6 Biggest Challenges of Automatic Speech Recognition (ASR) for Hindi

Dan Shafer
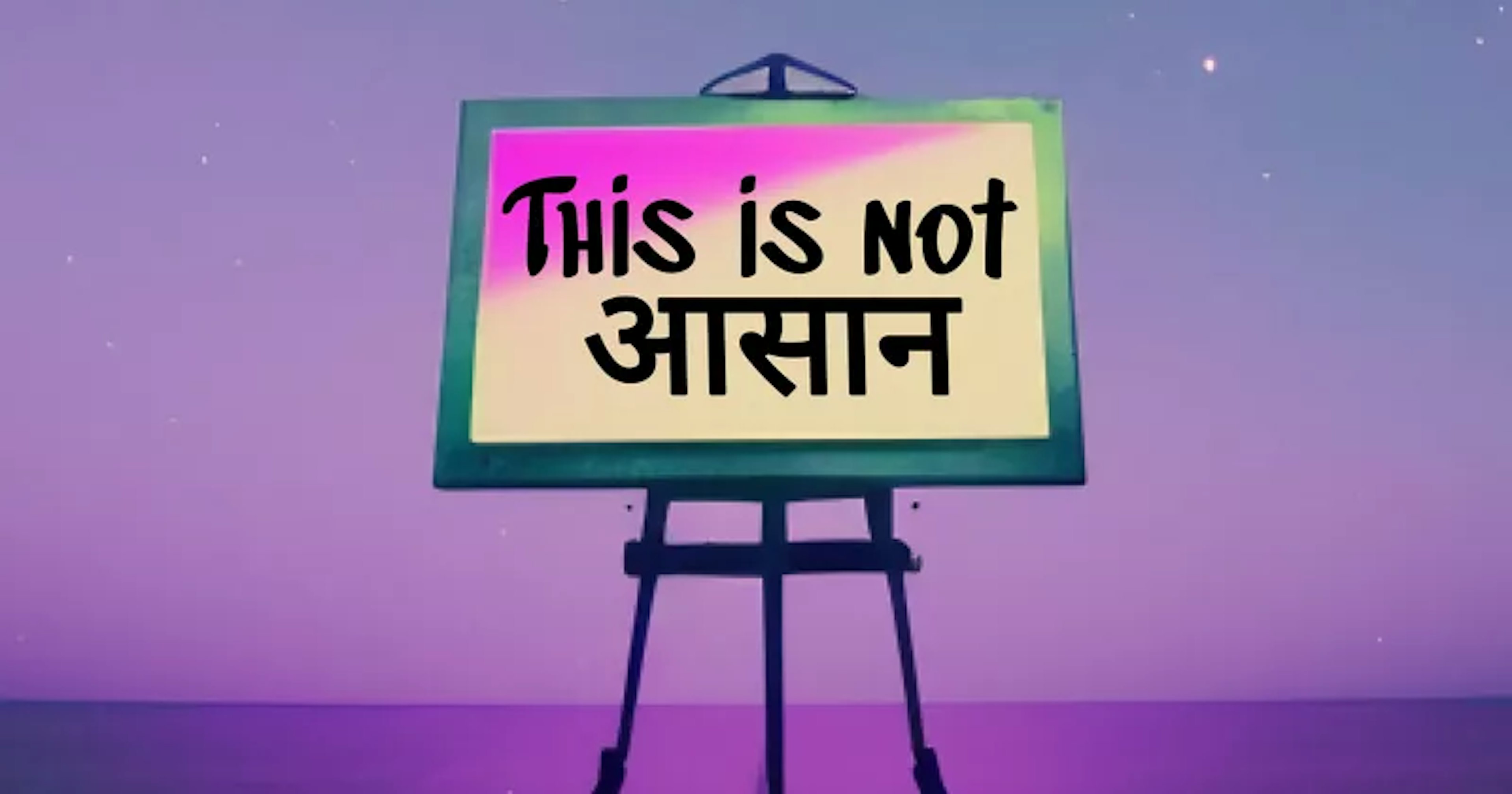
As Deepgram expands the number of languages that we offer automatic speech recognition (ASR) for, we're bound to run into languages that present different challenges than we encountered for English. In this blog post, we'll review six of the biggest challenges that are present for people looking to create a Hindi speech-to-text model. Before we dive into the specifics, let's take a look at what Hindi is and where it's spoken.
What is Hindi?
Hindi is an Indo-European language spoken in the northern part of India, in the so-called Hindi Belt, and is one of the two official languages of the government of India. With some 322 million native speakers with an additional 270 second-language users, Hindi is one of the most widely-spoken languages in the world.
6 Biggest Challenges for Hindi ASR
With that background out of the way, let's look at six ways that Hindi can create challenges for speech-to-text systems.
1. Limited Resources
Perhaps the first challenge that arises when trying to build an ASR model for Hindi is that the language is what's sometimes called a low-resource language. This means that there isn't as much data available for training ASR models as there is for languages like English. For example, the open source Common Voice project, which releases crowd-sourced and crowd-validated utterances for dozens of languages, released a Hindi dataset for the first time at the end of 2020, with a mere half an hour of labeled (validated) audio. That number has since grown to 11 hours. Compare that with 217 validated hours for Tamil (another Indian language) or 2186 for English. Training a robust supervised ASR model typically requires several thousand hours of labeled audio, so the lack of available audio can create real challenges.
2. Multilingualism and Dialects
Because Hindi is a lingua franca in India, maybe people speak it as a second (or even third or fourth) language. This means that, even in conversations that are in Hindi, speakers may be switching between it and other languages, a phenomenon called code switching. This can make it difficult for an ASR model to track what's being said. Even if speakers from other languages aren't code switching, Hindi has a lot of loanwords from other languages. This can make it difficult for ASR to correctly identify the words, since the pronunciation may not follow the usual rules of Hindi. Add on to that the fact that Hindi has several different dialects, and this can again make it difficult for ASR to correctly recognize words, since the same word can be pronounced differently in different dialects.