Understanding Automatic Speech Recognition (ASR) in the Future of Communication
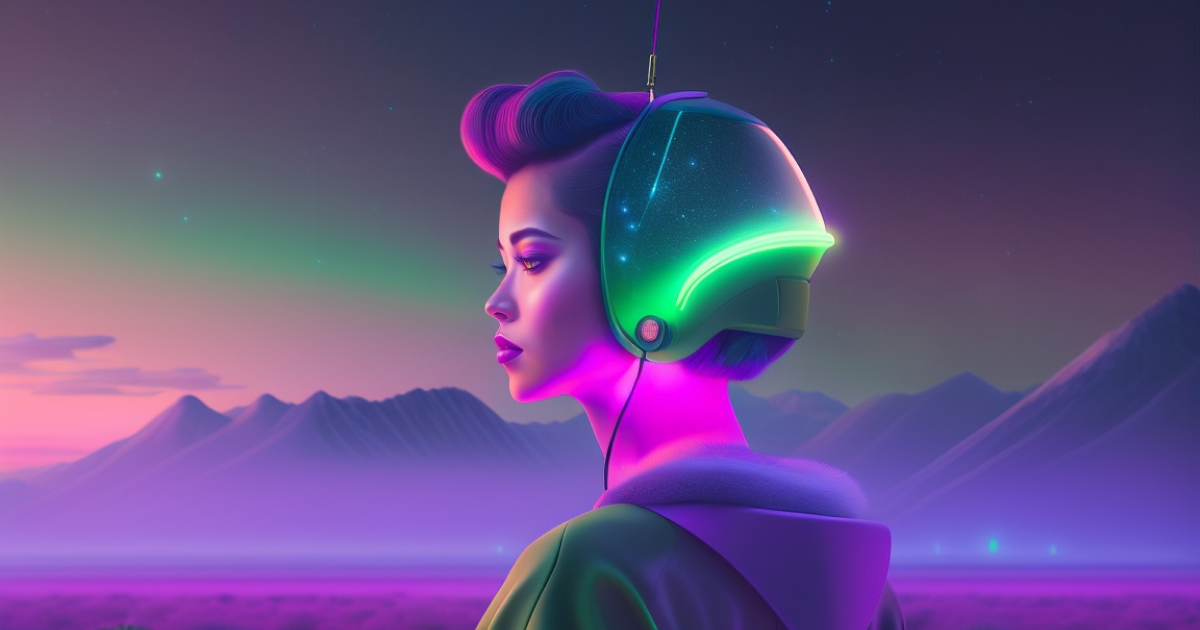
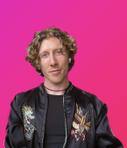
We’re stepping into a world where machines understand us, no longer just by the click of a button or a typed command, but also by simply hearing our voice. Enter the realm of automatic speech recognition (ASR) - a dynamic intersection of voice recognition (VR), machine learning (ML), and natural language processing (NLP). To say the ASR intersection is full of “crossed paths” would be an understatement.
At its core, ASR is the technology that transforms spoken language into written text; and it’s quickly approaching human-like accuracy levels. Despite being a well-understood technology for those in the know, VR remains a treasure trove of untapped potential, with many businesses still not transcribing all of their audio data for use cases. We can’t place blame on affordability or accessibility anymore with so many model options on the market today. So why is it continuing to be underutilized?
We are leaning towards the reality that maybe everyone still needs to be made aware of its full capabilities.
Whether you’re a curious beginner eager to understand the magic behind Siri and Alexa or an experienced ASR practitioner looking to demystify anything in your ASR directory, this guide to the future of communication is for you, from start to finish.
Join us as we delve into the enlightening history, inner workings, present application, and future of ASR.
History and evolution of ASR
The history of automatic speech recognition is a story of human ambition, technical evolution, and the relentless pursuit of replicating one of our most innate abilities: speech.
Let's take a stroll down memory lane and see how ASR has transformed over the years.
Our journey began in 1952 when Bell Labs introduced the world to “Audrey” (not Hepburn), the first-ever ASR system. Audrey was a simple digit recognizer, capable of understanding spoken numbers. Rudimentary by today’s standards, Audrey was a groundbreaking invention for her time, setting the stage for the future of voice recognition. By 1962, researchers made great strides in enhancing Audrey’s ability to recognize essential spoken words, such as “hello.” Imagine the thrill of a machine recognizing a casual greeting for the first time!
The origins of ASR weren’t limited to civilian applications.
The Cold War era saw significant research into ASR for military purposes. While the broader public remained largely unaware, ASR was slowly gaining ground behind closed doors. Thanks to government-funded projects like the Wall Street Journal speech dataset, the '90s researchers made significant progress, some would say pivotal. Machine learning and natural language processing began playing crucial roles, ensuring that VR was, not just accurate but also, contextually relevant.
By 2014, Baidu’s deep learning research catapulted ASR to new heights. Propelling automatic speech recognition’s accuracy and accessibility to unprecedented levels. The integration of ML and NLP with ASR systems meant that these technologies could now understand speech with nuances, emotions, and context.
Today, we’re at the pinnacle of ASR technology. We’re witnessing ASR models that are not only incredibly accurate but also affordable and fast. The advancements are so profound that today’s ASR systems, such as Deepgram Nova, can reduce word error rates by a staggering 22% and offer inference times up to 78x faster than the model's predecessors.
ASR has come a long way, from the basic digit recognition of Audrey to the sophisticated deep learning-powered systems of today. One thing is clear: the story of ASR is just getting started, and the best chapters have yet to be written.
Well, what has been written? How ASR works today.
How ASR works
Let’s dive right into the intricacies of ASR, the role of machine learning and natural language processing, and the challenges faced in this fascinating domain.
Automatic Speech Recognition: The Basics
At its core, ASR is about converting spoken language into written text. It's the technology that powers voice assistants, transcription services, and many other applications we interact with daily.
The Role of Machine Learning in ASR
ML is the backbone of modern ASR systems. Here's why:
Acoustic Modeling: ML algorithms are trained on vast datasets containing diverse speech samples. This helps the system recognize phonemes, the most minor sound units, in any given speech input.
Continuous Learning: The more data an ASR system processes, the better it gets. ML allows ASR systems to learn from their mistakes and continuously improve their accuracy.
Natural Language Processing: Making Sense of Speech
While ML helps recognize sounds, natural language processing ensures that transcribed text makes sense. NLP is all about understanding context, grammar, and the nuances of human language. It's what ensures "I need to eat" isn't transcribed as "I knead to eat." (Apologies for anyone's hangry being triggered by that example.)
The Speech-to-Text Process: A Step-by-Step Breakdown
Let's dissect the journey from spoken words to written text:
Signal Acquisition: It all starts with capturing the spoken words, typically through a microphone.
Preprocessing: The raw audio is cleaned to remove any background noise.
Feature Extraction: The cleaned audio is then broken down into distinct features representing different sound patterns.
Acoustic Modeling: The system matches the features to known phonemes using ML.
Language Modeling: NLP comes into play here, predicting the likelihood of a sequence of words and ensuring the transcribed text is coherent.
Decoding: The system constructs the final output, converting the recognized phonemes into words and sentences.
Post-Processing: This step involves refining the transcribed text, adding punctuation, and making any necessary corrections.
Challenges in ASR: It's Not Always Smooth Sailing (le sigh)
Despite the advancements, ASR systems still face challenges:
Accents and Dialects: How a word is pronounced in Spain can be vastly different from its pronunciation in Argentina or Mexico. Catering to the myriad accents and dialects globally is a tall order.
Noise: Background noises, like traffic or other people talking, can interfere with the system's ability to transcribe speech accurately.
Speaker Variability: Factors like the speaker's mood, health, or age can affect speech patterns, making the recognition process more complex.
Automatic speech recognition is full of challenges and opportunities. As we continue to refine and advance ASR systems, the dream of flawless voice-to-text conversion inches ever closer to reality. One application at a time.
Applications of ASR
ASR applications are seamlessly integrating into modern life. Many may need to realize how much this tool is reshaping our daily interactions and professional landscape. We’ll start with a typical automatic speech recognition application example to get the juices flowing.
Take a moment and think about the last time you asked Siri about the weather or told Alexa to play your favorite song. Who doesn’t love putting on their favorite song effortlessly? These voice assistants have become integral parts of our daily routines, and we owe their responsiveness to ASR. Beyond these intelligent assistants, our smartphones are also harnessing the power of automatic speech recognition.
Whether it's voice typing a message to a friend or asking for directions, this powerful technology ensures our devices understand and act on our commands.
A few other applications of ASR worth mentioning include:
A Lifeline for the Hearing-Impaired
ASR's impact goes beyond convenience; it's a tool of empowerment. ASR offers a bridge to the world of sound for the hearing-impaired community. Real-time speech captioning can transform experiences, from attending lectures to enjoying movies. By converting spoken words into text, ASR ensures that content remains accessible, breaking down barriers and fostering inclusivity.
The Transcription Titans
In the professional realm, ASR is revolutionizing documentation. Medical professionals, for instance, can dictate their observations during a patient's check-up, and by the time they're done, a complete report is ready, thanks to ASR. Similarly, in the legal world, ASR ensures that every word spoken in courtrooms, client meetings, and consultations is captured with precision. This accuracy is invaluable in sectors where every word can carry significant weight.
Revolutionizing Telecommunication and Customer Service
The telecommunication and customer service industries are also reaping the benefits of ASR. Remember the days of navigating through endless automated menus when calling a helpline? Yes, and cringe. Those days are numbered. With ASR-powered interactive voice response systems, customers can simply state their concerns and be directed to the correct department.
Moreover, businesses are leveraging ASR to transcribe and analyze customer calls, gleaning insights that can drive service improvements. And let's remember the chatbots and virtual agents that provide round-the-clock support. These bots can understand and address user queries in real-time thanks to ASR, enhancing customer experience.
In the Opus Research and Deepgram State of Voice 2023 report, voice technology’s impact was weighed against its current abilities. Naming customer experience analysis is the most transformative use case, with 14%. Do you agree with the results? Let us know in the comments and if there are any use cases you feel should have made it into this graph.
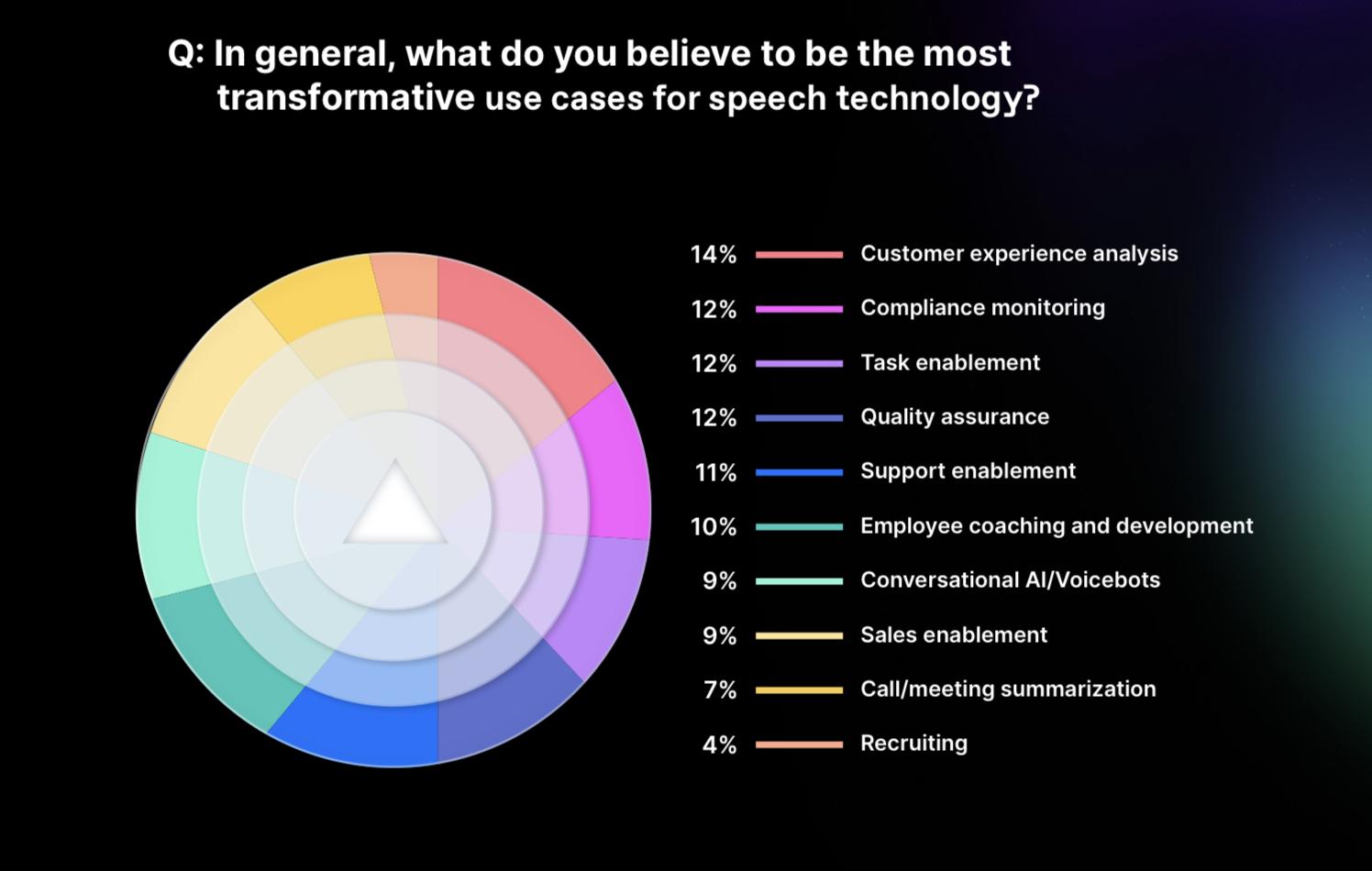
Opus Research and Deepgram State of Voice 2023 report.
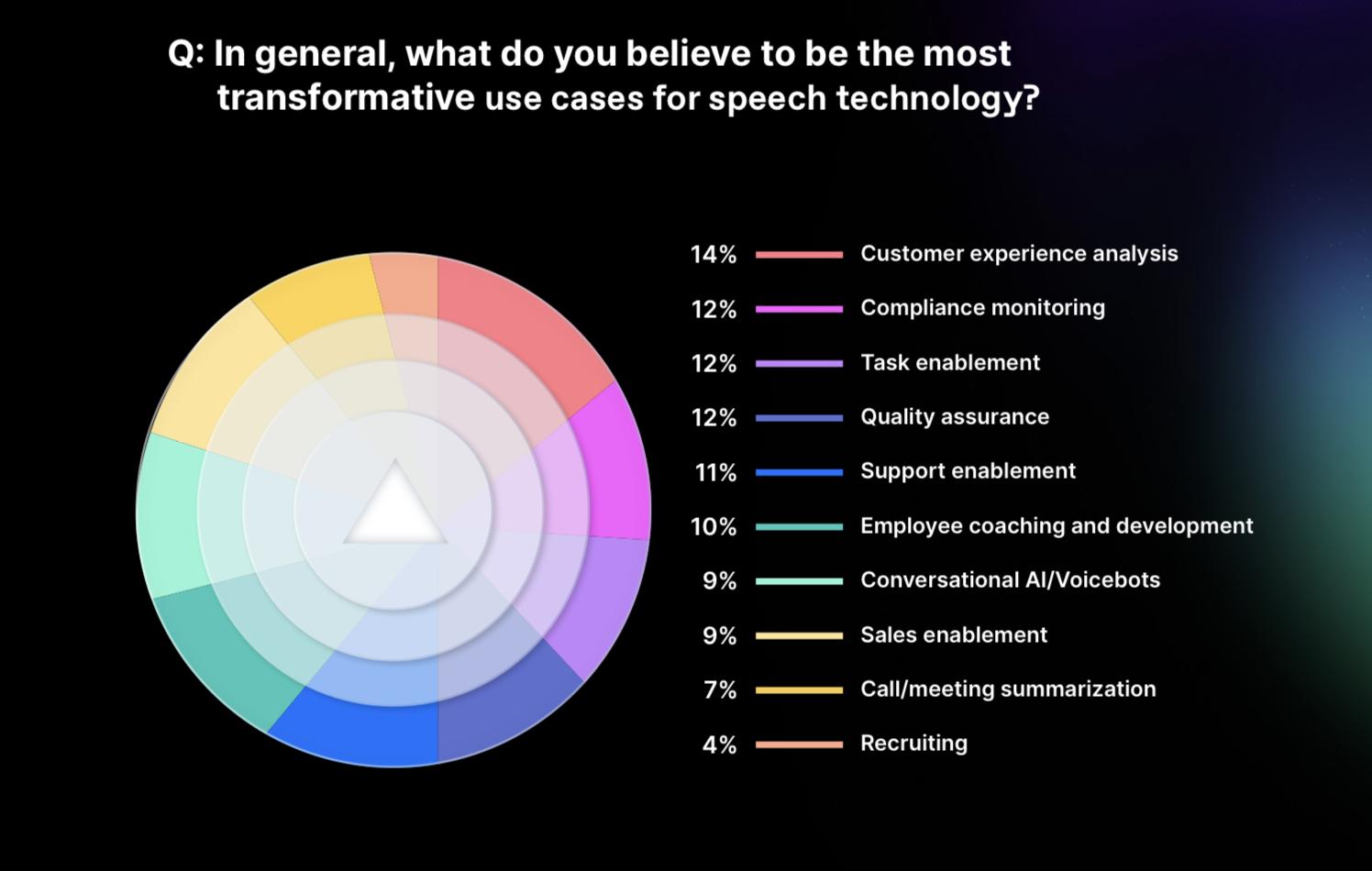
Opus Research and Deepgram State of Voice 2023 report.
What we can all agree on is that applications of ASR are vast and varied. ASR is making its mark from our personal devices to the customer service desks of global corporations. As technology continues to evolve, we can only anticipate even more innovative uses for automatic speech recognition, further integrating it into our daily lives and professional endeavors.
But what does the future actually look like?
The Future of ASR
Imagination time. Picture a world where your devices not only hear you, but truly understand you from the nuances of your tone to the sentiment behind your words (if you thought of MEGAN, no, you didn’t) and even the context of your conversations.
Rest assured, this is the future of automatic speech recognition.
Recent surveys from The State of Voice 2023 report suggests this isn't just a fleeting trend. A whopping 72% of respondents believe voice-enabled experiences will become mainstream in just a few years. 54% of respondents think voice bots will reach human-like levels of interaction in one to three years.
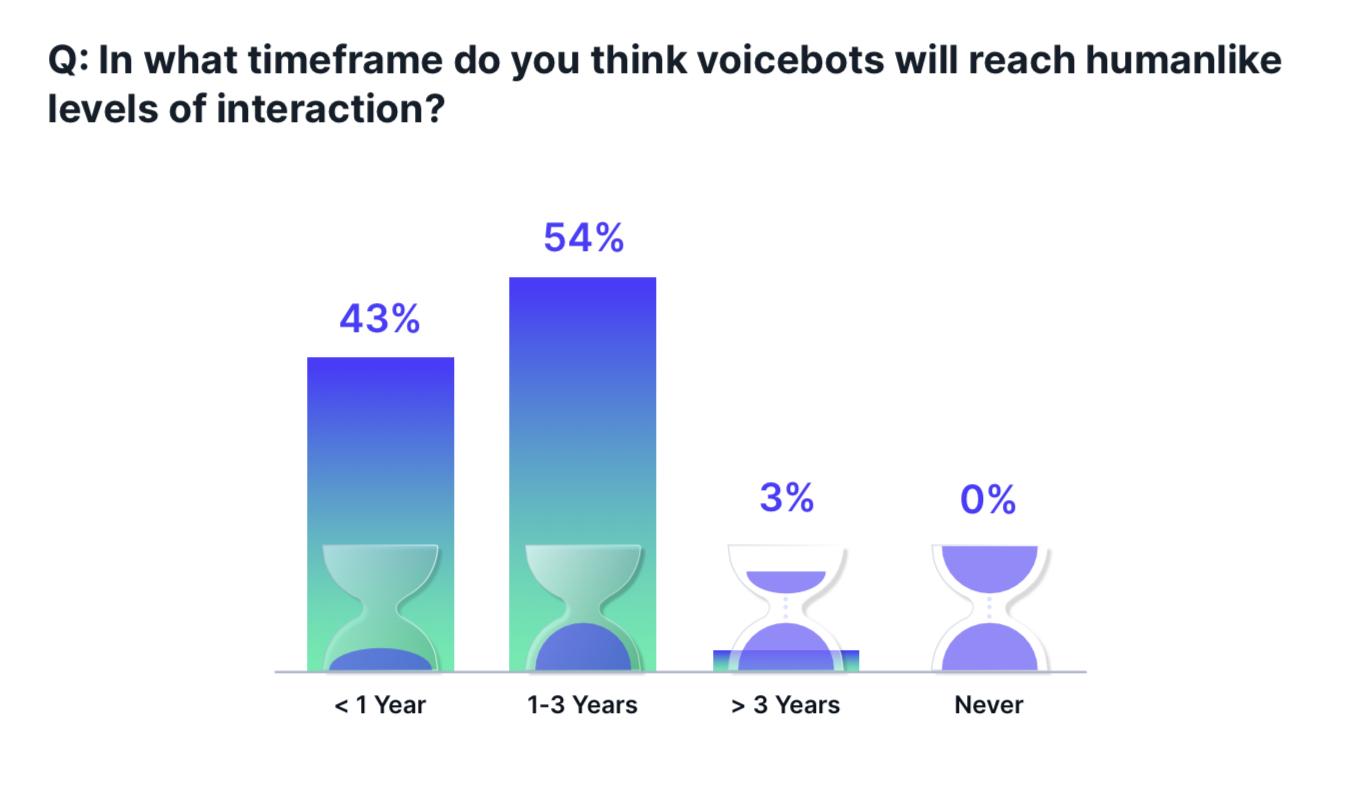
Recent surveys from The State of Voice 2023 report.
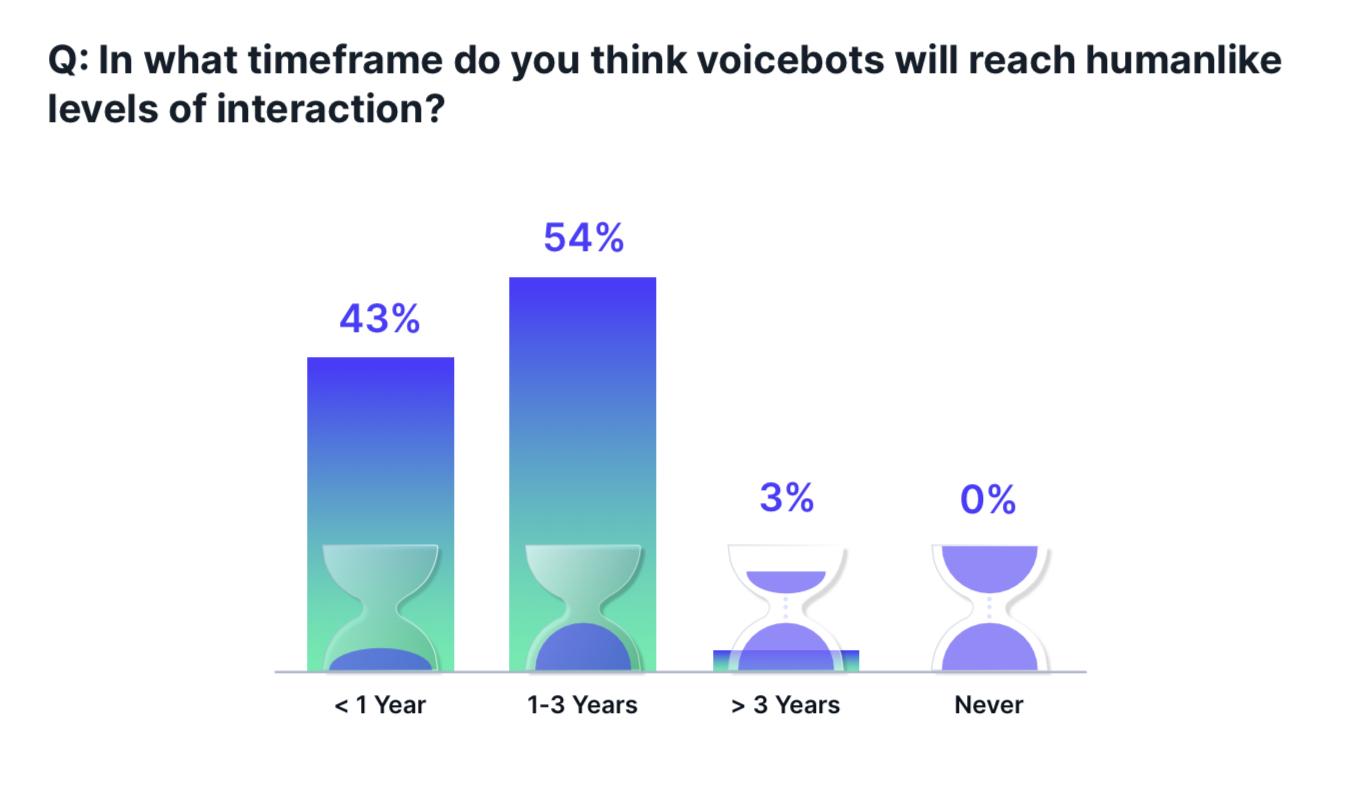
Recent surveys from The State of Voice 2023 report.
But what's driving this rapid adoption?
Companies like ours are at the forefront, championing the belief that language is the golden key to AI's vast potential. We envision a future where natural language isn't just a feature but the foundation of our interaction with technology. Cue Super Mario’s Powerup sound effects.
The tech world is buzzing with innovations, from domain-specific language models that offer unparalleled accuracy to new methodologies that promise to make ASR more efficient and cost-effective.
So, as we stand on the cusp of this exciting new era, one thing is clear: The relationship between humans and machines is about to get a lot more conversational. And in this symphony of voices and technology, ASR will be the maestro, orchestrating a future where communication barriers are a thing of the past. The future is not just about being heard; it's about being understood.
Conclusion
As we unraveled automatic speech recognition’s transformative journey, from its inception with "Audrey", to today's sophisticated systems that convert spoken language into text with near-human accuracy, we hope we were able to demystify any previous confusion, if there was any, for our seasoned pros and ASR newcomers.
Now, let's play a game (that was for all of you horror film lovers). Name ASR’s future era in the comments below. Is automatic speech recognition given in Requiem by Mozart or Renaissance by the Beyonce era? Have fun, until next time.
Unlock language AI at scale with an API call.
Get conversational intelligence with transcription and understanding on the world's best speech AI platform.