Money doesn't grow on trees: The Environmental Cost of AI
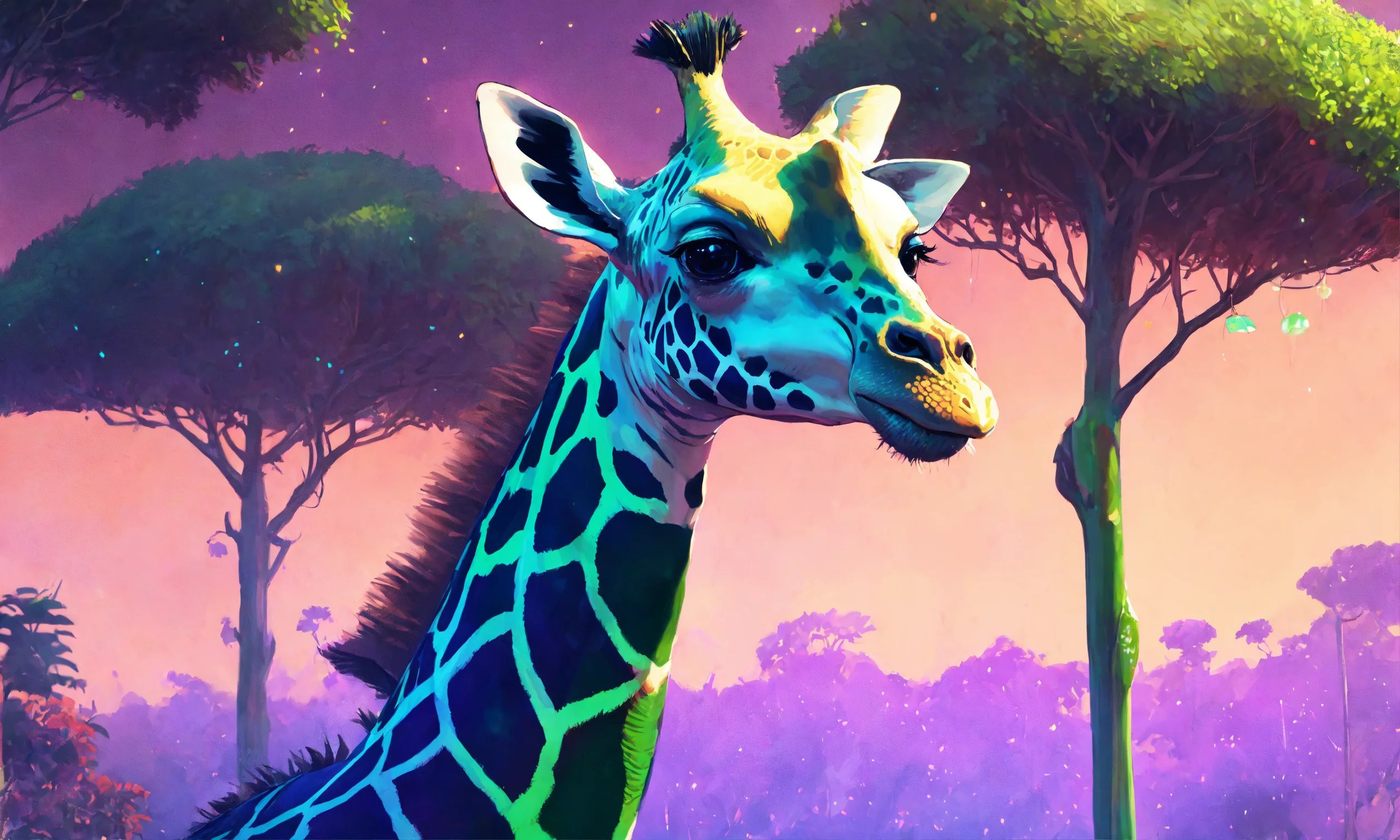
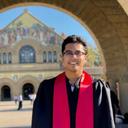
There's quite a lot of discussion on the financial cost of AI, but what about the environmental cost? Well a few companies and universities have crunched the numbers, and here's what they have to say.
Note: Much of the content here is duplicated from the AI Minds newsletter, edition number six.
🌲The environmental cost of inference
First thing’s first: How much energy does it take to run an AI? Well, an Oregon State University paper mentions that, on an individual level, it depends on your GPU+CPU setup. The graphic below delves into further detail:
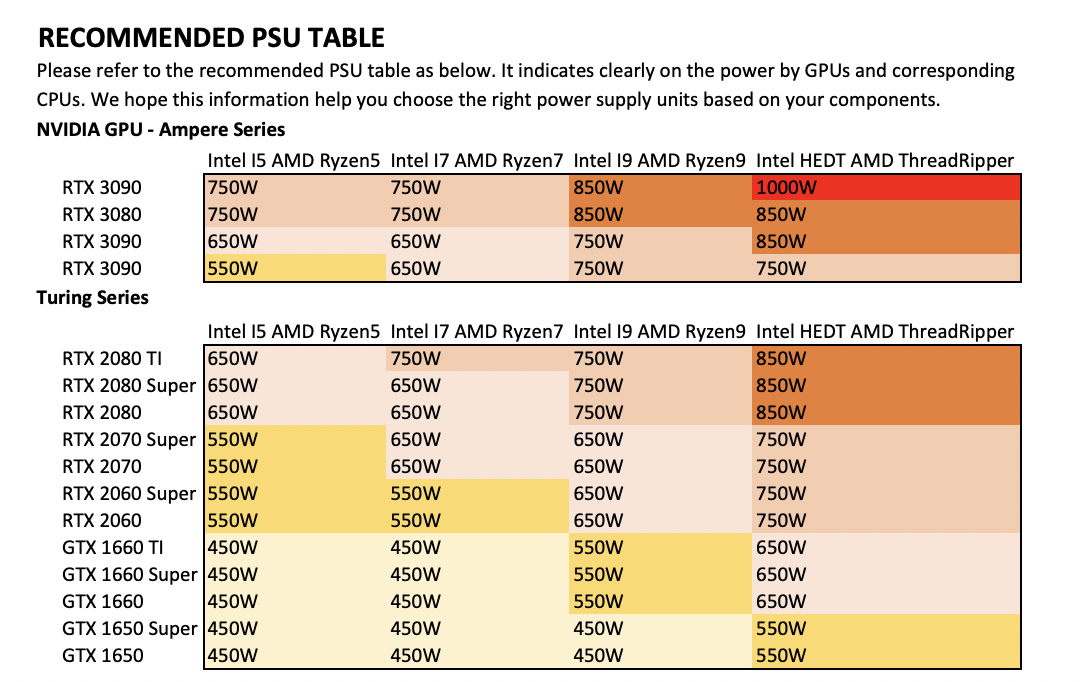
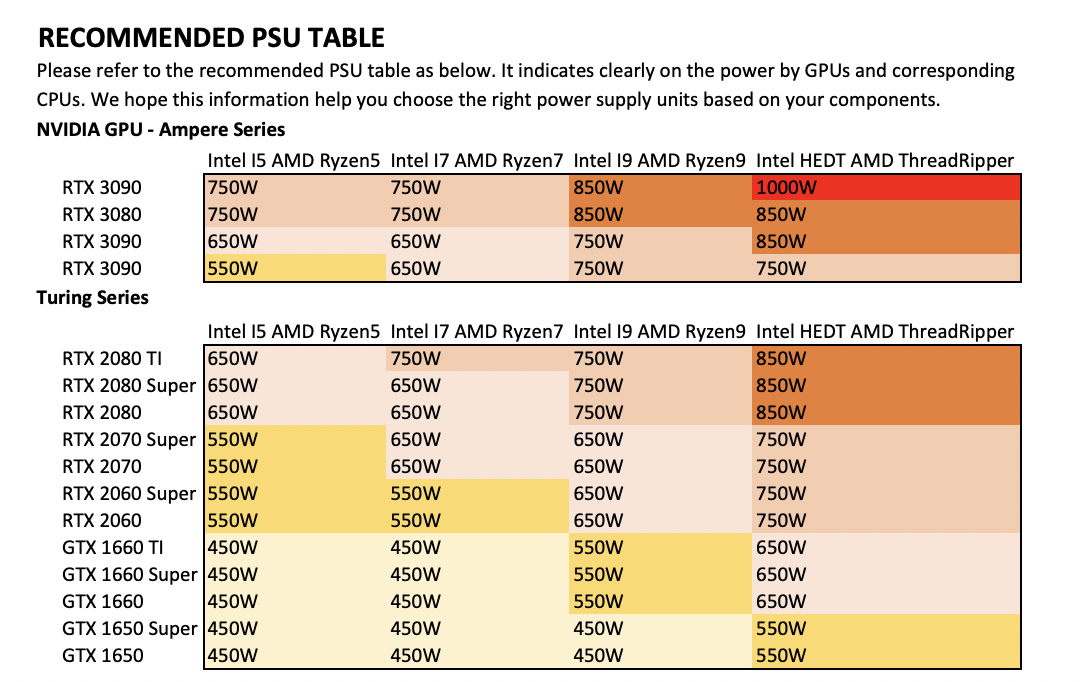
However, that’s merely the amount of energy used once you have an AI ready-to-go. The bigger question is this: How much energy does it take to train a model in the first place? Do Large Language Models come with large environmental costs?
Researchers from University of Massachusetts Amherst reveal the amount of kiloWatt hours needed to train language models from BERT to GPT, alongside the amount of carbon dioxide (and carbon dioxide equivalent) emitted. Check out the full paper here.
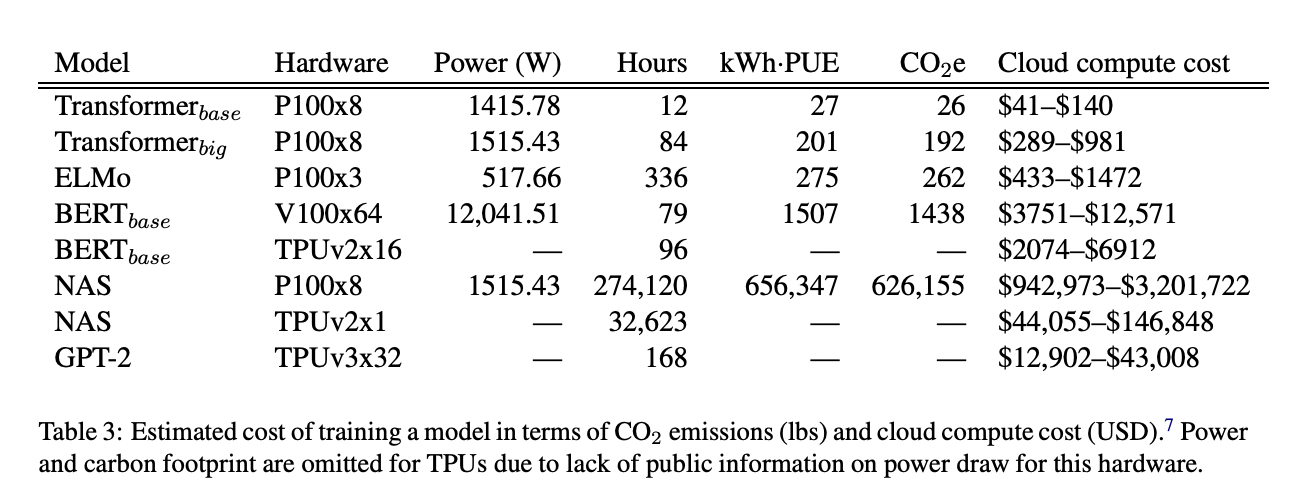
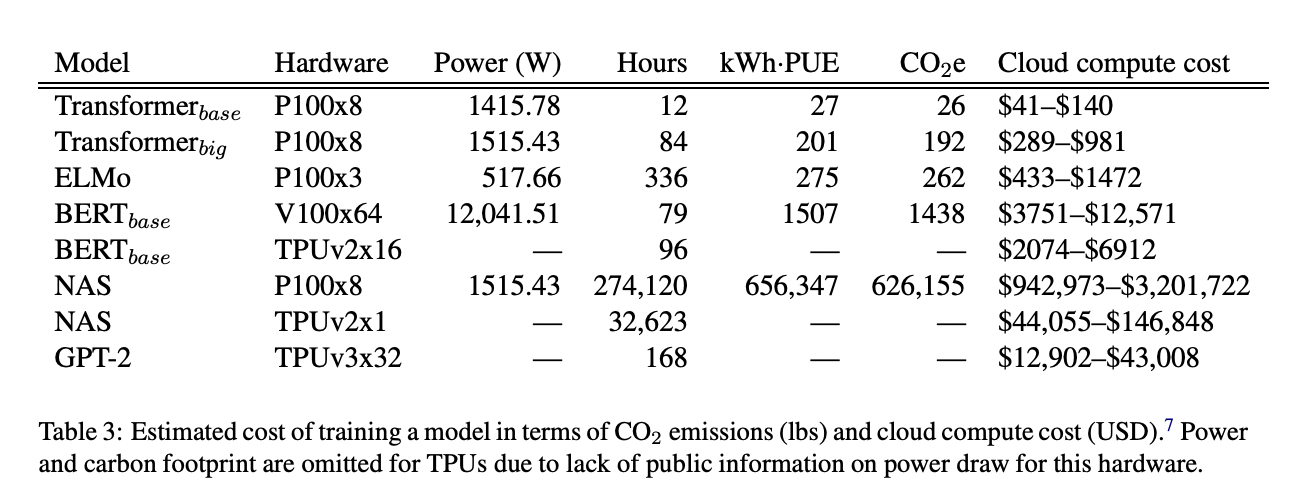
And, of course, as compute usage increases, so does the amount of electricity needed to conduct those calculations. (We will talk about this later.)
As a result, the price continues to increase as well:
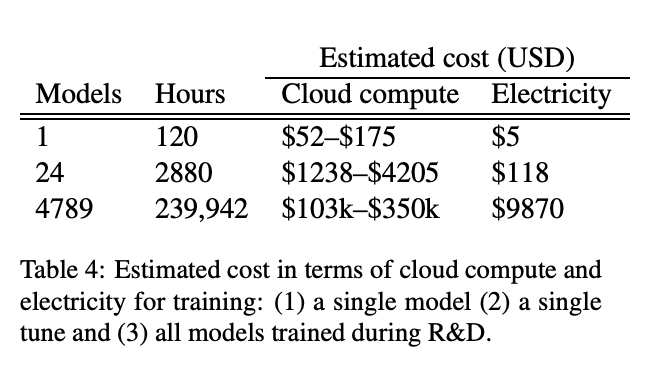
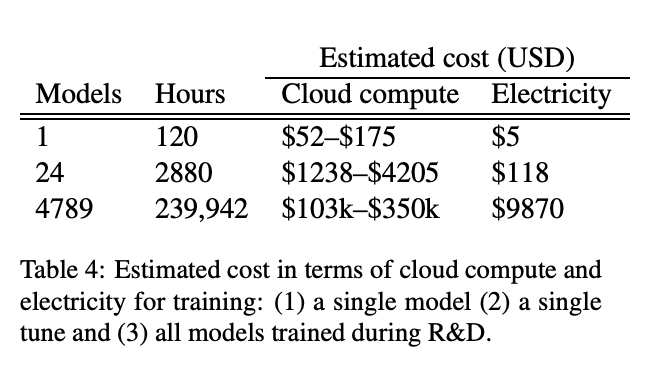
🌴The environmental cost of training
That being said, companies are quick to the draw when it comes to combating claims of environmental negligence. For example, Meta argues in their 78-page paper on Llama-2:
100% of the emissions are directly offset by Meta’s sustainability program, and because we are openly releasing these models, the pretraining costs do not need to be incurred by others.
That is, they’re basically saying: Although training Llama-2 caused 539 tons of Carbon Dioxide equivalent (CO2eq) into the atmosphere, Meta’s internal environmental efforts compensated for those emissions; thus, Meta is helping the environment more than hurting it.
To put things into perspective, it takes about 2 metric tons of CO2eq to fly a plane for an hour. That means training Llama-2 emitted just as much carbon as 269.5 straight hours of flying. Or, to make things more concrete, that's equal to flying from Los Angeles to Tokyo and back 10 times.
Is that a lot? Depends on whom you ask.
On one hand, that's quite a lot of flying. On the other hand, when looking at the airline industry as a whole, Llama-2 emissions are a drop in the ocean.
What a tolerable amount of carbon emissions specifically amounts to depends on what business leaders, politicians, and environmental scientists decide.
(And by the way, we read and did a breakdown of the entire 78-page Llama 2 paper. Check it out here. But you should totally read the original paper, though. It’s great.)
🍃 The end-to-end environmental cost analysis
Furthermore, in an analysis of Meta, a publication from MLSys 2022 notes that the breakdown of power capacity used in AI is 10:20:70 distributed over Experimentation, Training, and Inference, respectively. Furthermore, the energy footprint of the end-to-end machine learning pipeline is 31:29:40 over Data, Experimentation/Training, and Inference, respectively.
Basically, the carbon footprint of machine learning is non-zero, but also not as large as other industries. Of all the technological and innovative domains that produce a significant carbon footprint, machine learning is one that is relatively easy to offset—as Meta demonstrated with Llama-2 above.
Ultimately, these researchers hope that “the key messages and insights presented in this paper can inspire the community to advance the field of AI in an environmentally-responsible manner.”
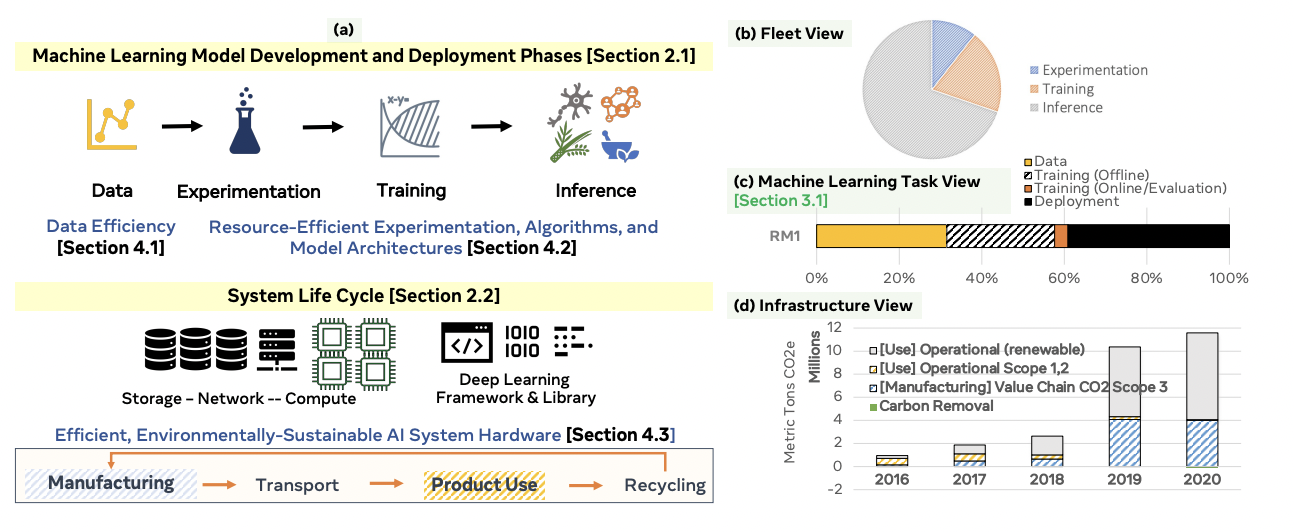
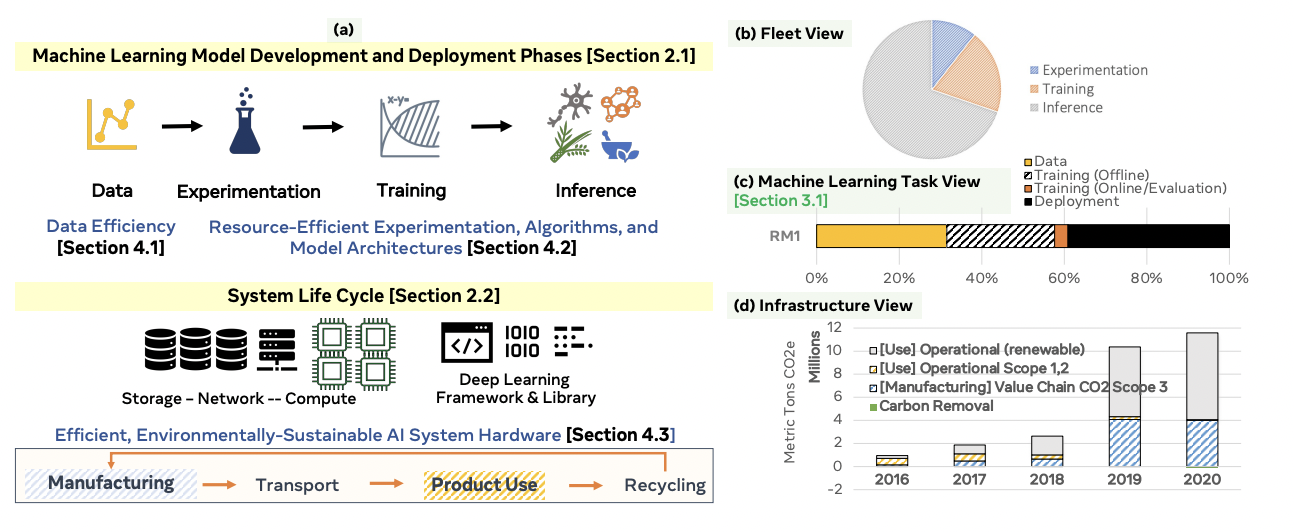
Whether those hopes will be fulfilled is a question yet to be addressed. In fact, a recent paper—which has picked up attention from reporters at Bloomberg and Fox—reveals that the AI industry might use more electricity than some small countries.
The graphic below illustrates just how much more energy-expensive AI is compared to a simple Google search. And if chatbots scale to become as consistently used as their search engine counterparts, we can only guess just how much the industry will spend on electricity in the upcoming years.
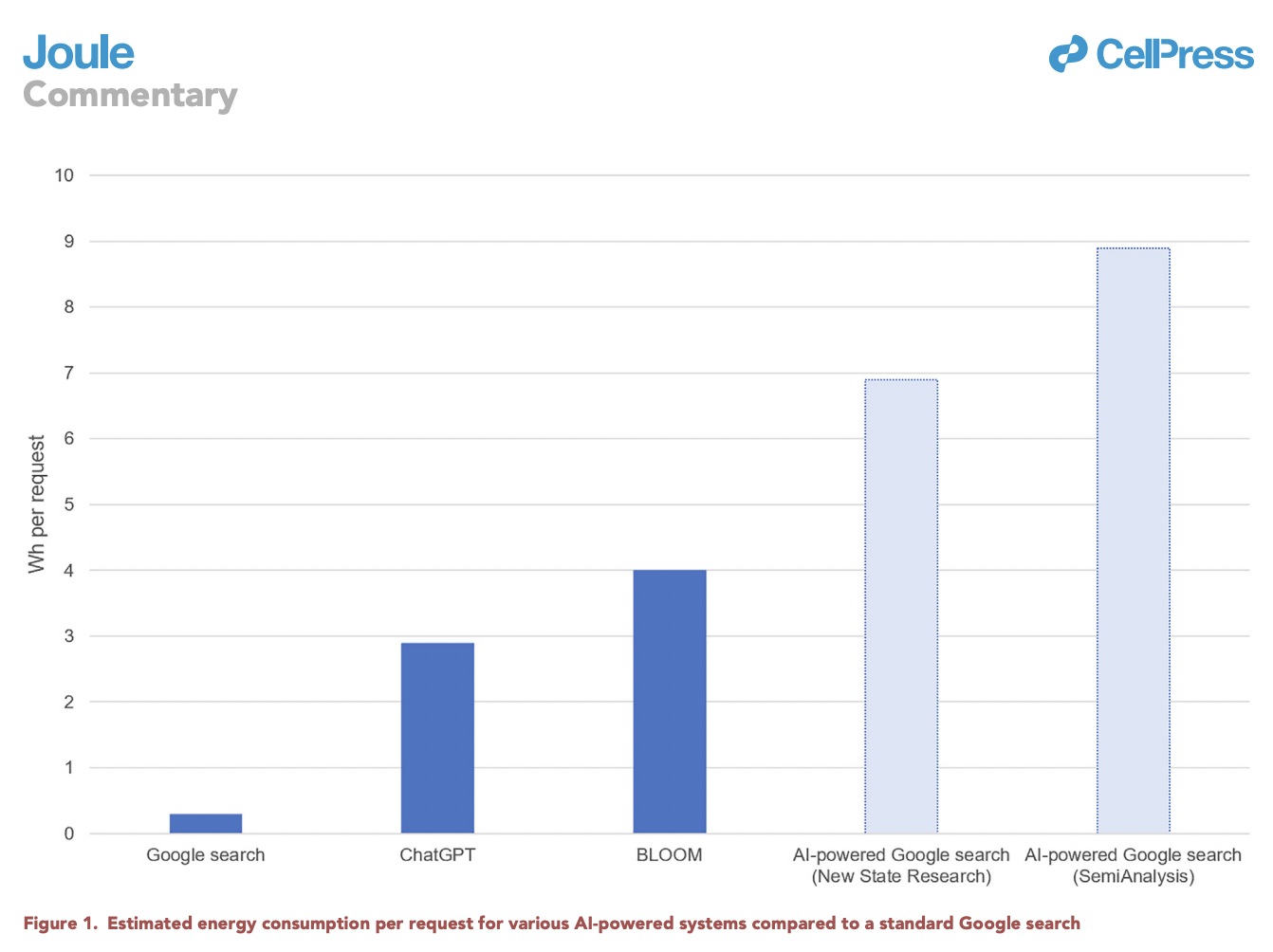
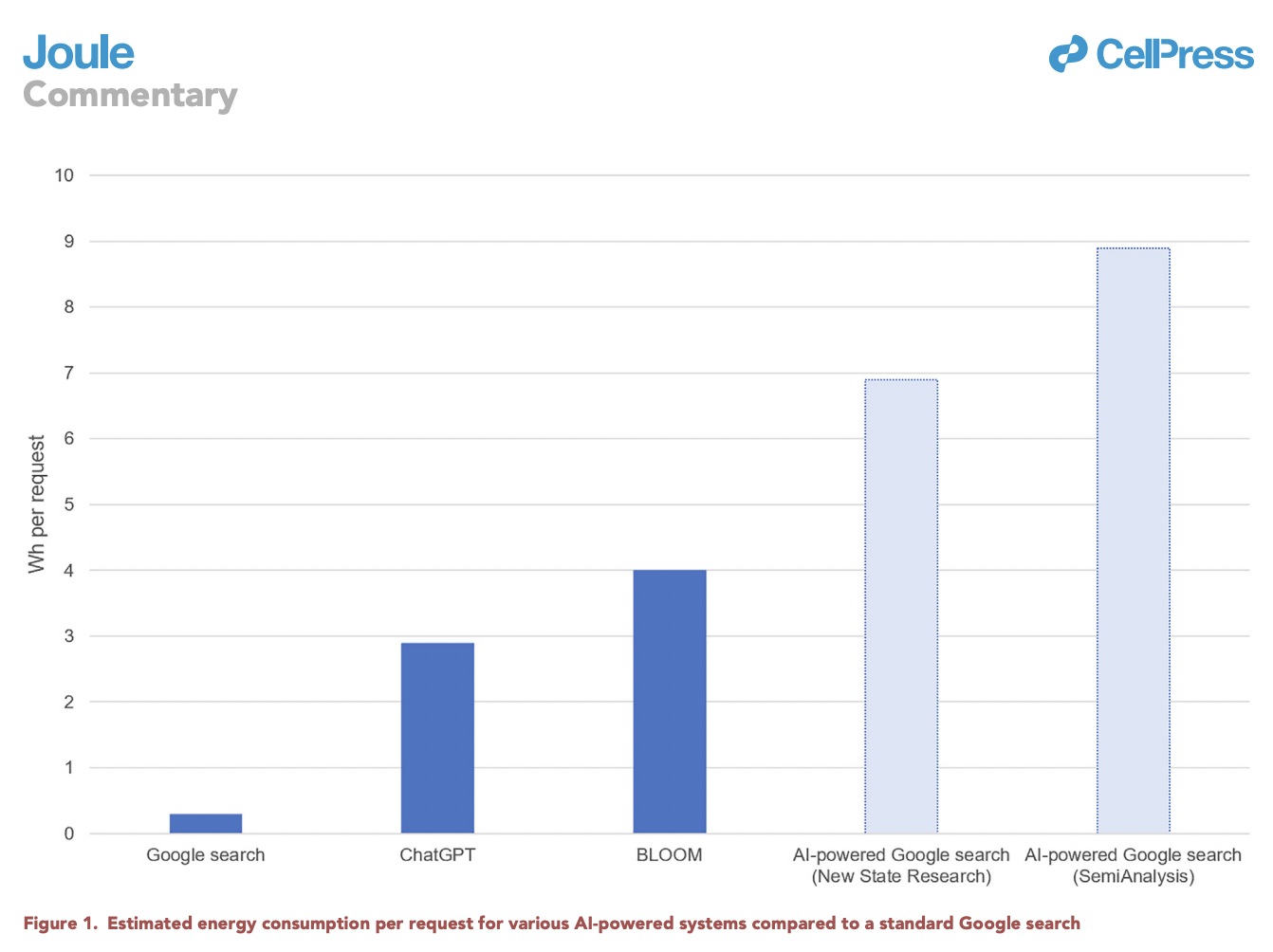
Luckily, companies like HuggingFace are indeed making efforts to help the environment while also furthering technological progress. Sasha Luccioni, PhD, is the AI Researcher and Climate Lead at HuggingFace, whose job revolves around evaluating the environmental and societal impacts of AI models and datasets.
She and her team have made tremendous efforts to ensure that the interests of AI innovators and environmentalists alike, though sometimes at odds, never interfere or hinder one another. Check out Luccioni's Twitter page here.
For additional resources on AI's impact to the environment, check out the following articles:
Uncovering the Carbon Cost of Machine Learning, by Tife Sanusi.
Artificial Intelligence's Environmental Costs and Promise by the Council on Foreign Relations
How to Make Generative AI Greener, by Harvard Business Review
💸 One final point…
One fact that often goes overlooked is that as environmental costs increase, so do financial costs. Yes, yes it's cheaper to use a gasoline fueled car than an electric one. However, in the AI space, notice that as compute increases so does electricity usage. And, as anyone who has ever paid an electric bill can attest, electricity usage drives up cost.
And if we're distilling the billion-parameter models into X00-million-parameter models, or even Y0–million-parameter models, then perhaps a feasible way to reduce both financial costs and environmental costs is cut out the billion-param models entirely, and stick to the cheaper, reasonably accurate multi-million parameter models.
It would cost less to build and distill a 500 million parameter model than it would be to conduct the same process with a billion-parameter counterpart. Sure, there's a potential accuracy reduction, but exactly how much accuracy are we sacrificing?
Again, this thought is up for discussion. And the cost-benefit analysis should be conducted by someone (or someones) with more resources and a bigger brain than I have. Nevertheless, the thought of putting a cap on parameter count for environmental and financial savings remains on the table.
Unlock language AI at scale with an API call.
Get conversational intelligence with transcription and understanding on the world's best speech AI platform.