How AI Consumes Water: The unspoken environmental footprint
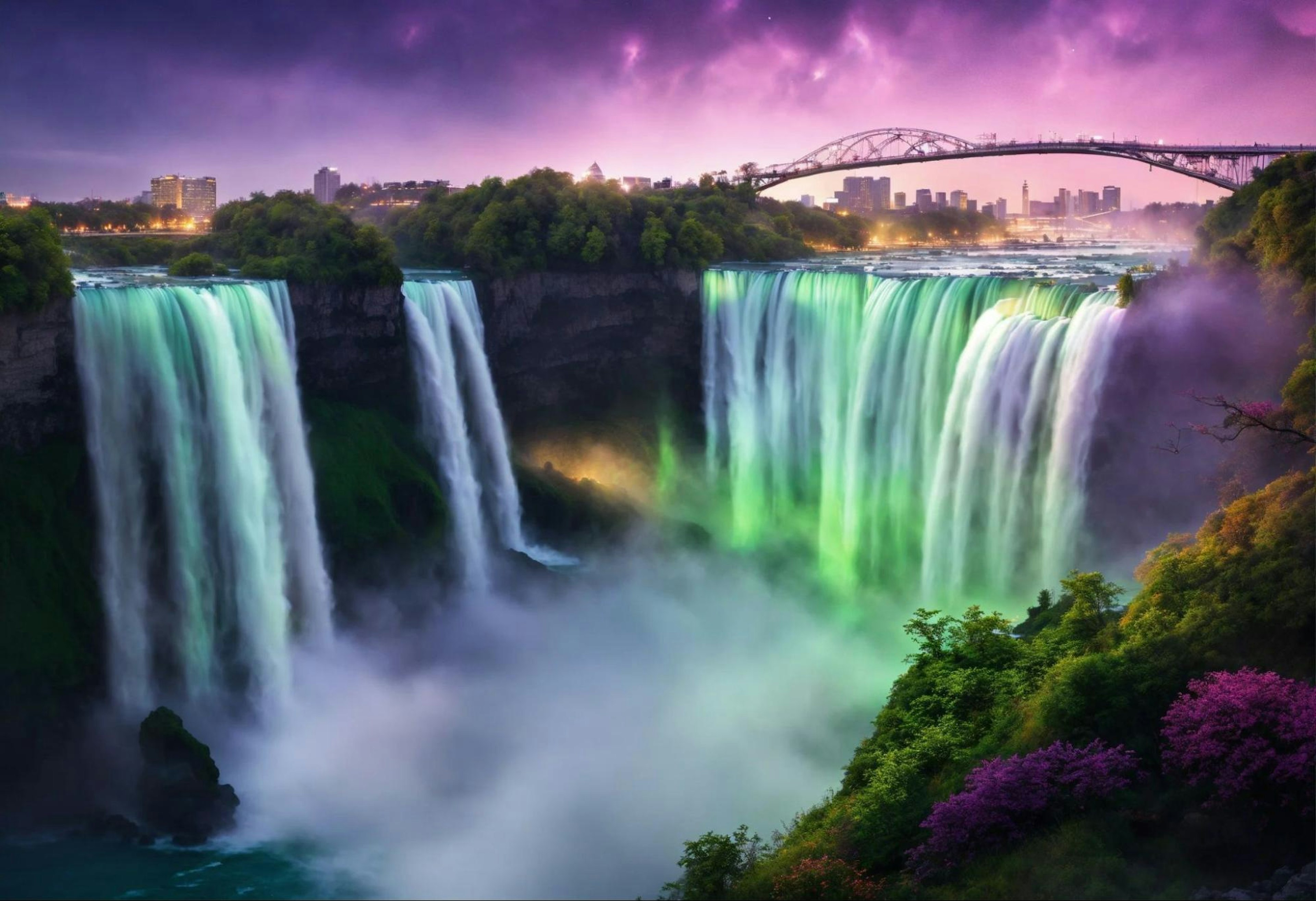
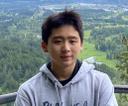
When someone mentions the negative environmental impacts of AI, electricity and their respective carbon emissions may be the first thing that comes to mind. In fact, the intersection of artificial intelligence (AI) and environmental sustainability is becoming a critical area of study and concern. As AI systems like the GPT-4 model become more complex and difficult to train, their environmental impact is coming into focus. However, what most people don’t realize is the alarming water footprints that AI models leave behind.
The concept of a "water footprint" in AI refers to the total volume of water used directly and indirectly during the lifecycle of AI models. This includes the water used for cooling vast data centers and the water consumed in generating the electricity that powers them. To put it in perspective, training a single AI model like GPT-3 could require as much as 700,000 liters of water, a figure that rivals the annual water consumption of several households. Moreover, a simple conversation with ChatGPT consisting of 20 to 50 questions can cost up to a 500ml bottle of freshwater.
Understanding the implications of this requires us to differentiate between "water withdrawal" and "water consumption." Withdrawal is the total volume taken from water bodies, which often returns to the source, albeit sometimes in a less pristine condition. Consumption, however, is the portion of withdrawn water that is evaporated, incorporated into products, or otherwise not returned to its source. It's this consumption that can exacerbate water scarcity, leading to a pressing global issue.
AI's water footprint is tied to its energy needs. The electricity that powers AI systems often comes from sources like hydroelectric power, which, while renewable, has its own set of environmental impacts, including water use. As AI models grow in complexity and size, their energy—and therefore water—demands follow suit.
Reducing the water footprint of AI models can be done from several aspects. This includes optimizing algorithms for energy efficiency, developing more sustainable data center designs, and shifting the timing of intensive AI tasks to coincide with periods of lower electricity demand, which can reduce the reliance on non-renewable energy sources.
How is AI Using Water?
Before discussing and investigating how to mitigate the significant water footprints of AI models, we need to first understand how water is used in training machine learning models. AI's water use is multifaceted, encompassing not just direct usage for cooling but also indirect consumption through power generation and server manufacturing.
Why is Water Needed for Cooling Systems?
AI systems, particularly those involving high-performance servers with hundreds if not thousands of graphics processing units (GPUs), generate a considerable amount of heat. To maintain a reasonable temperature in data centers and avoid overheating of processing units, data centers employ cooling mechanisms that are often water-intensive.
Water is a superior cooling agent for data centers due to its high specific heat capacity and thermal conductivity. It can absorb a lot of heat before it gets warm, making it efficient for regulating the temperatures of high-performance servers. This is especially crucial in AI data centers, where equipment runs continuously and generates significant amounts of heat.
Moreover, water's ability to be pumped and recirculated through cooling systems allows for a stable and continuous transfer of heat away from critical components. This ensures that the data centers remain at optimal operating temperatures, which is essential for the reliability and efficiency of the AI systems they house.
The Three Scopes of Usage
Data center cooling systems can be generally categorized into 3 scopes in different stages of data centers.
The first scope of water use in AI involves the direct operational cooling of data centers. These facilities house the servers responsible for AI computations and, due to their high-density power configurations, generate considerable heat. To maintain optimal temperatures, data centers traditionally use water cooling systems. These systems function either by absorbing heat through evaporation in cooling towers or by transferring heat away in a closed-loop system. The water can be recycled and reused to some extent, but there's always a portion that is lost to evaporation or discharge, making it a consumptive use of water.
The second scope is less direct but equally significant. It pertains to the water used in the generation of electricity that powers AI systems—often referred to as 'off-site' water use. This water usage is dependent on the energy mix of the grid supplying the power. For instance, a data center powered by hydroelectricity or a fossil-fuel plant will have different levels of water withdrawal and consumption based on the efficiency and cooling requirements of these power plants.
Lastly, the third scope encompasses the water used in the manufacturing of AI hardware, including the servers and the semiconductors within them. This process, which often requires high-purity water, can have a substantial footprint, considering the sheer scale of global semiconductor production. This is a more indirect form of water use but contributes to the cumulative water footprint of AI and AI advancement. The continued development of larger and mode complex models will increase the demand of processing units and thus the production of hardware will rise as well.
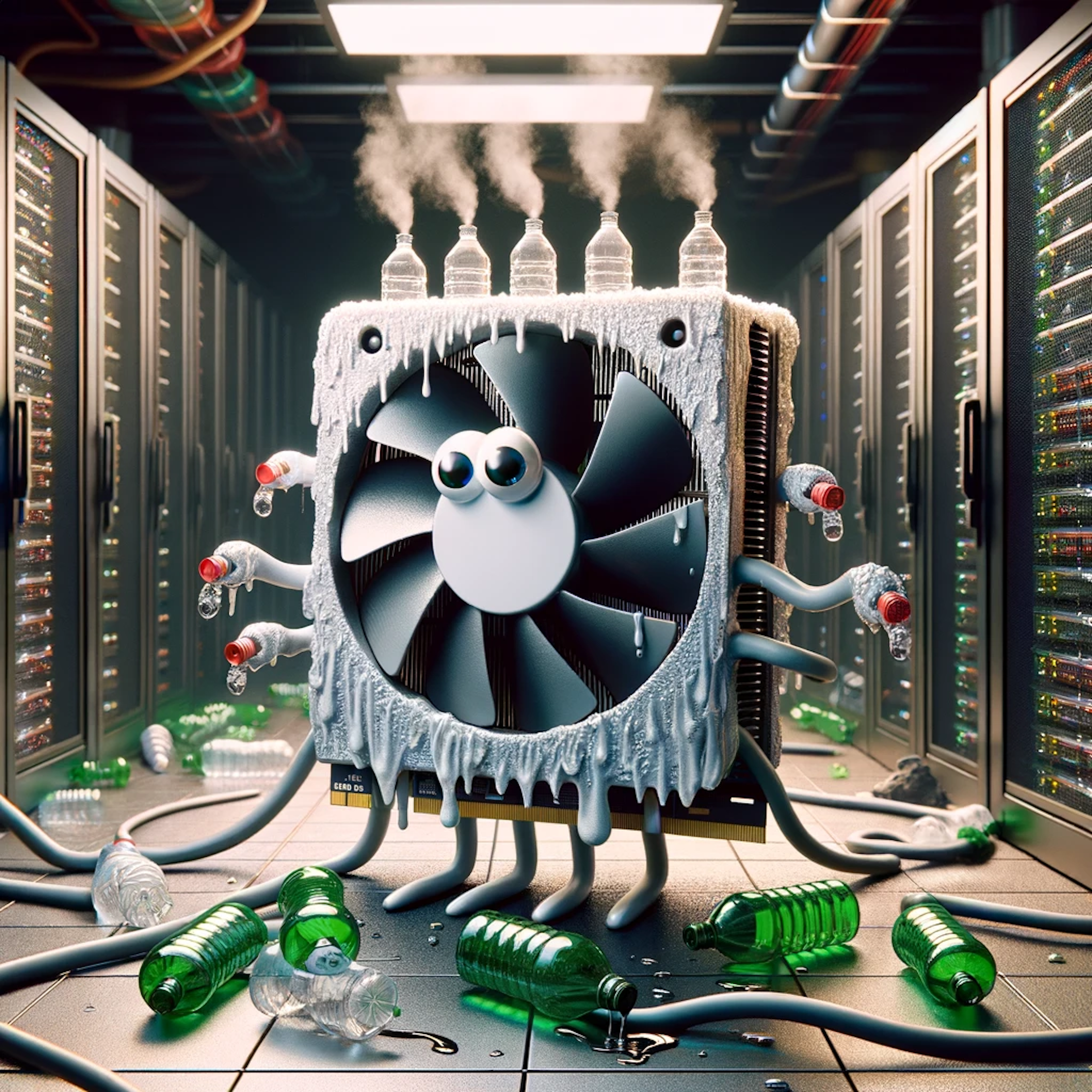
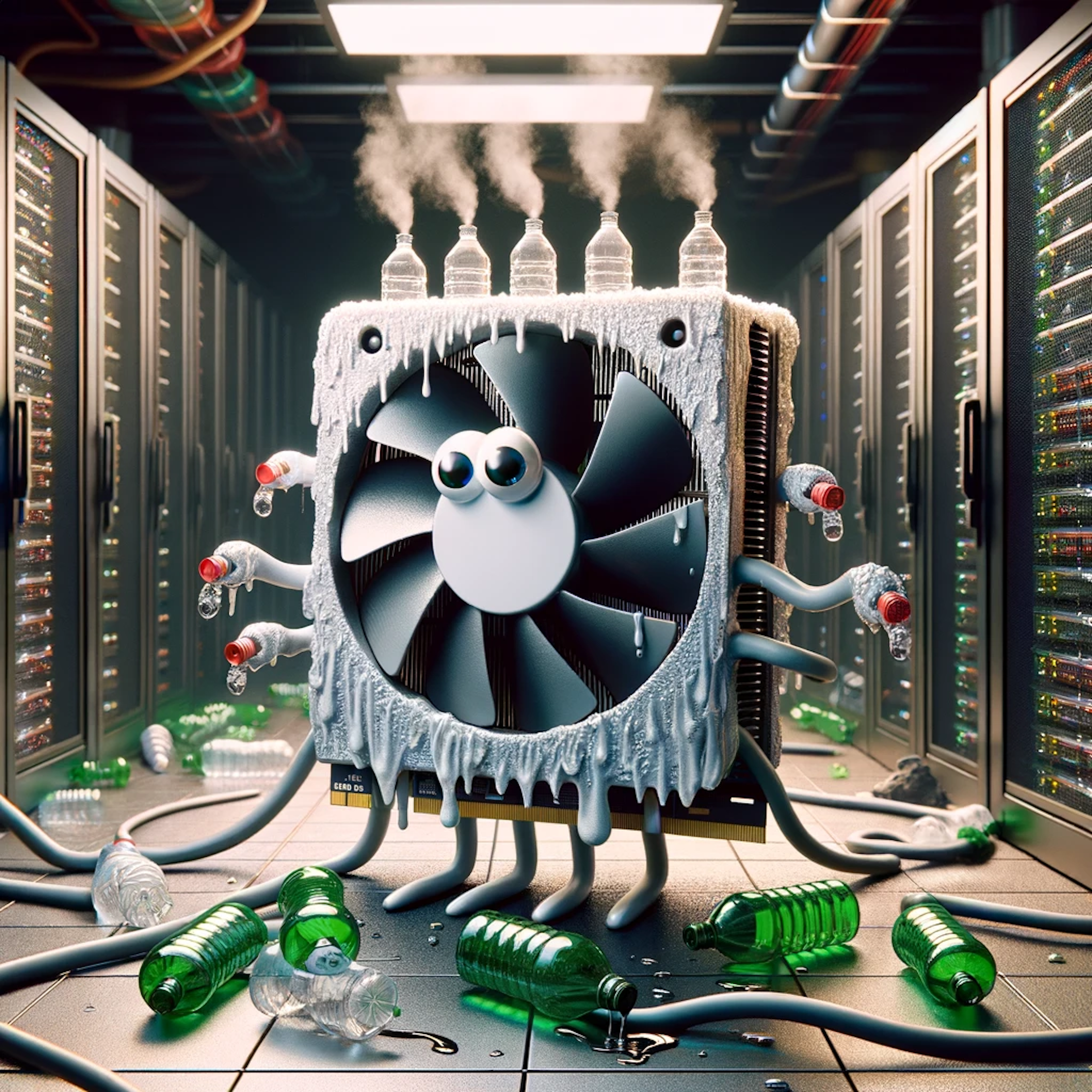
Estimating Water Consumption of AI Models
The task of quantifying the water footprint of AI models is a complex task that involves multiple factors and aspects. To accurately measure this footprint, a multi-faceted approach is required, taking into account direct water use, the intricacies of energy generation, and the manufacturing processes of AI components.
The authors of the paper “Making Al Less "Thirsty": Uncovering and Addressing the Secret Water Footprint of AI Models”, propose a novel approach to estimating the water footprint of AI computations.
At the operational level, direct water usage is tracked through the Water Usage Effectiveness (WUE) metric, which captures the volume of water used for cooling per kilowatt-hour of energy consumed by AI systems. This is particularly relevant for data centers where AI models are operational, as these facilities often require significant cooling to manage the heat generated by high-density servers. The WUE is sensitive to a range of factors, including the external temperature, which can lead to higher cooling requirements during warmer periods.
Turning to indirect water use, the equation becomes more complex. Here we introduce the concept of water consumption intensity factor (WCIF), which is linked to the energy source powering the data center. Since the energy mix can vary — incorporating coal, natural gas, hydroelectric power, nuclear energy, and renewable sources — the WCIF is subject to change. It encapsulates the average water used for every unit of electricity that comes from the grid, inclusive of variables like evaporative losses at power plant cooling towers.
The overall operational water footprint (W_operational) of a machine learning model is then calculated using the following equation:


In this equation, `e_t` is the energy consumption at time t. The theta-1 term denotes the fraction of that energy cooled by on-site water, and the theta-2 term denotes the fraction cooled by water via off-site electricity, each with their respective WUE and WCIF.
But the footprint extends beyond operational use. The embodied water footprint (W_embodied) accounts for the water used in the life cycle of server manufacturing and maintenance, distributed over the hardware’s expected lifespan:
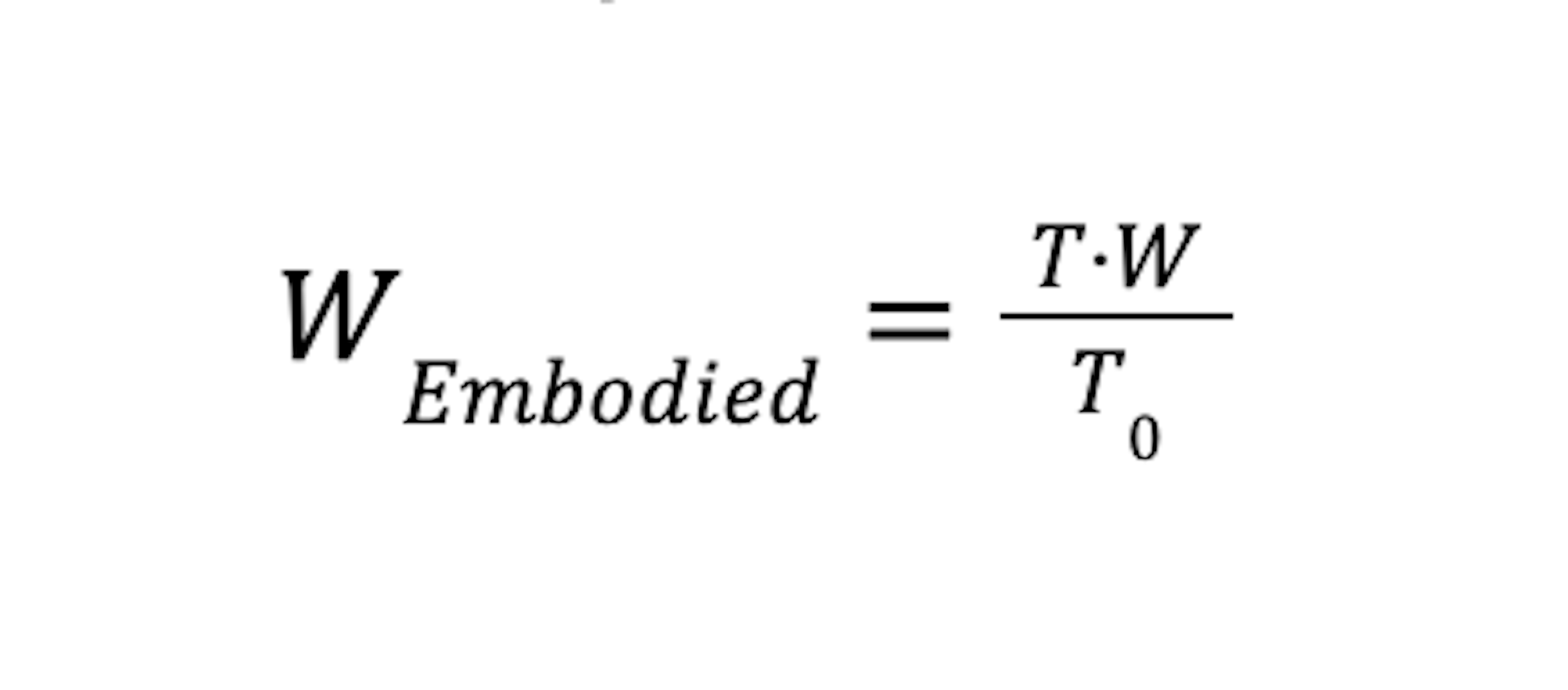
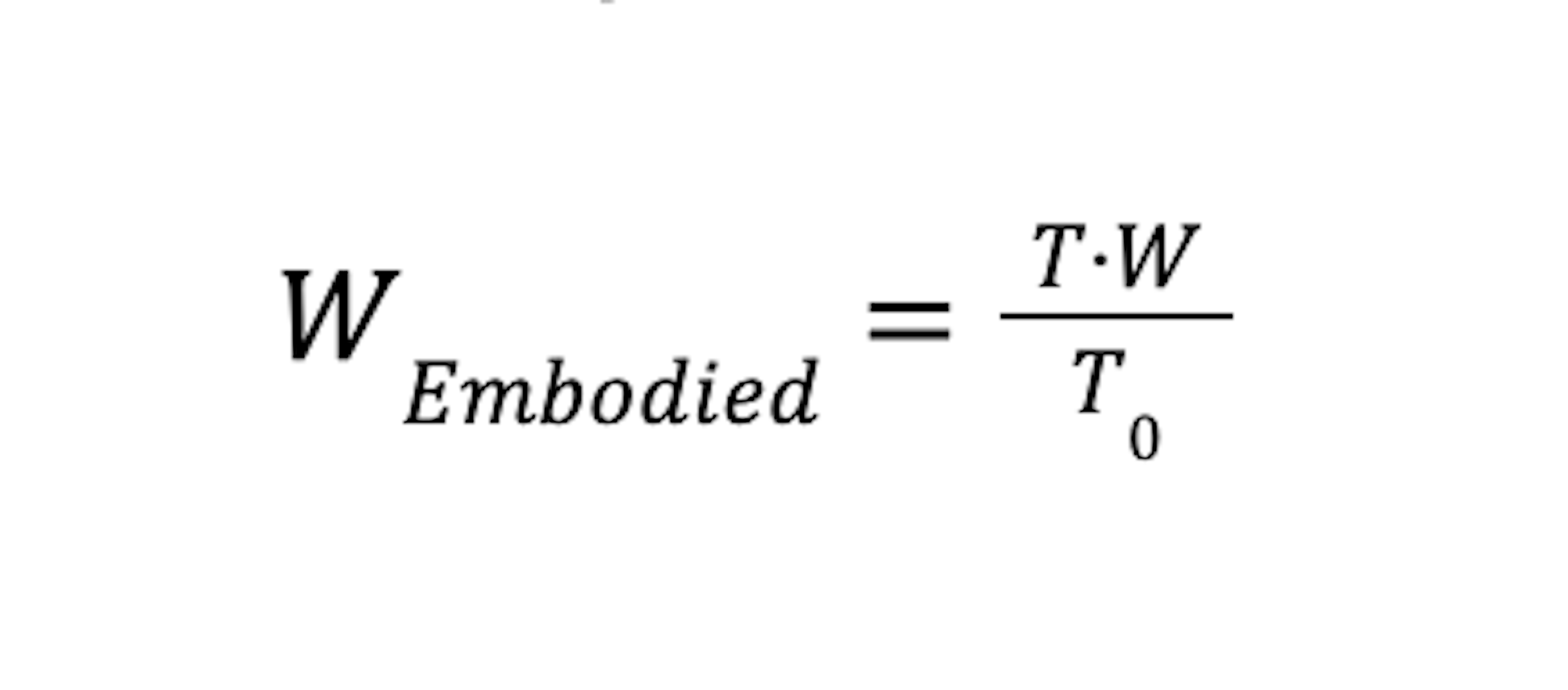
Here W represents the total water used in the manufacturing, T representing the operational time period, and T0 being the hardware’s projected lifespan.
Combining these elements gives us the total water footprint:
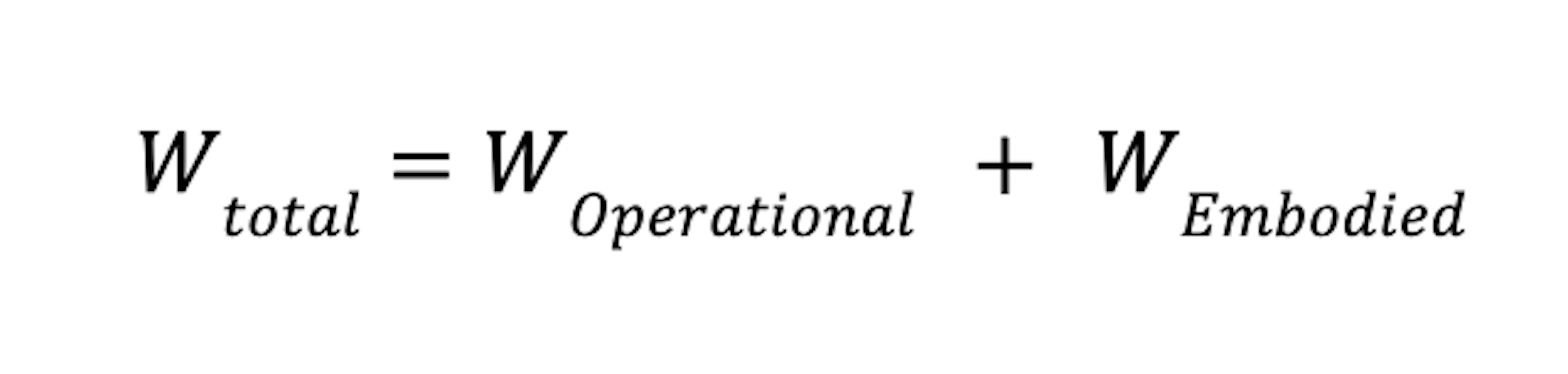
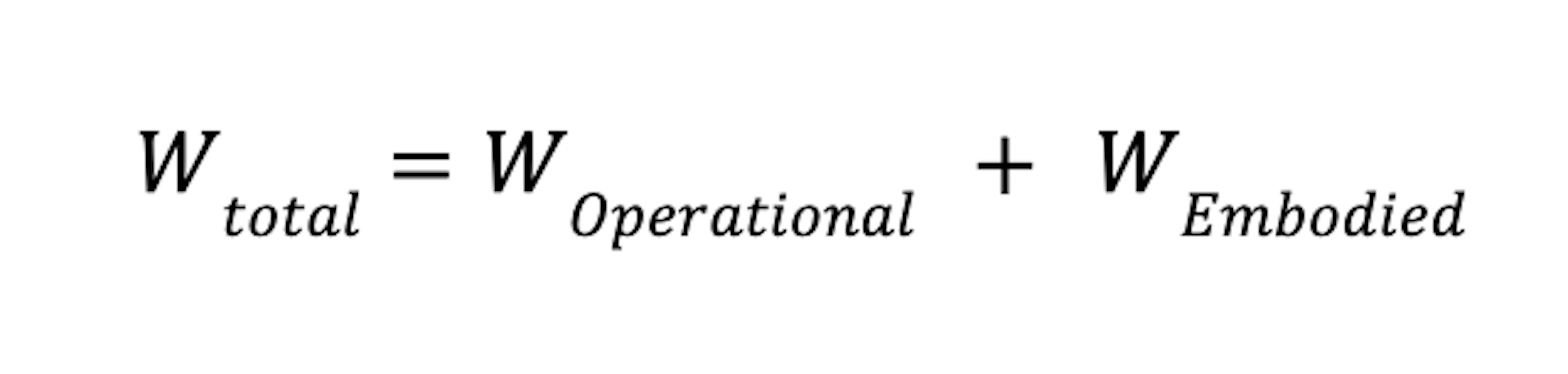
This formula provides a holistic view of the AI model’s water footprint, combining real-time operational data with the broader environmental context of AI's lifecycle.
Below is a data table from the paper outlining water consumption of the GPT-3 model.
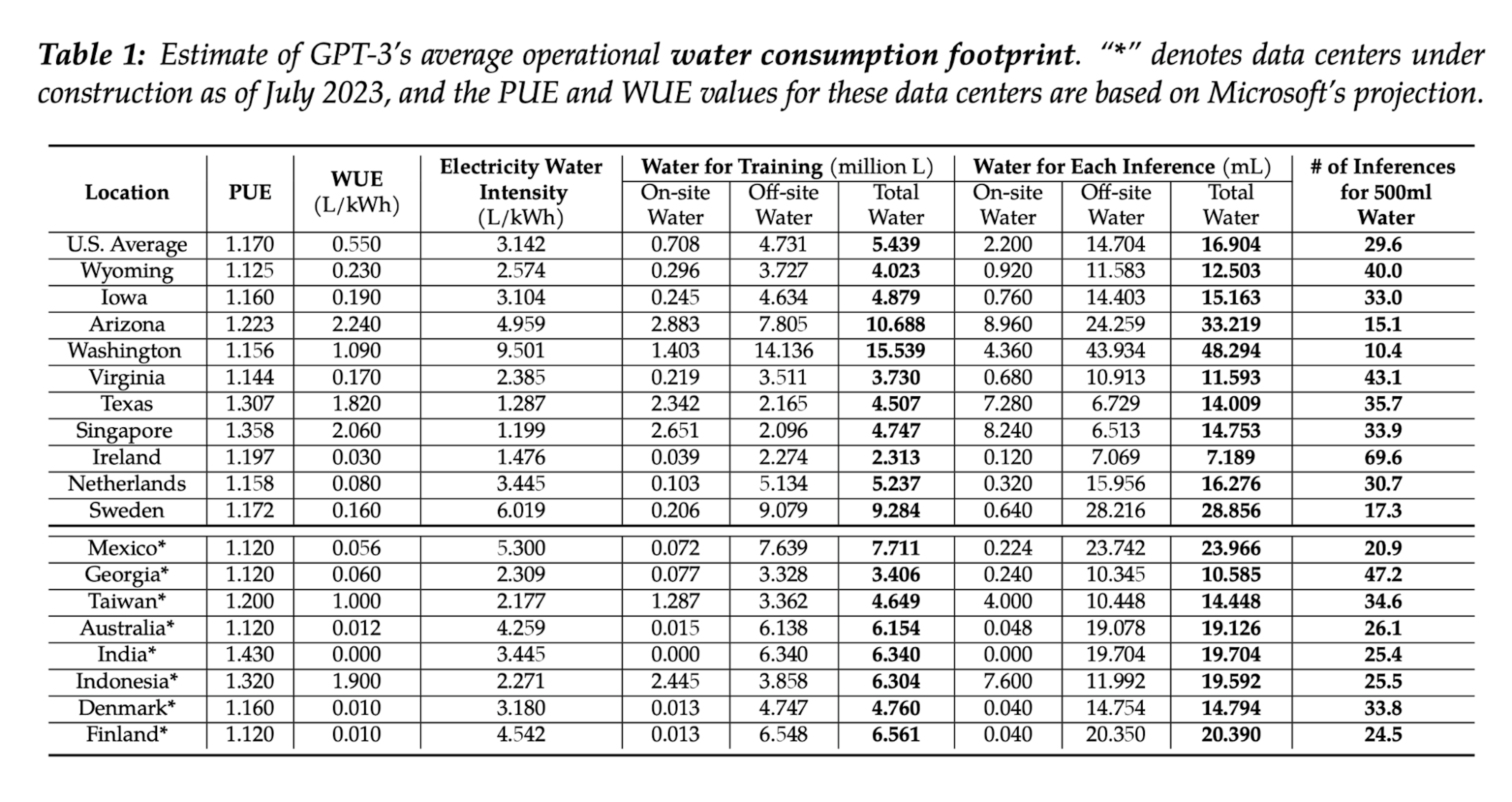
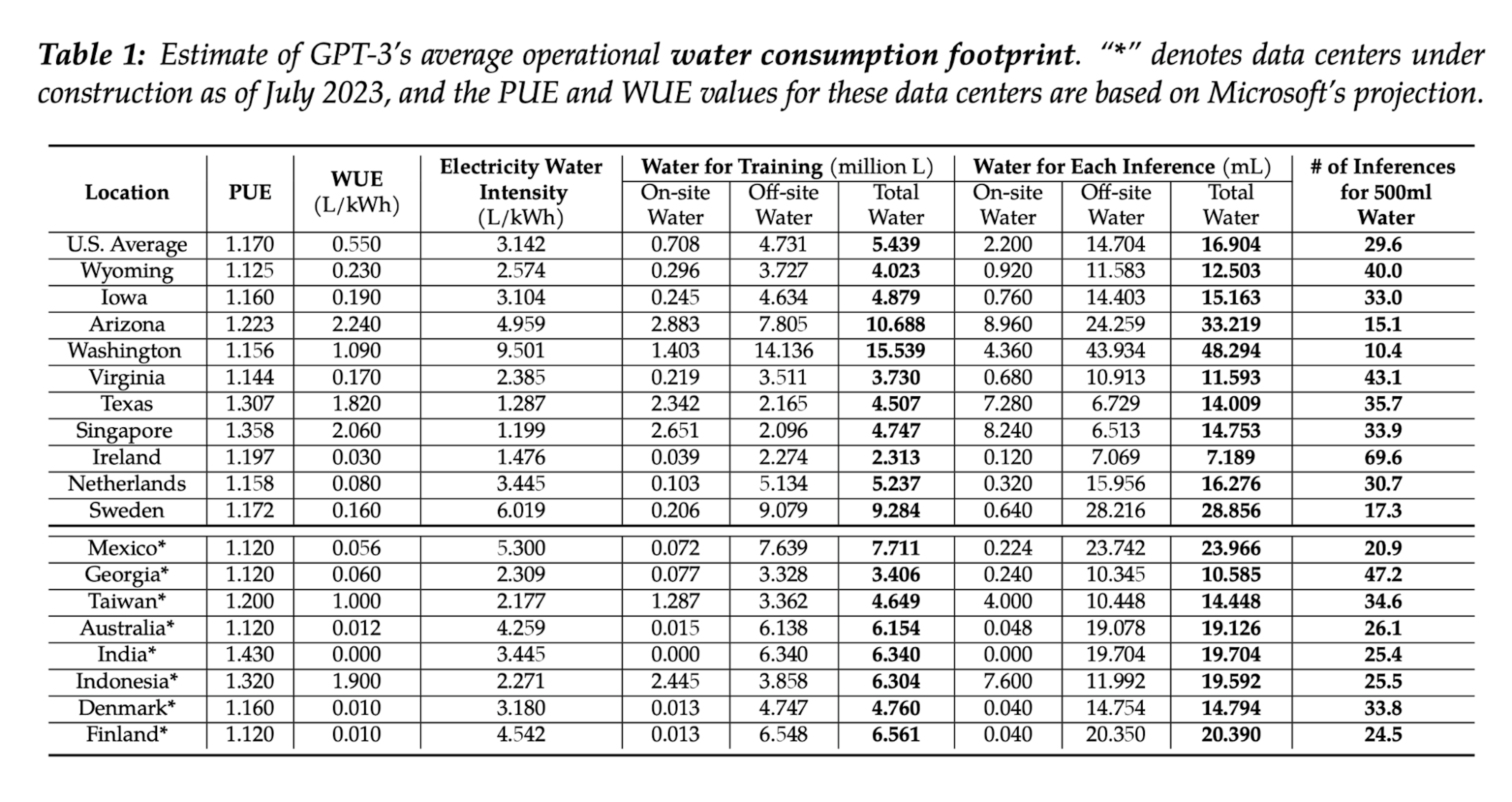
Diving into the granular data provided on GPT-3's operational water consumption footprint, we observe significant variations across different locations, reflecting the complexity of AI's water use. For instance, when we look at on-site and off-site water usage for training AI models, Arizona stands out with a particularly high total water consumption of about 10.688 million liters. In contrast, Virginia's data centers appear to be more water-efficient for training, consuming about 3.730 million liters.
These differences are largely attributable to the Water Usage Effectiveness (WUE) and Power Usage Effectiveness (PUE) values, which vary by location. WUE and PUE are indicative of the efficiency of a data center's cooling infrastructure and overall power usage, respectively. For instance, the WUE in Virginia is recorded at a low 0.170 liters/kWh, suggesting that the data centers in this state are among the most water-efficient in the table provided. This is a stark contrast to Arizona, where WUE is much higher at 2.240 liters/kWh, hinting at a less water-efficient cooling process in a hotter climate.
The table also points to the water used for each AI inference, which can be startling when we consider the scale of AI operations. Taking the U.S. average, for instance, we can see that it takes approximately 16.904 milliliters of water to run a single inference, of which 14.704 milliliters are attributed to off-site water usage. To put this into perspective, if we think about the number of inferences that could be run on 500ml of water — the size of a standard bottle of water — the U.S. average would allow for about 29.6 inferences.
With more than 100 million active users to date along with the introduction of GPT-4, the water consumption resulting from day-to-day usage is staggering. Additionally, GPT-4 is almost 10 times as large as GPT-3, possibly increasing the water consumption of inference and training by multiple folds compared to GPT-3. It is somewhat ironic that we are taught to reduce shower time or reuse water in order to conserve the usage of water, without knowing just how much water is disappearing from talking to an AI Chatbot.
What Does it Mean for the Future?
Of course, this is not to say that all AI training and usage should be halted in favor of saving water. The paper's results and insights serve as a fair warning to the resources being consumed, many of which have mostly gone unnoticed. That said, the tech industry is not turning a blind eye to the environmental costs of AI and data center operations. In fact, there's a wave of innovative efforts to mitigate these costs.
For instance, Google's AI subsidiary, DeepMind, has applied machine learning to enhance the efficiency of Google's data centers, achieving a 40% reduction in energy use for cooling. This advance translates to a 15% reduction in overall Power Usage Effectiveness (PUE) overhead, which is a measure of data center energy efficiency. This innovation not only reduces energy consumption but also indirectly decreases water use, as less energy required generally means less water used for cooling.
Moreover, Microsoft has ventured into the depths with Project Natick, experimenting with underwater data centers. The ocean provides a natural cooling environment, which dramatically reduces the cooling costs that contribute significantly to a data center's operational expenses. Notably, an underwater data center tested by Microsoft off the Scottish coast achieved a PUE as low as 1.07, which is impressively more efficient than the PUE for conventional, newly-constructed land-based data centers, which is about 1.125. This innovation not only speaks to cooling efficiency but also to the potential for integrating data centers with renewable energy sources like offshore wind, solar, tidal, and wave power.
These initiatives are not mere drops in the ocean but significant strides toward sustainable computing. They underscore a commitment to finding solutions that balance the unrelenting demand for AI and computing power with the imperative to preserve environmental resources. As these technologies continue to develop, they offer a blueprint for reducing the water footprint of AI and other energy-intensive industries.
In conclusion, the journey towards eco-friendly AI is multi-faceted, involving advancements in AI itself to optimize energy use, exploring unique solutions like underwater data centers, and a broader shift towards renewable energy sources. These efforts collectively form a promising horizon where AI's thirst for water is quenched not by drawing from our precious reserves, but through innovation and the relentless pursuit of efficiency. The insights from the paper serve as a reminder and a catalyst for ongoing efforts to ensure that AI's footprint on our planet is as light as possible while ensuring the pace of technological advancement.
Note: If you like this content and would like to learn more, click here! If you want to see a completely comprehensive AI Glossary, click here.
Unlock language AI at scale with an API call.
Get conversational intelligence with transcription and understanding on the world's best speech AI platform.