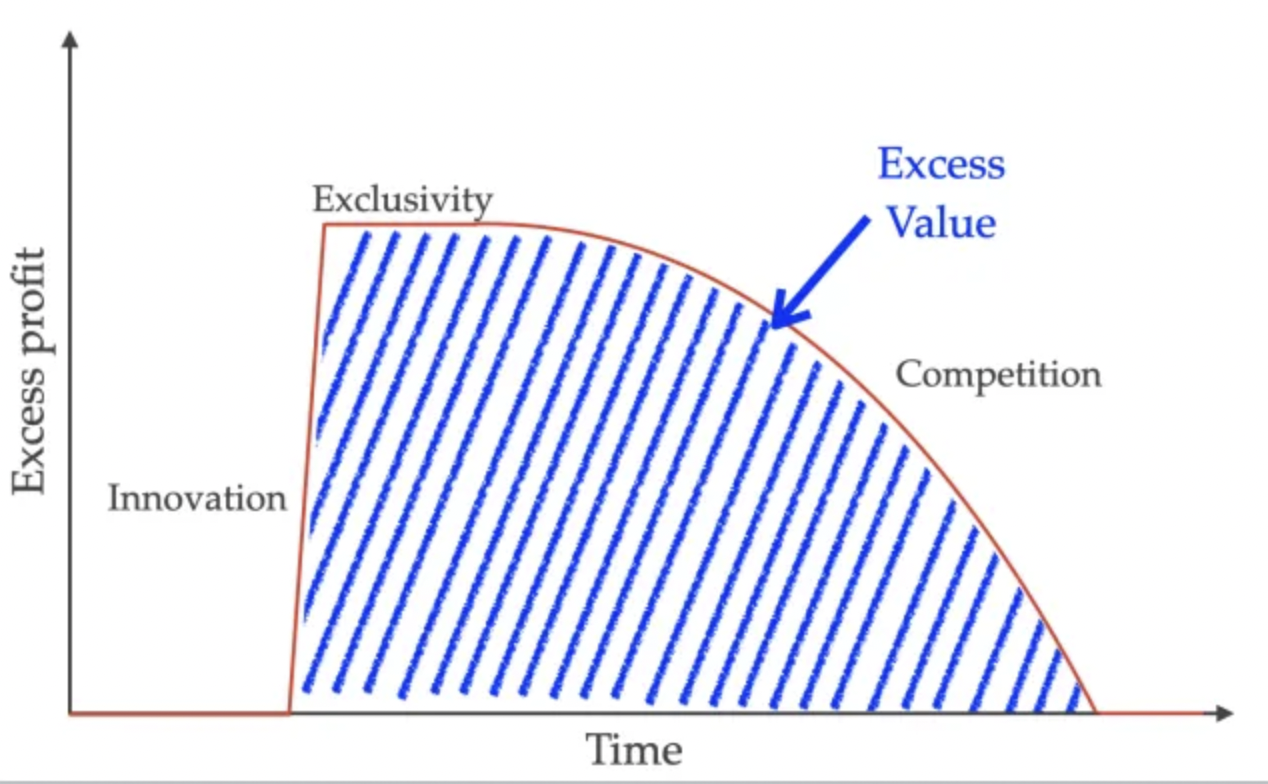
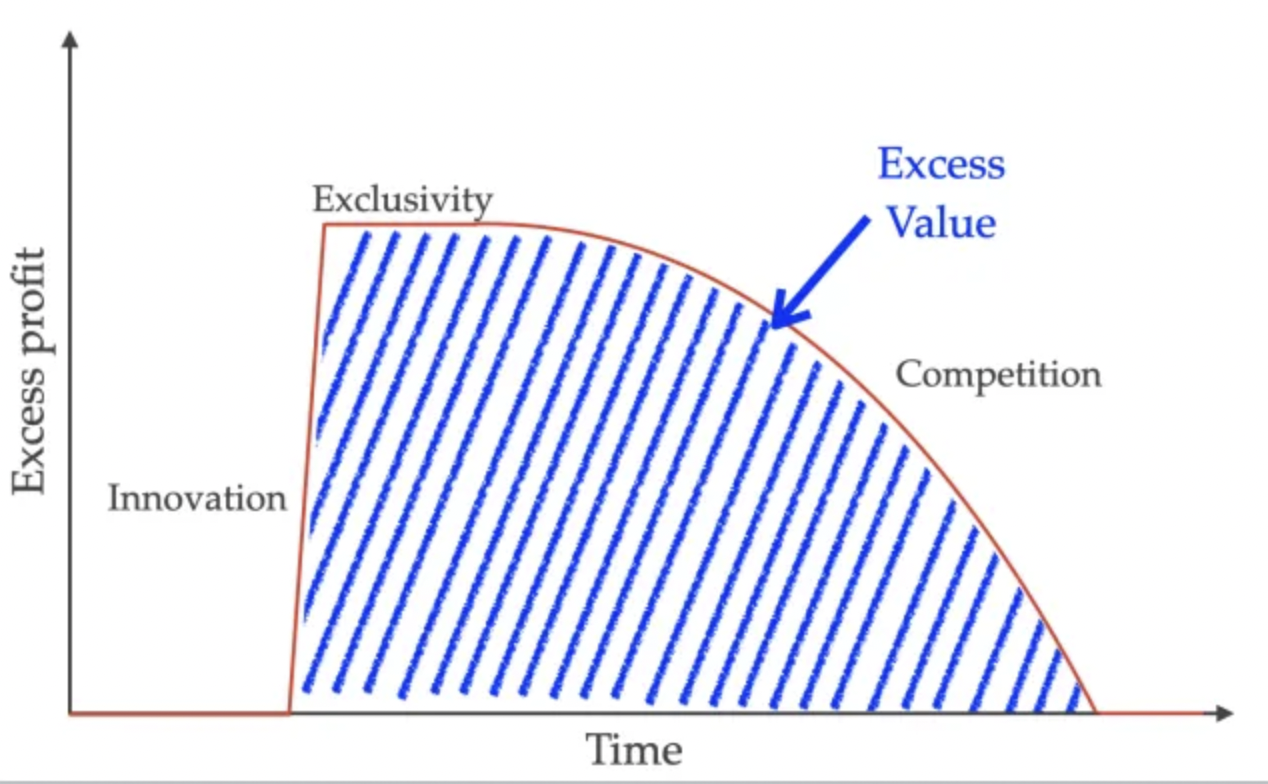
In McCormick’s view, investing heavily in defensibility before achieving product-market fit amounts to premature defensibility obsession, which is pointless for early-stage startups, so avoid this. Instead, use your obscurity period to focus on product-market validation. But once the spotlight discovers you, the gig is up, so shift to digging a moat.
With this in mind, let's proceed by analyzing AI startups and Big Tech’s competitive strengths and weaknesses relative to one another (many incumbents aren’t “Big Tech”, but for clarity, I’ll use incumbents and “Big Tech” interchangeably). Another point of clarification: competitive advantages tend to be short-term characteristics that help startups sprout and grow a bit; defensibility is what allows them to age into stalwart oaks. The boundary between these is fuzzy, but good to keep in mind. Let’s start with the most blatant advantage a startup or an incumbent can get—cash.
Funding
AI incumbents normally enjoy a clear funding advantage over AI startups. Early-stage AI startups, in particular, have less funding than incumbents, translating to less marketing, less research and development (R&D), less compute, and less of nearly everything (except for drive, perhaps).
This can be problematic because the data and compute necessary to train big AI models from scratch is costly (as the leaked Google memo revealed, though, it’s becoming increasingly cheaper and easier to get great results by finetuning existing open-source models on specialized data).
Beyond training costs, startups might eat significant inference costs for years before the market accurately prices the value of their product or service. Whether a startup survives this can depend on how quickly they find product-market fit relative to their fundraising successes. Microsoft, for example, likely has deep enough pockets to go on losing money on CoPilot for a long time—at least longer than most AI startups could do the same.
To survive the period between launch and finding PMV, early-stage startups often raise money from VCs. How much they raise influences AI startups’ ability to scale because they often need compute to train AI and more compute once they have customers (for inference).
So, while startups are at a monetary disadvantage relative to incumbents, capital investment allows them to hire faster (which, ideally, translates to developing, upgrading, and shipping products faster). If all goes well, this can turn into a momentum of follow-on investments for that startup and increasing hesitancy from other investors to back that well-funded startup’s competitors.
This means that startups funded more than competing startups have an advantage over competing startups. But this can become a double-edged sword because, with each fundraising round, startups tend to cede increasing control from the startup founders to their investors. VC backers can eventually gain enough influence to push founders to build fast for an exit, even if that wasn’t the founders’ initial goal. Thankfully, VCs aren’t startups only means toward funding, though.
Strategic Partnerships
Big Tech can invest in AI startups the same way VCs do, plus bring something even more valuable to the table—compute. This gives Big Tech so much leverage over VCs that some folks are even questioning why AI startups would prefer VC’s capital-only investments over the funding and compute Big Tech offers.
If new foundational AI models require increasingly more parameters and thus cost increasingly more to train, startup’s chances of training foundational models will dwindle without compute-strapped backers like Amazon, Google, Microsoft, Meta, and others, especially during the current fierce competition for chips. This dynamic is pushing many AI startups to seek Big Tech backing.
But what happens when AI startups team up with Big Tech for their compute? Is that a win-win? Or does one side absorb most of the benefits?
Matt Rickard believes that Big Tech often partners with AI startups because doing so fulfills a threefold objective:
Big Tech harness startups’ abilities to develop products quickly, thanks to startups’ financial incentives (potential for equity upside) and lack of bureaucracy
Big Tech can offload their risk if things go awry (e.g., a privacy breach, failure to find produc-market fit, etc.) because the startup serves as a reputational barrier between any screw-ups and Big Tech
If an incumbent-backed startup does great, Big Tech can gush about them and reap some of the profits
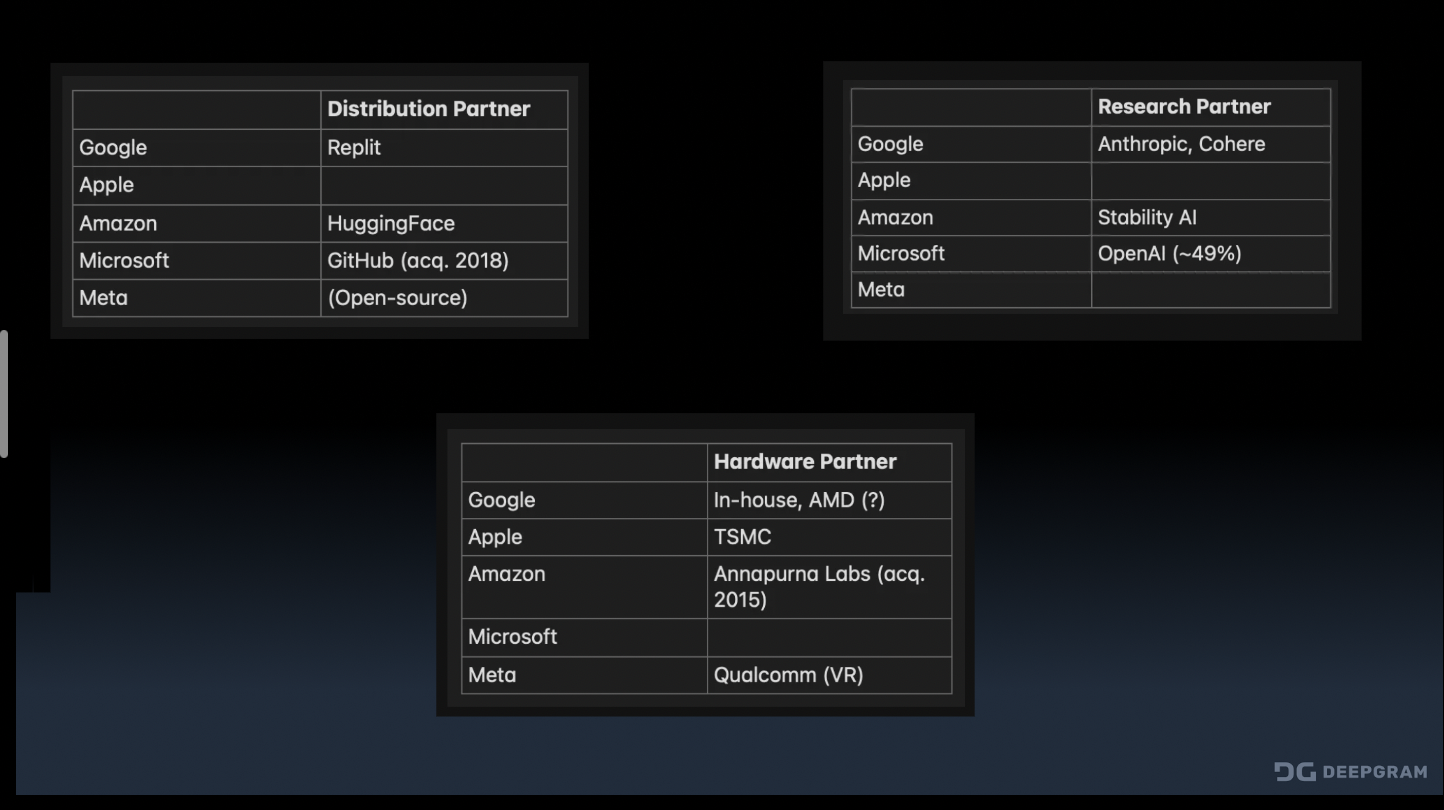
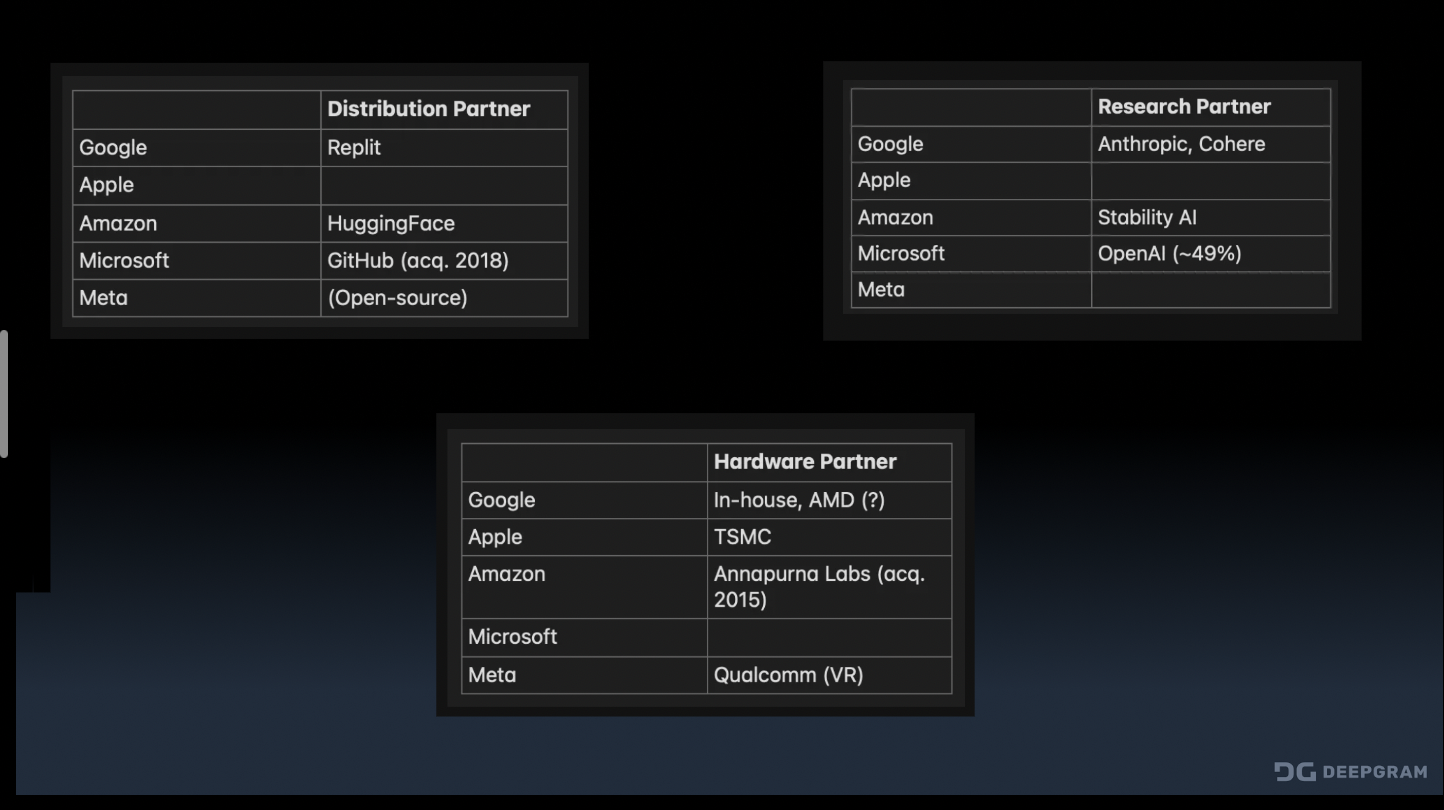
Open Markets Institute’s Max von Thun discusses an incumbent goal related to Rickard’s first point above. Big Tech’s increasing strategic partnerships with generative AI startups (like the recent Amazon-Anthropic deal) serve as contingency plans against their own in-house generative AI projects failing. Since these partnerships often make it easier for the incumbent backers to acquire the startups, von Thun likens these moves to historical “killer acquisitions” designed to eliminate competition (e.g., Google buying YouTube or Facebook buying WhatsApp).
And if that’s not enough competitive advantage for the leviathans, incumbents can also back open-source models as a proxy war of sorts against competitors. IBM, for example, backed Linux to counter Microsoft’s server software in the 1990s. Could Meta’s release of Llama 2 have similar aims?
Startups aren’t doomed to partner with incumbents, though. People are increasingly discovering creative, cheaper ways to train AI models (e.g., sharding, finetuning, etc.). Plus, governments, sovereign funds, and philanthropists sometimes offer grants with fewer strings attached than typical Big Tech or VC deals. Additionally, some ancillary tech firms like NVIDIA provide advanced computing resources to startups without competing directly against them (the more AI companies running inference, the better for NVIDIA). And sometimes startups—Deepgram being one of them—even offer resources to other startups.
All said, incumbents generally enjoy more of the advantages of strategic partnerships than AI startups do. But after a certain point, startups need to scale (a moat we’ll visit later), and to do so, they might not be able to avoid partnering.
Given these investor dynamics, how can AI startups compete and grow while maintaining sovereignty (assuming that’s what they want)? Thankfully, money and compute ain’t everything—people matter too.
Human Talent and Organizational Structure
Big Tech incumbents have a significant advantage over AI startups in attracting top engineers. They can woo over technical experts by offering them interesting, challenging, impactful technical problems along with plenty of resources to tackle those problems, not to mention ample career advancement opportunities and financial compensation.
This is a blessing and a curse, though.
AI talent frequently departs large, established companies’ entrenched, stifling bureaucracies, seeing AI startups’ freewheeling flexibility, lack of red tape, and flatter organizational structures as greener pastures—and when they do, they bring their know-how with them.
For example, within five years after Google released their pivotal "Attention is All You Need" paper, alerting the world to transformers’ power, only one of its authors remained a Googler; some that left did so to escape Google’s restrictive pace. And more recently, a non-trivial number of former Google and Meta engineers migrated to OpenAI, meaning startups can nab top talent from incumbents too.
Employees jumping ship is generally an incumbent vulnerability, but startups aren’t entirely immune to this type of brain drain. Several OpenAI employees, for example, left OpenAI to found Anthropic, now a contender to OpenAI, purportedly to focus on building safer LMs.
Startups enjoy a few more human advantages beyond being able to nab engineers from Big Tech. People interested in working at a startup that pays, at least in part, equity that might become worthless if the startup doesn’t make it (a strong probability) likely have a high risk tolerance (or didn’t think things through well). Folks looking for a safer bet often prefer placing their chips with an established firm. This often translates to startup cultures that are comfortable making bolder moves, sometimes finding gaps in incumbents’ defensive posturing (assuming those bold moves are the right moves).
Another startup strength is that they tend to be lean, flat organizations. Though sometimes more chaotic, this allows them to execute faster. Big Tech’s typical organizational structure, on the other hand, is bloated and hierarchical, which, by its nature, equires an incessant passing of things up and down cumbersome approval chains.
While offering more control, this slogs up the time to implement changes and saps initiative and innovation. Charles River Ventures‘s Vivian Cheng sees this influence in incumbents’ attempts to cram AI into their existing products. Cheng describes these efforts as “redundant, indefensible, and generally unimaginative,” arguing that most of the recent AI innovation arose from bottom-up startups rather than top-down incumbents. This is likely thanks to, in no small part, the people at AI startups and their (lack of) organizational structure.
Data
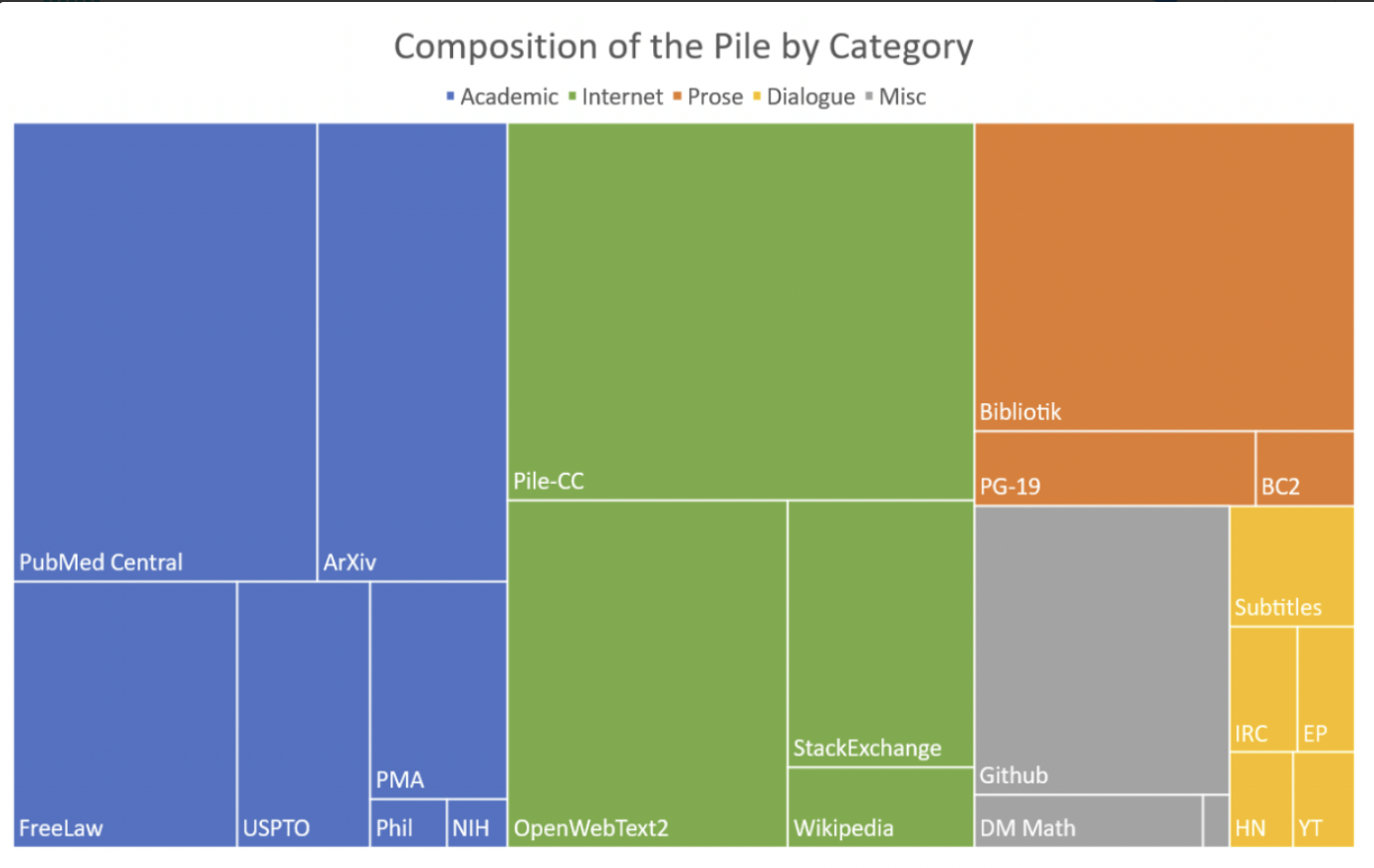
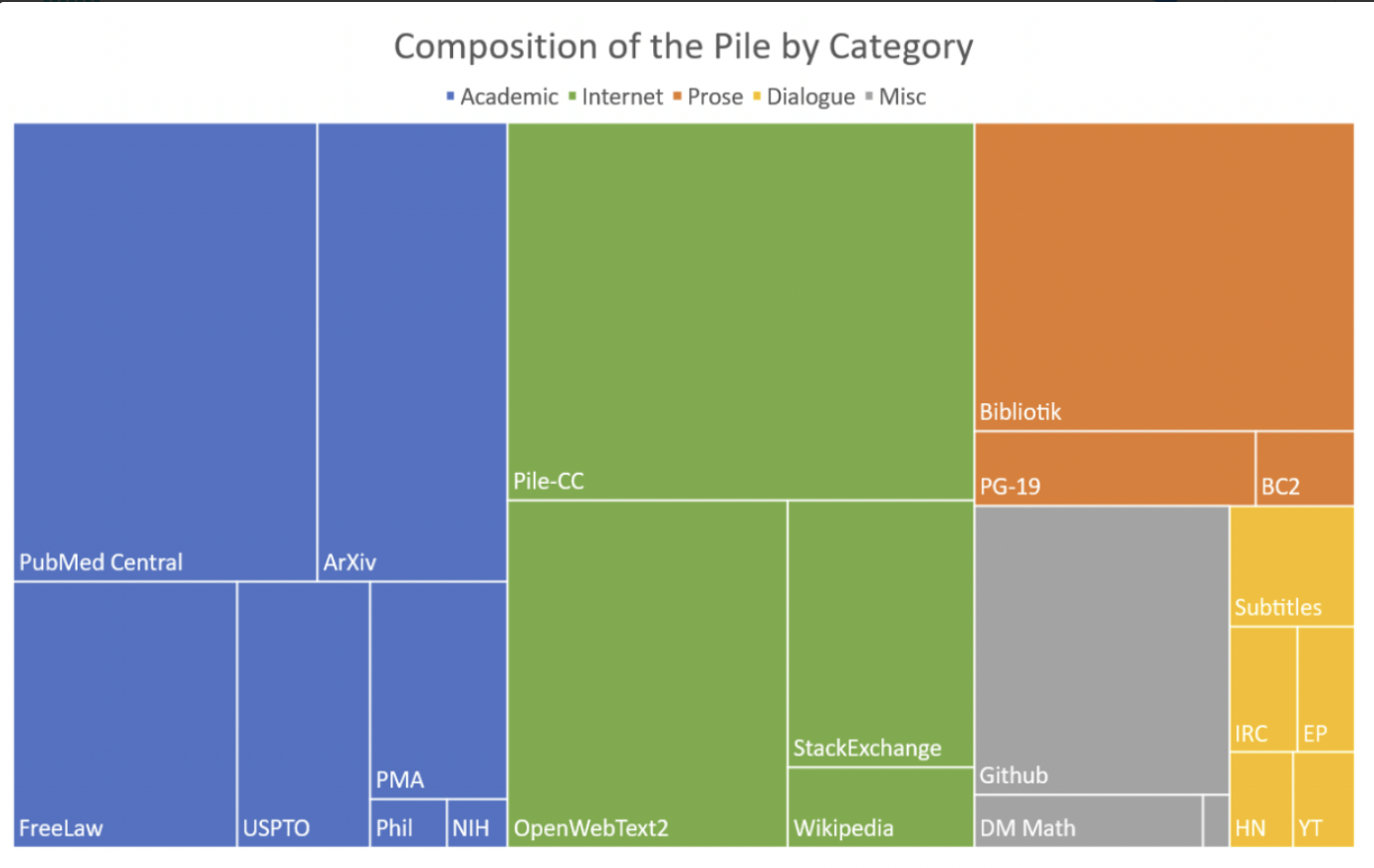
Next is data, a crucial component for useful AI models. It’s tough for startups to compete with the sheer oceans of data that Big Tech firms’ swipe from billions of users “accepting” their long, legalese privacy policies. But startups can gain data advantages in other ways.
First, quality data trumps data quantity. Unique, niche, cleaned, or proprietary data can give startups strategic advantages compared to the often uncurated data that Big Tech gulps up en masse.
In addition to having access to good external data, Brandon Gleklen, Principal at Battery Ventures, says that early-entry startups can see their own user data as a competitive advantage because it creates feedback loops for later AI model improvements. Additionally, breakthroughs in using synthetic data to augment real data could also create moats for startups (or incumbents), at least until such techniques become mainstream. Data also plays into the next defensibility factor—the type of market an incumbent or startup targets.
Vertical and Horizontal Markets
Vertical markets are like spear fishing (very targeted); horizontal markets are like casting a wide net. Currently, AI startups can gain more of a competitive advantage by pursuing vertical markets than they would by going after horizontal markets.
Finetuning a language model on niche legal data, for example, allowed EvenUp, a personal injury legal AI-related startup, to raise $50 million in their Series B valuation. There are many, many vertical markets that language AI, for example, can add value to (too many for incumbents to target all of them). But, since vertical markets don’t scale the way horizontal markets do, a startup in a vertical market needs to evaluate how valuable that market is overall and what percent of it they might capture.
Horizontal markets, on the other hand, offer plenty of room to scale but are more competitive than vertical markets. Google, for example, which focuses on search, doesn’t need to worry about scaling (tons of people use search engines); they need to worry about competition instead. Horizontal markets are tougher for AI startups to attack, but not impossible.
Counter-Positioning
The business tactic of taking the bull by the horns is counter-positioning. Digital cameras, for example, overtook the film photography giant Kodak by challenging them head-on like this.
How exactly did Kodak lose their dominance?
Digital cameras were lousy in their early days, so, even though digital cameras represented a potential rivalry (assuming they’d eventually improve), Kodak didn’t divert much funding from their cash cow (film photography) to digital camera R&D for such an uncertain, far-off threat. But even if Kodak had aggressively funded such R&D, there’s no guarantee they would have pivoted to an effective business strategy for a market with less film demand. Most photography moved from cameras to phones, and images moved from prints to the cloud—a non-obvious development to predict. So Kodak kept playing defense and eventually lost to the insurgents. Similar scenarios played out with Skype versus long-distance telephone plans, Uber versus taxis, and AirBnB versus hotels.
AI startups might do the same. Perplexity.ai, for example, is counter-positioning against Google’s cash cow—search. Google must balance protecting their main ad revenue-generating product with developing new products like Bard. Perplexity, on the other hand, can focus solely on getting generative search right and a business strategy to go with it (perhaps generative ads built into their results). If this ends up working for Perplexity, their defense will have been their strong offense (counter-positioning), but Google’s size advantage makes Perplexity’s work cut out for them.
Economies of Scale
Size can be a moat in itself. Incumbents tend to enjoy benefits derived from scale more than AI startups, including cheaper access to suppliers, partners, compute, data, and more. This advantage, called economies of scale, also makes it easier to gobble up the majority of certain resources.
Cornered Resources
Incumbents who’ve achieved massive scale across horizontal markets can corner the majority of material or human resources. For years, for example, Google gobbled up many AI PhDs. Now, OpenAI is trying to coax some of Google’s AI experts to switch companies by dangling $10 million carrots (i.e., salaries) in front of them. Similarly, Nvidia has the GPU market well cornered, which is causing OpenAI, for example, to deliberate acquiring their own hardware business. And if that’s not enough, powerful incumbents can even corner “legislative resources” to their advantage.
Regulatory Capture
Regulation can carve out a Grand Canyon for the titans of industry—a moat that’s nearly insurmountable for small AI startups. Realizing this, incumbents often seek to sway regulation to their advantage and to their challengers’ detriment, something we’re seeing unfold in real time.
OpenAI, formerly a nonprofit, pivoted to OpenAI-in-name-only, a “capped-profit” entity, shortly after Microsoft invested $1 billion in them in 2019. Fast forward a few years to 2023, and OpenAI’s briefly former but now returned CEO Sam Altman—with his war chest fattened to $13 billion thanks to Microsoft—has aggressively advocated for AI regulation that, a real shocker, Altman offered advice in crafting. Sam Bankman-Fried took a similar approach prior to FTX’s collapse, courting CFTC and SEC officials in an unsuccessful attempt to steer financial clearinghouse requirements toward FTX’s benefits.
Who knows. Maybe some of the same folks who—under the cover of legal gray areas, non-scrupulously ingested a big chunk of the entire internet, including many artists’ and authors’ works—trained several iterations of a dangerous-enough-to-beg-for-regulation-but-safe-to-ask-you-to-fork-over-20-bucks-a-month large language model (i.e., GPT 3.5, 4, 4.5) magically discovered a deep sense of responsibility toward the greater good.
What we do know is that regulation, intended or not, often amounts to regulatory capture. Licensing processes, often convoluted and complex, stymie new market entrants like early-stage AI startups that lack the battalions of lawyers and lobbyists that Big Tech employs. Worse (for startups), regulatory bodies often evolve into vicious industry-to-regulator-back-to-industry-again revolving doors. Once this happens, fledgling startups’ voices, already barely a whisper, fade to a pipsqueak.
AI researchers Andrew Ng and Yann LeCun perceive the White House’s latest AI executive order as a rushed and perhaps uninformed response to veiled attempts at regulatory capture. To its credit, the White House’s recent AI executive order did place caveats between foundational models and otherwise (though this boundary is fuzzy). Ng suggests that if open-source and smaller startups don’t challenge AI safety sensationalism, Big Tech will solidify regulatory capture for AI.
So what can startups and open-source do?
Band together and crowdsource their fans and customers to annoy their government representatives. This might look like a centralized Github repo where numerous AI startups and open-source projects suggest and vote on what they’d like to see included in or left out of AI regulation. Then, each startup could push out the consensus view to their email lists, requesting their subscribers to contact their congressional reps. Much of this could be partially automated. While such an effort might amount to a drop in the ocean compared to Big Tech’s lobbying, a drop still creates ripples.
Network Effects
Next is networks. Products where each new user added creates value for every other user (i.e., a positive feedback loop) have strong network effects. A social media product (e.g., Facebook, LinkedIn, Twitter, Instagram, etc.) is typically more useful for any single user the more users are on that platform. Similarly, AirBnB is more useful to guests the more hosts there are (and vice versa). Same deal with Uber for drivers and riders.
Network effects make wide (and valuable) moats. Microsoft acquired LinkedIn for $26 billion in 2016 and Facebook acquired WhatsApp for $19 billion in 2014, largely thanks to the moat that their network effects created.
Reinforcement Learning from Human Feedback (RLHF) might create a network effect for ChatGPT. Assuming people give feedback to its responses, the more people that interact with ChatGPT, the better it should become, which should attract more users, then more feedback and further improvements, and so on. Similarly, the more people that host their models on HuggingFace, the more useful that platform becomes for the people using it. Network effects might be one of the more feasible moats for AI startups to get, though exactly how to build them into AI products is mostly uncharted territory.
Switching Costs
Next is switching. Lindy’s CEO, Flo Crivello, gives this example: if you’ve used a gmail address to communicate with your friends and family for several years, you’re not likely to switch to another provider even if you can’t stomach gmail’s UI or tracking policy because doing so costs you the hassle of sending everyone you’re new address and archiving or migrating old messages. This sometimes intentional phenomenon, switching costs, can create defensibility and can be achieved through a few different avenues.
SAP and Oracle, for example, are complicated and costly enough to integrate into businesses that their customers rarely switch to competitors; it’s not worth their time or money to do so. Related to integration costs are the sunk costs incurred learning a new platform or framework. Crivello also gives this example: if you’ve spent months learning Photoshop, you’re likely to stick with it so you don’t feel like the time you invested in learning it is wasted.
Integration switching could become a moat for AI startups offering custom finetuned models for vertical markets and accompanying specialized technical talent to integrate these models into customers’ workflows (something like SAP but geared specifically for niche AI applications). Switching costs might, eventually, also factor into the next defensible aspect we’ll discuss.
Distribution
Where will we run AI models five years from now? Just as few could foresee back when the iPhone was released that it’d eventually have GPS capabilities that’d give rise to taxi industry-disrupting apps like Uber or Lyft, we can only guess what AI’s main distribution channels will be.
And this matters a lot.
For example, llama.cpp outshined the Python version that Meta released because llama.cpp can run on more machines than the original (it runs on CPUs). AI models running on phones or IoT devices, for example, will open novel use cases, so AI startups that are mobile first will likely enjoy a distribution advantage over AI startups without a mobile app. But, here’s the catch: Big Tech incumbents, namely Apple and Google, already own big mobile product distribution channels: the App Store and the Play Store. Startups’ best bet here is likely to diversify across different distribution channels and think ahead (maybe wearables will catch on).
Geographic Location
Beyond digital product locations (distribution), physical location matters too. AI Startups located near capital, press, and big pools of engineers have a leg up on startups outside of geographical tech hubs. Startups in Silicon Valley, for example, generally gain advantages in several regards over startups based in Winnemucca.
But AI startups outside of Silicon Valley can still create geographical moats. They might, for example, strategically locate themselves near a university that specializes in research related to their product to more easily hire their graduates. Or AI startups in nations with less Big Tech might harness their geography to their advantage. A language AI startup in Mogadishu, for example, is better positioned to curate unique Somali legal data than one based in Silicon Valley.
Intellectual Property
In many fields, intellectual property (IP) forms a strong moat. While patents can become moats in software too, software companies often don’t bother with them because software is relatively easy to reverse-engineer.
Even if their inner code varies, competitors can usually build something that—given the same inputs you’d feed into your program—produces similar enough outputs to be functionally the same as yours. This is probably even more true of AI models since they’re less interpretable than traditional software. So, an AI startup might create an IP moat, but they’re less likely to do so than incumbents.
Types of AI Models
Related to IP, we should think about the types of AI models that incumbents and startups develop. Models trained from scratch, finetuned models, and API-based models all have different levels of defensibility.
Models Trained From Scratch
Big Tech and startups can train their own foundational AI models from scratch. How feasible this is increasingly depends on the modality. Training an image model from scratch, for example, is considerably cheaper than training a LM from scratch. So, currently, modality affects how defensible a foundational model is, but even LMs, despite being more computationally and financially expensive to train, are less and less defensible as open-source and leaked LMs continue proliferating. Plus, it’s feasible that new LMs will routinely replace incumbent LMs. If, for example, new top-performing foundational LMs are trained every 6 months, they’d lose their competitive advantage quickly, failing to serve as a moat.
Finetuned & Open-Source AI Models
Even less defensible are finetuned and open-source models because they’re more readily accessible, faster, and cheaper to train than trained-from-scratch models. This is an advantage for startups with fewer resources who might iterate via routine finetuning, but—since its an advantage that every other startup enjoys too—it’s not a moat.
AI Models via API
Another option is accessing AI models via APIs. Often, when a startup builds on top of such APIs, the incumbent can eventually build a similar tool, nullifying the need for the “wrapper” that the startup provided.
A common criticism of startups relying on OpenAI’s APIs, for example, is that they amount to “wrappers startups” completely at the mercy of OpenAI. Given that some have described OpenAI’s recent DevDay as the "ChatGPT wrapper apocalypse" (OpenAI’s new features wiped out several startups’ differentiability), it seems this was a prescient criticism.
AI APIs are undoubtedly helping product-focused folks enter a market previously dominated by researchers, which helps more people build minimally viable products faster, but these APIs—by themselves—don’t offer much defensibility.
Brand
Brands influence people's perceptions, which in turn influence their consumption behavior. Because of this, startups and incumbents’ brands can become moats. Apple fans, for example, rarely compare an Apple product to a non-Apple product; rather, they compare Apple products to other Apple products, thanks to Apple’s brand. The same can take place with AI startups.
Warranted or not, it’s undeniable that OpenAI snagged some of the “AI company” narrative from Google (with machine learning integrated into many of their products, Google remains an AI giant but recently has lost much of the perception game). Since LM products only recently became mainstream, people using them are happy to experiment and switch often, meaning brand moats are still ripe for digging, even for small AI startups. By treating their customers well, listening to them, and involving them in product roadmaps, brand is likely the defensible element most within AI startups’ locus of control.
Planning is Everything
Ok, so we’ve now analyzed many of AI startups’ and incumbents’ fundamental strengths and weaknesses relative to one another. But how can an AI startup actually gain defensibility against incumbents?
First, just acknowledge that no castle, not even your own, is impenetrable; there’s always a way in. Accepting this propels you to plan. By keeping the above in mind and continuously monitoring the market and assessing your competitors and yourself (as Sun Tzu advised), startups can develop plans (make many contingencies, not just one) for etching out defensible positions.
Just don’t plan on your plans working out.
Speaking about an upcoming opponent, Mike Tyson once told a reporter, “Everyone has a plan until they get hit for the first time.” While reality throws most plans awry, they’re far from futile, something General Dwight D. Eisenhower once succinctly described when he said, "Plans are worthless, but planning is everything.” In other words, the mental processes involved in thinking through different scenarios give you an edge over those who neglect planning.
So plan away and dig your moats, but be ready to pivot on a dime since none of us can read the tea leaves perfectly. Black swan and wildcard scenarios exist. A tiny startup might engineer artificial general intelligence. Or new learning algorithms could disrupt everything the way transformers overtook convolutional models at many tasks. An AI startup, for example, might apply liquid neural networks to language models, slashing reliance on compute resources and opening the AI market to anyone with a personal computer. Since AI innovation moves fast, often throwing many factors into flux all at once and forcing a complete reworking of your business strategy, an AI startup’s deepest moat might be their flexibility and agility.
Note: If you like this content and would like to learn more, click here! If you want to see a completely comprehensive AI Glossary, click here.
Unlock language AI at scale with an API call.
Get conversational intelligence with transcription and understanding on the world's best speech AI platform.