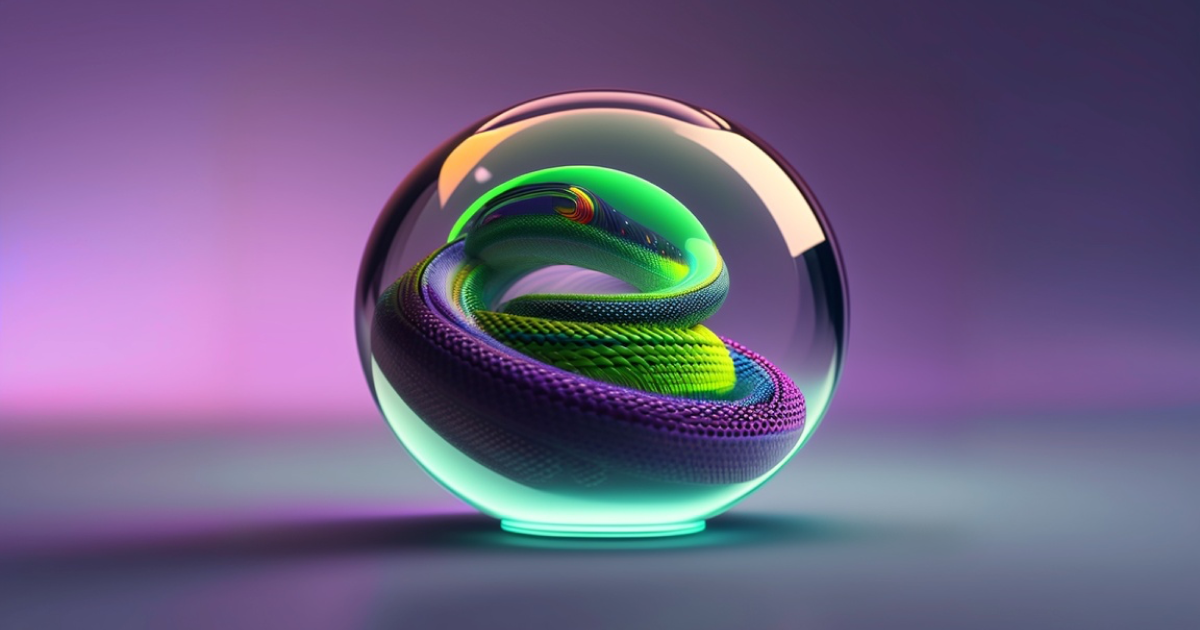
Generative AI models are currently experiencing a boom in mainstream consciousness with the launch of ChatGPT, the wildly popular text-based model, alongside other well-received AI models. From ChatGPT, which is now a go-to source of assistance and resources, to Midjourney, which is creating images that can almost pass as real, generative AI is quickly becoming a daily tool for millions of people over the world.
The outputs of these models, which are trained on large amounts of data, can also be used to replace real-world data in some cases. ChatGPT, for example, can be prompted to generate data that is similar to actual data and can be used to train other AI models. This is called synthetic data, and it is usually used when real-world data is not available or to diversify datasets. But according to a new study, models trained on these outputs are likely to collapse or go “MAD” after five training cycles.
Model Autophagy Disorder (MAD)
Using synthetic data to train AI models is often cheaper and more convenient, especially if a large amount of data is needed or there’s a need to protect sensitive data. In some cases, synthetic data works better compared to real-world data, but most times, they are used because we are quickly running out of easily accessible and high-quality real-world data to train large language models. In fact, it is likely that machine learning datasets will deplete all high-quality language data by 2026. As AI outputs and synthetic data continue to make up a larger portion of available data, we might get to a point where the generated data may end up polluting the training set of the next generation of models, leading to model collapse.
Model Autophagy Disorder (MAD) is a phenomenon whereby a model collapses or “eats itself” after being repeatedly trained on AI-generated data. Coined by researchers at Stanford University and Rice University, model autophagy disorder occurs when there isn’t enough fresh data in self-consuming loops, leading to a degradation in the quality and diversity of future loops. This disorder can be seen in anything from text chatbots like ChatGPT to image-based generative models like Midjourney. Repeating the process of training generation after generation of the model exclusively on AI outputs causes a degenerative process where models forget the true data distribution over time.
The AI Ouroboros
In July 2023, just eight months after the launch of ChatGPT, a new study found that ChatGPT’s ability to write code and perform other tasks has gotten significantly worse than when the model was first released. When a team of researchers from Stanford University and the University of California Berkeley tested generated codes from the March and June versions of GPT 3.5 and GPT 4, they found that only 10% of the generated responses of GPT 4 were executable as opposed to the 53% that was executable in March. 2023. GPT 3.5 also went from 22% correct in March to 2% in June.
While it is hard for anyone to tell what is going on under the hood as OpenAI has a notoriously closed-off system, it is obvious that some of the capabilities of the model have degraded over time. This degradation is a good example of what happens when a model begins to eat itself because there is no fresh training data available. While learning with generated data is possible with language models, and models can successfully learn some underlying tasks, there has to be some preservation of the original data to allow for better model performance.
Conclusion
A question to ask at this point is what does it say about the output of these models if training other models exclusively on them can result in their breakdown? We already know that while ChatGPT and other generative AI models have made incredible progress, they are still primarily distinguishable from human outputs. ChatGPT’s output, for example, is still a little too stilted and awkward to pass for human writing. While this might seem like the apparent cause of autophagy, it is more likely that training a model on the output of another model can cause a distribution shift that, over time, causes the model to misperceive the underlying learning task. And as high-quality data becomes scarce, this is going to continue to be a bigger challenge in the creation of generative models.