Voice Tech is Growing. How can that Happen Equitably?
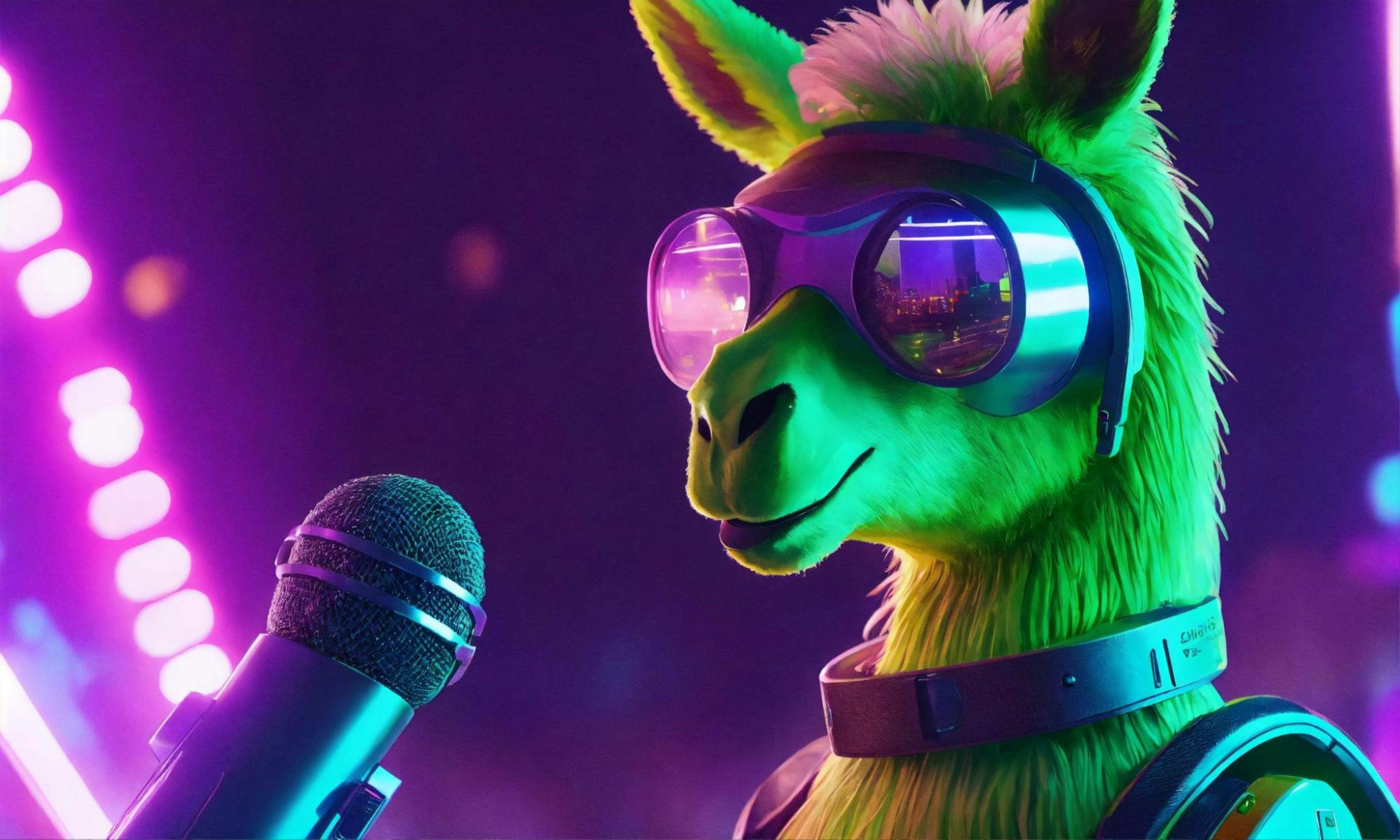
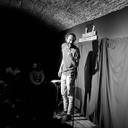
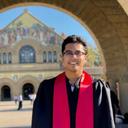
Voice Tech (and NLP at large) was for engineers. Then linguists started to get a say on the matter. And now, we’re reaching a point where it’s free for the taking. The gift of abstraction is its own biggest flaw: you don’t need to know how the abstracted idea works. For example, Alan Turing didn’t need to speak any German to crack the Nazi’s enigma code in WWII. Likewise, the engineers who developed the chess-playing Stockfish—an AI engine that has defeated multiple generations of World Champions—are nowhere near grandmaster status.
Abstraction works pretty well in most situations, but I wanted to take this opportunity to talk about some of the gaps. This article is going to discuss what—or better yet who—may suffer certain oversights in ASR practices.
This article won’t get super technical, and probably won’t be a great reference for your next paper on ethics in tech. So why am I writing this? Well, if you have access to the latest AI technologies, it’s important to engage in the equality discourse. So long as we’re reaching the point that these machine learning resources are becoming accessible for those outside the closed doors of academic environments, so too should the discourses around their responsible use.
I won’t get super into the statistics. I simply want this article to be a primer on some of the concepts and terminology surrounding the topic. Talking about inclusion can be a little intimidating. It gets even trickier when you get into the sociolinguistics of it: maybe you’ve never been introduced to the idea of dialects and sociolects, for instance. Nonetheless, I want this article to be a welcoming ice-breaker for tech enthusiasts who want to dip their toes into the world of ethics.
This article will generalize to non-standard varieties/dialects/ways of speaking across languages. That being said, what’s true for my examples may not be true for every example. Often, analyzing different ethical scenarios on a case-by-case basis is necessary. If you believe I got something wrong, please let me know. The whole point of this piece is to take a moment to consider perspectives that might tend to go unheard.
Second: I’m careful about my terminology here. You’ll see the word variety used rather liberally in this work. I’ve chosen that term, because it feels just a little less rigid than dialect. I’m deliberate about communicating my claims to all readers, regardless of demographics, educational background, or language of origin.
Finally, I’m deliberately choosing the term Voice Technology for its broadness. We know it can refer to a lot of things, from speech-to-text (STT) to deepfake detection. I use the term Voice Technology as an umbrella for all of these innovations as a whole.
Lots of STT applications are for broad consumption. The motivation for standardized language is pretty clear here: the message needs to be understood by as many people as possible. That’s where standardized language tends to shine. It’s not only a way that people try to talk, but it’s the best-resourced entry point to discourse.
What do I mean? Well, in general, people educated in this standardized language can understand it, which means that the same message can reach people across a wide geographical and sociological range of people. It can also go overlooked how useful standardized language is for those who have essentially unlimited access to it. For example, Google Translate is incredibly easy to use for standard orthography in well-resourced languages, and remarkably worse otherwise.
But the less positive by-product of favoring language standards is that the technologies will tend to favor those who are better represented in the standard. So that begs the question: Who exactly is “better represented”?
Standard language tends to be based on the language of a generally privileged slice of society—the slice that develops advanced technology. As a result, funding and resources are allocated towards technological advancements that benefit an already advantaged population, further exacerbating social inequities. That means this all-encompassing, widely-accessible “code” we call a language standard just so happens to be more or less the everyday language for this lucky echelon. Looking at it through this lens, the idea of educating societies on standard language goes from being a matter of “can you communicate effectively to make yourself understood?” to “can you learn to talk the way we do to earn your seat at the table?”
Machine learning, which is largely the basis of Voice Technology, relies on generalization. So that begs the question: What do machine learning models generalize? Well the answer is that these models generalize patterns in high-resource languages—languages like English, Mandarin, Spanish, and French. Meanwhile, African languages, Native American languages, and Scandinavian languages lack sufficient amounts of data to be modeled by AI. Or, at least, these low-resource languages can’t be modeled as accurately as their high-resource counterparts.
As a result, machine learning models are biased towards “standardized” language because the data these models learn from is all written in standardized language—thereby obfuscating low-resource languages and, by extent, the people who speak them.
Let’s walk through an example. The word “though” has a weird spelling, but it’s used a lot, and it’s always spelled the same. Getting the same accuracy for less represented expressions and pronunciations means having enough consistently labeled data. And by definition, a low-resource language lacks that consistently labeled data. Consequently, we’re forcing large populations of people to learn a high-resource language if they want equitable access to the latest AI technology.
Can we combat the disadvantages of being non-standard by rebranding the group of low-resource languages as its own, independent group with its own, independent standard?
Maybe.
Best case scenario, some variety gets enough consistently labeled data to have not only its own standard orthography, but the institutions supporting it so that further resources can more easily be made available in that variety. That, very generally speaking, is when we go from colloquially calling something a dialect or accent to a language. And that’s great– for that variety. But, firstly, until that point is reached, spelling something differently, i.e. non-standardly can look as much like an act of caricaturization than one of representation.
Part of the reason this is so tricky is that dialectology is dialectic: a matter of many moving parts. We can do our best to splinter language dialectics into as many different standardized varieties as possible, but:
The volume of dialects is so large that such splintering becomes outrageously unrealistic, and
Rewriting the standard may actually serve to further exaggerate differences and limit the availability of resources across different communities; the opposite of our goal.
When you think about it, standard isn’t necessarily something categorical.
So that begs the question: Why not just standardize the non-standard?
Well, languages and dialects have fuzzy boundaries, and capturing those boundaries goes back to the argument above: There’s some obvious advantage to having a single standard, but no elegant or equitable way of going about choosing it, or where the boundary lies between varieties that do or do not fall under the umbrella of that standard. The politics of language-versus-dialect debates is messy.
Overall, the points in this article are inconclusive. They’re not solutions, because these problems are hitherto unsolved. After all, isn’t that how societal advancement is? Exciting and unjust?
Take this as an encouragement to keep your eyes peeled for skewed representation, be it in a faulty YouTube auto-captioning or the limited variation in speech data in a low-resource language.
That being said, the state of affairs isn’t completely somber. As the field grows, there are an increasing number of voices holding language technology and machine learning as a whole accountable for bettering their practices.
Have a look at Jordan Harrod and Rachael Tatman's YouTube Channels for discussions that range from technical and practical to social discussions on the topic.
Loads of work is being done right now to assist people affected by dysarthria. These include data collection efforts and building speech synthesis models, to name a few.
There’s always new research ensuring the quality of ASR on marginalized varieties. To name a few, psychological impacts of poor performance of ASR systems on African American users and appraising racial disparities in data, along with proposals for better data collection practice
Here’s an interesting article on the poor representation of LGBTQ+ identity in AI systems.
Unlock language AI at scale with an API call.
Get conversational intelligence with transcription and understanding on the world's best speech AI platform.