Challenging LLMs: An in-depth look at Text-to-Speech AI
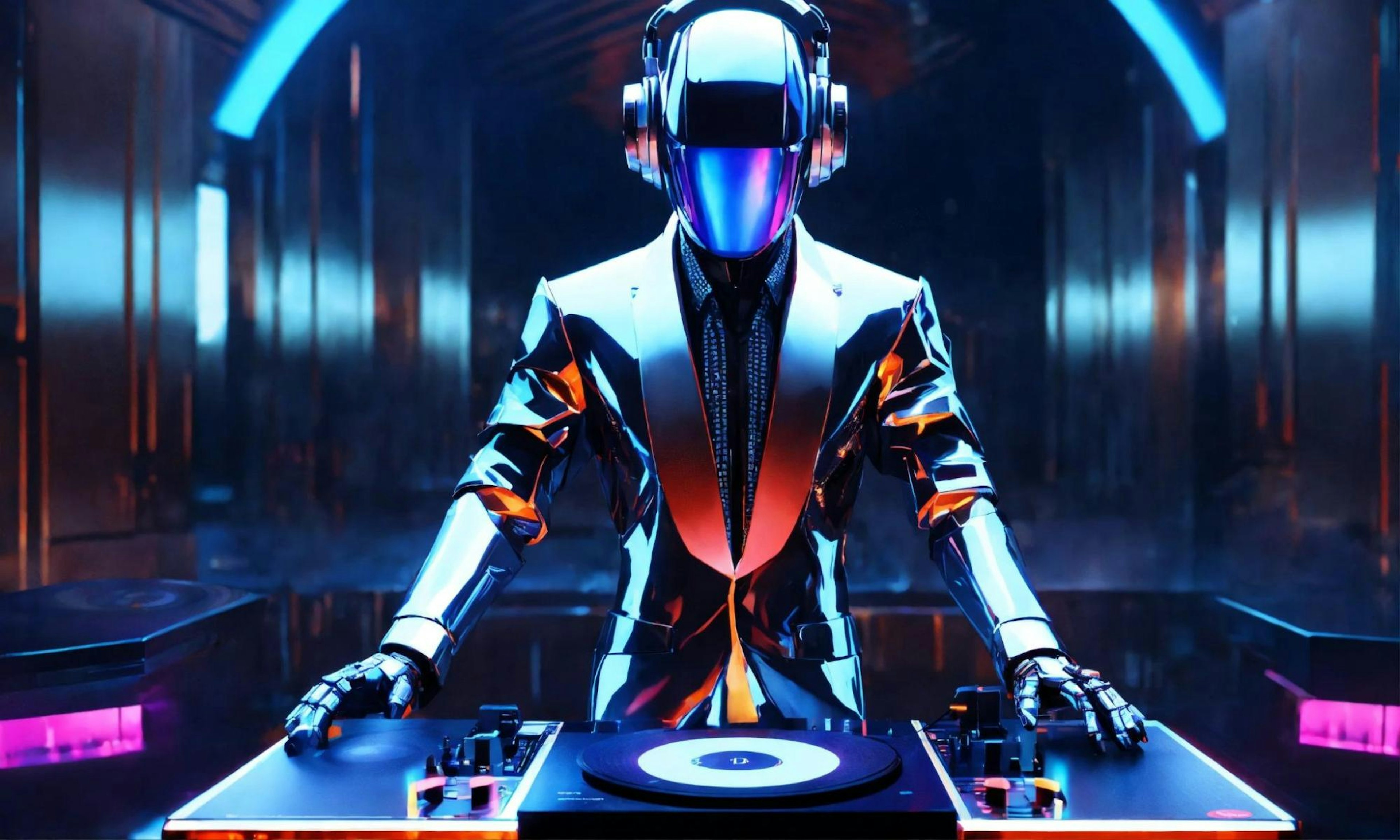
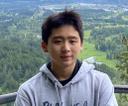
Text-to-Speech (TTS) technology, a marvel of artificial intelligence, has come a long way, transforming the way we interact with machines and enriching the user experience across various platforms. The journey from robotic voices to near-human speech synthesis reflects the rapid advancements in this domain. Today, with models like VALL-E, Google’s Audio LM, Spear TTS, and WhisperSpeech, we are witnessing a revolution in speech technology. This article delves into the contemporary state of TTS models, explores the trajectory of their development, and gazes into the potential future of this exciting technology.
The Current State of TTS Models
The modern-day TTS models have reached a level of sophistication where they can generate audio that is almost indistinguishable from human speech. The state-of-the-art models are characterized by their ability to produce natural-sounding synthetic voices with emotions, pauses, and realistic tone. The leader in the TTS speech in terms of quality is no doubt ElevenLabs. With their proprietary model, anyone can upload minutes of audio, and ElevenLabs’ models will produce voices indistinguishable from the original. Additionally, their models support up to 30 languages in terms of speech synthesis. However, there are still major roadblocks in the TTS fields that must be recognized and addressed.
A Brief History of Modern TTS
The development of Text-to-Speech (TTS) technology over the past decade is a story of remarkable transformation, marked by key innovations and paradigm shifts that have progressively shaped its current state.
Siri’s Inception and Pre-Transformer Era
The journey into modern TTS began with the early appearance and popularization of smartphone assistants, when Apple introduced Siri with the iPhone 4S. Siri’s initial voice was crafted using advanced concatenative speech synthesis, or sometimes referred to as “Speech-to-Speech” (STS). This technique involved stitching together small, pre-recorded speech segments without the use of machine learning techniques.
While it sufficed for its needs at the time, it often resulted in speech that lacked the natural fluidity and intonation of human conversation. During this period, TTS was primarily characterized by two methods: the mechanical-sounding concatenative synthesis and the more flexible but still limited parametric synthesis, as seen in systems like eSpeak. In 2023, we can all agree that Siri’s voice on iPhones aren’t the best in the field (as well as its intelligence).
Breakthrough with WaveNet and the Rise of Transformers
The real breakthrough in TTS came in 2016 with Google’s introduction of WaveNet, a deep neural network capable of generating more natural and human-like speech by directly modeling the raw audio waveforms. WaveNet’s ability to produce speech that closely resembled human voice quality set a new benchmark in the field.
Subsequent to WaveNet, another significant advancement was the adoption of transformer models in TTS. Transformers, initially designed for natural language processing tasks, brought about a revolutionary change in handling long-range dependencies in speech, leading to improvements in prosody and overall speech quality. This innovation led to the development of models like Tacotron 2 around 2020, which further refined the naturalness and expressiveness of synthetic speech.
The Current Landscape
In recent years, the TTS landscape has been enriched by a variety of sophisticated models:
VITS (2021): An end-to-end generative TTS model combining variational autoencoders (VAEs) with GANs (Generative Adversarial Networks). VITS stands out for its ability to produce diverse and expressive voice synthesis, capturing emotional tones and nuances in speech.
Google’s Audio LM (2021): This model represented a leap in integrating natural language understanding with speech synthesis. Audio LM’s large-scale training on diverse datasets allowed it to capture a wide range of speech nuances, further bridging the gap between synthetic and human speech.
VALL-E (2022): Introduced by Microsoft, VALL-E demonstrated remarkable capabilities in synthesizing high-quality speech from minimal samples of a target speaker’s voice, thanks to its encoder-decoder architecture. It emphasized personalized voice synthesis with high fidelity.
Spear TTS (2022): Known for its efficiency and light computational footprint, Spear TTS provided a solution for systems with limited resources without compromising on voice quality.
TorToiSe TTS (2023): This model gained recognition for its multi-speaker framework, leveraging both diffusion models and autoregressive decoders to produce fluid and natural speech patterns. However, akin to its name, the speech generation process requires much longer to complete compared to other models with lower quality outputs.
Sound Storm (2023): It addressed the challenge of synthesizing high-quality speech in noisy environments, an essential feature for voice assistants and telecommunication systems.
Challenges in Modern TTS
Although there are significant advancements made in the last decade or so in Text-to-Speech technologies, there are of course, many challenges and drawbacks: TTS is still at its infancy, along with any other generative AI technologies.
From a Consumer’s Perspective
Despite a massive leap from the 2010s, the quality of text-to-speech technologies is still far from perfect. For the consumer, there are vast number of options available ranging from their quality, ease of use, scalability, and accessibility. From the built-in text to speech function on TikTok to OpenAI’s Whisper API, the options for the consumer is endless.
The evolution of Text-to-Speech (TTS) technology, while impressive, faces several challenges that impact its efficacy and acceptance among users. These challenges can be broadly categorized into issues related to prosody, emotional range, contextual understanding, and pronunciation.
Prosody Issues in TTS
Prosody, which encompasses the rhythm, stress, and intonation of speech, is a critical aspect of natural-sounding language. One of the main challenges TTS faces is replicating the natural prosody of human speech. Often, synthetic speech either sounds too monotonous or unnaturally paced, lacking the fluidity and expressiveness of natural speech.
This issue can significantly impact user engagement, as monotonous speech can be tedious to listen to, and unnatural pacing can hinder comprehension. Even some of the best real-time text to speech readers in audiobook platforms or plain article readers still suffers significantly from this issue.
While advancements in deep learning have improved prosody in TTS, achieving a level that consistently matches human speech remains a challenge. The complexity lies in the subtleties of human speech patterns, which are influenced by a multitude of factors like emotions, context, and individual speaking styles.
Emotional Range and Expressiveness
Another notable challenge is the limited emotional range of TTS systems, or the exaggeration of it. Human communication is rich in emotional nuances, and replicating this in synthetic speech is a formidable task. Current TTS technologies, despite being able to convey basic emotions, often fall short in expressing complex emotional states and subtle variations in tone. This limitation becomes particularly evident in applications like audiobooks, virtual assistants, and customer service bots, where emotional expressiveness is crucial for creating engaging and relatable interactions.
The development of more advanced algorithms capable of capturing and reproducing a wider range of human emotions in speech is essential for enhancing the user experience and making TTS technology more versatile and effective.
Even with some of the highest quality text to speech services, like those of ElevenLab’s, emotional control is handled much more elegantly, but there are still occasional “spikes” in emotion during generated audio when not needed or unusual tone throughout a sentence, especially with the generation of shorter audio.
Contextual Understanding and Pronunciation
Contextual understanding and responsiveness are critical for TTS systems, especially when used in interactive applications. TTS technology often struggles with understanding the context of a conversation, interpreting user intent, and managing dialogue flow.
TTS also struggles with heterophones, which are words that are spelled the same, but pronounced differently depending on the context. For example, the word “polish”, can be pronounced in one manner, translating to a definition of making something smooth or glossy while in a different context, it may mean relating to Poland, which would have a different pronunciation.
This leads to challenges in maintaining coherent and meaningful interactions, particularly in complex or nuanced conversations. Improving natural language processing capabilities and context-aware computing is vital for TTS systems to accurately interpret and respond to user inputs. This includes understanding different accents, dialects, and cultural nuances, which are integral to effective communication.
From a Technical Perspective
On the other side of the technology, there are many challenges that researchers are overcoming or hoping to overcome which may not seem apparent to the average consumer.
Balancing Speed and Quality in TTS Systems
A significant technical challenge in TTS is balancing the processing speed and quality of speech synthesis, especially in neural network models. Advanced TTS models like WaveNet and Tacotron, which use deep neural networks for generating lifelike speech, require substantial computational resources. This high computational demand makes it difficult to achieve real-time speech synthesis without compromising quality. For instance, while these models can produce highly realistic speech, their complex architecture often leads to slower processing times, limiting their use in real-time applications such as interactive voice response systems or virtual assistants.
Many advanced models, such as the open source TTS system TorToiSe, incorporates more than one heavy deep learning components. In the case of TorToiSe, it utilizes both a autoregressive and diffusion decoder, which can significantly slow down its speed in real-time applications.
Data Collection and Model Training
The quality of a TTS system heavily depends on the quantity and diversity of the data used to train the model. Collecting a large and varied dataset that includes different accents, dialects, and speaking styles is challenging but essential for creating a versatile and accurate TTS system.
For the consumer, obtaining audio recordings of themselves or whoever they want to clone the voice of may not be cheap or convenient. Requiring hours of sample data is simply unfeasible for the average consumer. For example, ElevenLab’s TTS system only requires a few minutes of audio sample, but the trade-off in performance may be larger than one might expect.
The difficulty of data collection can also arise in the form of quality. Making sure that there are no background noise in the recordings is crucial for generating quality synthetic audio without extraneous artifacts. Or, on the other hand, the consumer may need audio with background noise, and creating these extra “natural” audio pieces may proven to be difficult.
Moreover, the training process itself is resource-intensive and time-consuming, requiring powerful hardware and sophisticated algorithms to effectively learn from the data.
Handling Long Dependency in Speech
Speech is a temporal sequence where past context can influence future pronunciation and intonation. Capturing these long dependencies in speech is a challenge for TTS systems. Traditional neural network architectures may struggle with this aspect, leading to unnatural-sounding speech. The integration of newer architectures like transformers and diffusion models, which are better at handling long-range dependencies, is one approach to addressing this issue.
Long range dependency issues can also arise with longer sentences. Even some of the best quality models tend to struggle with aligning the punctuation in the sentences with appropriate pauses. Without actually “understanding” the context and english like how a human does, it may be extremely difficult for the model to replicate a natural sounding human voice, especially in longer sentences and speeches.
The Road Ahead for Text-to-Speech Technology
As we have explored, the journey of Text-to-Speech (TTS) technology is marked by significant advancements as well as enduring challenges. From its early days of robotic intonations to the latest models capable of near-human speech synthesis, TTS has undeniably revolutionized our interaction with technology. The evolution of models like WaveNet, Tacotron, and VALL-E highlights the remarkable progress in this field. Yet, as we look to the future, several areas require further innovation and development.
The balance between speed and quality in TTS systems remains a critical technical hurdle. While models like TorToiSe demonstrate advanced capabilities, the trade-off in real-time processing speed for quality is a challenge that needs addressing for wider practical application. Furthermore, the complexities involved in data collection and model training, particularly for creating diverse and inclusive speech models, are significant. These models must not only capture a wide range of linguistic nuances but also do so efficiently.
While there are challenges ahead, the possibilities and potential of Text-to-Speech technology are boundless. Its continuous evolution is not just a testament to the advancements in AI and machine learning but also a window into a future where technology speaks in a voice that is increasingly indistinguishable from our own. For a time where Large Language Models (LLM) are one of the most popular fields of machine learning, the potential usabilities for TTS systems is huge. As TTS technology continues to evolve, it promises to open new avenues for creativity and communication in our increasingly digital world.
Note: If you like this content and would like to learn more, click here! If you want to see a completely comprehensive AI Glossary, click here.
Unlock language AI at scale with an API call.
Get conversational intelligence with transcription and understanding on the world's best speech AI platform.