The AI Echo Chamber: Model Collapse & Synthetic Data Risks
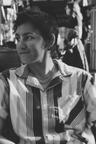
Erin Beck

One of the things that has always made artificial intelligence (AI) cool, at least to us data nerds, is the sheer amount of information required to train these models to reliability. The act of feeding a machine millions or even billions of data points and watching it learn patterns, languages, and sometimes even unexpected insights is awe-inspiring. A vital part of model development is sourcing datasets to train from. This isn't just a matter of accumulating a vast amount of data; it's about the quality and diversity of that data. Accurate, high-quality datasets are essential for training models to respond effectively and reliably to various queries and tasks.
Many companies, like OpenAI, claim to train their models (GPT-3 & GPT-4) on datasets that are 100% human-generated, which creates high-quality outputs to user prompts. By using human-generated content, the models learn the nuances of language, cultural contexts, and even some level of emotional intelligence. This assures that the AI output often feels natural, coherent, and, at times, indistinguishable from something a human might say or write. These outputs may occasionally require secondary fact-checking, but responses have generally been considered reliable.
However, a recent development has created a harrowing twist to the AI narrative. A paper written by researchers from the likes of Cambridge and Oxford uncovered that the large language models (LLMs) behind some of today’s most exciting AI apps may have been trained on “synthetic data” or data generated by other AI. This revelation raises ethical and quality concerns. If an AI model is trained primarily or even partially on synthetic data, it might produce outputs lacking human-generated content's richness and reliability. It could be a case of the blind leading the blind, with AI models reinforcing the limitations or biases inherent in the synthetic data they were trained on.
In this paper, the team coined the phrase “model collapse,” claiming that training models this way will answer user prompts with low-quality outputs. The idea of "model collapse" suggests a sort of unraveling of the machine's learning capabilities, where it fails to produce outputs with the informative or nuanced characteristics we expect. This poses a serious question for the future of AI development. If AI is increasingly trained on synthetic data, we risk creating echo chambers of misinformation or low-quality responses, leading to less helpful and potentially even misleading systems.
Inbreeding, and Model Collapse, and Habsberg AI– Oh My!
Though the paper on model collapse is gaining traction in the AI research community, it was not the first to cover the phenomenon of developing an LLM on synthetic data produced by another model. Another set of researchers called this condition “Model Autophagy Disorder.” Their paper explored AI training itself on a self-consuming loop of content, which determined this training method could result in generative AI tools producing cursed outputs that lack “quality” and “diversity,” two traits most folks expect to find in a generative AI model.
Another researcher in Australia, Jathan Sadowski, dubbed this as Habsburg AI, which he defined as “a system that is so heavily trained on the outputs of other generative AI's that it becomes an inbred mutant, likely with exaggerated, grotesque features.”
So, it’s a concept that has been introduced previously, and researchers are appropriately concerned about the potential risks and effects this practice could have on the AI industry as a whole.
Why Shouldn’t AI Eat Itself?
We have already covered a bit about what AI eating itself looks like, but we have yet to go over the adverse implications of it or what AI experts suspect would follow as a result. In an essay released by Ryan Wang on August 26th, 2023, he discusses the potential consequences of the AI development race and helpfully lists them from most likely to least likely, but we will help break some of those down here too.
Hallucinations
AI hallucinations happen when a model generates a confident response that cannot be or appears not to be justified by its training data. We just saw this occur recently when Snapchat’s My AI bot posted to users’ stories independently without prompting; the model then ceased to interact with users altogether—Snapchat referred to this as a “glitch” when it was actually a signal of taking the training wheels off too soon.
Machine scale security attacks
As much as AI can be helpful in developing state-of-the-art cybersecurity products, it can also be employed to do the exact opposite, which it already has. Things like ChatGPT are allegedly becoming cybercriminals’ favorite digital sidekicks, assisting in developing more aggressive and intelligent attacks using malware generation and polymorphic malware techniques.
Algorithmic biases
Developing a model without bias is a bit of a Sisyphean task; to have a genuinely unbiased LLM, it has to be trained on content that lacks bias, so if models are consuming information and data from the open internet, including data from other models, it will likely express inherent biases. The thing is, all datasets have their biases.
In his essay, Wang describes these three concerns as “most likely” to occur due to AI consuming its own outputs during training. However, he listed out more extreme and less likely scenarios that are worth mentioning, if not just for speculative fun, but because they are thought-provoking at the very least.
It’s important to think in terms of longevity, not just the immediate effects that are playing out before our eyes. Though these scenarios may be uncomfortable to ponder, considering their eventual probability now could be precisely what prevents them from becoming real-world problems that require laborious solutions.
Loss of human innovation and creativity
With AI generating several types of content, it’s only reasonable to think people will embody the mindset of “work smarter, not harder,” which raises the concern of individual innovation and creativity. It also begs the question of who really owns the intellectual property at that point.
Extinction risks
Extinction risk is perhaps the most told tale of AI mishaps. We have seen it across various cinematic expressions, and it is perhaps the most pushed theory by AI skeptics and curmudgeons. Though this is unlikely, the Center for AI Safety released a statement—signed by 350 leading AI experts—urging global participation to mitigate the risk of AI-caused human extinction. This statement followed an open letter from the Future of Life Institute that called for a pause on AI research in March of 2023 and proposed similar sentiments about potential threats to life.
AI overlords and authoritarianism
Concerns about AI becoming the operating authority of the world is among the more farfetched scenarios when it comes to AI consuming itself, according to Wang. It is his belief that if this were to happen, we would likely witness AI-wars or wars between different models. Major corporations with insight access would likely be able to impede individual freedoms and rights as a direct result.
Public Information Scarcity, Reality or Fiction?
It’s hard to say for sure if AI content born of an incestuous model would ultimately lead to a scarcity of public information, but it isn’t impossible. With AI scouring the web and crawling news publications for information and data, it is reasonable to believe that reputable organizations will inevitably start to paywall content with more ferocity than we have ever seen.
Inherently, this isn’t too much of a concern at present, but in the future, especially for people experiencing lower socioeconomic status, paywalls will lead to a sort of digital dark age when it comes to obtaining global and local news. This would further marginalize already overlooked communities and remove their right to make informed decisions personally, politically, or otherwise.
On the flip side, there are companies dedicated to building AI models that fight this exact concern. In the past, false information that was developed by AI was referred to as “deep fakes,” and many startups have developed AI models to detect them. Here are some startups and companies working in that space:
Reality Defender — Reality Defender provides enterprise-grade deepfake detection and protection solutions to help businesses and organizations identify and combat the threat of deepfake content.
DeepMedia — DeepMedia is an AI communication company that uses proprietary data and patented AI algorithms to power products like Universal Translation and DeepFake/AI Detection.
Intel — Intel has developed a real-time deepfake detector called FakeCatcher that analyzes features like facial blood flow in video pixels to quickly and accurately identify whether a video is real or fake.
WeVerify — WeVerify is a project that utilizes technology and artificial intelligence to verify and counter disinformation, including deepfakes, by providing tools and solutions for journalists, fact-checkers, and researchers.
To make a long story short, AI has come a very long way in an unbelievably short amount of time, and with these developments, there is a certain level of moral responsibility to be had. Companies developing LLMs must carefully select their training datasets and utilize responsible AI development practices, and a general rule of thumb should always be to diversify. By prioritizing diversity in the research, development, and implementation stages, models will, without a doubt, become more reliable and well-rounded.