Nova-2: #1 Speech-to-Text API Now Available in Multiple Languages
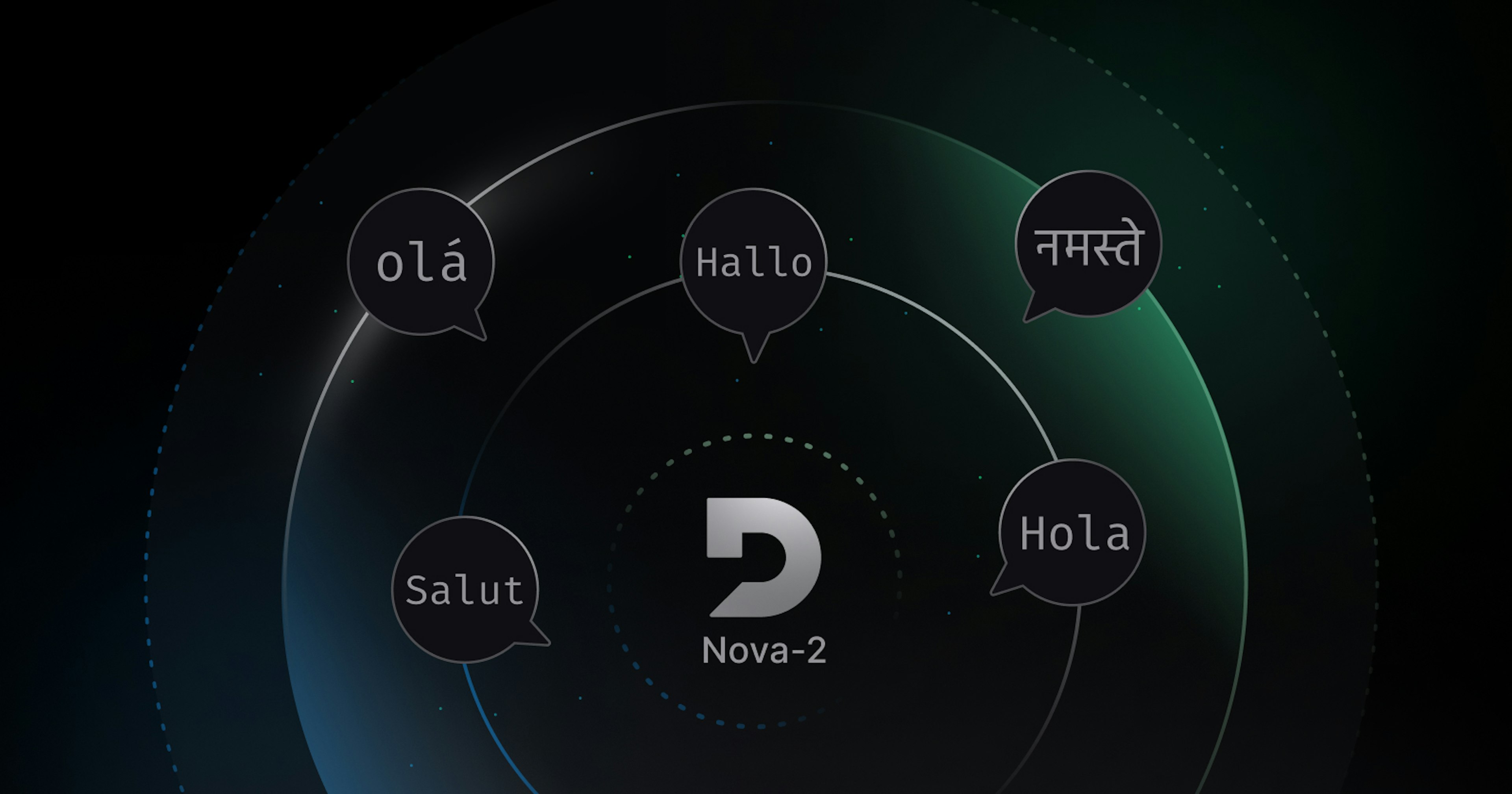
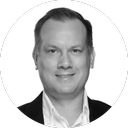
tl;dr:
With more than 60M+ minutes transcribed, our next-gen speech-to-text model, Nova-2, is now generally available.
Nova-2 outperforms all alternatives in terms of accuracy, speed, and cost (starting at $0.0043/min), and extends support for popular languages like Spanish, German, and Hindi as well as custom model training.
Sign up now to get started with Nova-2 and receive $200 in credits absolutely free, or try out all of our models and features in our API Playground! If you're building voice apps at scale, contact us for the best pricing options.
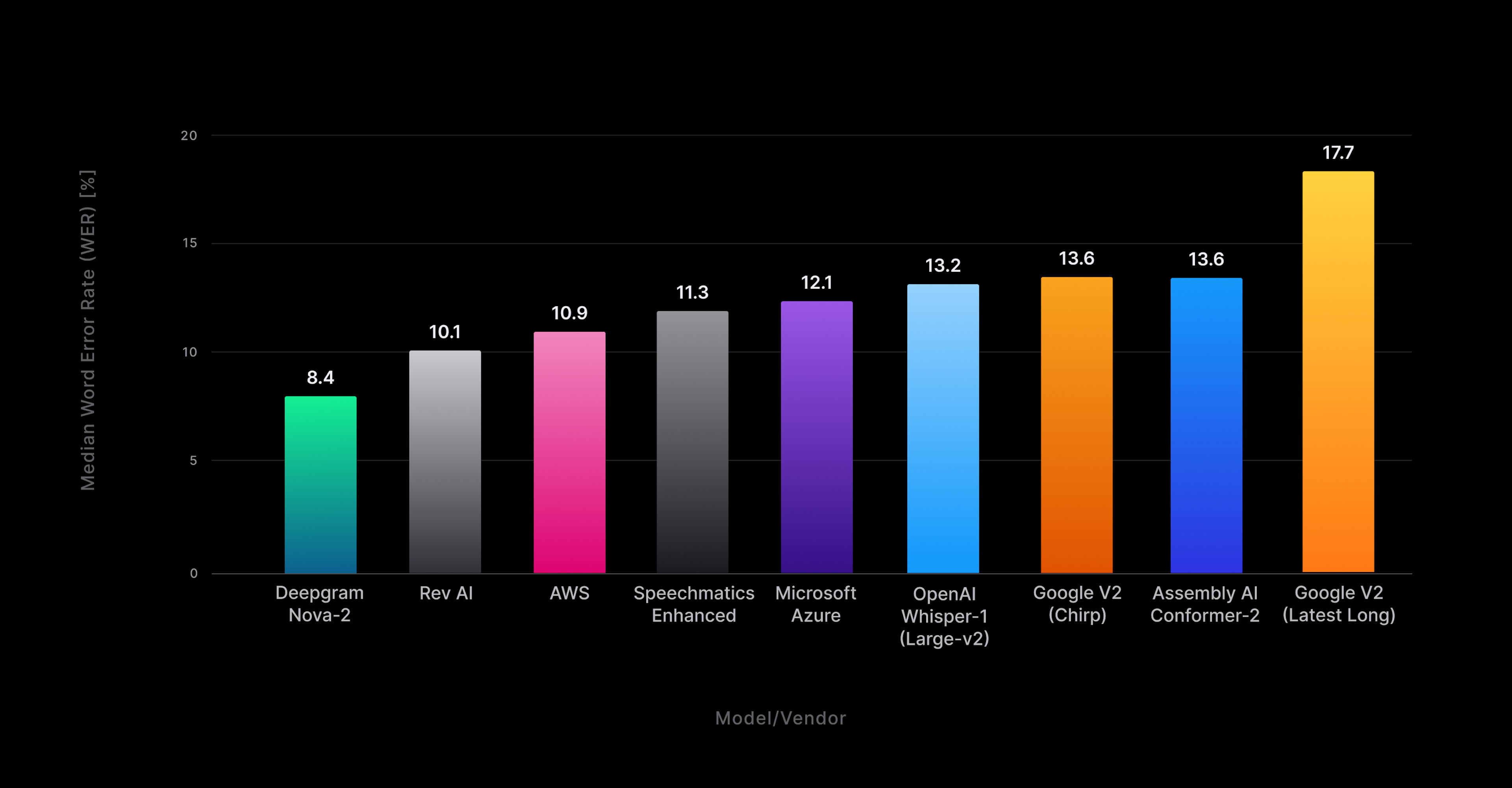
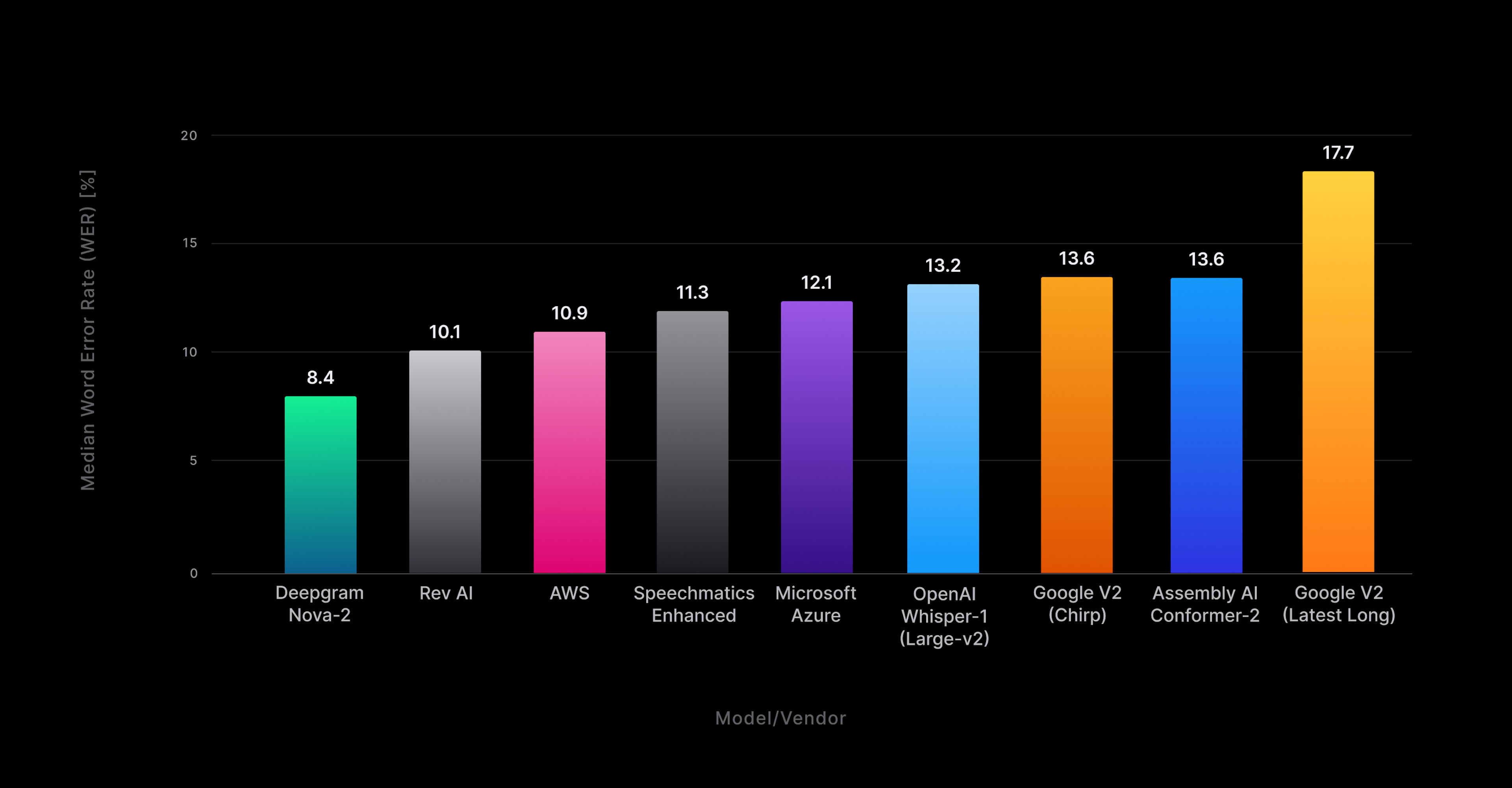
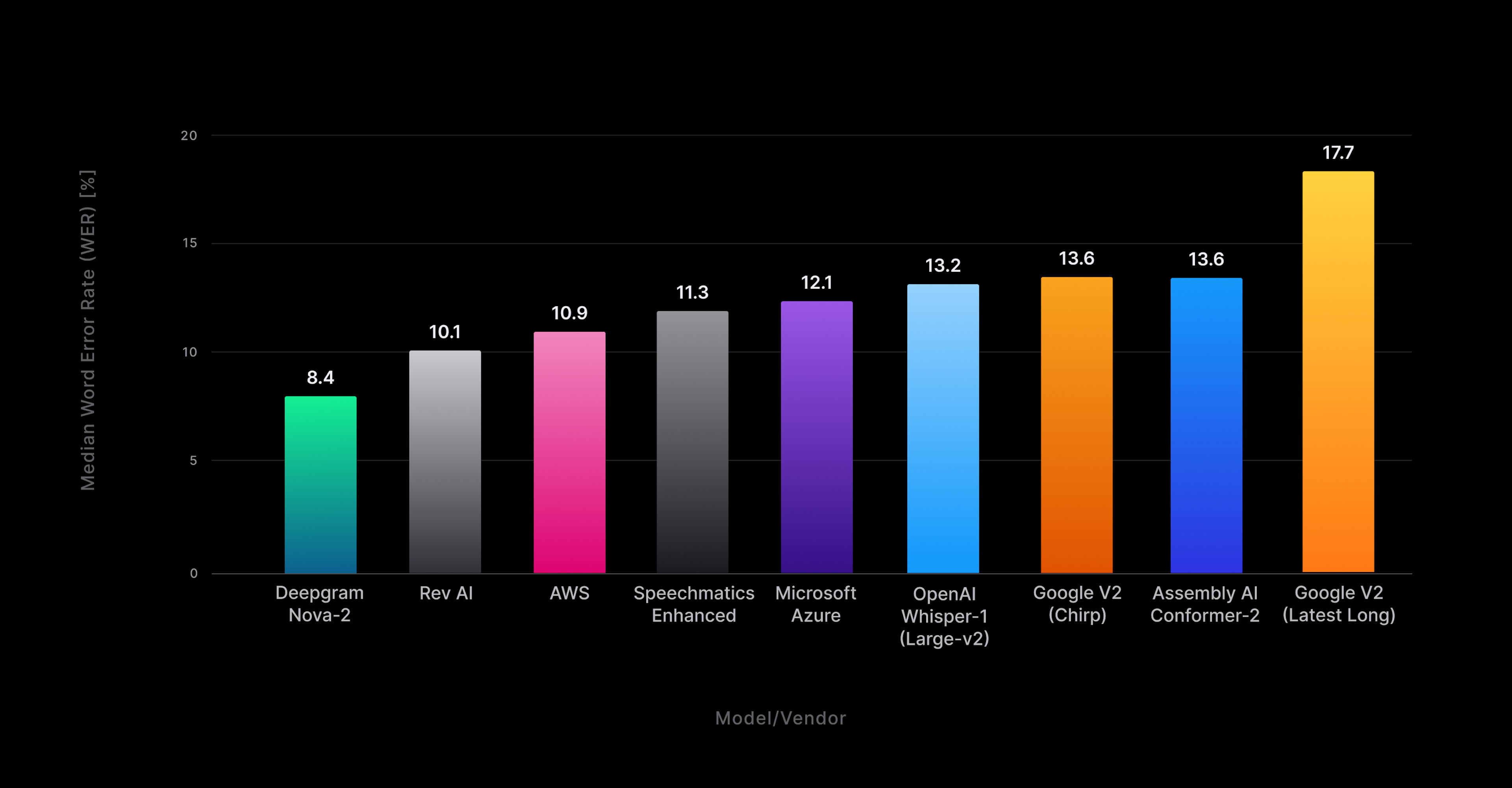
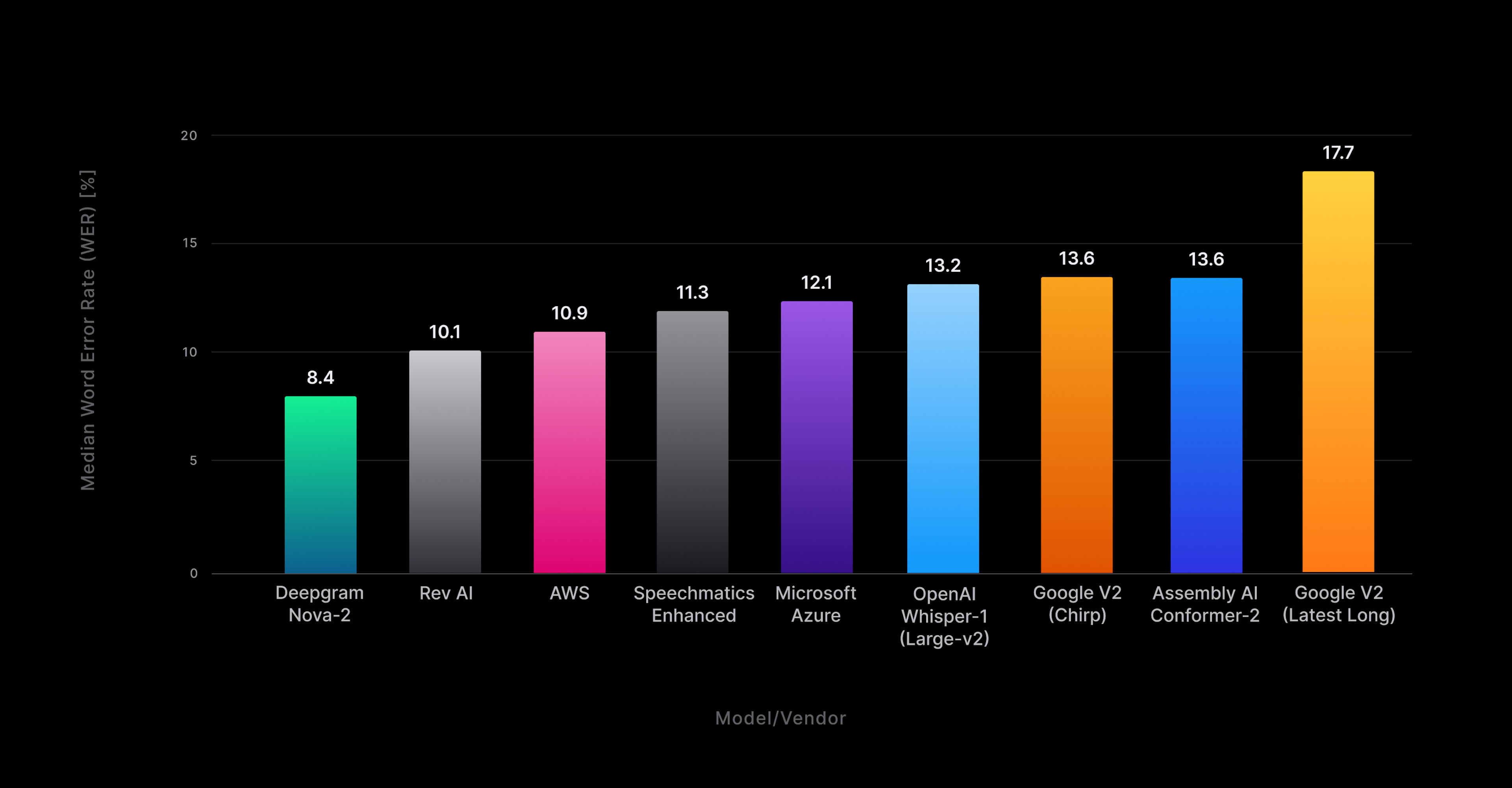
Deepgram Nova-2: It checks all the boxes
When we first announced the release of Deepgram Nova-2, our latest speech-to-text (STT) model impressed early access developers for its unmatched performance and value compared to competitors, delivering:
An average 30% reduction in word error rate (WER)[1] over competitors for both pre-recorded and real-time transcription
5-40x faster pre-recorded inference time
The same low price as Nova (starting at only $0.0043/min for pre-recorded audio) with 3-5x lower cost than the competition
Completeness of features including speaker diarization, smart formatting, word-level timestamps, filler words, and real-time streaming support
Since the early access release, thousands of projects have been created by existing and new customers across scores of domains and use cases–from autonomous AI agents and coaching bots to call center analytics and conversational AI platforms–transcribing more than 60 million audio minutes in a little more than a month. However, the early access model was limited to English audio only. Today, we are pleased to announce the general availability of Nova-2 which includes support for custom model training, on-premises deployment, and non-English transcription in our customers’ most utilized languages.
Unbeatable Speech-to-Text Performance
Human preference evaluation
If you’ve read any of our past announcements, you’re already familiar with our benchmark results centered around industry-standard accuracy metrics for speech-to-text like word error rate (WER) and word recognition rate (WRR). But many research topics in natural language processing (NLP), such as explanation generation, dialog modeling, or machine translation, require evaluation that goes beyond such standard metrics for accuracy and toward a more human-centered approach.
For evaluating LLM-powered speech generation systems, human preference scoring is often considered one of the most reliable approaches in spite of its high costs compared to automated evaluation methods. Even in speech recognition (speech-to-text) and speech synthesis (text-to-speech) domains, traditional metrics are best suited as part of a holistic evaluation approach that may also incorporate human preference scoring, so we thought we’d let users like you participate in a blind test and pick for yourselves.
To this end, we conducted human preference testing using outside professional annotators who examined a set of 50 unique transcripts produced by Nova-2 and three other providers, evaluated in randomized head-to-head comparisons (for a total of 300 unique transcription preference matchups). They were asked to listen to the audio and give their preference of formatted transcripts based on an open-ended criterion.
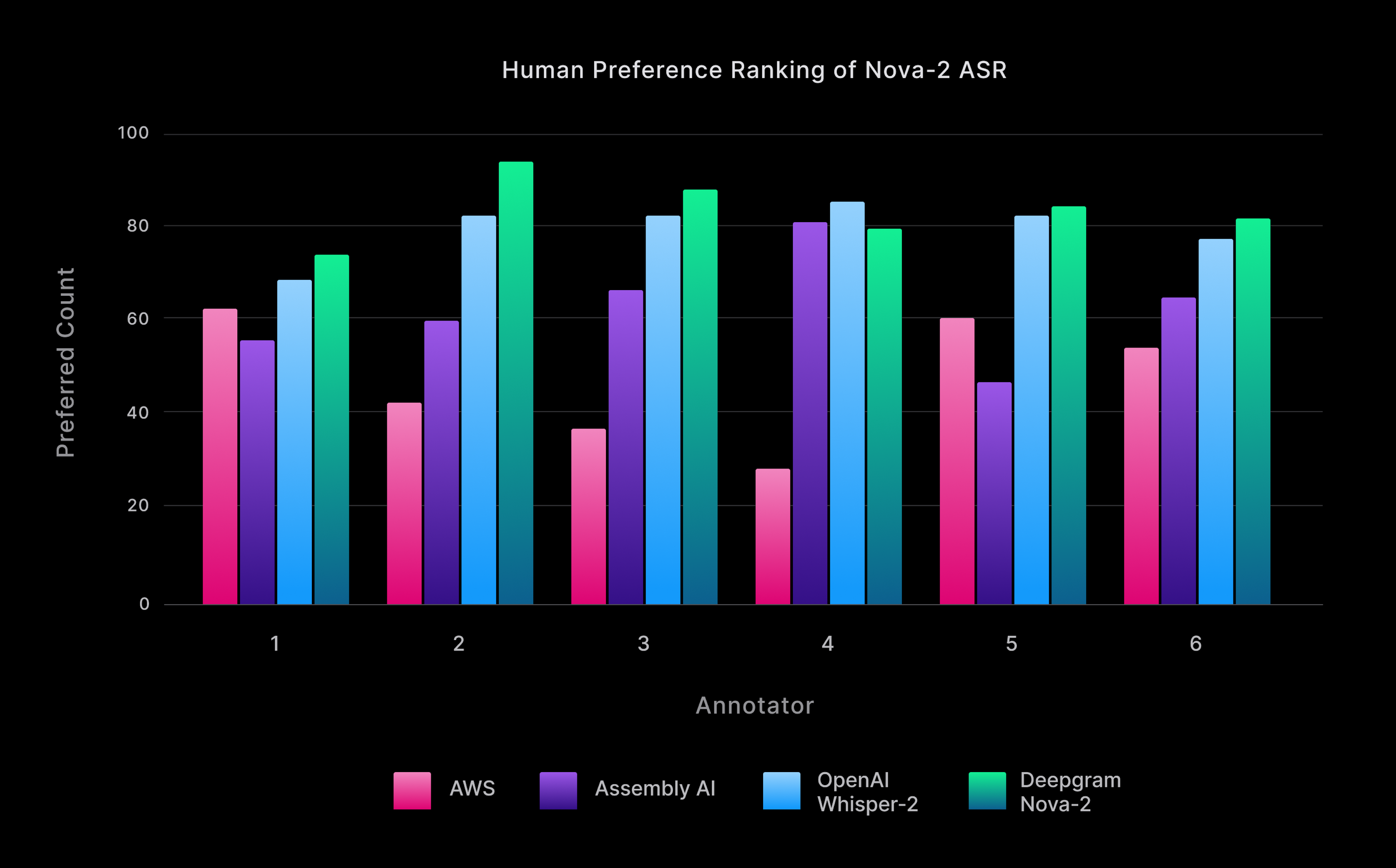
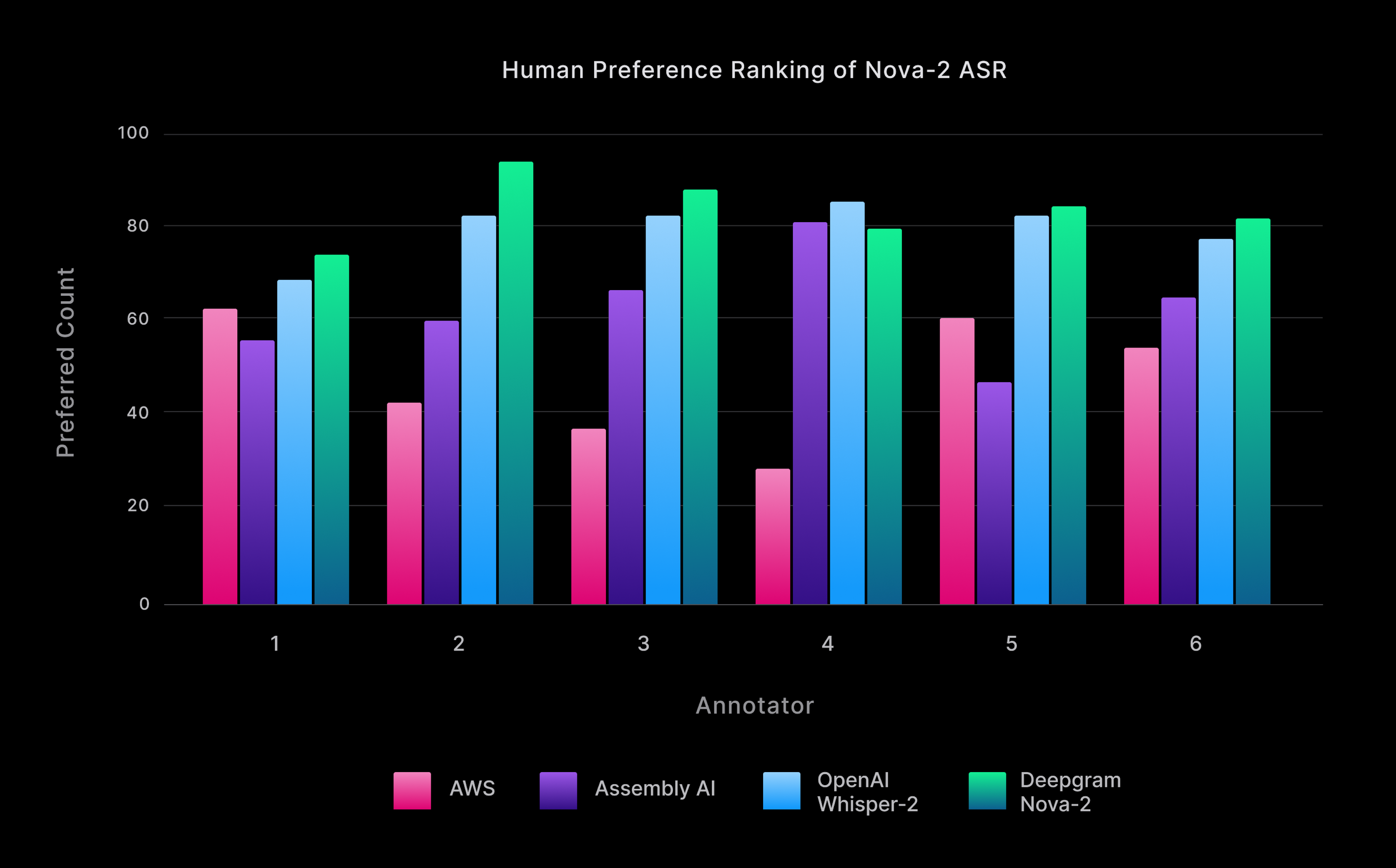
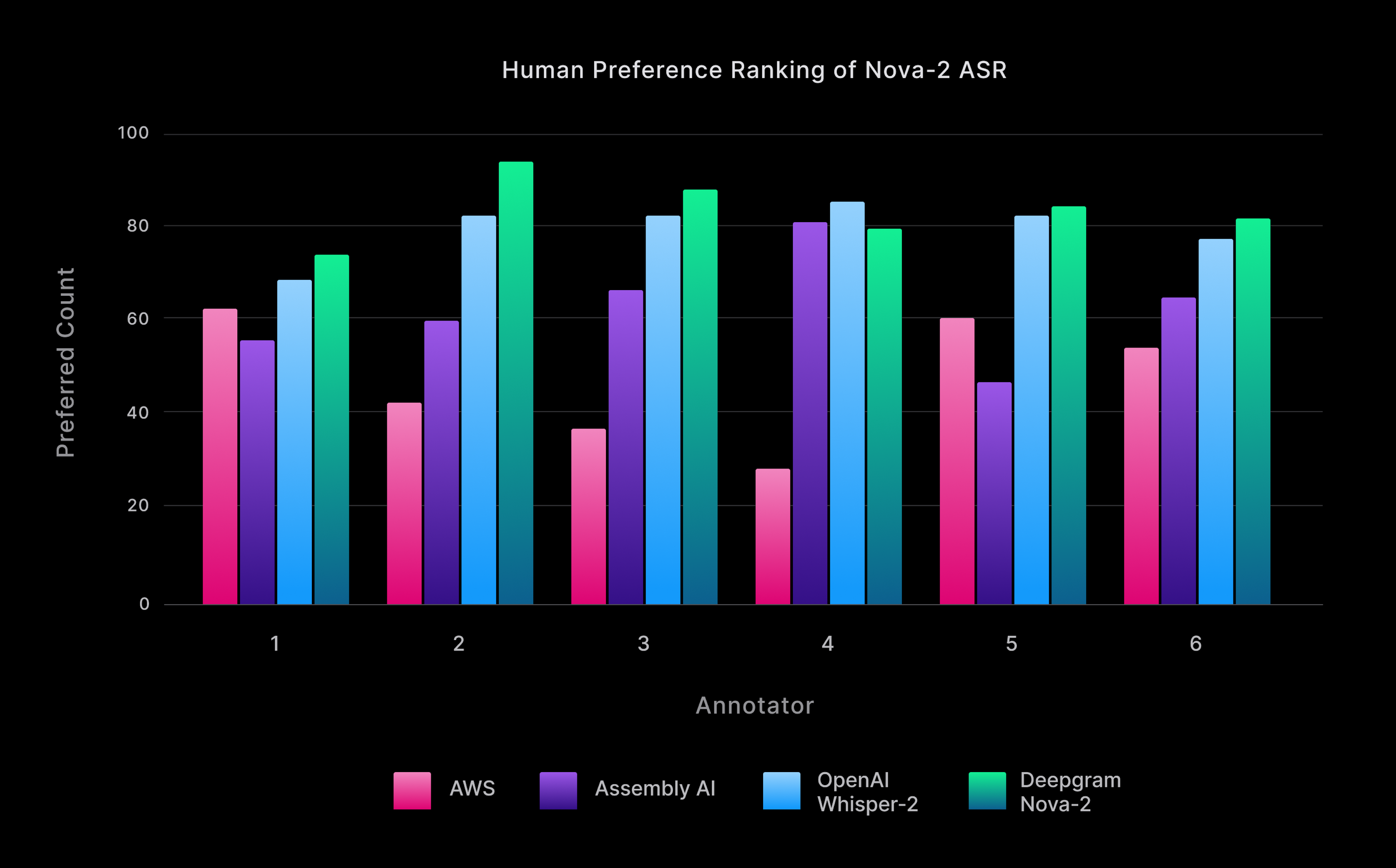
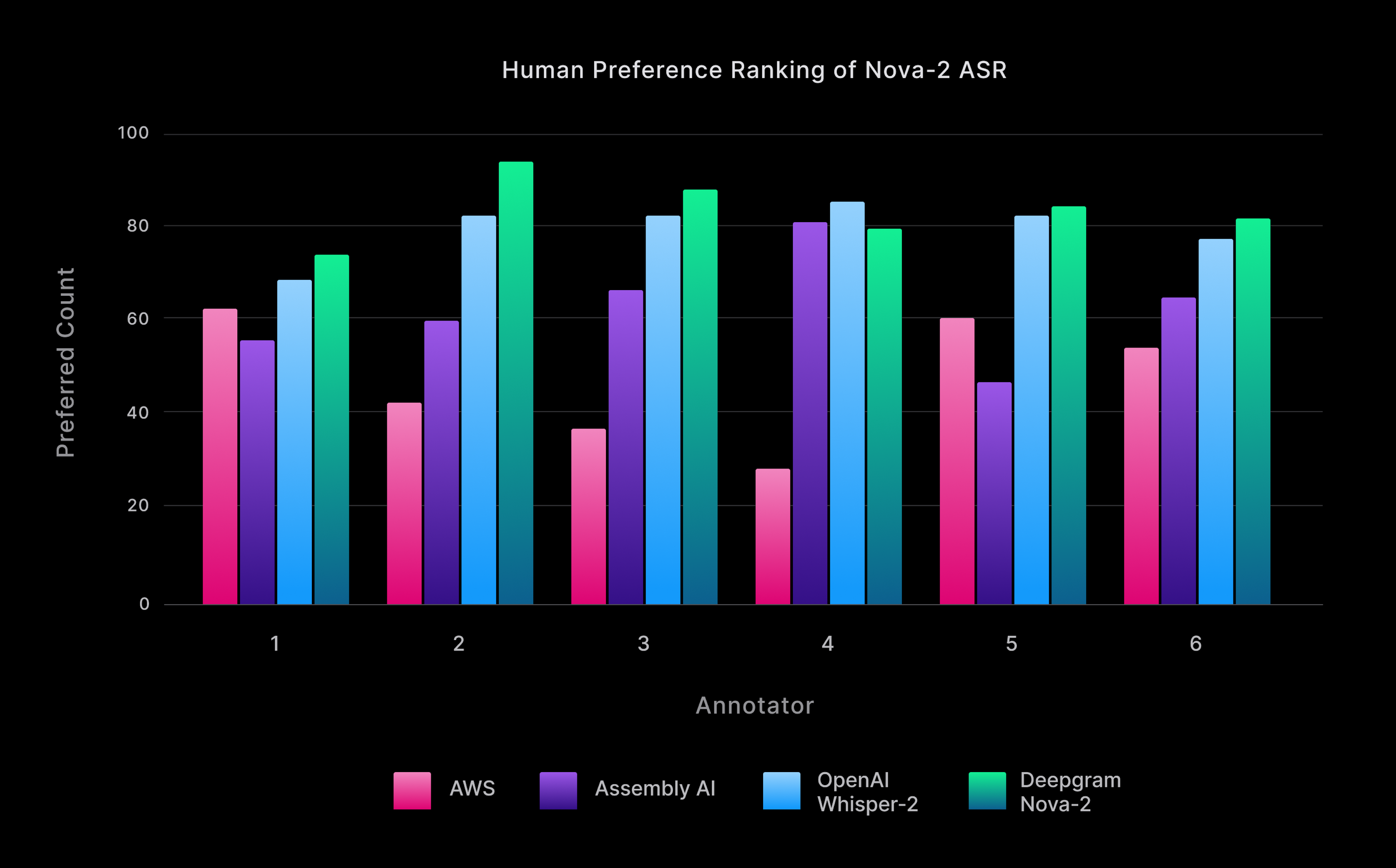
Quality transcripts are key to a positive user experience, often containing specific entities such as dates, email addresses, phone numbers, and monetary figures. Top-tier transcription services not only capture these details with high accuracy but also present them in a reader-friendly format.
Nova-2 enhances Nova-1's intelligent entity recognition, boosting the clarity and usefulness of transcripts for subsequent processing. It achieves this with a 15% relative drop in entity errors and marked enhancements in punctuation and capitalization accuracy—improving by 22.6% and 31.4%, respectively.
In head-to-head comparisons, transcripts generated by Nova-2 were preferred over the other vendors ~60% of the time, and 5 out of 6 annotators preferred formatted Nova-2 results more than any other STT vendor. Nova-2 had the highest overall win rate at 42%, which is a 34% higher win rate than the next best, OpenAI Whisper[2]. The overall win rates by vendor are shown in Fig. 3.
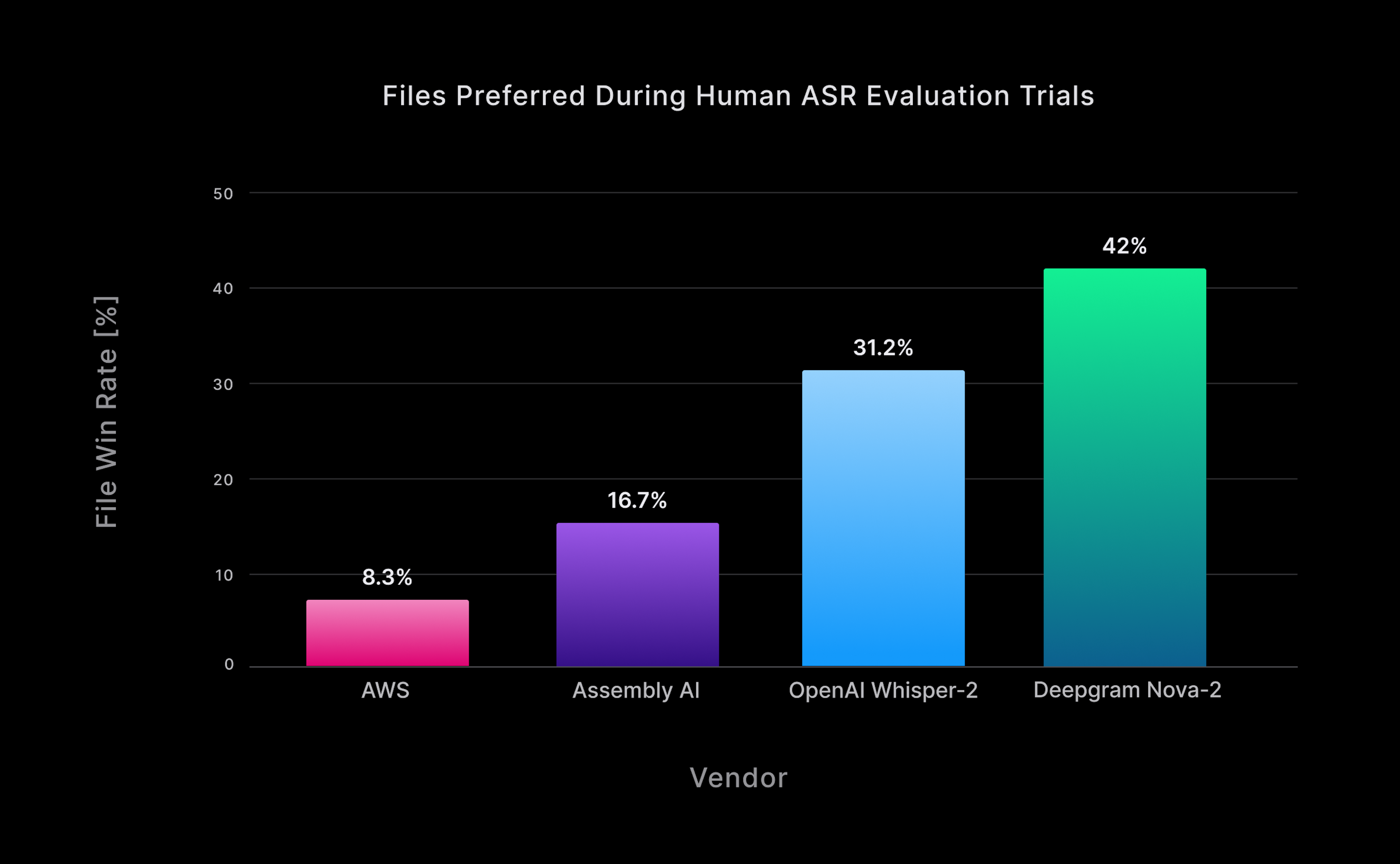
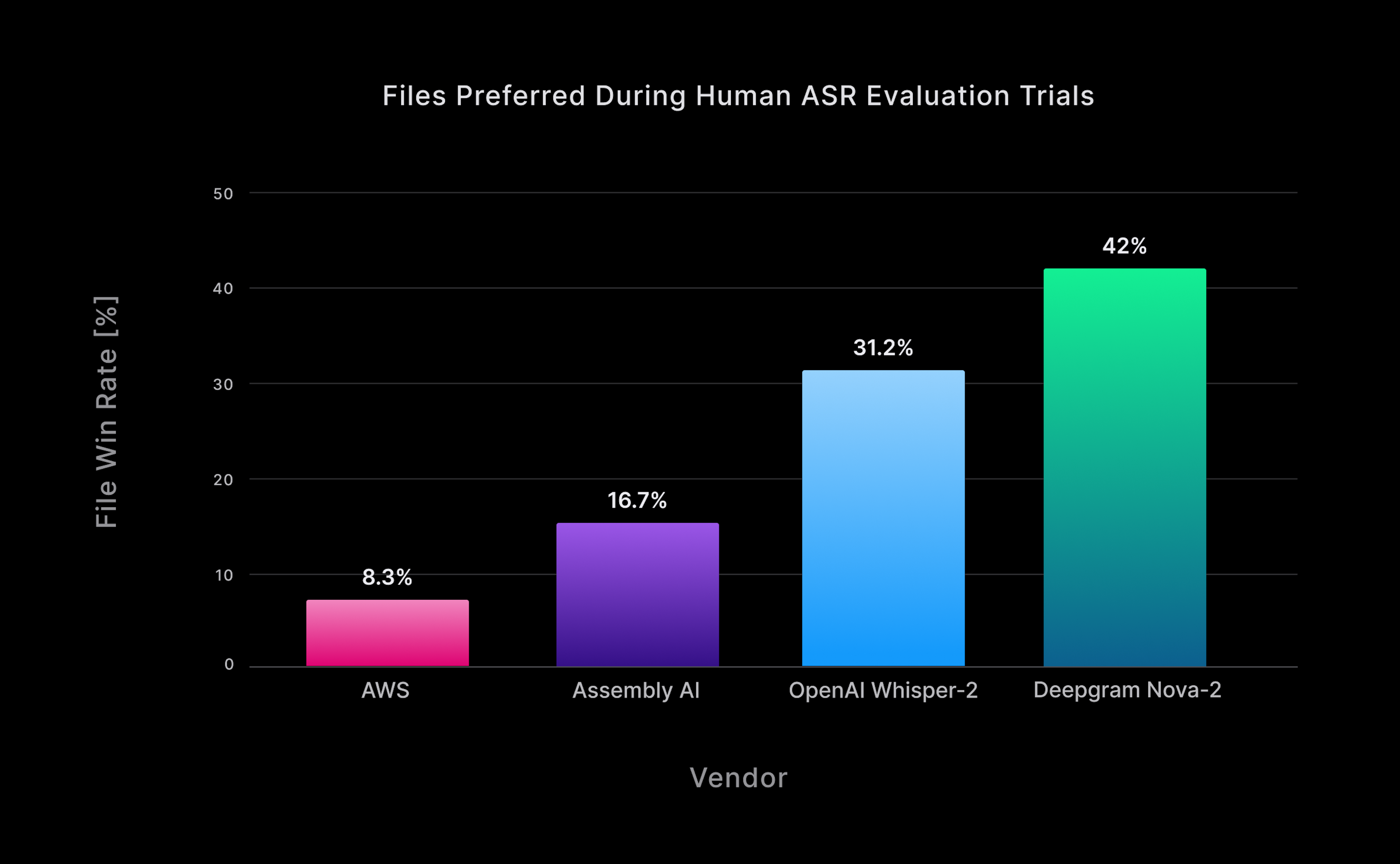
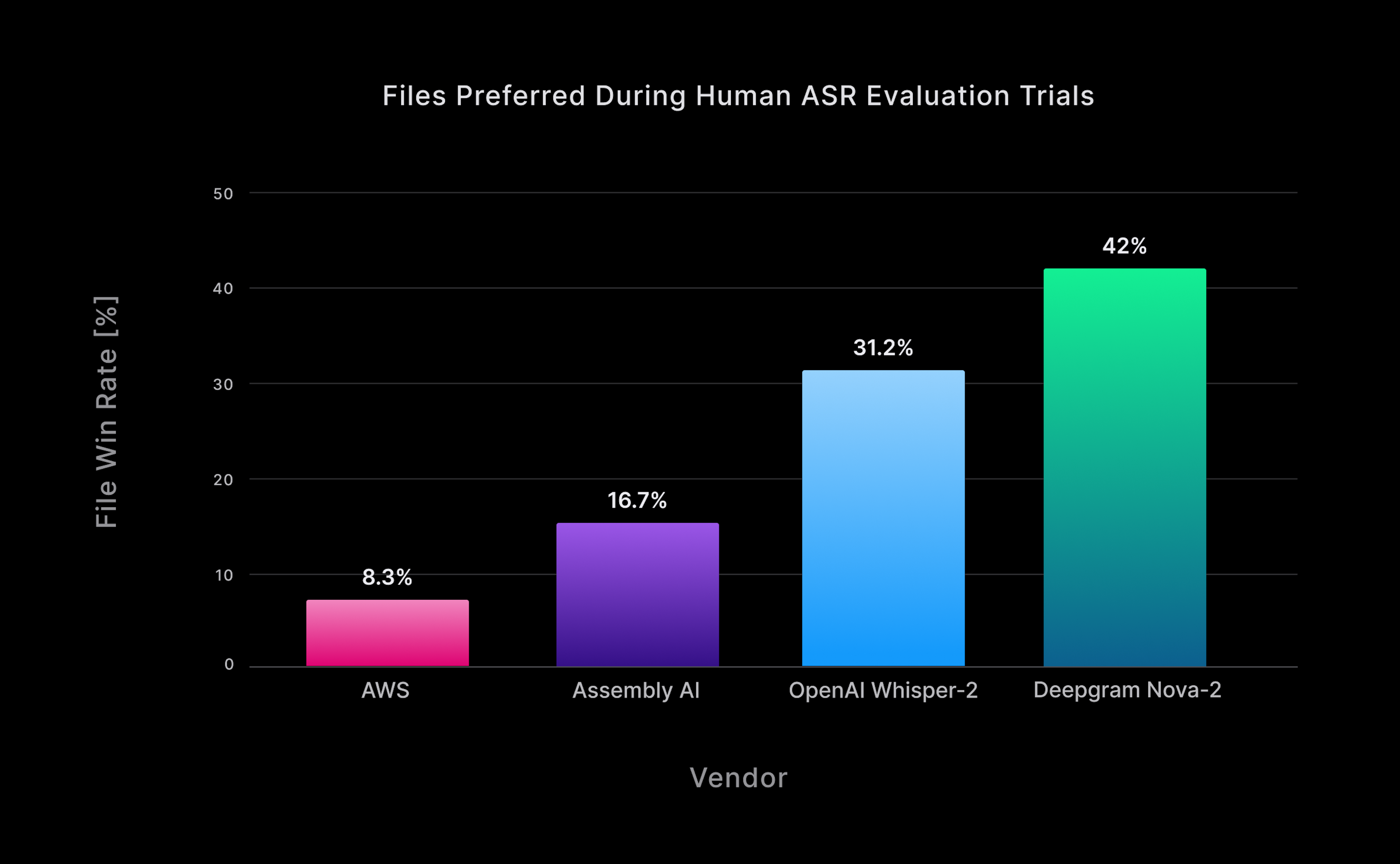
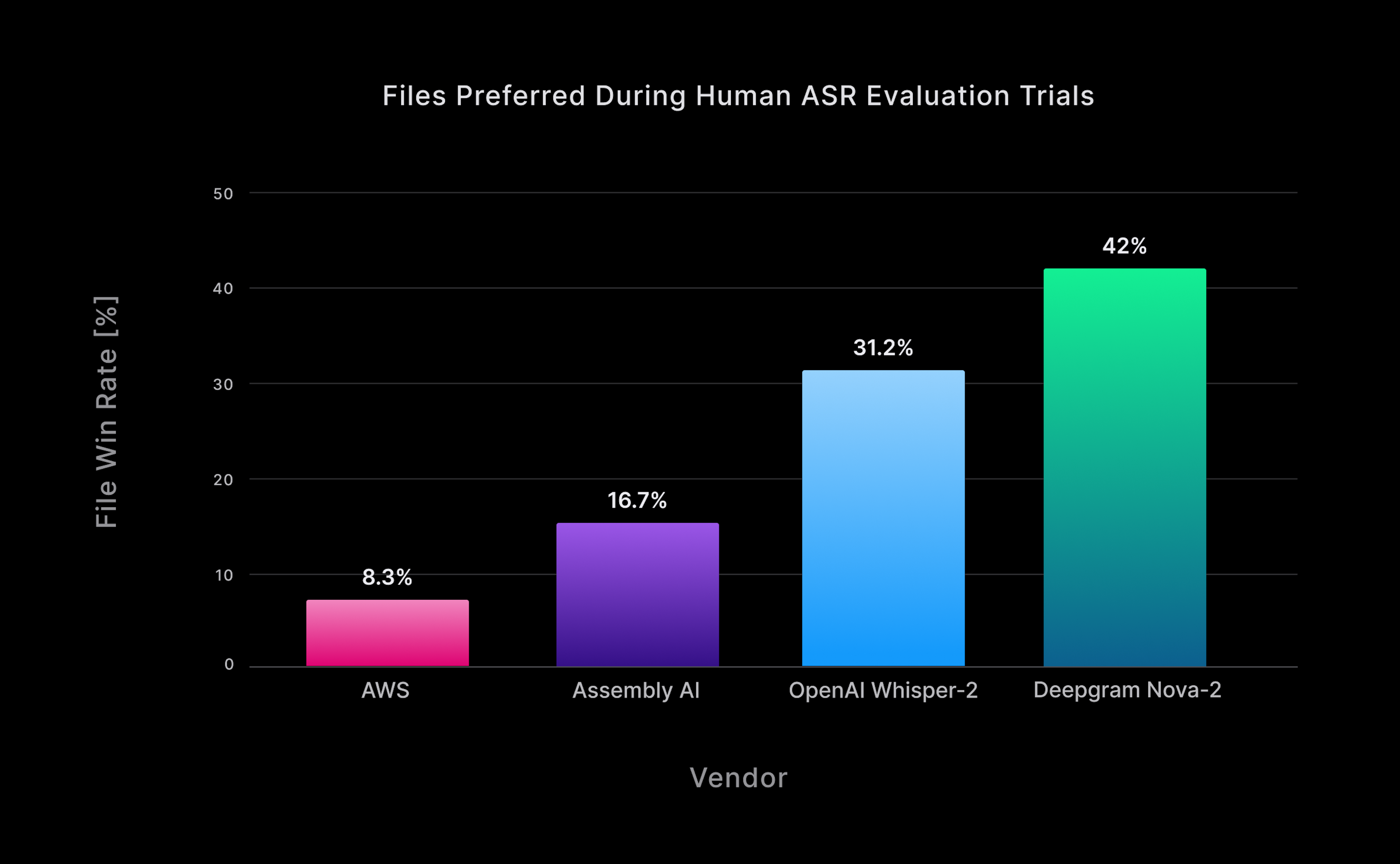
We conducted benchmarks against OpenAI’s recently released Whisper-v3, but were a bit perplexed by the results as we frequently encountered significant repetitive hallucinations in a sizable portion of our test files. The result was a much higher WER and wider variance for OpenAI’s latest model than we expected. We are actively investigating how to better tune Whisper-v3 in order to conduct a fair and comprehensive comparison with Nova-2. Stay tuned and we will share the results in an upcoming article once we’ve completed this exercise.
30% more accurate in non-English languages than the competition
Our customers support end users operating in dynamic, multilingual settings, necessitating versatile transcription services that go beyond English. They span numerous audio areas—ranging from AI chatbots to podcasts, and from corporate meetings to customer service and sales calls. With multilingual speech-to-text capabilities, businesses can deliver tailor-made experiences, ensuring language-specific call routing, enhanced media accessibility, and compliance with language-specific regulations. However, the cornerstone of their operations remains the transcription service's core performance—its speed and accuracy—across all spoken languages.
Support for non-English speech recognition has traditionally been inadequate. Performance has been unbalanced, with English and Spanish achieving word error rates (WER) of approximately 7-20%, while other languages, like Hindi, experience WERs of 15-60% (see Fig. 4). Our Nova-2 architecture aims to bridge this gap by enhancing language support, which we believe will substantially improve global access to effective automatic speech recognition.
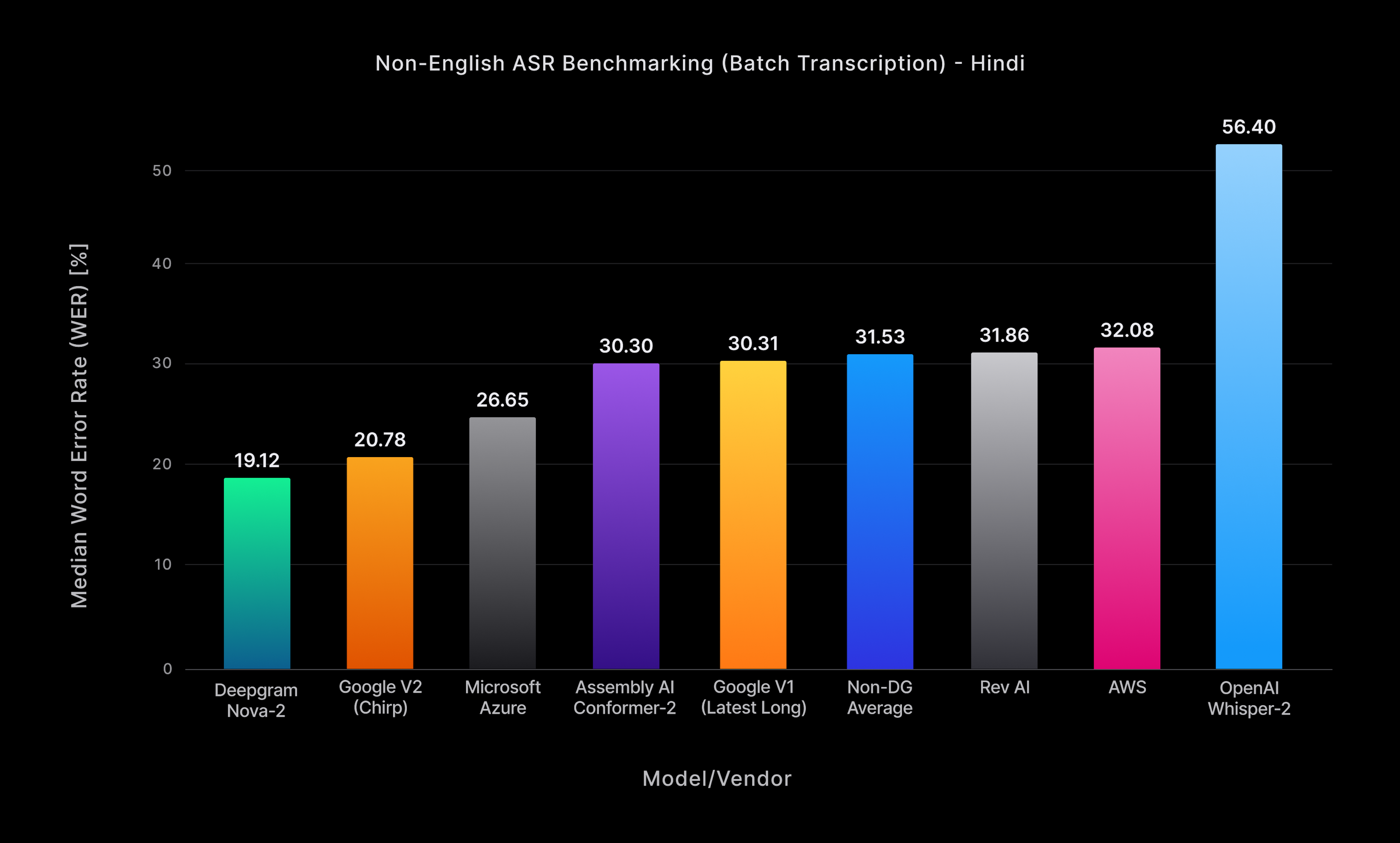
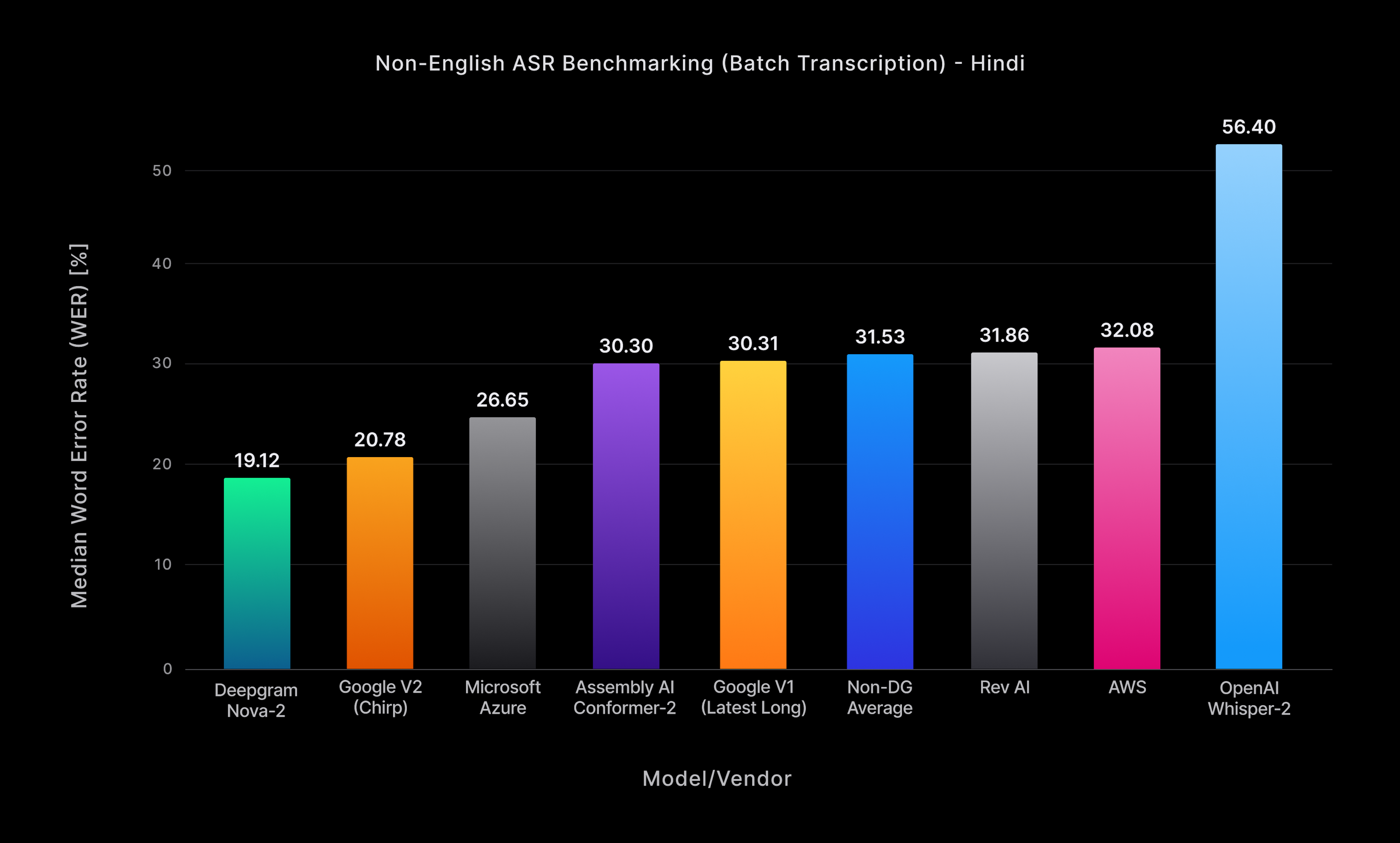
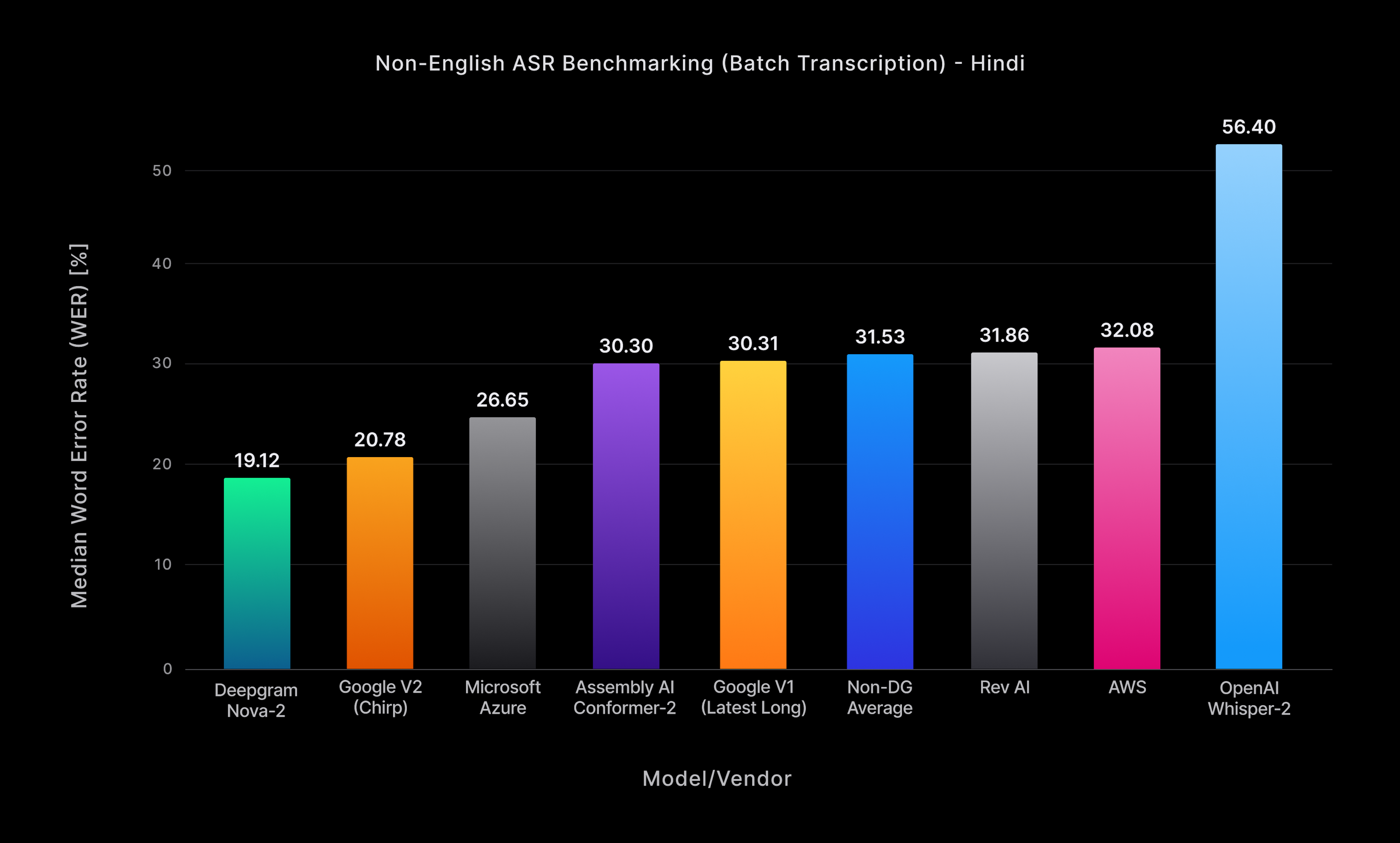
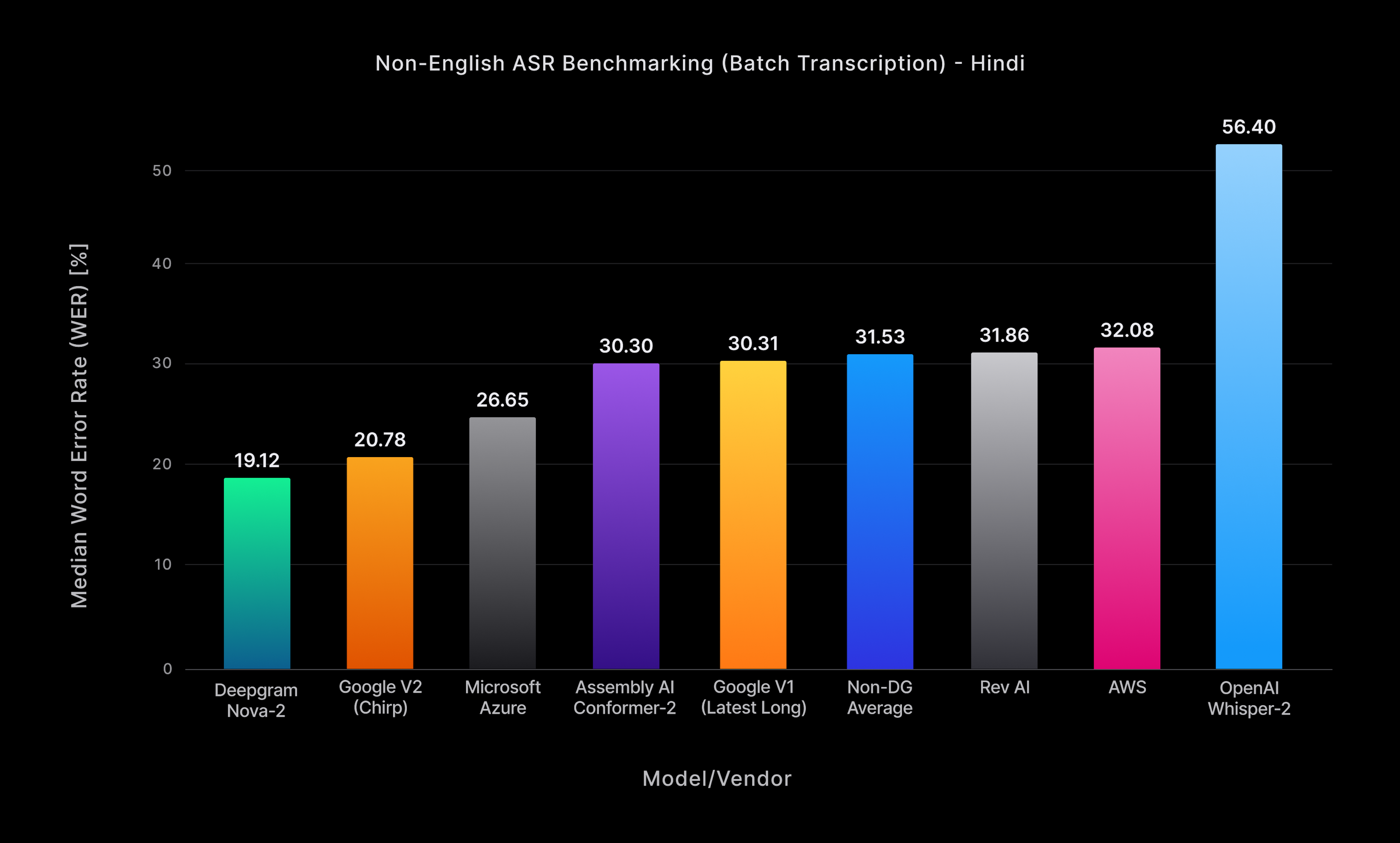
Our benchmarking methodology for non-English languages with Nova-2 utilized over 50 hours of high quality, human-annotated audio carefully curated by our in-house data ops team. As is standard in our testing, this encompassed a wide range of audio lengths, varying environments, diverse accents, and subjects across many domains, including phone calls, meetings, newscasts, podcasts, radio, etc.
We transcribed these data sets with Nova-2 and some of the most prominent STT models in the market for leading non-English languages of interest. We then normalized[1] the results to follow the same formatting standard to ensure a fair comparison. Finally, we calculated the resulting word error rate (WER) in pre-recorded and streaming modalities where applicable. A number of providers support pre-recorded audio only, and others only support real-time streaming in a few select languages and were thus omitted from the respective tests.
The results show Nova-2 beats the performance of all tested competitors by an average of 30.3%. Of particular note is Nova-2’s sizable performance advantage for Hindi (41% relative WER improvement on average), Spanish (15% relative WER improvement) and German (27% relative WER improvement), as well as a 34% relative WER improvement over OpenAI’s popular Whisper (large-v2) model.
Figure 5 demonstrates that Nova-2 not only outperforms rivals in accuracy but also shows less variation in results, leading to more reliable transcripts across diverse languages in practical applications.
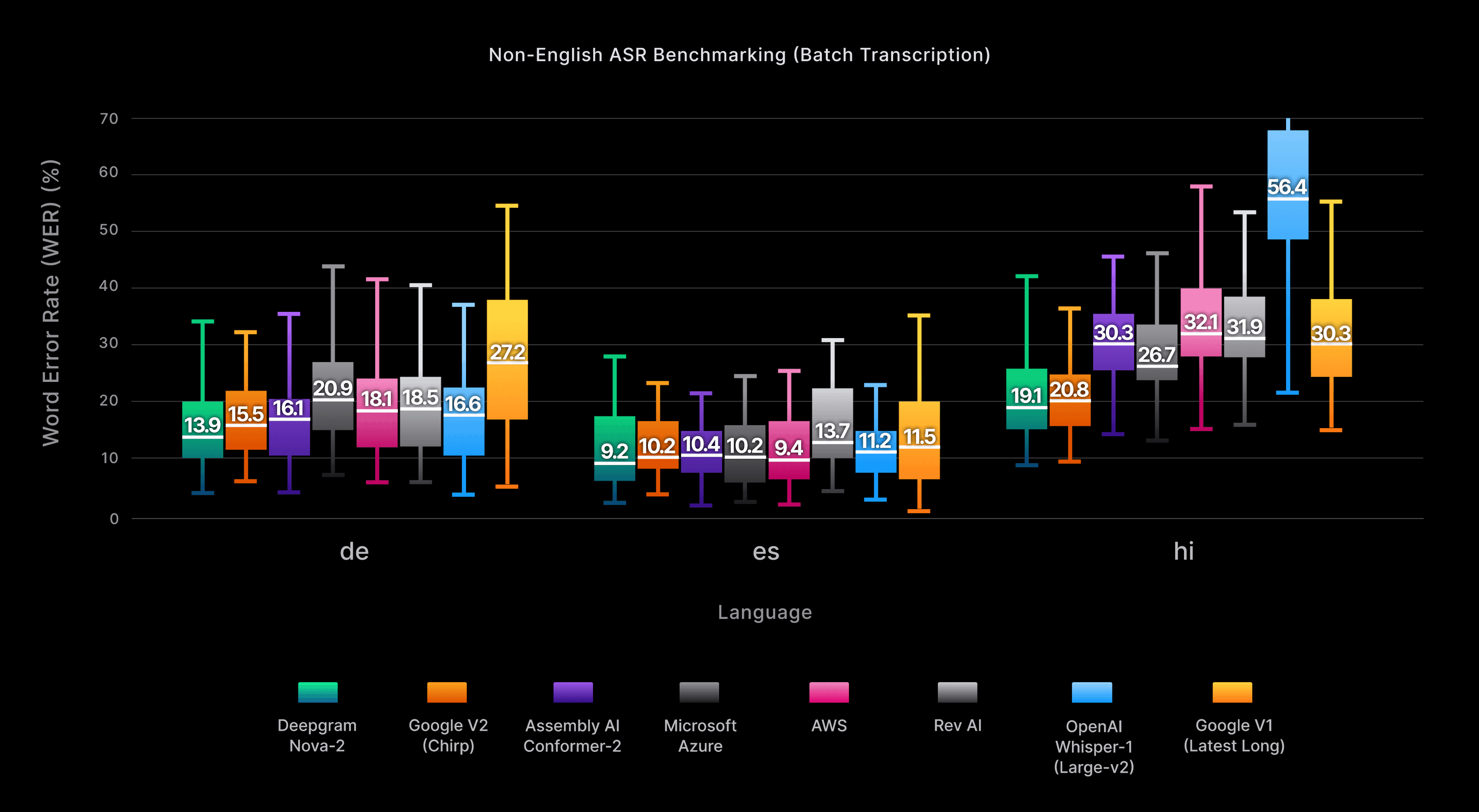
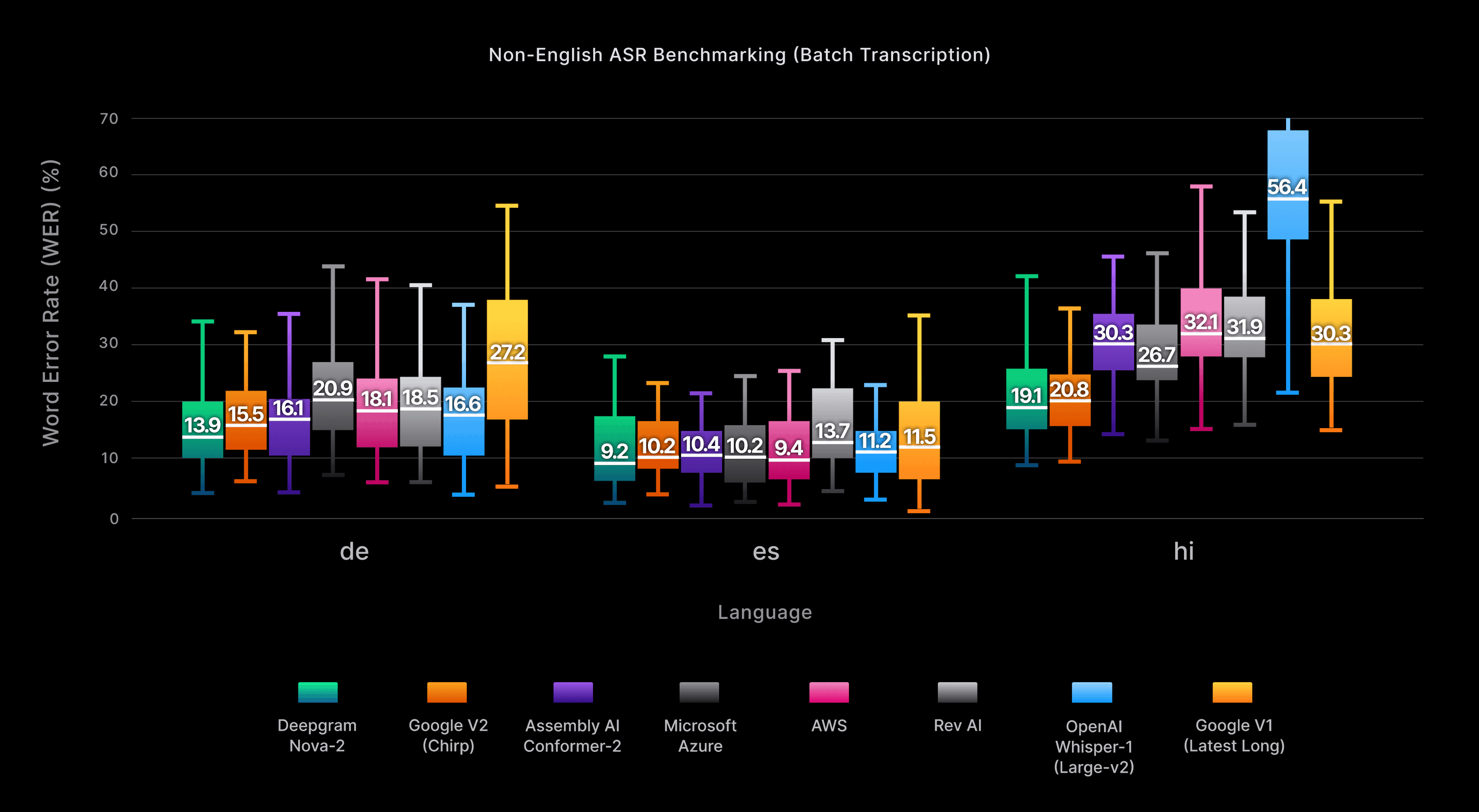
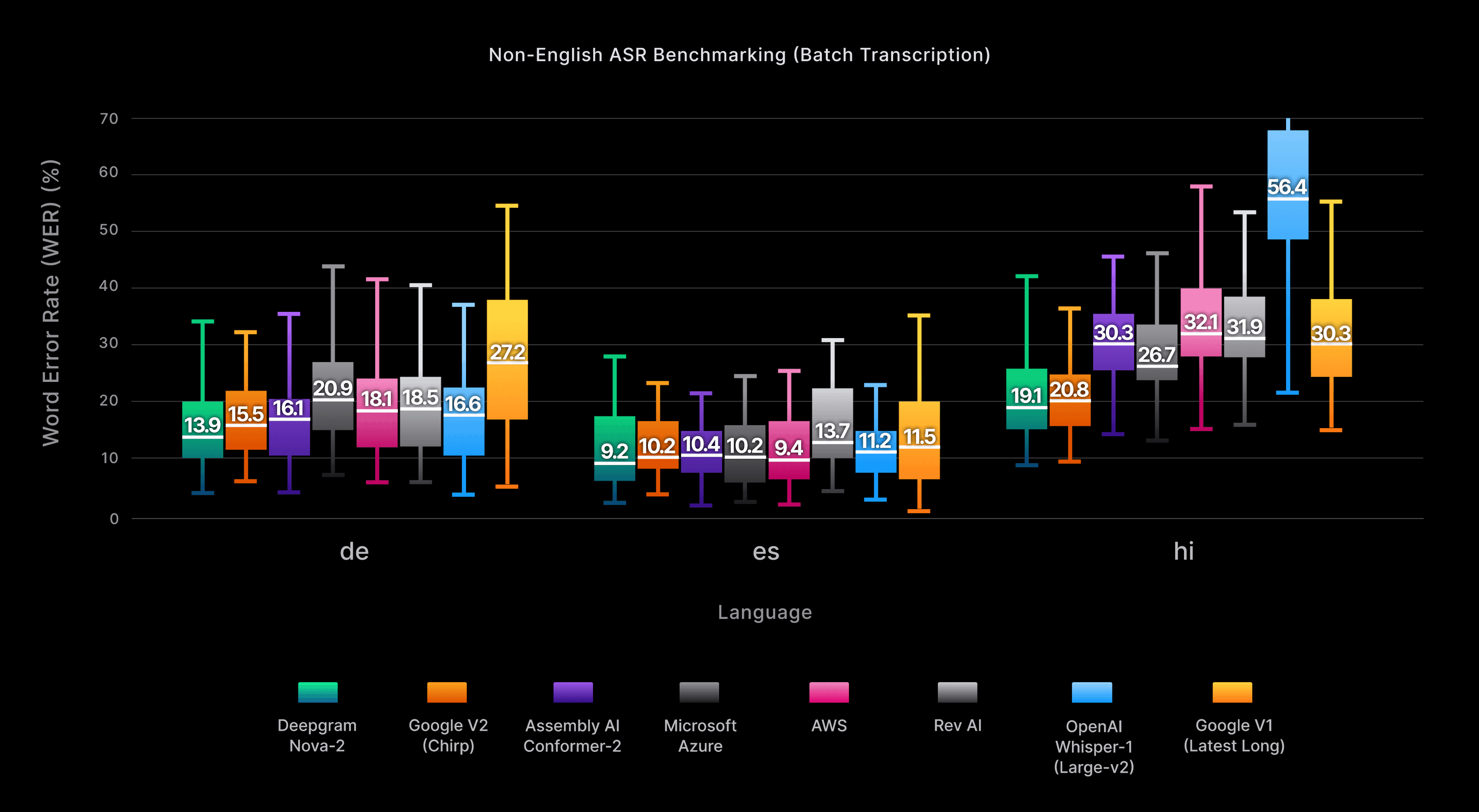
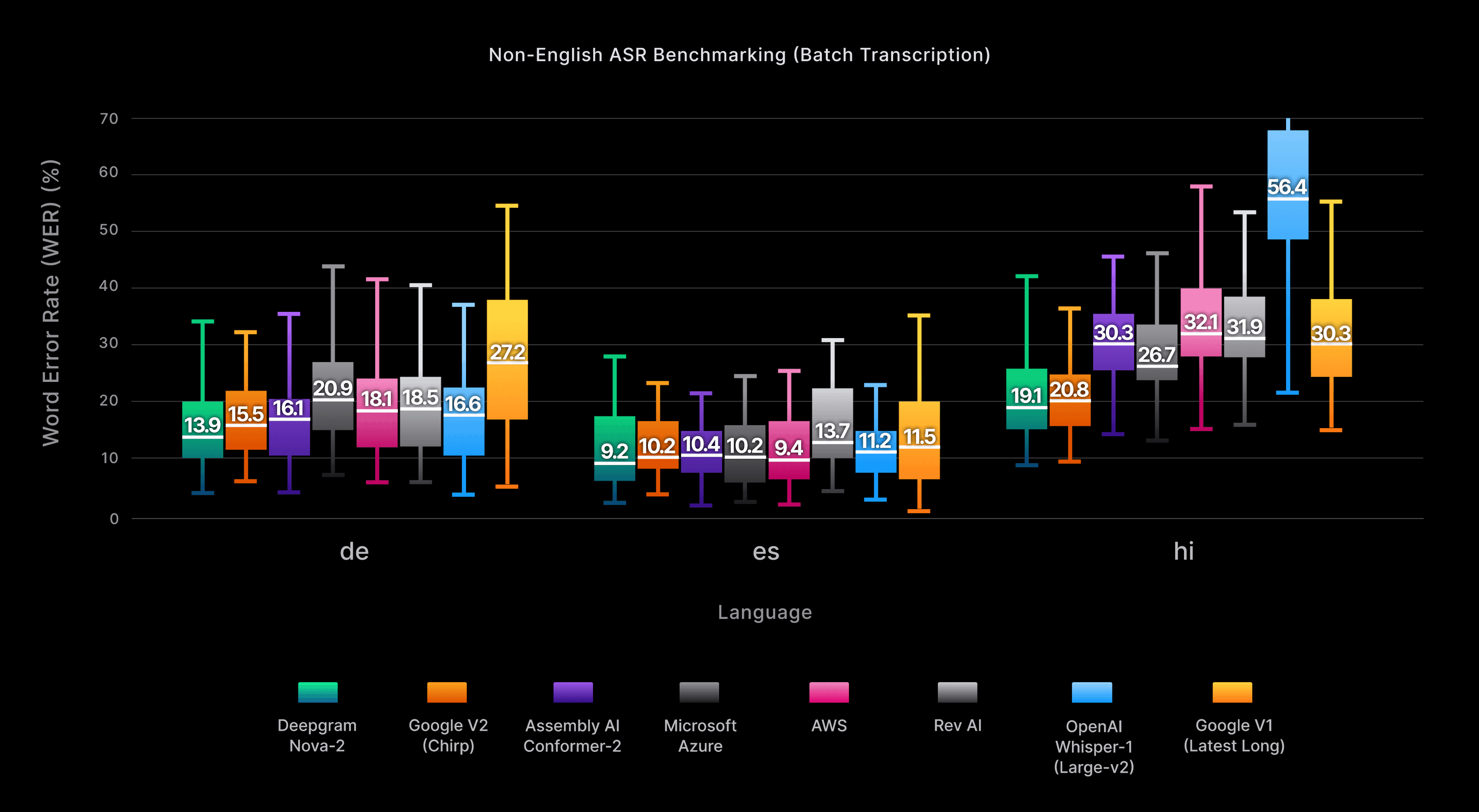
Unrivaled performance in real-time accuracy
For non-English streaming, Nova-2 beats the competition by more than 2% in absolute WER over all languages combined with a 23% relative WER improvement on average (and 11% relative WER improvement over the next best alternative, Azure) as shown in Fig. 6. This advantage continues to grow in importance as contemporary voice applications–such as real-time agent assist and customer care voicebots–increasingly rely on instant transcription features to automate user interactions and provide superior customer experiences for multilingual end users.
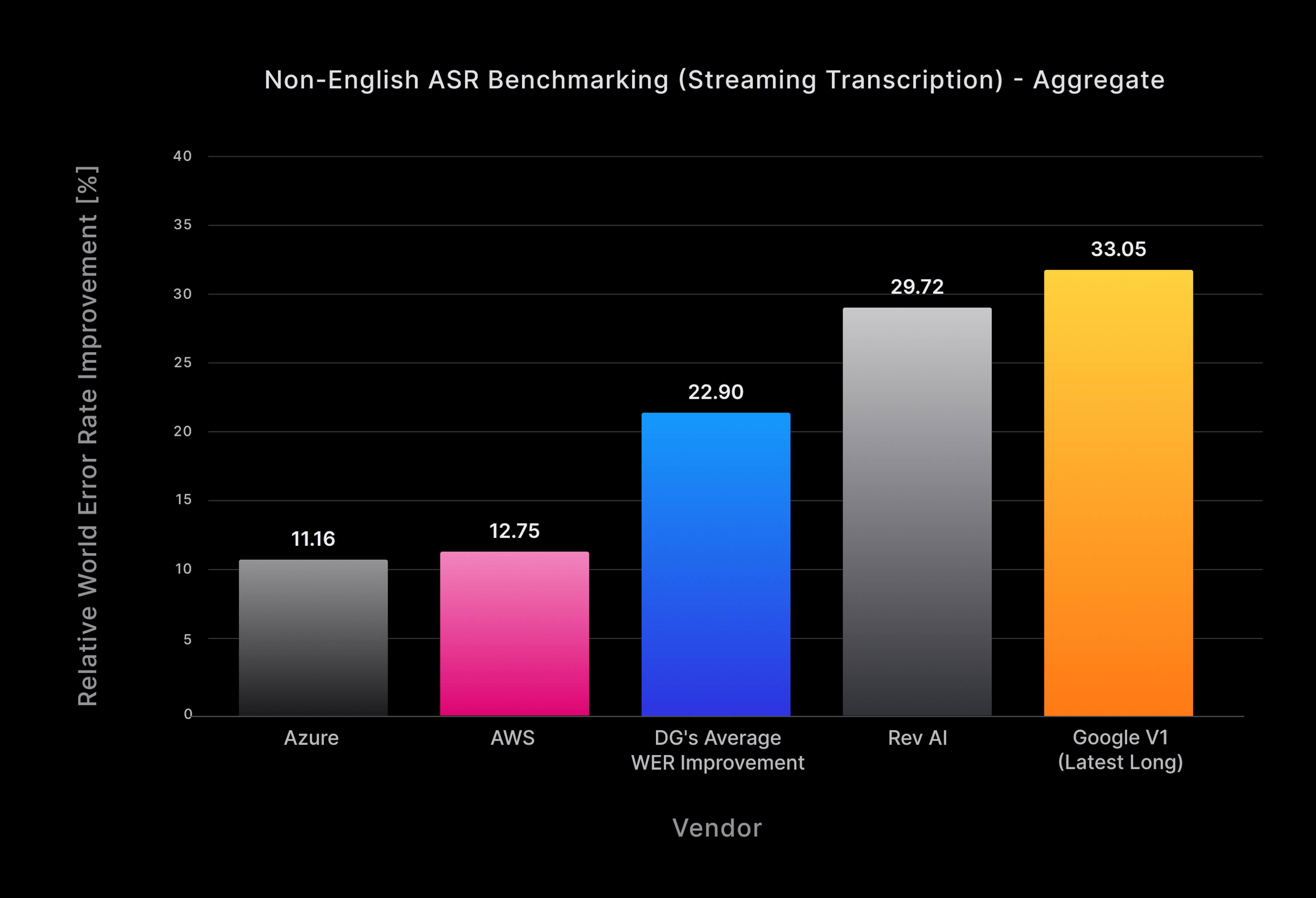
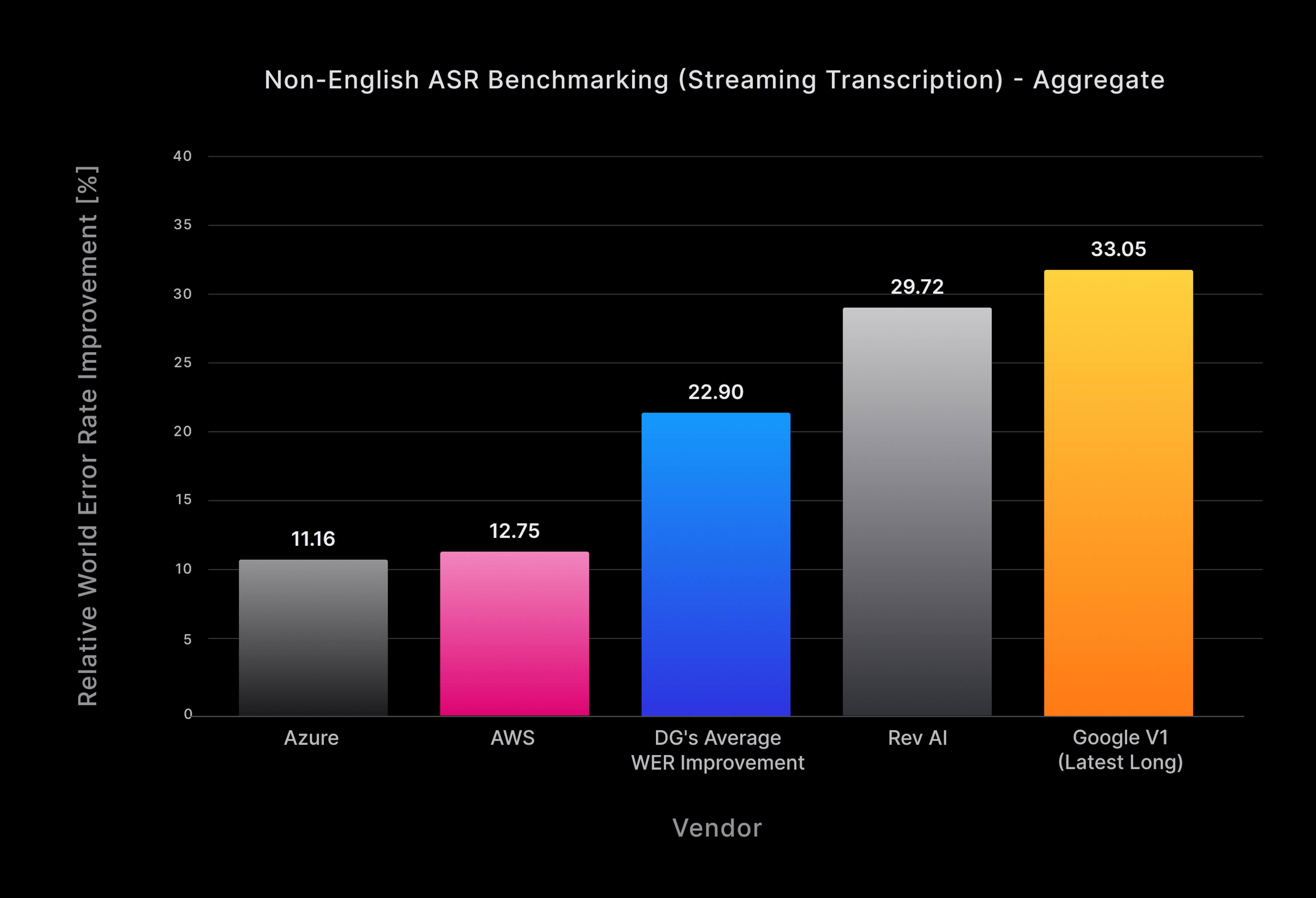
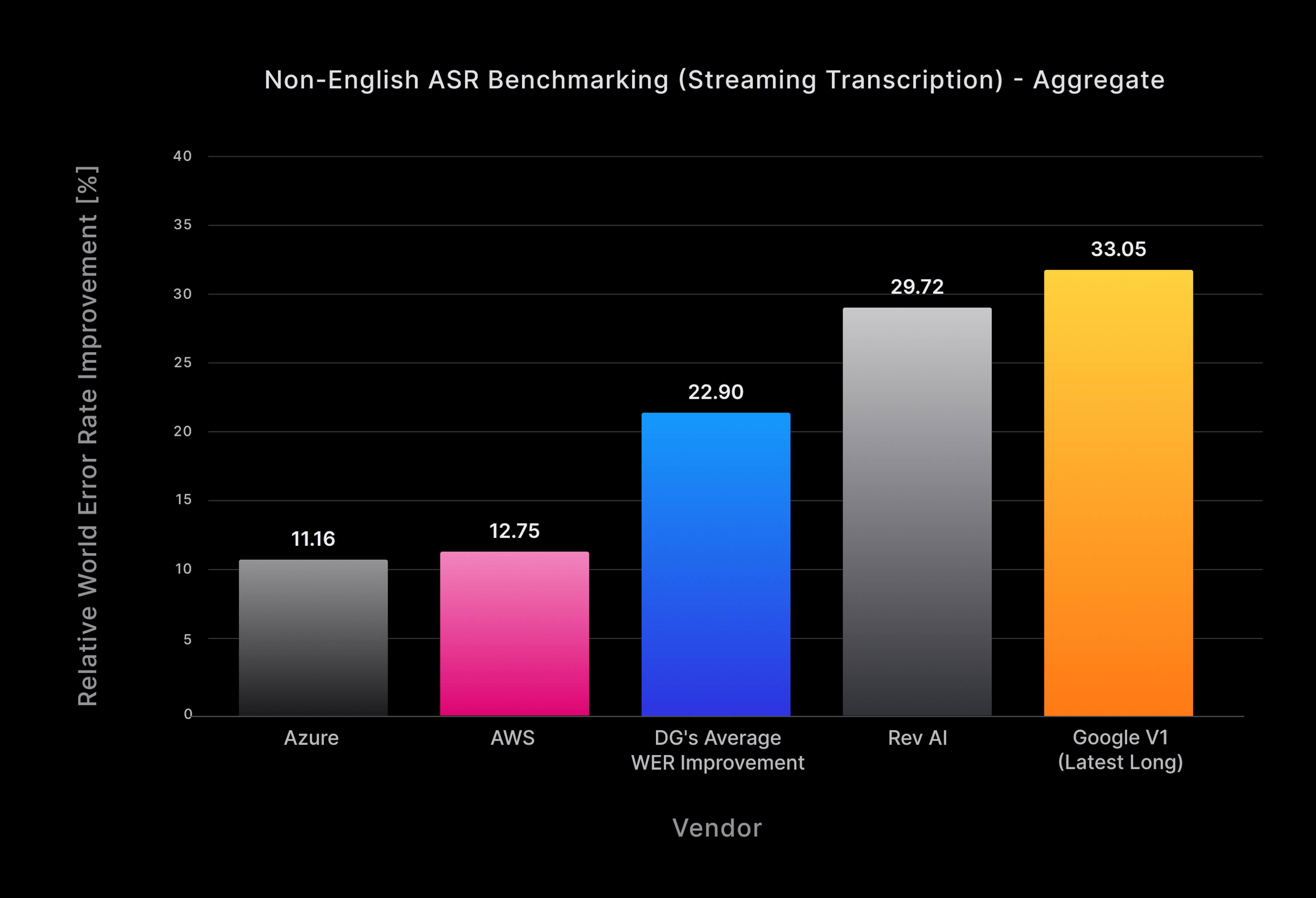
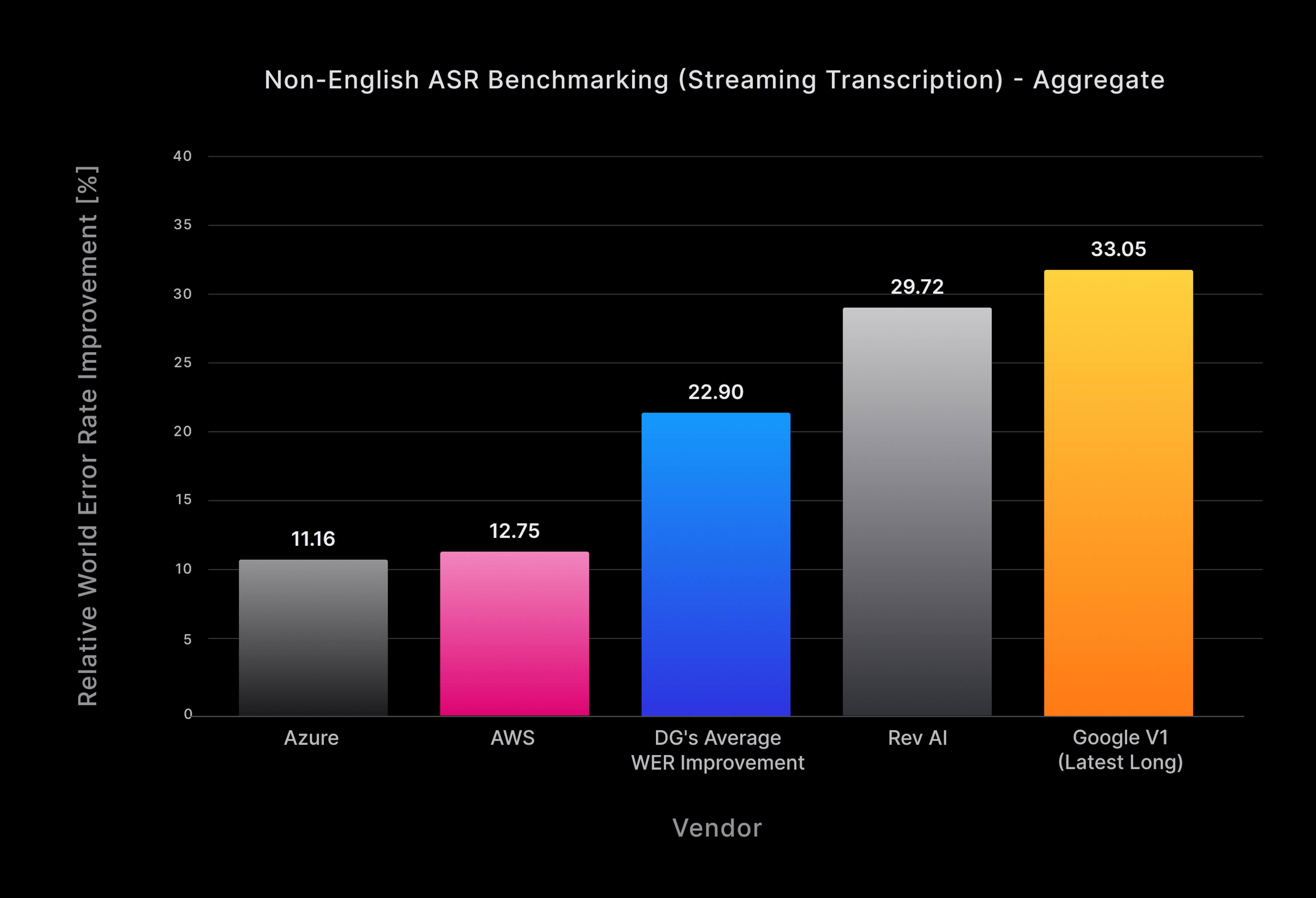
The biggest bang for your buck
Deepgram Nova-2 certainly stands out for its speed and accuracy, but it also continues the legacy of its predecessor by being cost-effective. Our advanced, next-gen models enhance operational efficiency, leading to significant cost savings for your business.
Priced competitively at just $0.0043 per minute for pre-recorded audio, Nova-2 offers the same affordability as the original Nova model, making it 3-5 times more affordable than other comprehensive providers in the market (based on current listings).
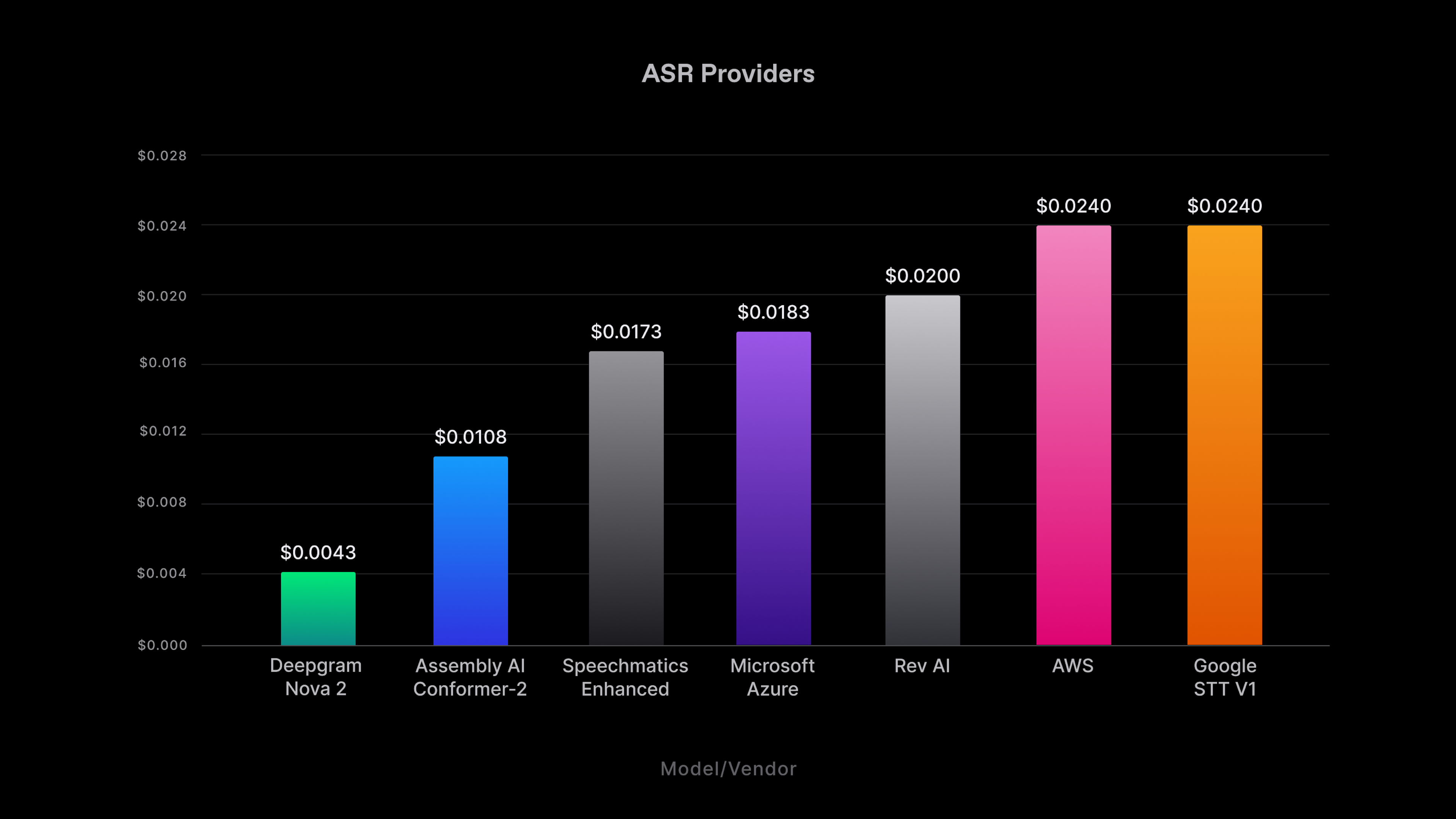
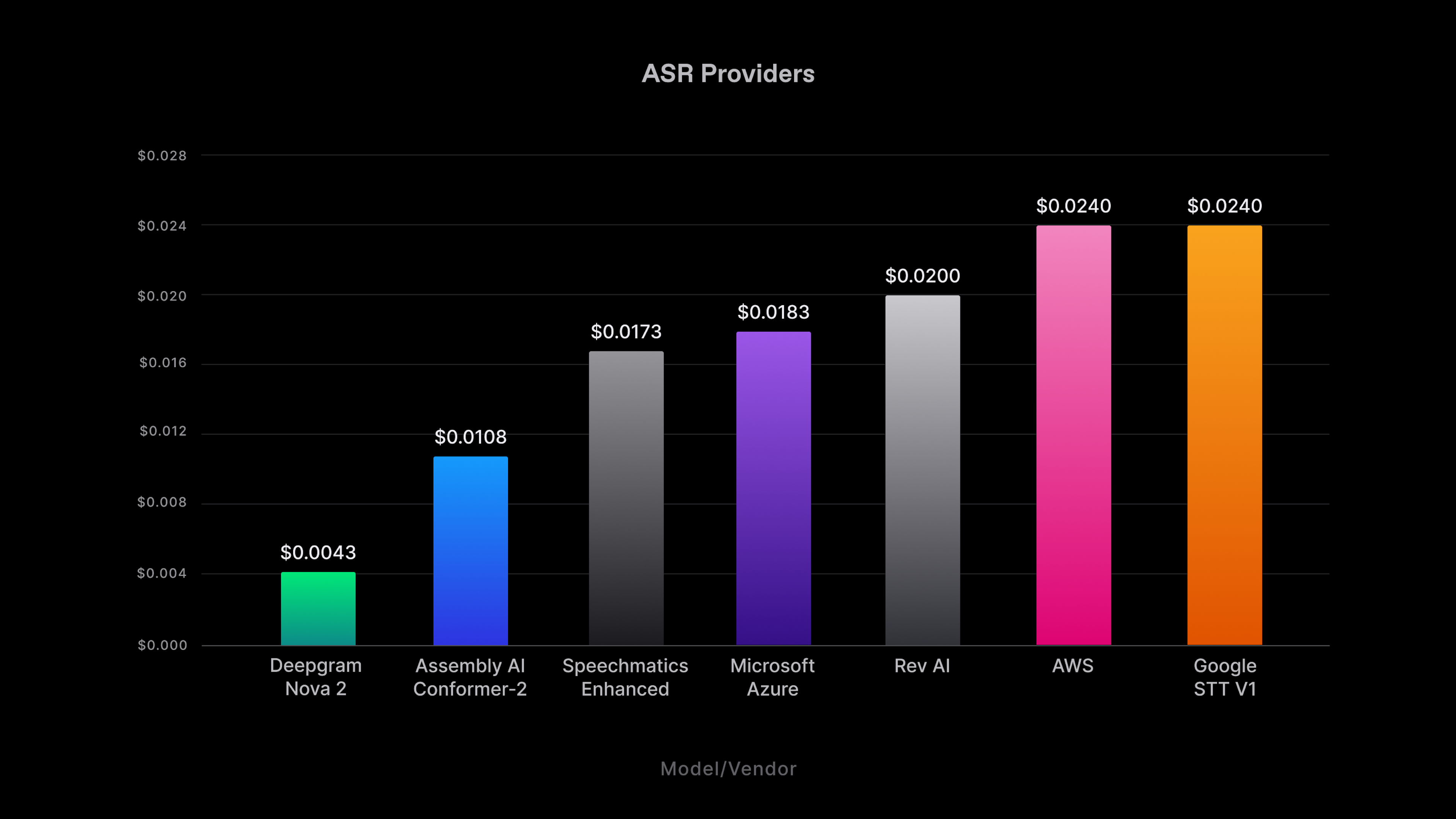
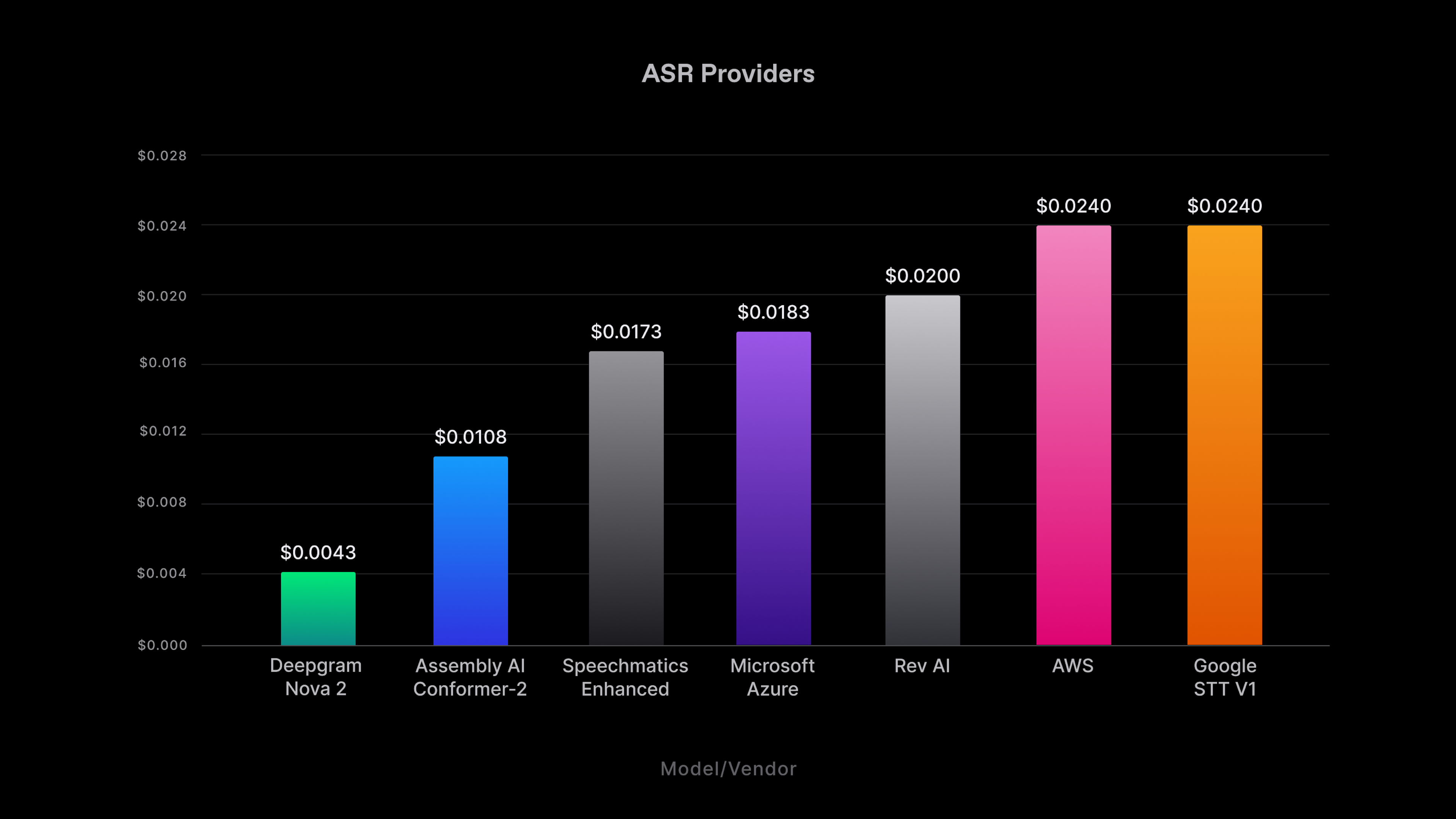
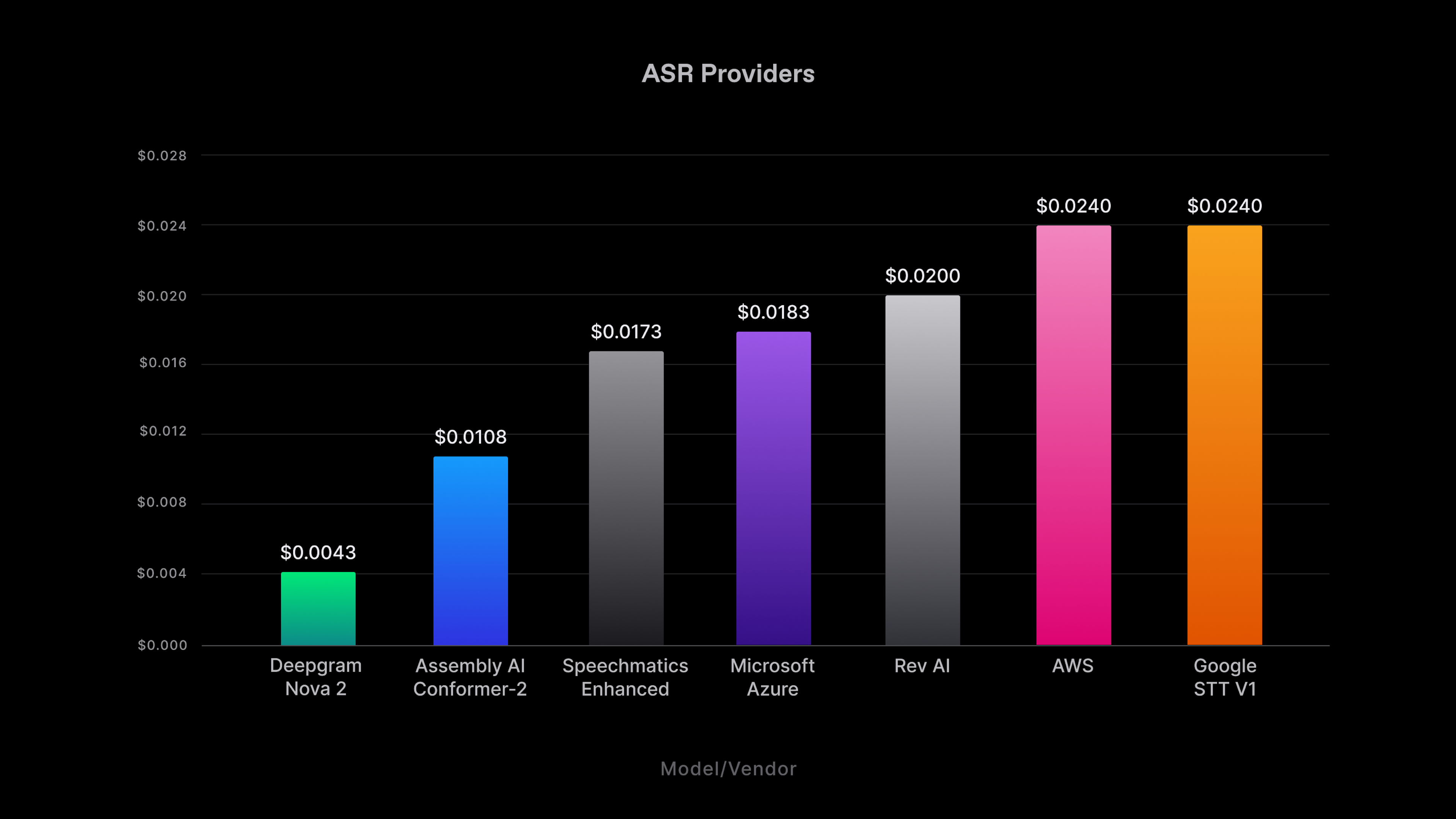
Nova-2 Custom Model training
As the AI market has come to recognize, customization can be key for making AI models work for your use case. Deepgram customers can now have a custom trained model created for them using the best foundation available–Nova-2. For customers who work in industries with lots of uncommon vocabulary or specific phrases, Deepgram recommends talking to our team about custom model training. Deepgram can also provide data labeling services, and even create audio to train on to ensure your custom models produce the best results possible, giving you a boost in performance atop Nova-2’s already impressive, out-of-the-box capabilities.
Contact us to learn more and fulfill your custom Nova-2 needs.
Getting Started
We hope you’ll visit our API Playground or sign up to try Deepgram Nova-2 for yourself. Test its performance and validate the accuracy and speed advantages we’ve shared from our benchmark results as you consider which speech-to-text solution is right for you.
To access the model, simply use model=nova-2 in your API calls. To use another language (e.g. Spanish), use model=nova-2&language=es. To learn more, please visit our API Documentation.
Deepgram remains dedicated to transforming our engagement with technology and one another through AI-powered communication. We are convinced that mastering spoken language understanding is essential for realizing AI’s full potential, paving the way for a future where human-computer exchanges rooted in natural language are the de facto way computing works. Equipped with top-of-the-line STT technology from models such as Deepgram Nova-2, we edge nearer to making this vision a reality.
With this general availability release, Deepgram is proud to have improved the accuracy of its best-performing models by more than 30% in 2023 alone. But rest assured, our work is never done. We’re excited about continuing to push the state-of-the-art further in speech AI through the end of this year and beyond. Stay tuned for more exciting announcements coming soon!
Footnotes
[1] To compare speech-to-text models accurately and obtain an objective score that corresponds to people's preferences, we first ensured that the output of each model followed the same formatting rules by running it through Deepgram's text and style guide normalizer.
[2] Human preference testing was done with OpenAI Whisper (large-v2) prior to their v3 release on November 6, 2023.
If you have any feedback about this post, or anything else around Deepgram, we'd love to hear from you. Please let us know in our GitHub discussions or contact us to talk to one of our product experts for more information today.
Unlock language AI at scale with an API call.
Get conversational intelligence with transcription and understanding on the world's best speech AI platform.