Why AI is the Future of Medical Documentation
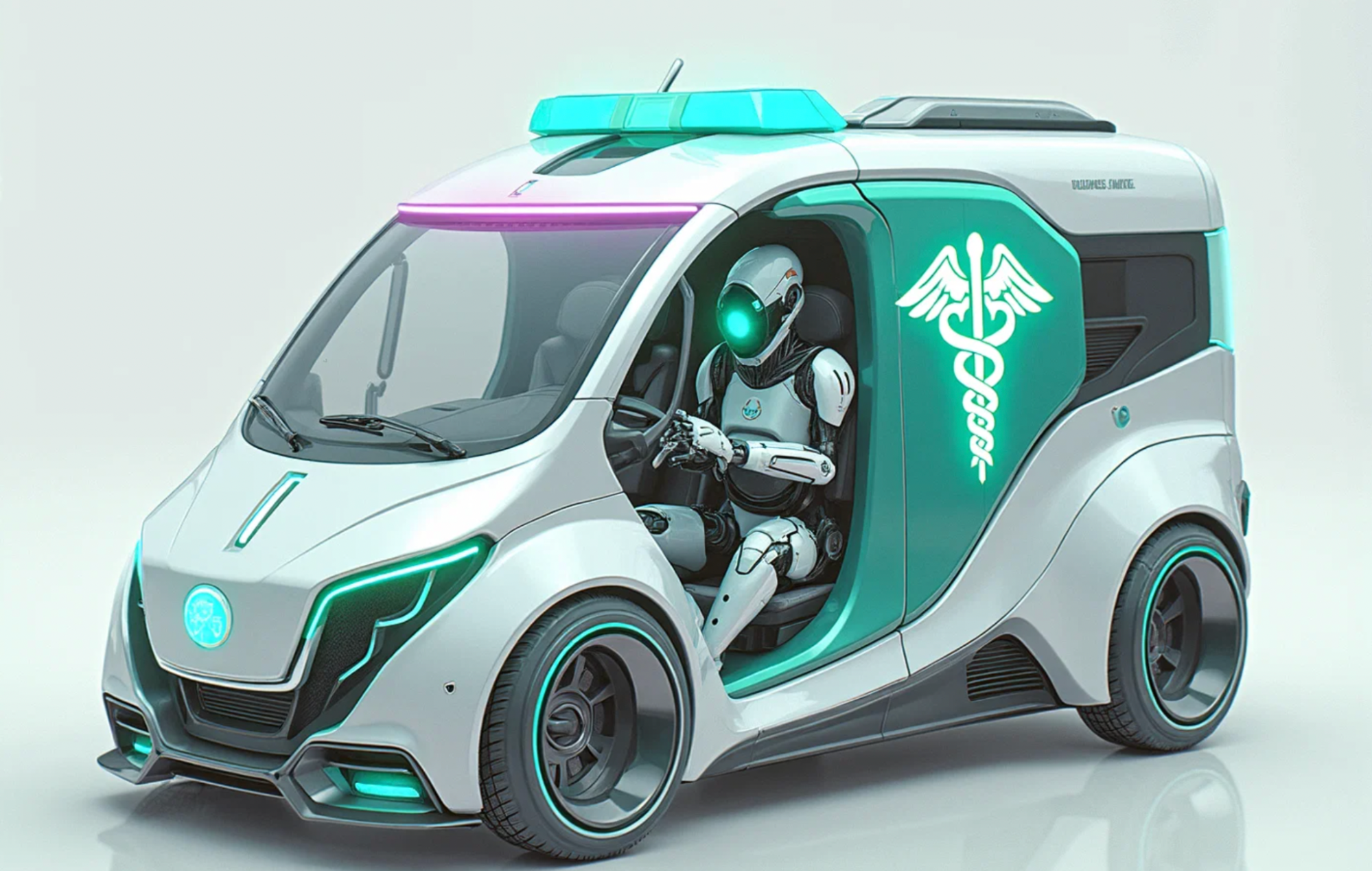
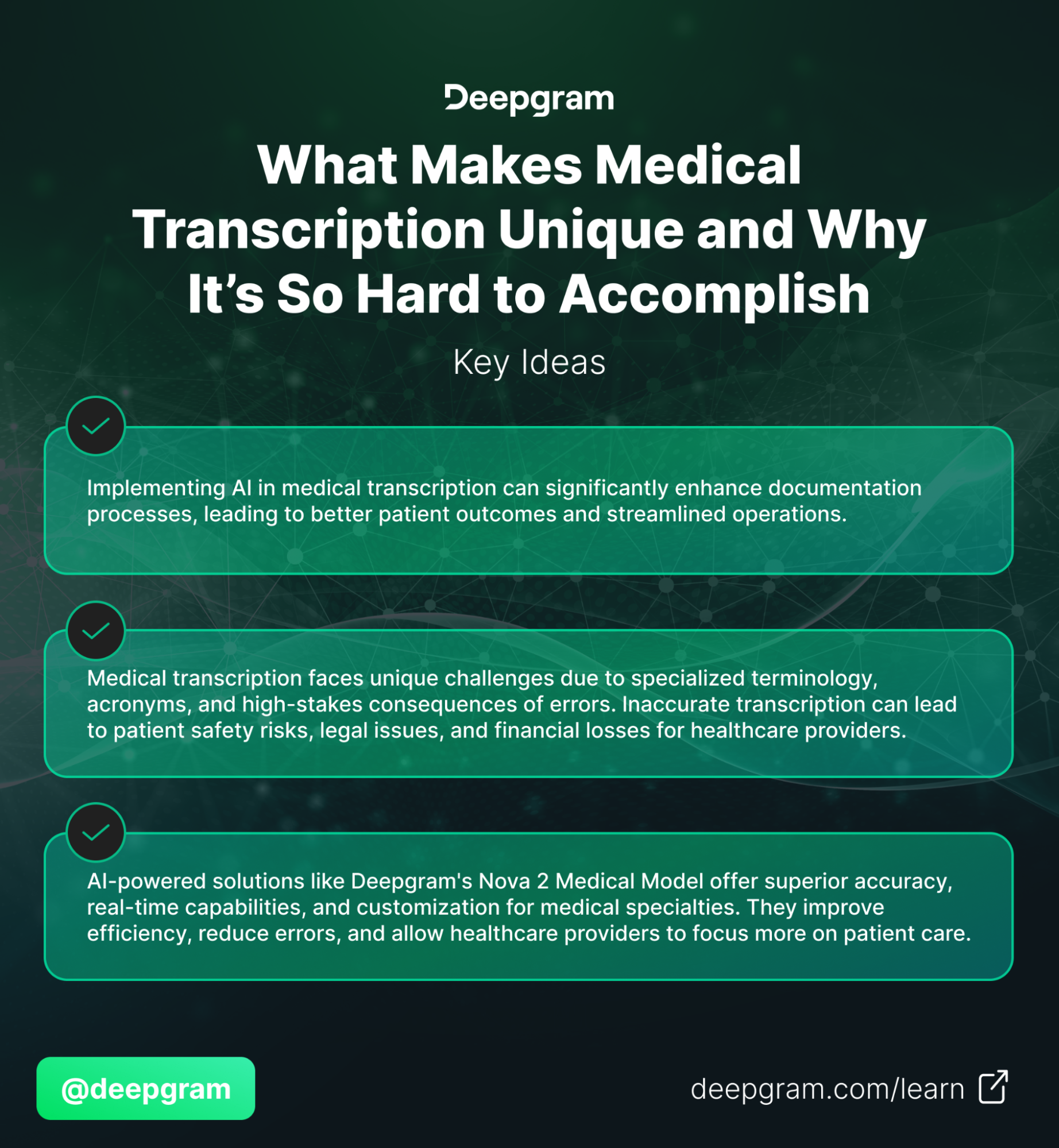
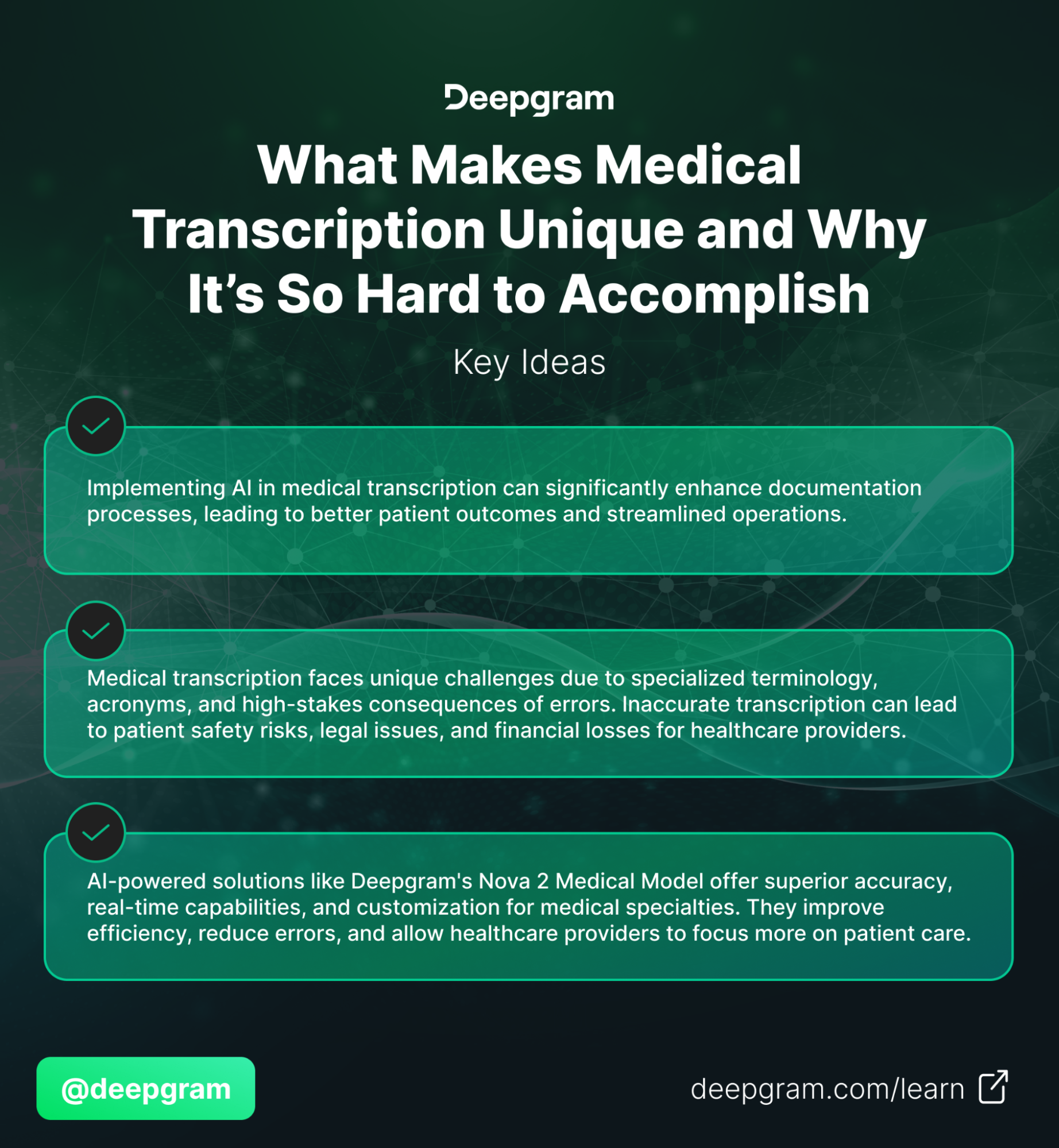
There are many moving parts in the healthcare industry, and doctors and other medical professionals now have roles beyond caring for patients. They also have to manage and make decisions. For example, doctors must decide whether to dictate or take real-time notes during patient visits. These decisions affect workflow, patient record quality, and direct patient interaction time.
The added workload increases the chances of errors in various patient care areas, such as medication administration, treatment planning, and communication between healthcare providers. These are problems with documentation, which is an important part of patient care.
Many doctors use AI-powered medical transcription tools to help with the lack of documentation. However, the medical field has its own language problems that make traditional Automatic Speech Recognition (ASR) models very limited.
This is because regular ASR models are made for everyday language and often have trouble with medical vocabulary. This is not a small issue in healthcare, where one wrong, misinterpreted word can affect patient safety.
In this article, you will learn:
The specific challenges that make medical transcription uniquely complex (specialized terminology, abbreviations, and varied speaking environments)
The critical impact of inaccurate medical transcription on patient safety, legal compliance, and healthcare finances
The key features and benefits of AI-powered medical transcription, including improved accuracy, real-time capabilities, and customization options
How Deepgram's Nova 2 Medical Model is solving these problems and changing the way medical documentation techniques
Ready? Let’s jump right in! 🚀
Challenges in Medical Transcription
Medical language is very complicated, which makes it hard for regular transcription tools to correctly understand specialized terms, abbreviations, and details that depend on the context. Because of this, there may be mistakes in the medical records, which could seriously affect the patients.
Let’s take a closer look at some of these problems.
Specialized Medical Terminology
Medical language is a sophisticated mix of specialized English, Latin, and Greek roots, terms, abbreviations, and acronyms. While this vocabulary is precise for healthcare professionals, it poses significant challenges for transcription, especially for automated systems (AI, robotics, assistants, etc.). For example:
Anatomical terms (e.g., "gastrocnemius", "sternocleidomastoid")
Disease names (e.g., "plasmodium vivax")
Drug names (e.g., "acetaminophen", "esomeprazole")
Medical procedures (e.g., "thoracentesis", "cholecystectomy")
The sheer volume and complexity of these terms can be overwhelming, even for experienced human transcribers.
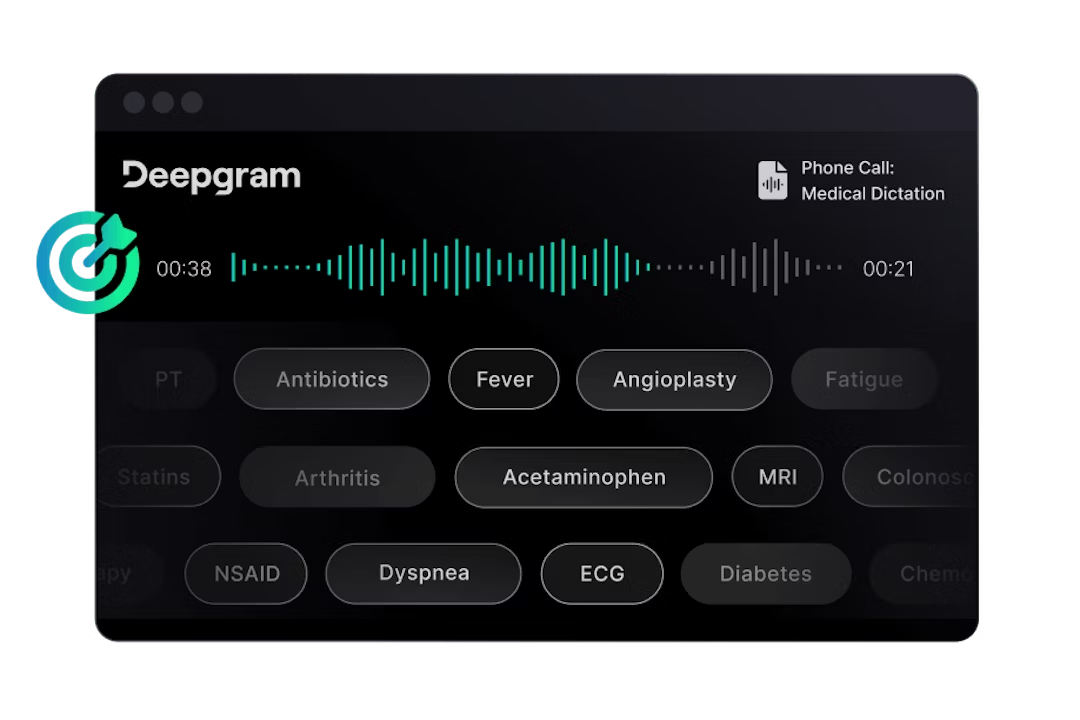
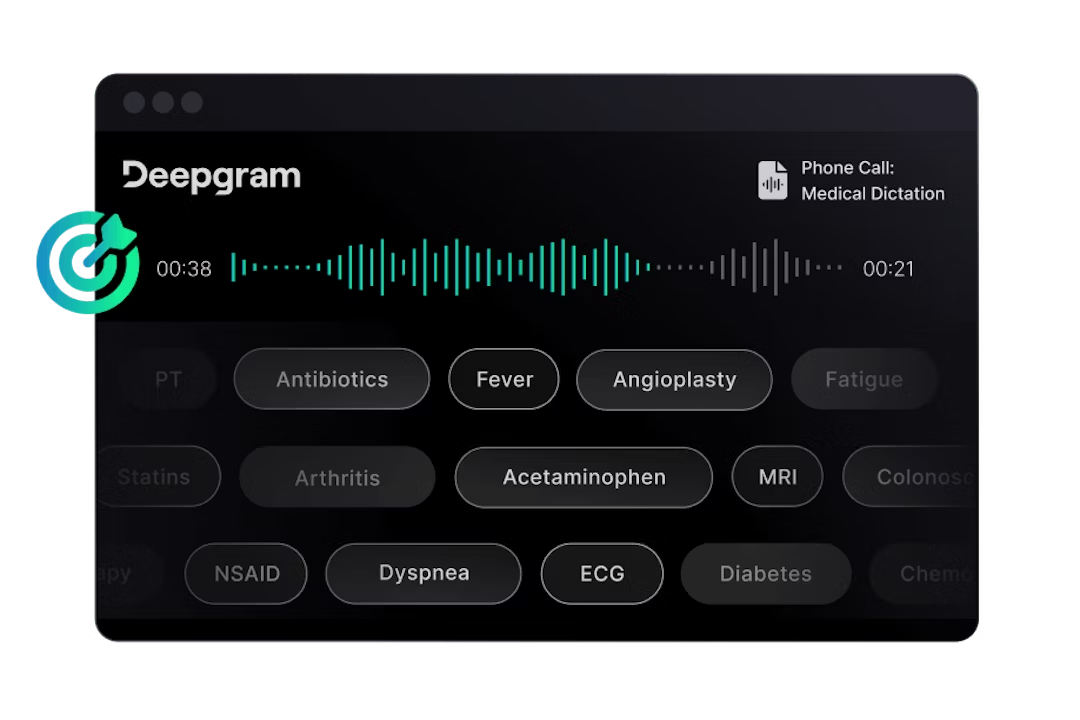
These words are rarely used outside medical contexts, meaning they're often underrepresented in the datasets used to train general-purpose speech recognition models. Their limited presence in typical audio datasets makes it challenging for standard ASR systems to accurately recognize and transcribe these terms.
Abbreviations and Acronyms
Medical professionals, especially doctors, are notorious for using abbreviations and acronyms. While these notations are efficient in a healthcare setting, they present transcription challenges like:
Interchangeable Use: Doctors often interchange full terms and acronyms, confusing transcription tools that aren't robust enough to recognize these patterns.
Context-Dependent Acronyms: Different medical specialties may use the same acronym to mean different things, adding another layer of complexity. For instance, the acronym "PD" could mean "Peritoneal Dialysis" in nephrology, "Parkinson's Disease" in neurology, "Personality Disorder" in psychiatry, "Pediatric Dose" in pediatrics, or "Pupillary Distance" in ophthalmology, highlighting the critical need for specialty-specific context in accurate medical transcription.
Lack of Punctuation: In spoken language, there's no clear indication of where an acronym starts or ends, making it difficult for ASR systems to correctly identify and expand these abbreviations.
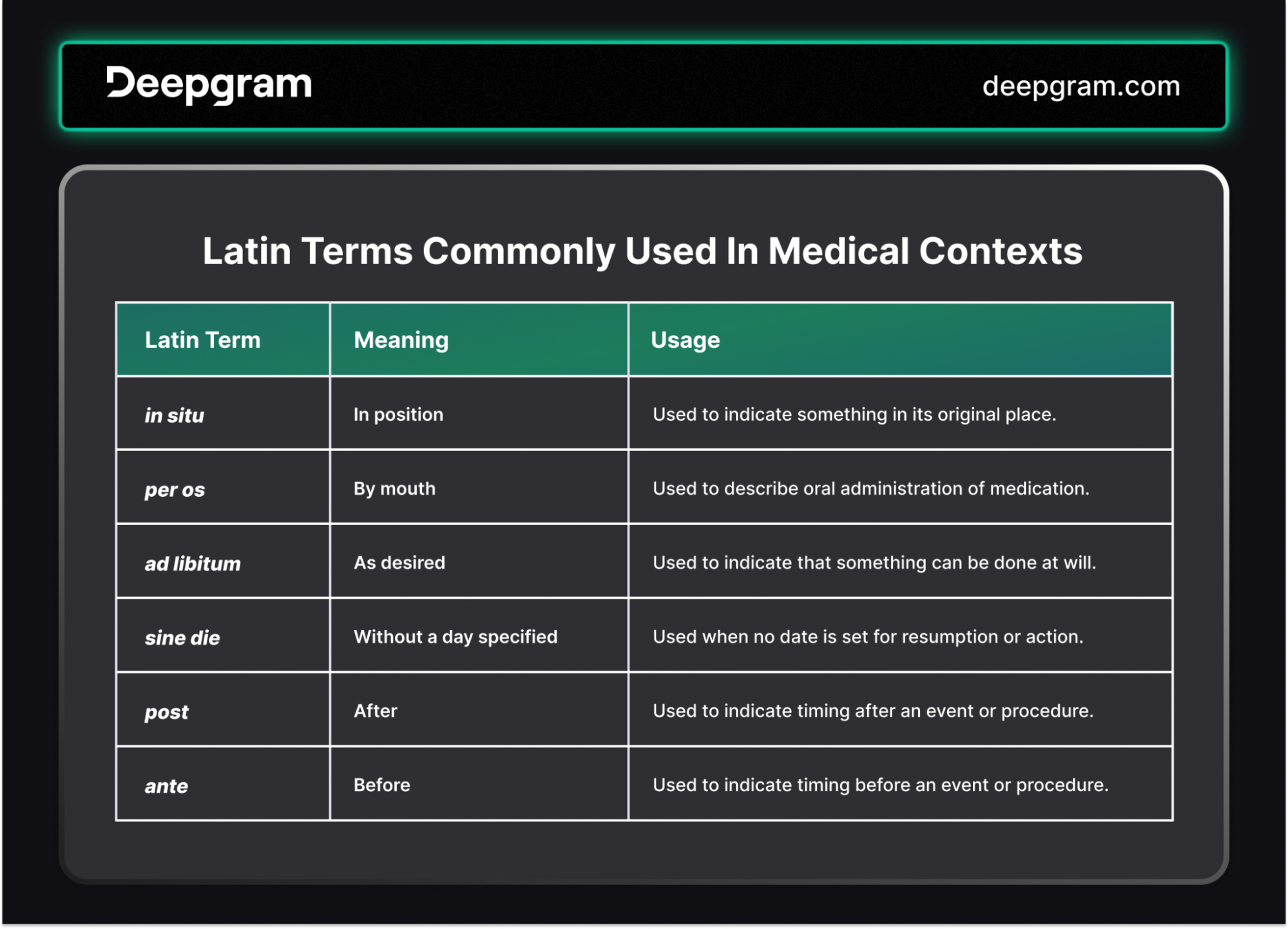
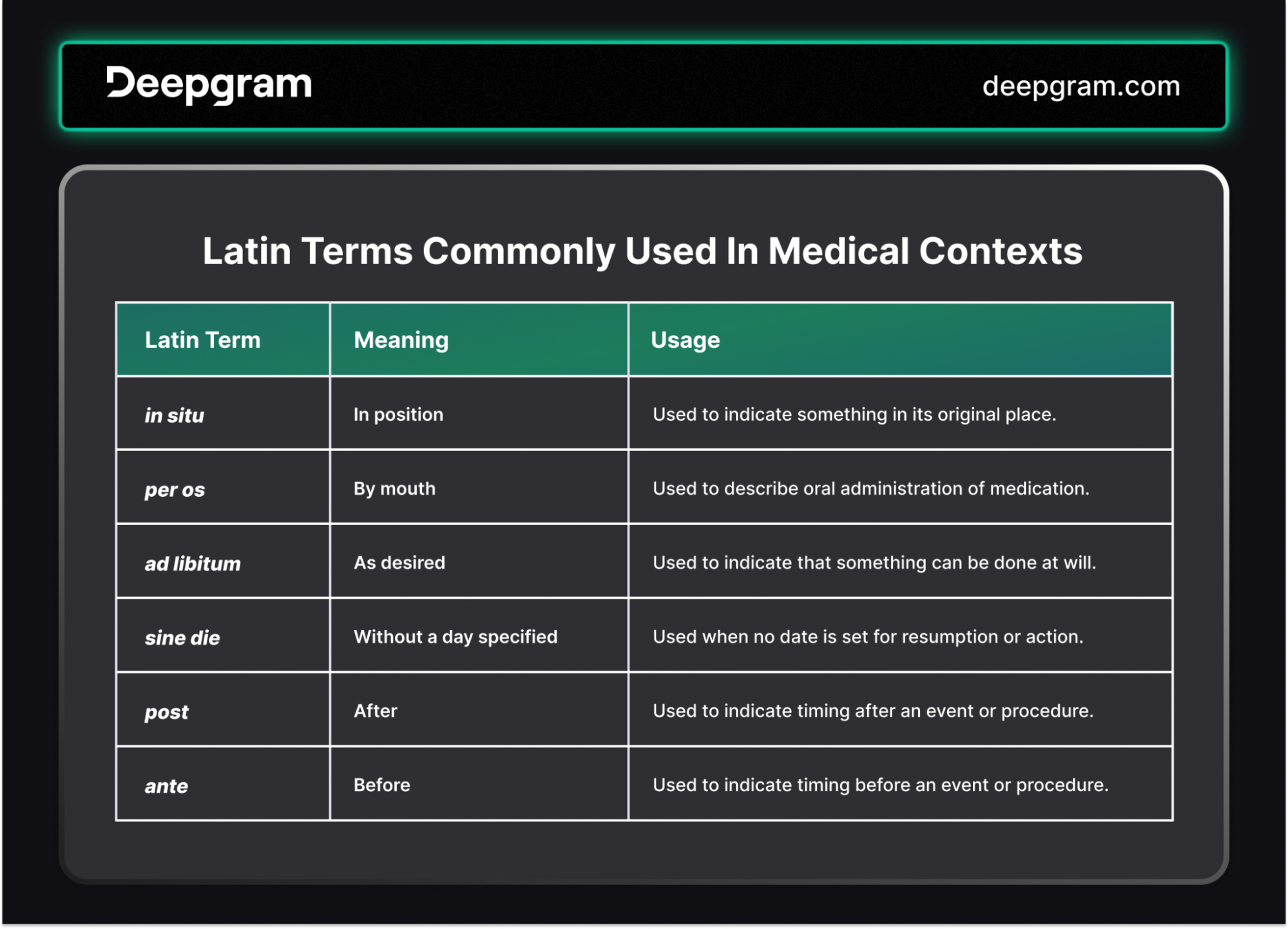
Varied Accents and Speaking Styles
The diversity of accents and speaking styles in medical settings adds another layer of complexity to transcription, including:
Diverse Medical Community: Healthcare providers come from diverse backgrounds, bringing various accents and speech patterns.
Patient Diversity: Patients and their families may speak with regional or non-native English accents, which can be challenging for ASR systems to interpret accurately.
Speaking Speed: Medical professionals sometimes speak quickly, especially in high-pressure situations, which can lead to missed words or misinterpretations.
Mumbling and Unclear Speech: Fatigue, stress, or the physical act of performing a procedure can lead to mumbled or unclear speech. People also use filler words like ‘umm’ and ‘ah,’ which could be unclear or disrupt the transcription process.
Varying Pronunciations: Medical professionals may have unique ways of pronouncing certain terms that differ from standard pronunciations.
Background Noise and Audio Quality
Medical environments are busy depending on the time of day, patient load, and specific procedures. This increase in background noise can significantly impact audio quality and transcription accuracy.
Beeping monitors, ventilators, and other medical devices create constant background noise. Operating rooms and patient wards often have multiple people speaking simultaneously, which makes isolating and transcribing individual voices challenging. Sudden alarms or overhead announcements can interrupt speech and create gaps in transcription.
Personal Protective Equipment (PPE) like masks can make understanding what people are saying harder. The quality of the recording device and where it is placed can also have a big effect on how clear the sound is, which can make medical transcription even harder to do correctly.
Impact of Inaccurate Medical Transcription on the Healthcare Sector
AI systems are designed to improve people's performance, especially in sensitive areas like healthcare, where small mistakes can have big effects. An inaccurate transcript could cause anything from a small misunderstanding to life-threatening medical errors, making precision and reliability essential when using AI in healthcare.
Those inaccuracies can impact patient safety, erode public trust, and lead to legal and financial issues for healthcare institutions.
Patient Safety Risks
The most critical concern with inaccurate medical transcription is its potential to compromise patient safety and create distrust of AI systems. Transcription errors can lead to a cascade of bad outcomes:
Misdiagnosis: Incorrectly transcribed symptoms or test results can misinterpret a patient's condition, resulting in an incorrect diagnosis. For instance, confusing "hypertension" with "hypotension" could result in a patient receiving medication that dangerously raises their blood pressure instead of lowering it.
Delayed Care: Errors that lead to confusion or misunderstanding of a patient's condition can result in delayed treatment, potentially exacerbating the patient's health issues.
If AI-powered transcription systems make big or repeated mistakes, people might not trust them. This could stop practitioners from using them and new ideas from coming up in healthcare, even if the systems are more accurate and efficient. This is because healthcare providers might not want to rely on AI.
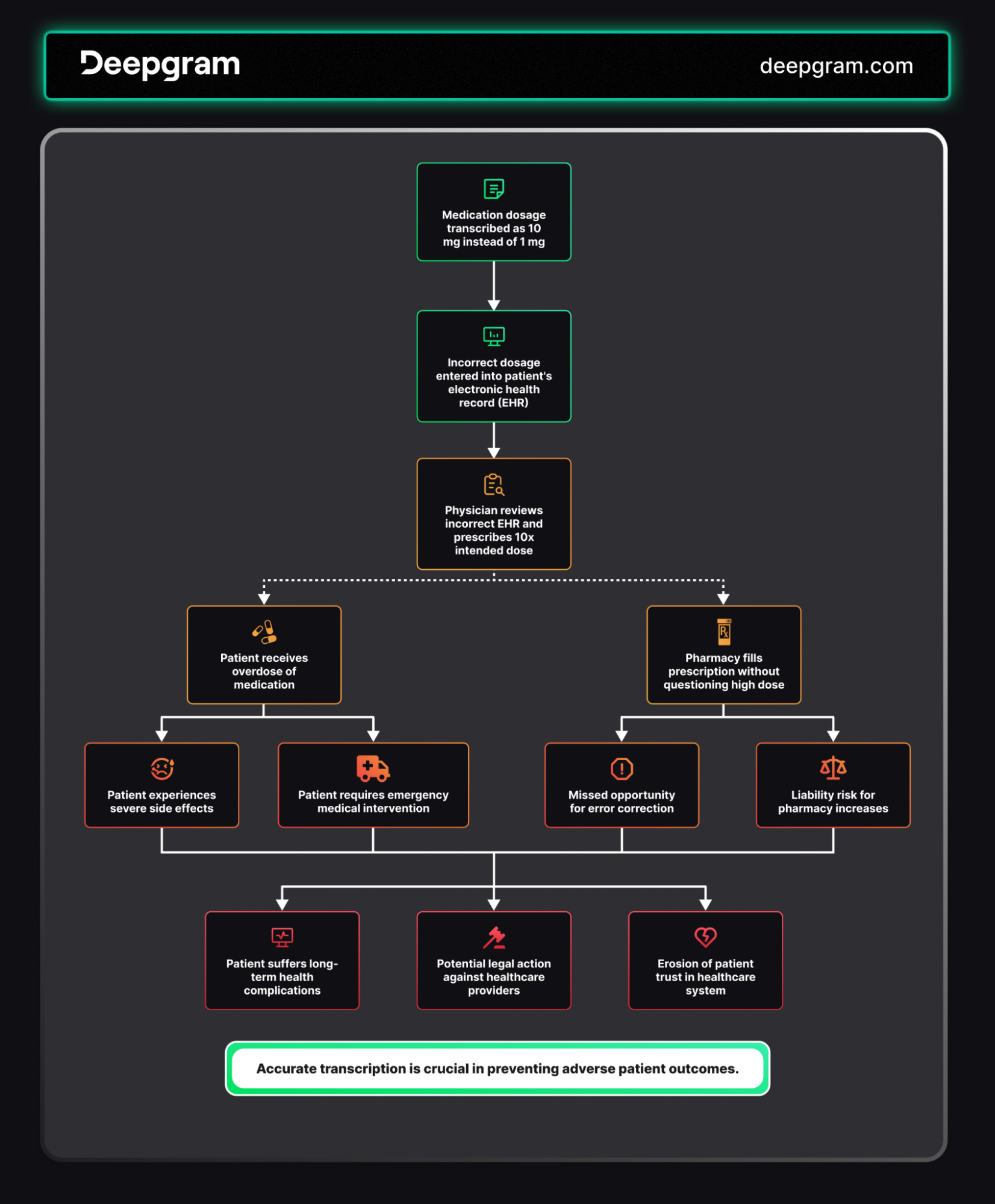
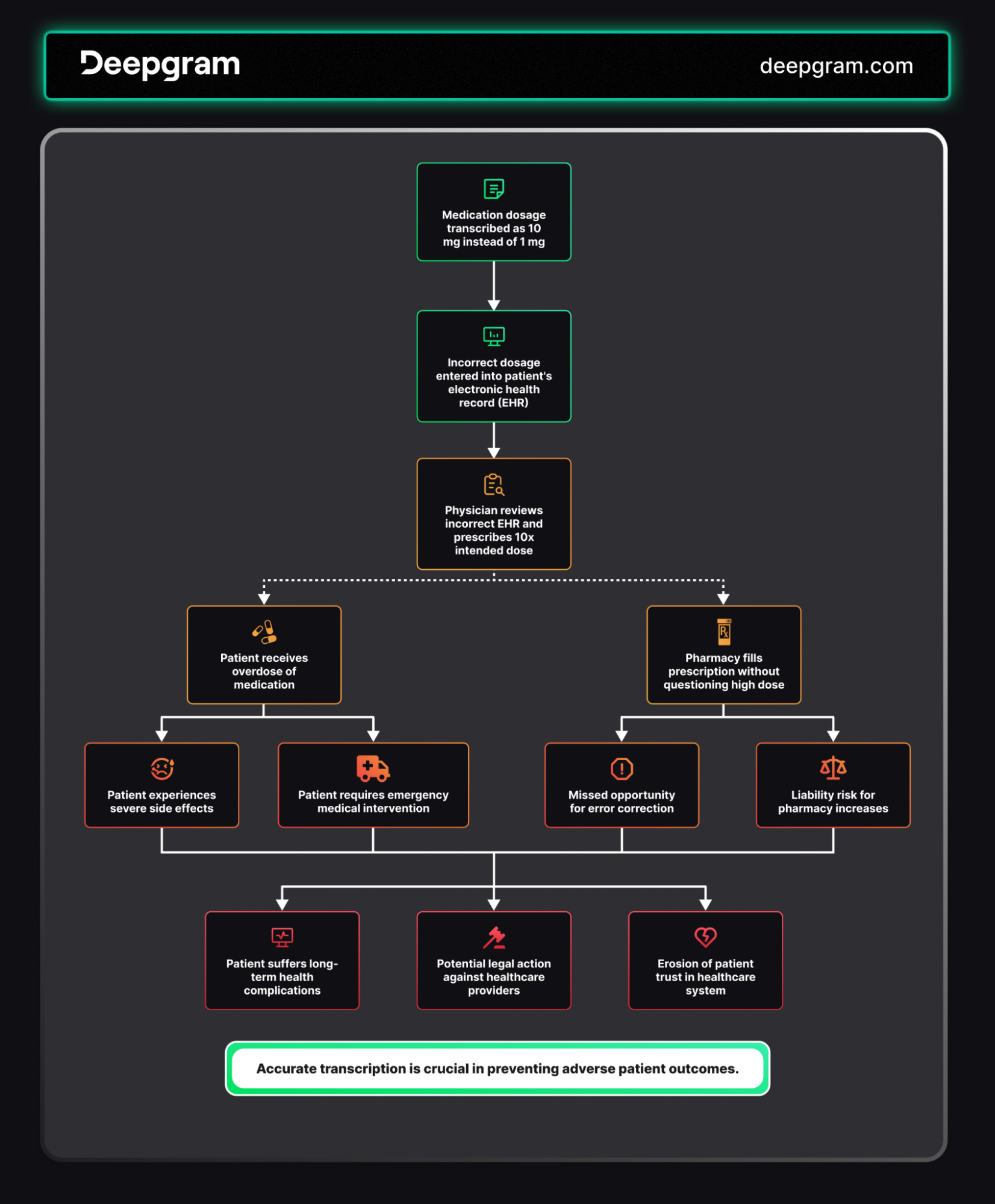
Legal and Compliance Issues
Inaccurate medical transcription can expose healthcare providers and institutions to significant legal and regulatory risks, including:
Malpractice Lawsuits: Errors in medical records that lead to patient harm can be grounds for malpractice suits. Accurate transcription is crucial for defending against such claims or, conversely, for patients seeking to prove negligence.
HIPAA Violations: The Health Insurance Portability and Accountability Act (HIPAA) requires protecting patient health information. This means that healthcare providers must enforce strict safeguards to protect transcription solutions from adversarial attacks that could lead to the leakage of patient information. A breach could violate HIPAA, leading to hefty fines and damaging the healthcare provider's reputation.
Insurance Claim Delays: Inaccuracies in transcribed medical records can lead to insurance claim delays or denials, causing financial stress for patients and administrative burdens for healthcare providers.
Regulatory Non-Compliance: Regulatory bodies, such as the Joint Commission (JCAHO) and Centers for Medicare & Medicaid Services (CMS), require accurate medical documentation. Failure to maintain precise records due to transcription errors can result in penalties or loss of accreditation.
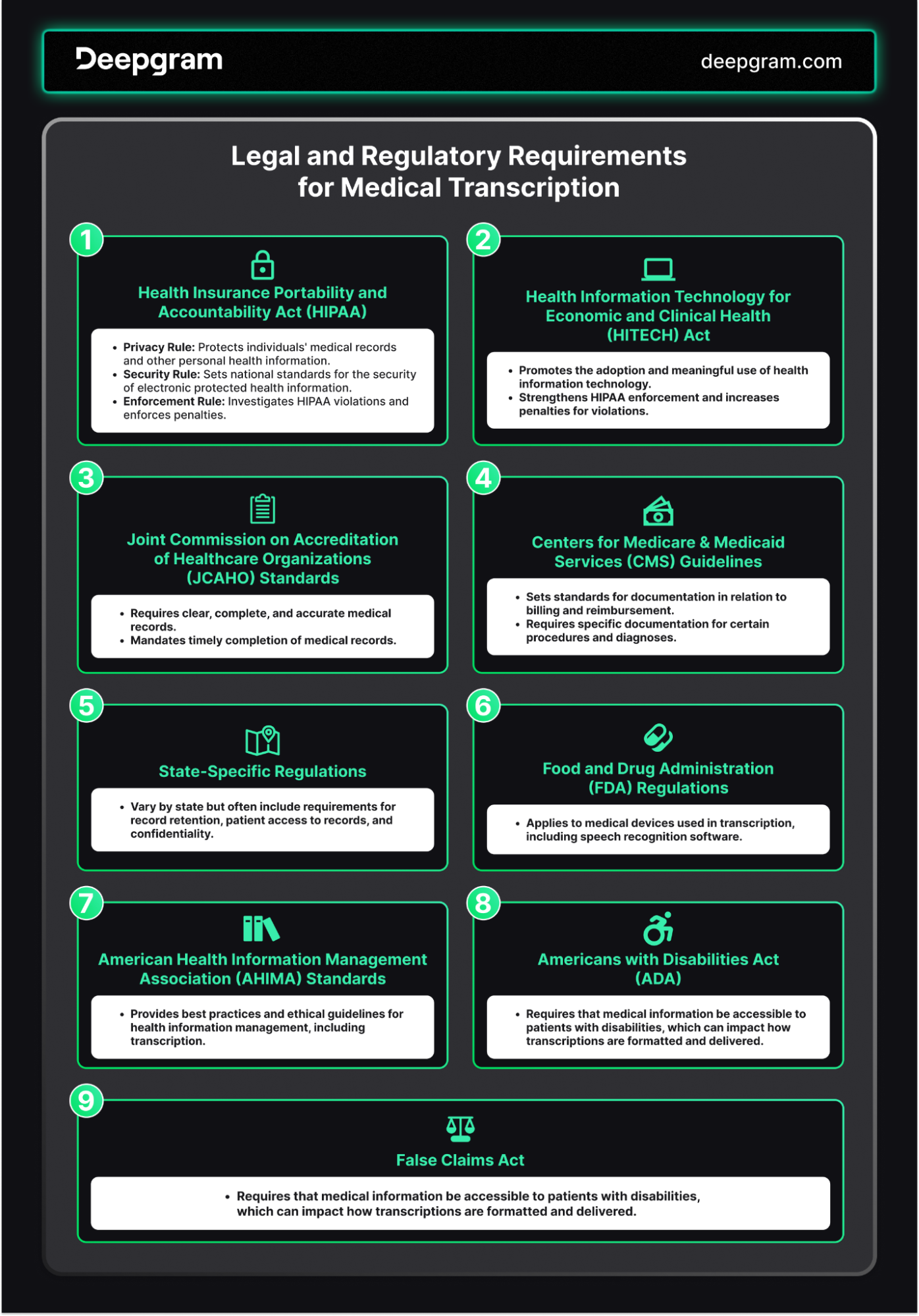
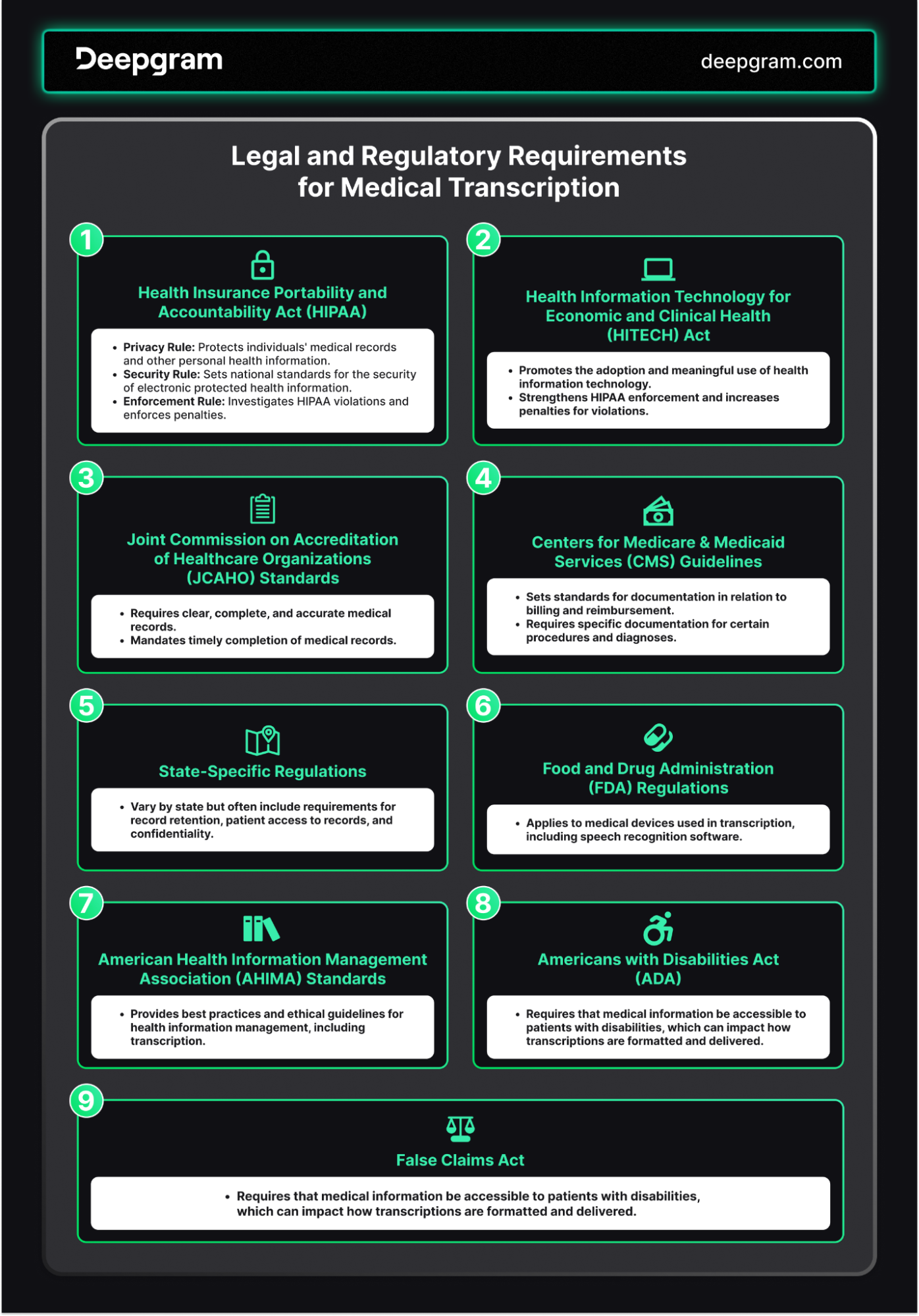
Financial Implications
The financial impact of inaccurate medical transcription can be substantial for healthcare institutions:
Costs of Correction: Time and resources spent identifying and correcting transcription errors represent a significant operational cost.
Billing Errors: Inaccurate transcription can lead to incorrect billing codes, which can result in claim denials, delayed payments, or even accusations of fraud.
Increased Labor Costs: The need for manual review and correction of transcripts increases labor costs and reduces overall efficiency.
Technology Investment: Recurring transcription errors may require investment in more advanced (often more expensive) transcription solutions.
Legal Defense Costs: Legal defense costs can be substantial in cases where transcription errors lead to legal action.
Reputation Damage: Frequent errors can damage an institution's reputation, potentially leading to the loss of patient referrals, which translates to lost revenue.
Using Word Error Rate (WER) to Measure the Performance of Medical Transcriptions
The challenges of inaccurate medical transcription highlight the need for highly accurate speech-to-text solutions in healthcare settings. The word error rate (WER) is a key metric used to assess transcription accuracy.
This statistic is calculated by dividing the sum of substitutions, deletions, and insertions by the total number of words in the reference transcript. A lower WER indicates better transcription accuracy.
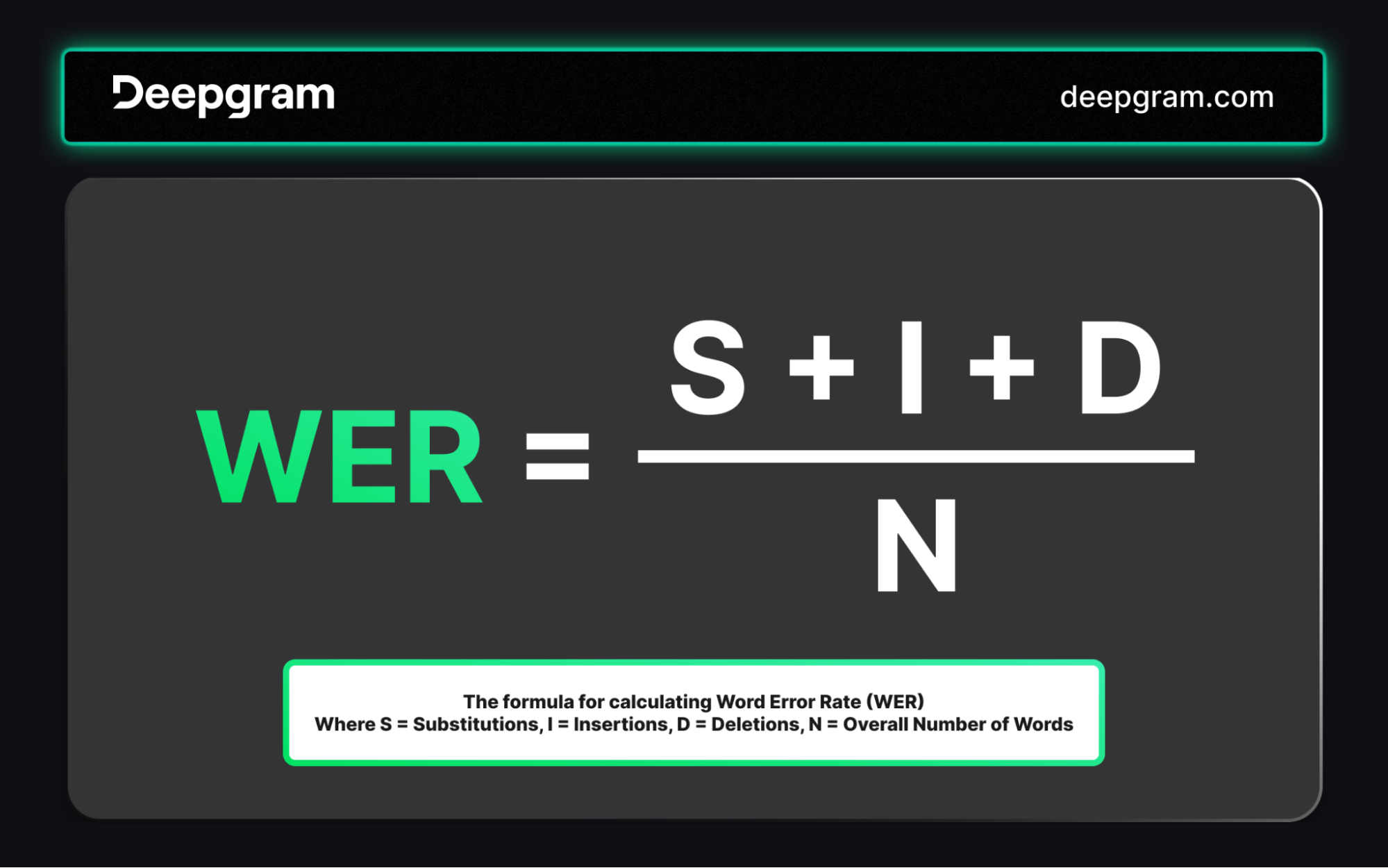
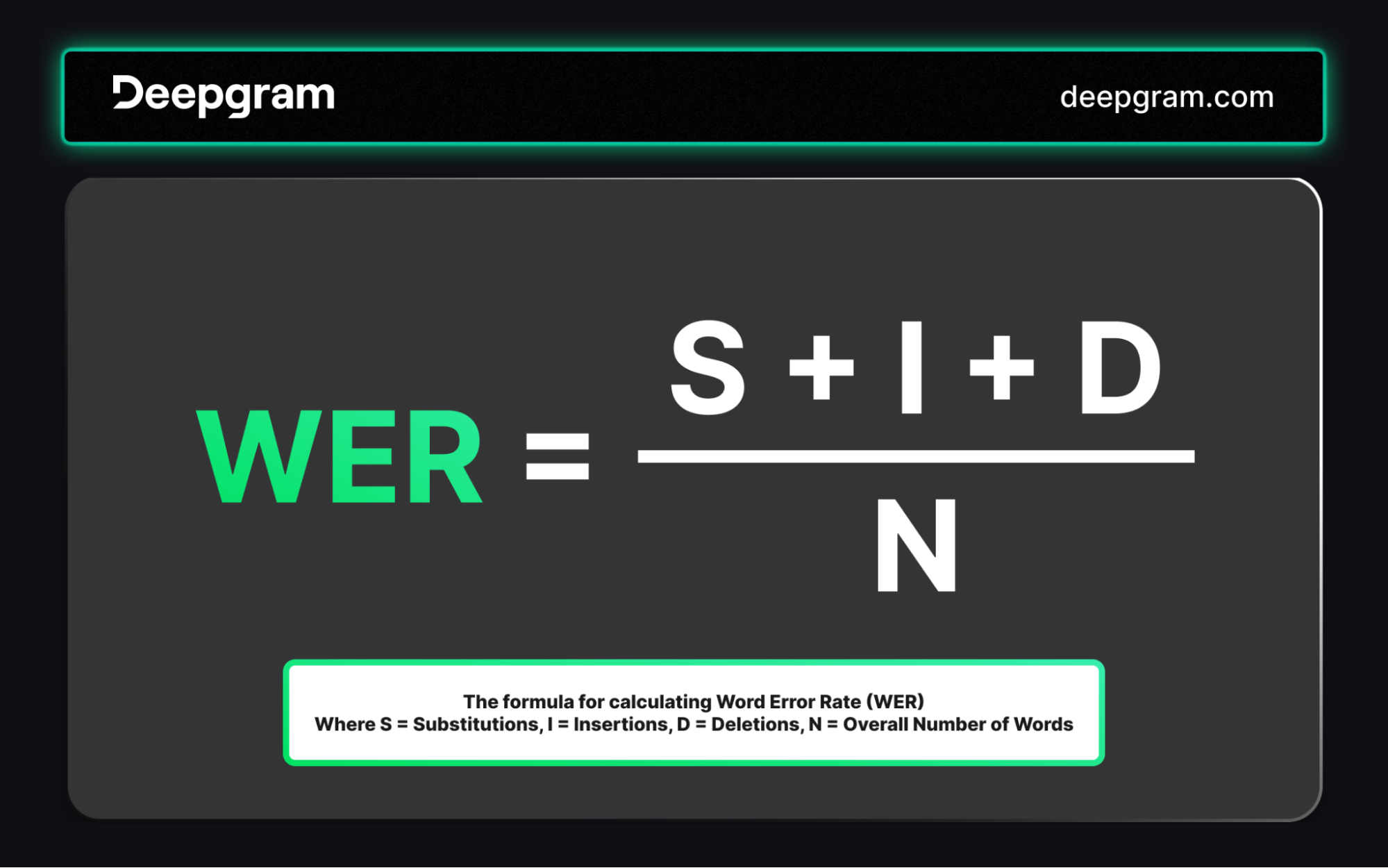
When it comes to medical transcription, Deepgram's Nova-2 model consistently gets the lowest WER of its competitors. Being so accurate means that medical transcriptions will be done correctly every time. However, to properly judge its strengths, we must see how it does in other important healthcare-specific areas.
WER provides accurate transcription but does not fully represent medical language and conversation. The transcription systems must accurately recognize the technical terminology, acronyms, and pharmaceutical names in healthcare. A medical transcription system must also support the speech patterns and accents of several medical fields and specialists.
The following section will detail how Deepgram's transcription model, Nova-2 Medical Model, tailored for medical settings, handles these medical transcription challenges. We will also look at how well it works in real-world healthcare, handles domain-specific languages, and is flexible across different medical specialties.
Deepgram’s Nova 2 Medical Model is Improving Medical Transcription
Fine-tuned for Medical Language
At Deepgram, we have specifically partnered with practitioners, healthcare institutions, and experts to train our Nova 2 Medical Model on large medical datasets so it can:
Recognize Terminologies: The model can accurately identify and transcribe specialized medical terms, even when rarely used in general conversation.
Handle Acronyms: It can recognize and correctly interpret various medical acronyms and abbreviations.
Be Accurate on Latin Terminologies: We trained the model to recognize and accurately transcribe Latin medical terms.
Importantly, the Nova 2 Medical Model complies with medical documentation standards and HIPAA regulations, ensuring that transcriptions meet legal and ethical requirements.
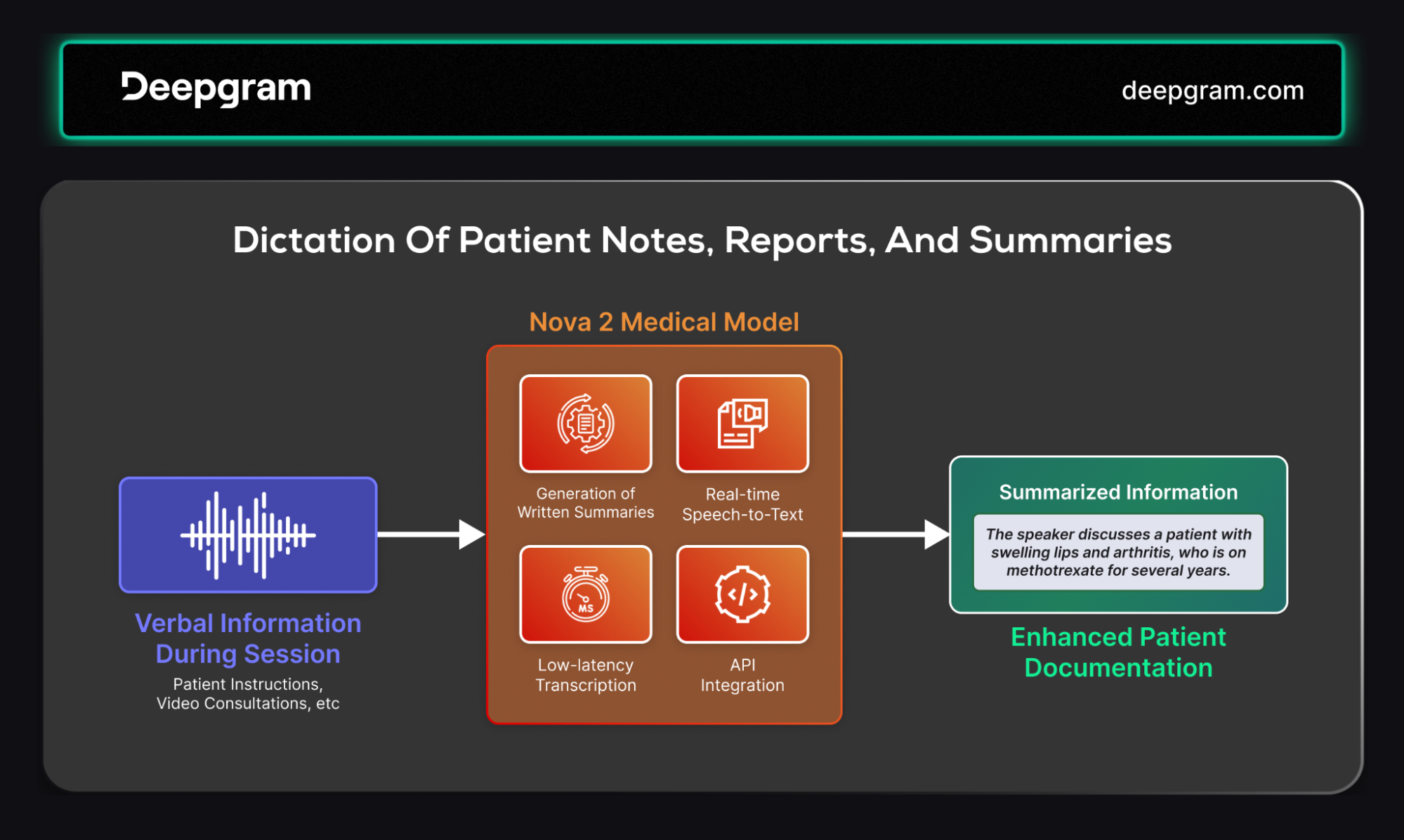
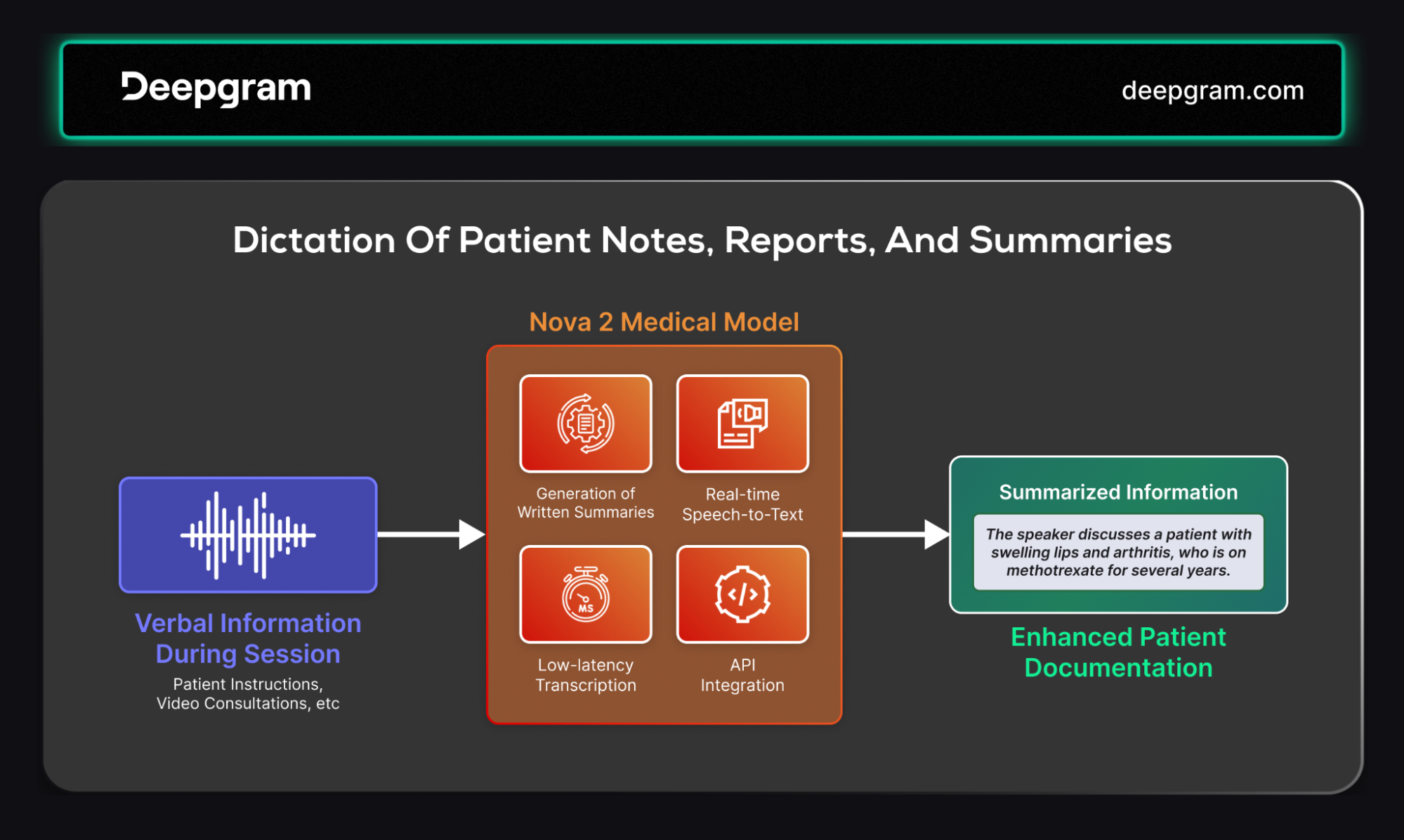
Improved Accuracy and WER
When it comes to medical situations, the Nova 2 Medical Model is more accurate than general ASR systems. Here are some statistics:
16% Relative Improvement in Word Recall Rates (WRR): The model performs better at correctly identifying and transcribing medical terms.
11% Relative Improvement in Word Error Rate (WER): This translates to fewer errors overall, resulting in more reliable medical documentation for pre-recorded transcription.
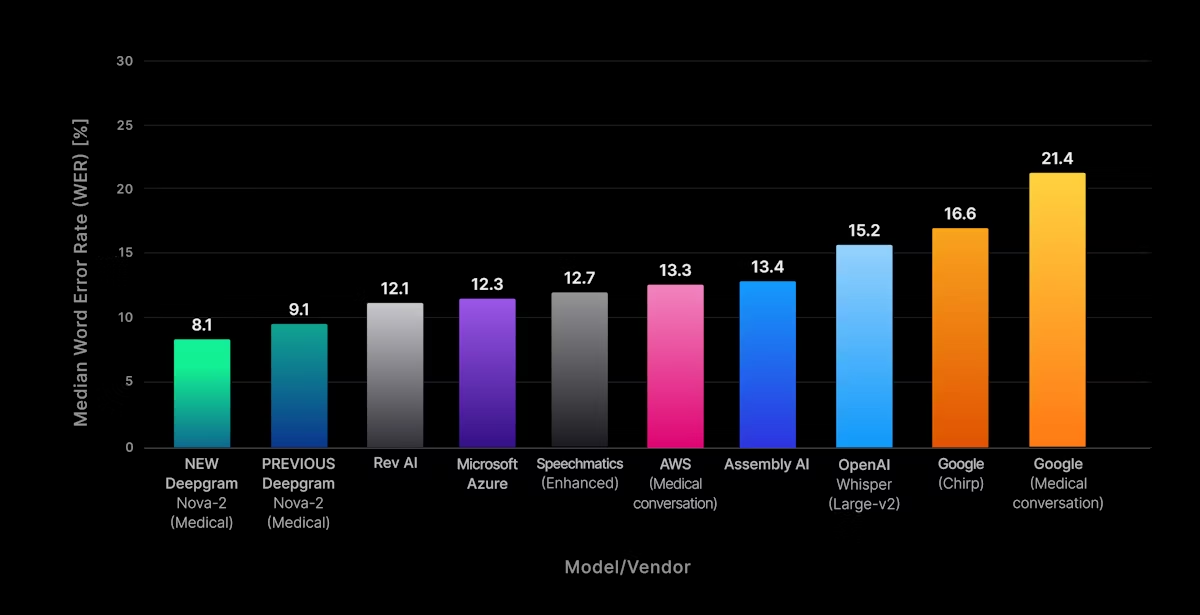
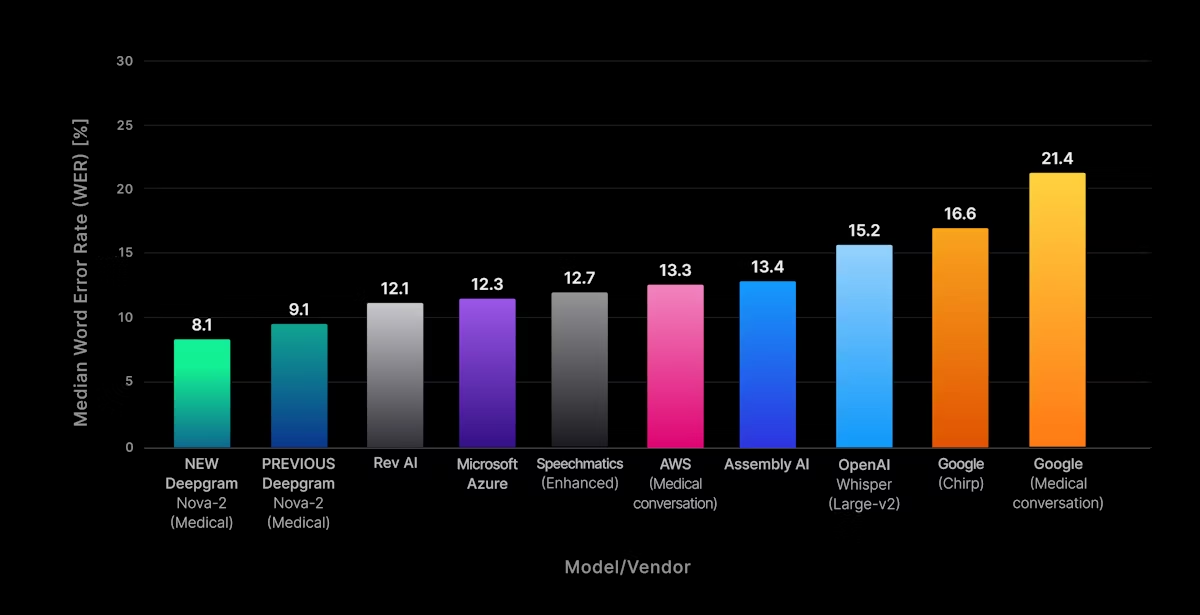
Contextual Understanding
One of the key strengths of the Nova 2 Medical Model is its ability to use context from surrounding speech, clinical setting, and speaker roles for improved accuracy:
Homophone Differentiation: The model can distinguish between similar-sounding medical terms (homophone) based on the surrounding context. For example, it can correctly differentiate between "ilium" (part of the pelvis) and "ileum" (part of the small intestine) based on the context of the medical discussion.
Domain-Specific Interpretation: The model adapts its understanding based on the discussed medical specialty, which improves accuracy in specialized fields.
Model Customization and Adaptability
You can customize the Nova 2 Medical Model to fit different needs:
Specialty Customization: For instance, fine-tuning the model for particular medical specialties to improve its precision for specific vocabularies.
Continuous Learning: You can update the model with new medical terms and usage patterns to adapt to evolving medical vocabulary.
Real-time Transcription and Integration
The Nova 2 Medical Model generates transcriptions in real-time to enable immediate documentation during patient consultations or procedures. Transcription speeds are 5 to 40 times faster than competitors, significantly reducing turnaround times.
Cost Efficiency
Even though it has advanced features, the Nova 2 Medical Model is cost-efficient compared to other models in the following ways:
Reduced Manual Review: Higher accuracy means less time spent on manual corrections, reducing labor costs.
Faster Documentation: Quicker transcription speeds can lead to more efficient healthcare delivery and potentially increased patient throughput.
Scalability: The model can handle large volumes of transcription tasks without a proportional cost increase.
You can also integrate it with Electronic Health Record (EHR) systems, streamlining the documentation process. For instance, Deepgram's Nova 2 Medical Model powers TORTUS and Phonely AI, which integrate with existing EHR systems to keep accurate records of conversations with patients and streamline the documentation process.
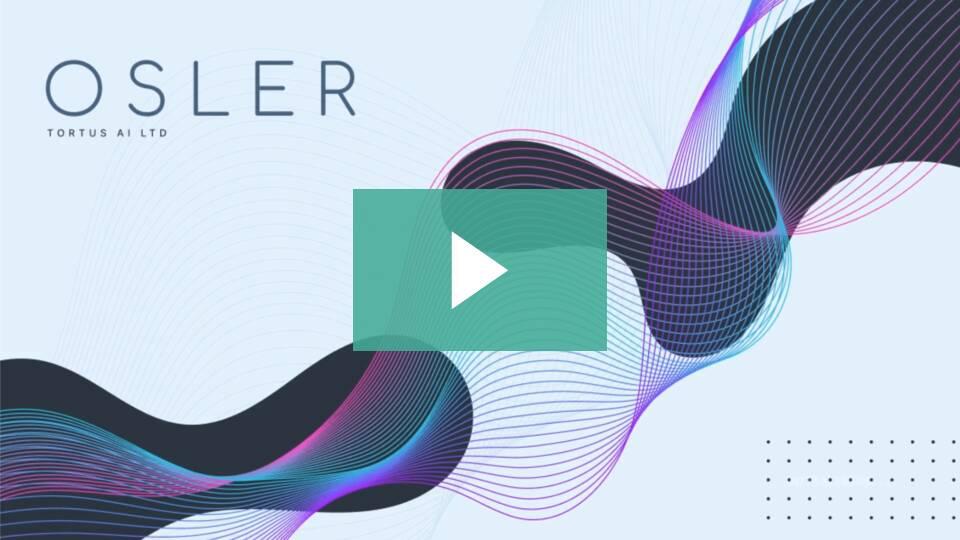
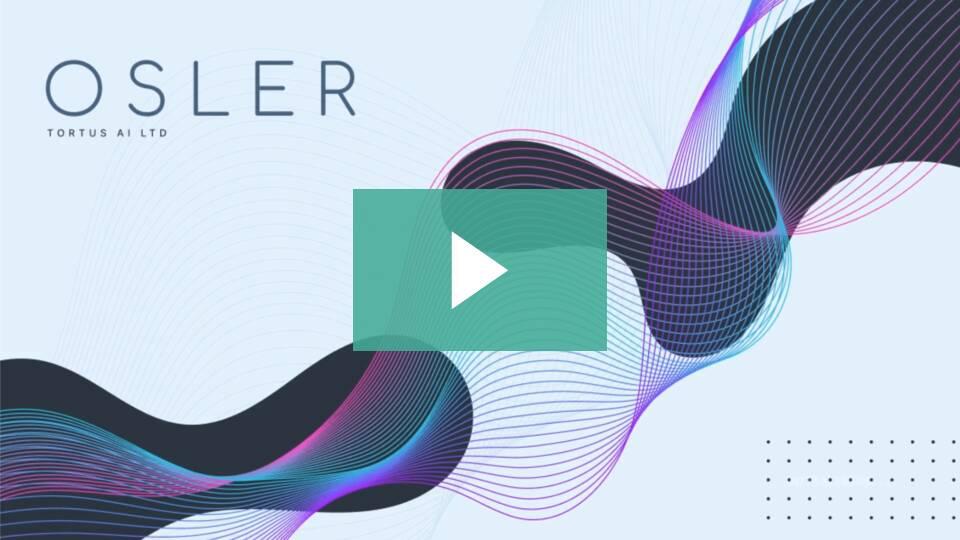
Considering the special difficulties of medical transcription, Deepgram's Nova 2 Medical Model is raising the bar for precision, speed, and dependability in medical writing. This model does more than just improve transcription; it also contributes to improving patient care, lowering risks, and making healthcare operations run more smoothly for practitioners.
Conclusion
Medical transcription is generally difficult because of specialized terms, accents, and background noise. Medical terms are hard for traditional speech recognition systems to understand, which leads to more transcription mistakes. If this is not done right, managed, and kept up to date according to federal and state laws and rules, it could make people break the law and lose their trust.
Deepgram's Nova 2 medical model solves these problems by better understanding and transcribing complex medical terms. Its real-time transcription features allow for real-time documentation in healthcare settings that move quickly.
It follows medical documentation rules and standards and can be fine-tuned to fit different medical specialties with custom vocabulary options. Deepgram's medical transcription APIs can help with accuracy, efficiency, and risk reduction. This can make the work of medical professionals easier and give them more time to focus on more important parts of patient care.
You can try it out on your own audio samples by visiting Deepgram's API Playground.
[VISUAL ASSET PLACEHOLDER: Call-to-action buttons for "Try the API Playground" and "Sign Up for Deepgram"]
Frequently Asked Questions
How accurate are current AI medical transcription models compared to human transcriptionists?
Models like Deepgram's Nova 2 Medical Model have shown significant improvements in accuracy. For example, it demonstrates a 16% relative improvement in Word Recall Rates (WRR) and an 11% relative improvement in Word Error Rate (WER) compared to general Automatic Speech Recognition (ASR) systems. While these models are becoming increasingly accurate, they are typically used with human review to ensure the highest accuracy in critical medical documentation.
What are the potential risks and consequences of inaccurate medical transcription?
Inaccurate medical transcription can lead to several serious consequences. It can pose patient safety risks through misdiagnosis or delayed care due to transcription errors. There's also an increased risk of legal and compliance issues, including potential malpractice lawsuits and HIPAA violations.
What are the main challenges in creating AI models that understand complex medical terminology?
The main challenges in creating AI models for medical terminology include dealing with specialized vocabulary that combines English, Latin, and Greek terms often underrepresented in general speech recognition datasets. Additionally, these models must navigate medical professionals' frequent use of abbreviations and acronyms, which can have multiple meanings depending on the context. They must also account for the varied accents and speaking styles of healthcare providers and patients from diverse backgrounds.
Unlock language AI at scale with an API call.
Get conversational intelligence with transcription and understanding on the world's best speech AI platform.