Exposing Voice Cloning: How Synthetic Voices Shape Futures
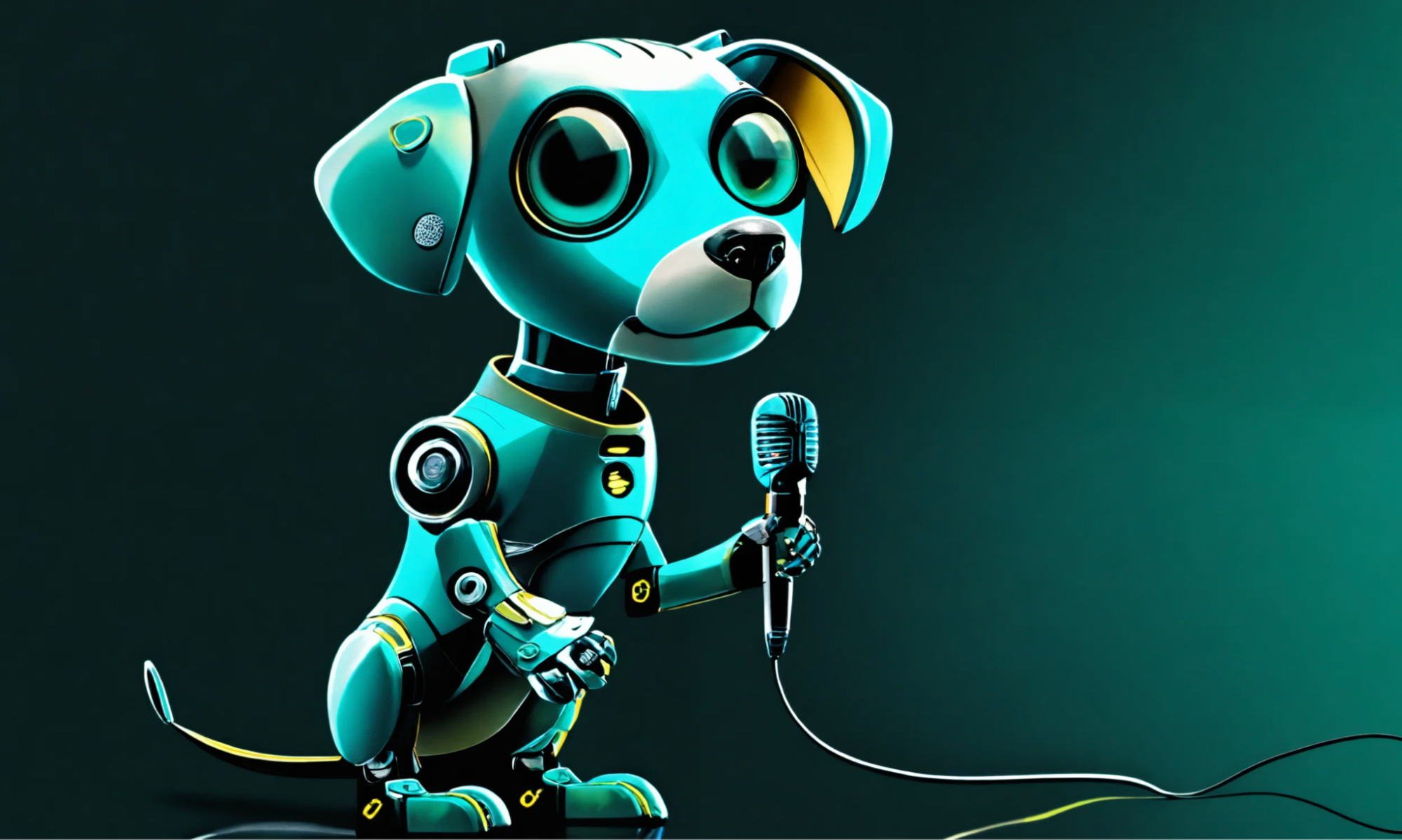
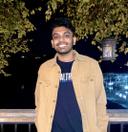
Imagine being able to capture and use the voice of your favorite celebrity or historical figure recreated with remarkable accuracy relative to the original. This is the promise of voice cloning technology, a rapidly evolving field that is pushing the boundaries of what was once thought impossible. With the rise of artificial intelligence and advancements in machine learning, we now have the ability to synthesize human-like voices from relatively small samples of audio data. This technology has the potential to revolutionize industries such as entertainment, advertising, marketing, etc.
At its core, voice cloning involves the use of neural networks to analyze and model the unique characteristics of a person's voice, including pitch, timbre, prosody, and intonation patterns. By training these models on recordings of the target voice, researchers can create highly realistic synthetic voices that can be used to generate new speech in that person's voice. The applications of voice cloning are vast and varied. Imagine being able to listen to an audiobook narrated by your favorite actor or having a virtual assistant that sounds just like a certain character or persona that matches the use case well. The technology could also be used to restore the voices of those who have lost their ability to speak due to illness or injury, providing a new level of independence and communication.
However, as with any powerful technology, voice cloning also raises significant ethical and legal concerns. The potential for misuse, such as creating deepfake audios for malicious intent, is a real threat that must be addressed. Additionally, questions around privacy, consent, and the ownership of one's voice have yet to be fully resolved. In this article, we will delve into the fascinating world of voice cloning, exploring the cutting-edge techniques and model architectures that make it possible. We'll examine the work of leading companies and researchers in the field, and discuss the ethical implications and challenges that must be navigated as this technology continues to evolve.
What is Voice Cloning
As stated before, voice cloning, also known as voice synthesis or speech synthesis, is the process of creating an artificial voice that mimics the characteristics of a real person's voice. By training deep neural networks on recordings of the target voice, researchers can generate highly realistic synthetic speech that closely resembles the original speaker.
The concept of synthesizing human-like speech has been a longstanding goal in the field of computer science and computational linguistics. Early attempts at speech synthesis date back to the 1700s, with the development of mechanical devices that could produce rudimentary vowel sounds. However, it wasn't until the mid-20th century that significant progress was made with the advent of more contemporary digital signal processing techniques. Over the past decade, the rise of deep learning and neural network architectures has revolutionized the field of voice cloning. Powerful models like WaveNet, Google’s Tacotron, and Transformer-based architectures have enabled the generation of high-fidelity synthetic speech that closely matches the nuances and expressiveness of human voices.
Voice cloning technology has a wide range of applications across various industries and domains. One of the most prominent use cases is in the entertainment industry, where synthetic voices can be used for audiobook narration, video game character voice overs, and even virtual celebrity appearances. Another significant application is in the field of accessibility, where voice cloning can be used to restore the ability to communicate for individuals who have lost their voices due to illness or injury. Voice cloning is also being utilized in areas such as virtual assistants, where synthetic voices can be tailored to specific personas or characters, enhancing the user experience and creating a more natural and engaging interaction.
How Voice Cloning Works
The voice cloning process typically involves several key steps. First, a large dataset of audio recordings from the target speaker is collected and preprocessed using various techniques. Effective voice cloning requires a large amount of high-quality audio data from the target speaker to train the machine learning models accurately. For cloning a specific individual's voice, you typically need several hours (5-10 hours or more) of high-quality audio recordings from that person. This data should cover a diverse range of speaking styles, emotions, and contexts to ensure the synthetic voice can capture the full range of the speaker's vocal profile. Data preparation, including tasks like noise removal, normalization, and speaker diarization, is also crucial for achieving optimal results.
These recordings are then analyzed using signal processing techniques to extract relevant aural features that capture the unique characteristics of the speaker's voice, such as pitch, timbre, and prosody. This signal processing “pipeline” so to speak involves leveraging techniques such as Fourier analysis, cepstral analysis, and linear predictive coding (LPC) to decompose the audio signal into its spectral and temporal components. This in turn enables the extraction of features like pitch, formants, and spectral envelopes.
Next, these extracted features are used to train machine learning models, specifically deep neural networks, to learn the complex patterns and relationships that define the target speaker's voice. Various neural network architectures (WaveNet, Transformer-based models, etc) have been developed specifically for this task. Specialized neural network architectures for voice cloning each have their own strengths and trade-offs. WaveNet, introduced by DeepMind, uses dilated convolutional neural networks to generate raw audio waveforms. Tacotron, developed by Google, employs an encoder-decoder architecture with attention mechanisms to generate mel spectrograms, which are then converted to audio using a vocoder. Transformer-based models, like Google's Transformer TTS, have also shown promising results in generating high-quality synthetic speech. We’ll dive into these a bit deeper soon. Once the models are trained, they can be used to generate new synthetic speech in the target speaker's voice by providing the desired text input. The model essentially learns to map the input text to the corresponding audio waveform, mimicking the speaker's vocal patterns. This involves a two-step process: first, the text is converted into a sequence of acoustic features or mel spectrograms using the trained model. Then, these acoustic representations are passed through a vocoder, which generates the final audio waveform that resembles the target speaker's voice. Post-processing techniques, such as waveform filtering and signal enhancement, may also be applied to improve the quality and naturalness of the synthesized speech.
Voice Cloning Model Architectures
At the heart of voice cloning technology lie complex deep learning model architectures and pipelines designed to capture aspects of human speech. While various approaches have been explored, several model architectures have emerged as frontrunners in this field. One prominent class of models is the encoder-decoder architecture, which consists of two main components working in tandem to generate outputs. The encoder maps the input text or speech features into a high-dimensional latent representation, while the decoder generates the corresponding output audio waveform or spectrogram from this representation. These models are trained in an end-to-end fashion, with classical variants like Tacotron 2 using recurrent neural networks (RNNs) like LSTMs, and more recent iterations employing transformer-based encoders and decoders. Generative Adversarial Networks (GANs) have also proven to be effective in voice cloning tasks. These models learn to generate realistic speech samples through an adversarial training process, where a generator network attempts to produce synthetic speech indistinguishable from real samples, while a discriminator network tries to differentiate between the two. This adversarial approach helps the generator learn to mimic the target speaker's voice distribution with high fidelity. GAN-based models like VawGAN and NVIDIA's HiFi-GAN combine adversarial training with autoregressive waveform generation. Inspired by their success in natural language processing, transformer architectures have also been adapted for voice cloning, leveraging multi-headed self-attention mechanisms to better capture long-range dependencies in speech signals. Models like Google's Transformer TTS can directly generate high-fidelity waveforms in an end-to-end fashion.
Regardless of the specific model architecture employed, a typical end-to-end voice cloning pipeline involves several key stages mentioned above. It all starts with data collection and preprocessing, where a sufficiently large and diverse corpus of audio recordings from the target speaker is gathered and subjected to various preprocessing steps like noise removal, resampling, segmentation, and speaker diarization to isolate the target voice. The preprocessed audio data and corresponding transcripts are then used to train the chosen deep learning model, with the aim of learning the mapping between text or speech features and the target speaker's unique voice characteristics. Once trained, the model can be used to generate synthetic speech by providing the desired text input, converting it into an acoustic representation such as a mel-spectrogram or waveform, which is then further processed by a vocoder or waveform synthesis module to produce the final audio output resembling the target speaker's voice. Finally, additional post-processing and enhancement techniques may be applied to the generated audio to further refine its quality and naturalness. These can include waveform filtering, spectral enhancement, prosody modeling, and voice conversion algorithms to better match the target speaker's voice characteristics.
Ethics
As impressive as the technical capabilities of voice cloning technology are, they also raise pertinent ethical concerns and challenges that must be addressed. One of the most pressing issues is privacy and security. The ability to generate highly realistic synthetic voices from audio samples raises questions about consent and ownership of one's voice data. In the wrong hands, this technology could be exploited to impersonate individuals without their knowledge or consent, leading to potential fraud, defamation, or other malicious activities. Closely tied to privacy concerns is the potential for misuse through deepfakes – synthetic media created with the intent to deceive. As voice cloning becomes more sophisticated, the risk of producing convincing deepfake audio increases, opening the door for the spread of misinformation, fake news, and even extremist propaganda on a large scale. Combating this threat will require robust detection and authentication methods as well as public awareness and media literacy efforts. Beyond privacy and misuse, there are also concerns around bias and fairness in voice cloning systems. Like many AI technologies, these models can perpetuate societal biases present in their training data, leading to potential discrimination or underrepresentation of certain demographics. Ensuring diverse and inclusive datasets, as well as rigorous testing and auditing processes, will be crucial to mitigating these biases and promoting equitable outcomes.
From a regulatory and legal standpoint, the landscape surrounding voice cloning technology is still largely uncharted territory. Just recently we saw OpenAI and Scarlett Johansson get into a legal beef over the use of her voice for their newest model. Questions around intellectual property rights, data privacy laws, and the potential need for specific regulations governing the use and distribution of synthetic voices remain unanswered. As this technology becomes more prevalent, policymakers and legal experts will need to grapple with these complexities to establish appropriate guidelines and safeguards. Moreover, there are broader philosophical and ethical debates to be had around the implications of being able to recreate and manipulate human voices in such a powerful way. What are the boundaries between artistic expression and violation of personal identity? How might this technology impact our perceptions of authenticity and trust in the digital age?
Who’s Working On Voice Cloning?
Several companies and research groups have more recently started actively working and building in the voice cloning domain. Eleven Labs, a leading provider of advanced voice synthesis solutions, has garnered attention for its state-of-the-art voice cloning models. Their platform allows for customization and personalization of voices, enabling businesses and developers to create unique and immersive experiences for end-users. Character.AI, a company focused on developing human-like virtual agents, has also ventured into voice cloning in a similar fashion. Their models aim to mimic real human emotions, offering a more natural and engaging conversational experience. Resemble.ai, on the other hand, specializes in professional AI voice cloning for various industries, including films, gaming, and marketing. Their model can create high-quality voice clones with as little as three minutes of voice data, making it a popular choice for projects with tight deadlines. Descript, known for its innovative work in audio technology, has also made strides in voice cloning through its research division, Lyrebird. Their solutions enable seamless integration of natural-sounding voices into various applications, from entertainment to accessibility. One company that stands out in terms of their computational efficiency is Cartesia. They have helped pioneer the use of state space models (SSM) by using an SSM architecture for faster inference and training for their voice cloning model. SSMs offer a more efficient and parallelizable approach compared to traditional architectures like Transformers and RNNs. They replace the self-attention mechanism in Transformers with a more lightweight recurrent state transition function. This state transition function can be computed in parallel across all sequence positions, unlike the sequential computations required by RNNs. By avoiding the quadratic complexity of the self-attention operation, SSMs achieve better computational efficiency, especially for longer sequences. As the demand for personalized and interactive experiences continues to grow, these companies are at the forefront of shaping the future of voice synthesis and human-machine interactions, pushing the boundaries of what is possible with AI-generated voices.
Future Applications and Potential
As voice cloning capabilities continue to advance, a myriad of exciting applications are emerging across many domains. In the entertainment and media realms, this technology is poised to reshape how we experience audio content. Voice acting and dubbing stand to be transformed, with the ability to recreate the voices of actors or voice artists with high accuracy. This opens up possibilities for seamless dubbing across languages, or even bringing back the voices of deceased performers to breathe new life into their iconic roles.
For publishers, voice cloning offers an opportunity to create audiobooks with synthetic narration from popular authors or celebrities, providing listeners with an unprecedented sense of immersion and personalized connection to the material. The video game industry also stands to benefit greatly, as developers can now create unique, hyper-realistic voices for game characters that enhance the overall immersive experience. Perhaps one of the most profound applications lies in the accessibility and healthcare spheres. Individuals with speech impairments or those who have lost their voices due to illness or injury could regain their ability to communicate naturally through personalized synthetic voices derived from their own earlier voice recordings. We recently saw Val Kilmer, who lost his voice to throat cancer, have his voice recreated with these models for the blockbuster Top Gun: Maverick.
The potential applications extend into customer service and marketing, where companies could deploy virtual assistants with cloned voices of real customer service agents, providing a more natural and personalized experience. Similarly, targeted advertising campaigns could leverage familiar voices to increase engagement and recall. In the realm of education and language learning, synthetic voices in various languages and accents could revolutionize language tutoring and pronunciation training. Educational content could also be enriched by the cloned voices of famous educators, scientists, or historical figures, making learning more interactive and engaging. Even the field of robotics stands to be impacted, as voice cloning could imbue robots and AI systems with more natural and human-like communication abilities, fostering better interactions and acceptance.As these applications continue to emerge, it will be crucial to address ethical concerns around privacy, consent, and the prevention of misuse. Continual vigilance will be necessary to ensure responsible usage.
The possibilities unleashed by voice cloning technology are vast and compelling, spanning entertainment, accessibility, education, customer service, and even enhancing our interactions with AI systems and robots. From resurrecting the voices of legendary performers to restoring communication for those who have lost their ability to speak, the applications could yield profound personal, societal, and creative benefits. Approached responsibly and conscientiously, this powerful technology could open new mediums of expression, connection and understanding.
Note: If you like this content and would like to learn more, click here! If you want to see a completely comprehensive AI Glossary, click here.
Unlock language AI at scale with an API call.
Get conversational intelligence with transcription and understanding on the world's best speech AI platform.