Top AI Models for Medical and Biotech Applications
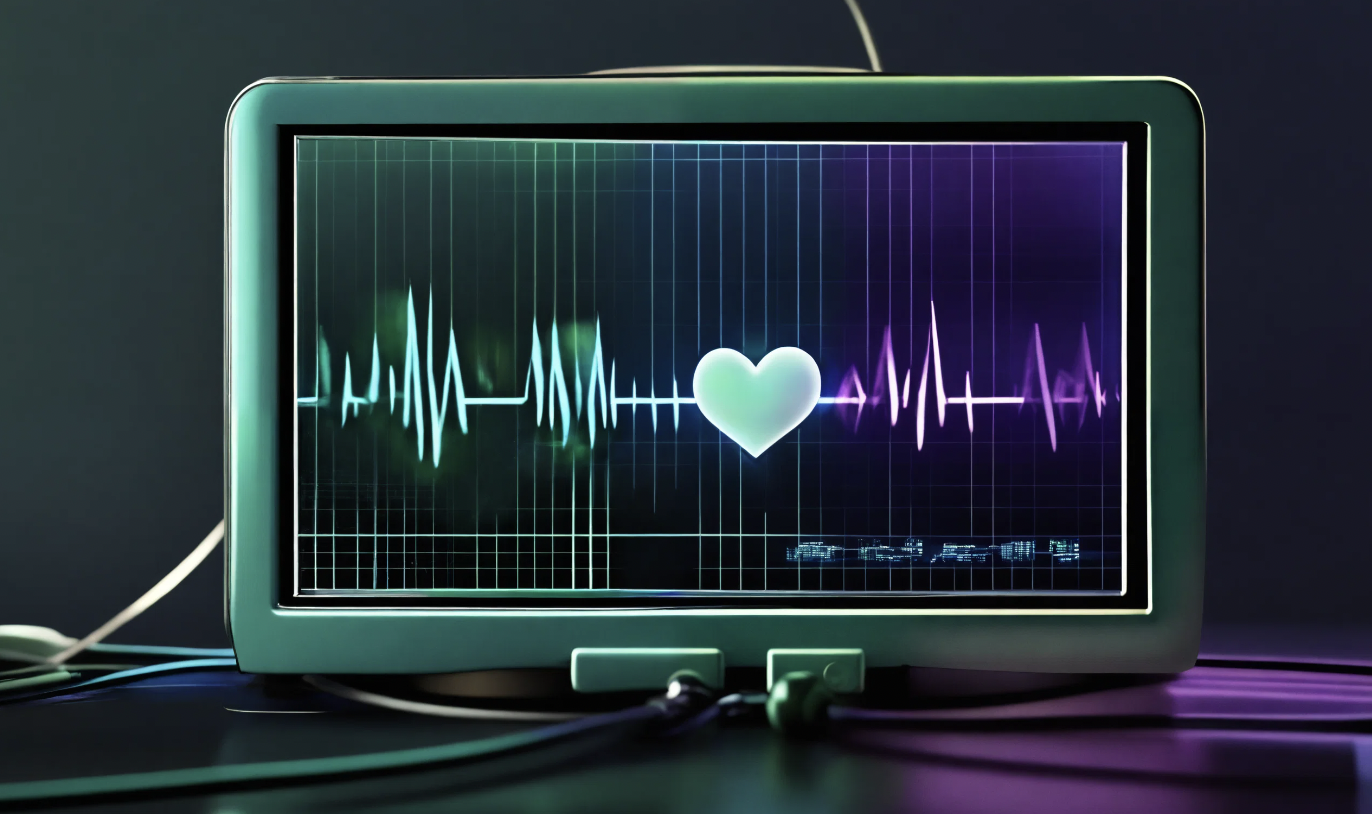
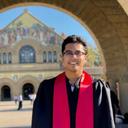
1. BioNeMo
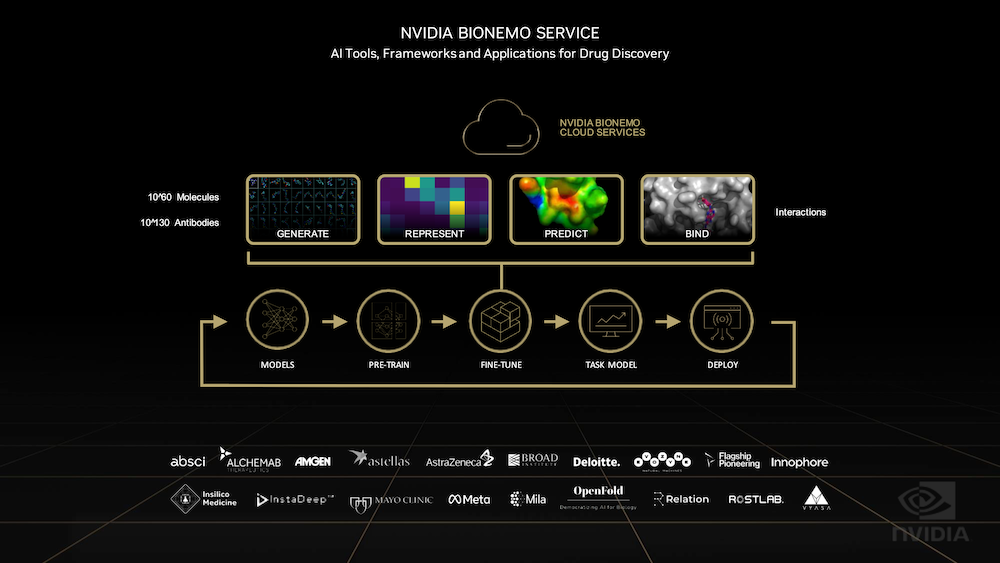
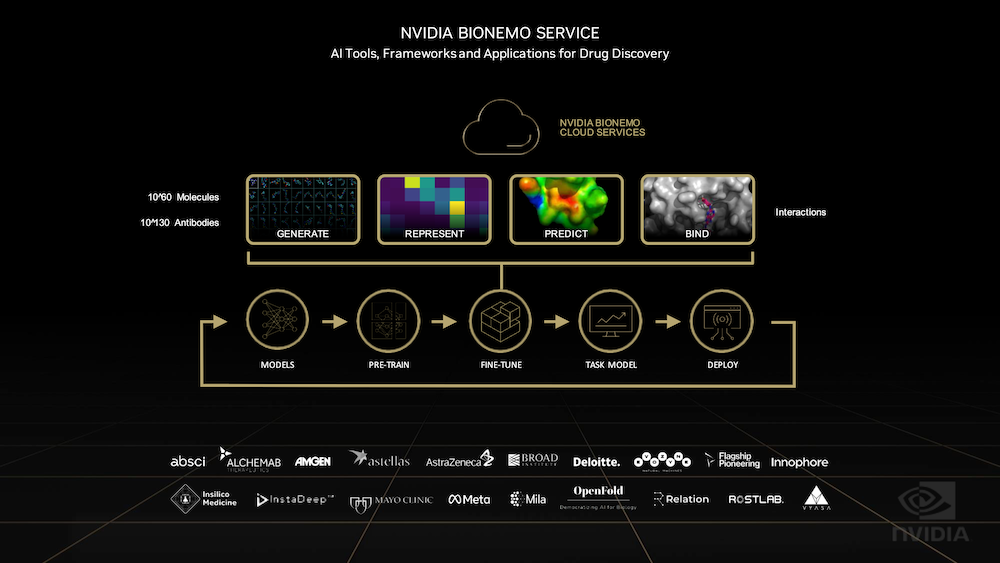
BioNeMo: NVIDIA's BioNeMo Cloud service offers pre-trained AI models that can be fine-tuned for drug discovery and development. Amgen has used BioNeMo to pre-train and fine-tune large language models on their proprietary antibody data, significantly accelerating their biologics discovery process.
Expanding on BioNeMo, it's worth noting how the synergy between high-performance computing and AI is transforming the landscape of drug discovery. Traditionally, identifying viable drug candidates was a labor-intensive process, often taking years of meticulous research and clinical trials. With BioNeMo, researchers can leverage vast datasets, integrating complex biological data with computational models, to predict and simulate how new compounds will interact with biological targets. This predictive capability not only shortens the timeline from discovery to development but also enhances the precision of the outcomes, potentially leading to more effective and safer biologics.
2. Protein Transformer Variational AutoEncoder
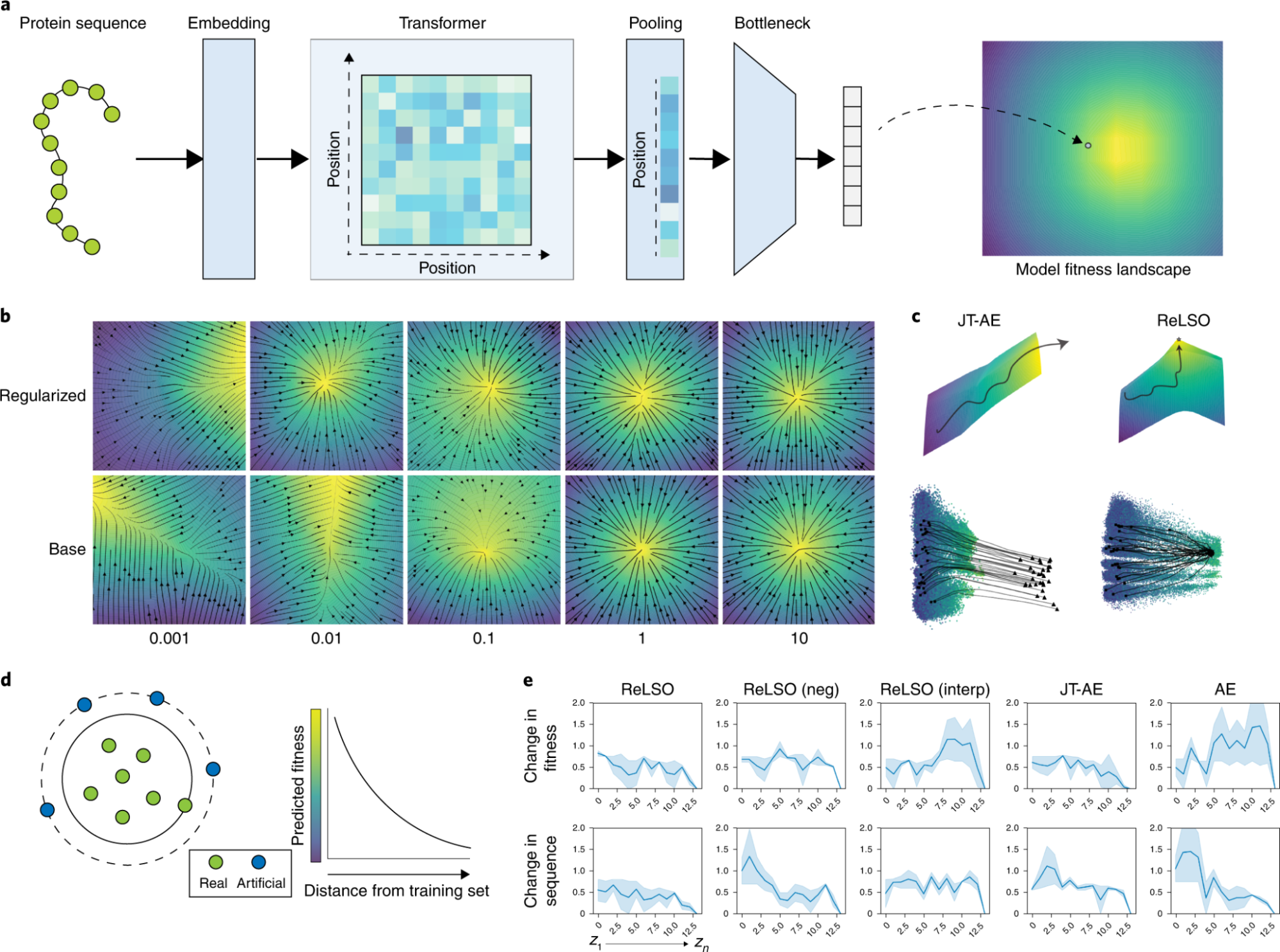
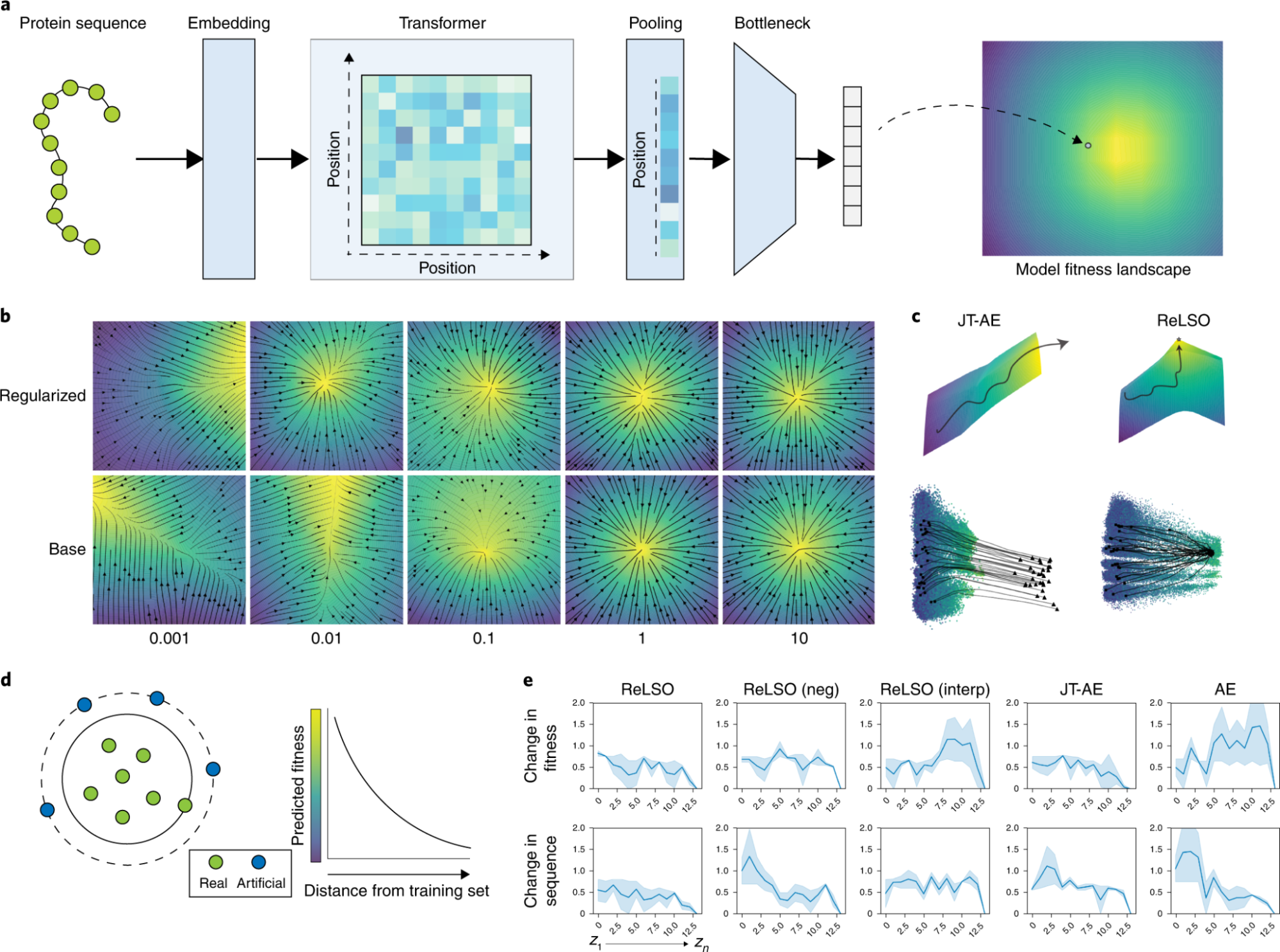
Protein Transformer Variational AutoEncoder: Developed by Evozyne in collaboration with NVIDIA, this BioNeMo-based deep learning model is fine-tuned on proprietary protein data to design synthetic enzyme variants with improved performance compared to natural enzymes.
To delve deeper into the Protein Transformer Variational AutoEncoder, consider the implications of designing synthetic enzymes. Enzymes are biological catalysts that accelerate chemical reactions in the body, and their synthetic variants can be tailored for specific industrial or therapeutic purposes. By utilizing AI to model and predict enzyme behavior, researchers can create enzymes that are not only more efficient but also stable under various conditions. This advancement holds promise for numerous applications, including biofuel production, waste management, and the development of novel therapies for diseases where traditional enzymes fall short.
3. ESM: Evolutionary Scale Modeling
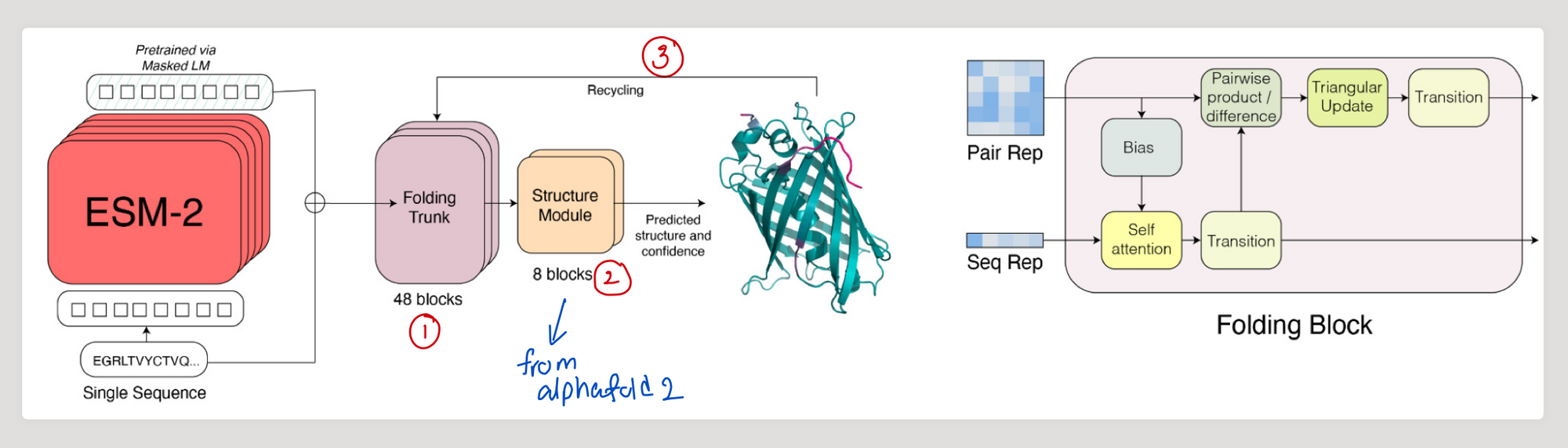
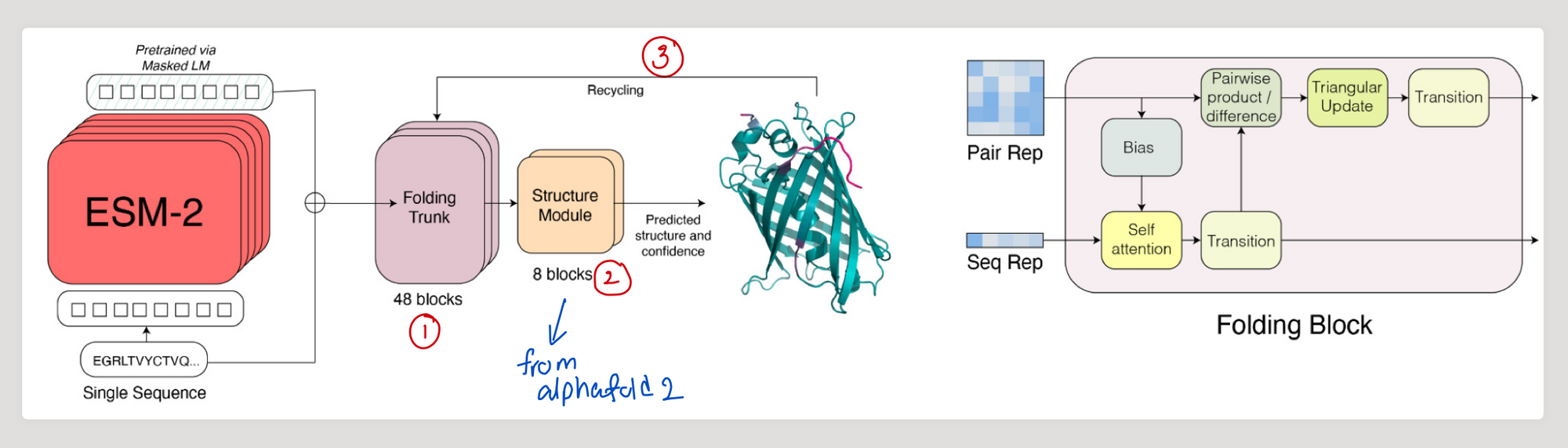
ESM (Evolutionary Scale Modeling): This model architecture, available through BioNeMo, was fine-tuned by Amgen using their proprietary data on antibodies. This customization allowed Amgen to reduce the time required to train five custom models for molecule screening and optimization from three months to a few weeks.
The ESM model underscores the importance of evolutionary principles in AI-driven drug discovery. By emulating natural selection and mutation processes, ESM can predict the evolutionary trajectory of molecules, enabling researchers to identify promising candidates more efficiently. This evolutionary approach not only speeds up the screening process but also improves the accuracy of predicting how molecules will evolve in response to various pressures, such as resistance from pathogens. The ability to rapidly iterate and refine molecular designs is a game-changer in developing next-generation therapeutics.
4. Insilico Drug Discovery Model
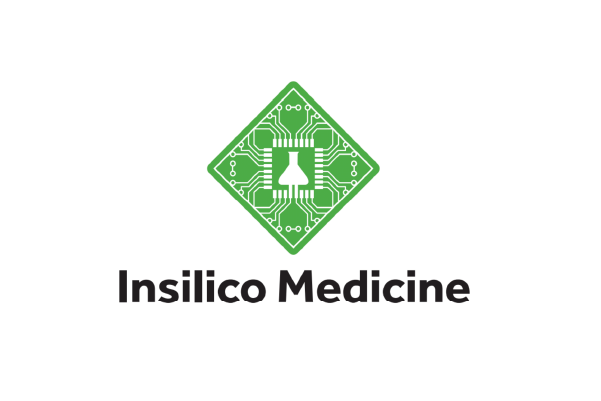
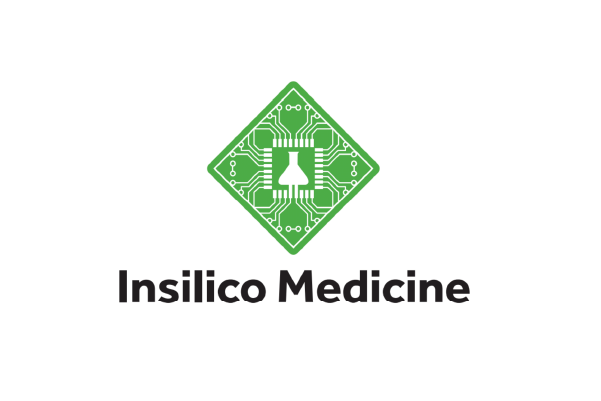
Insilico Medicine's Drug Discovery Model: While not named specifically, Insilico Medicine has used BioNeMo to fine-tune models for accelerating the early drug discovery process. Their approach uses generative AI from end to end, significantly reducing time and costs in identifying preclinical candidate drugs.
Insilico Medicine's approach highlights the transformative potential of generative AI in drug discovery. By generating novel molecular structures and predicting their pharmacological properties, these models can explore vast chemical spaces that would be impractical through traditional methods. This end-to-end integration of AI not only speeds up the identification of viable drug candidates but also enhances the diversity of potential therapeutics. Moreover, by reducing the reliance on costly and time-consuming laboratory experiments, generative AI democratizes drug discovery, making it accessible to smaller research institutions and startups.
5. MONAI (Medical Open Network for AI)
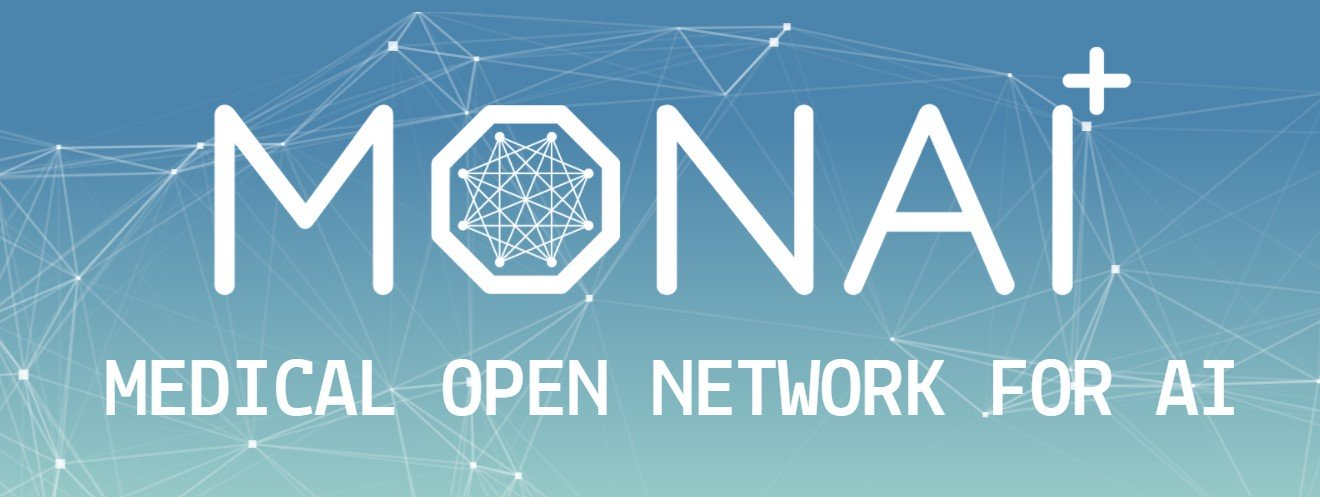
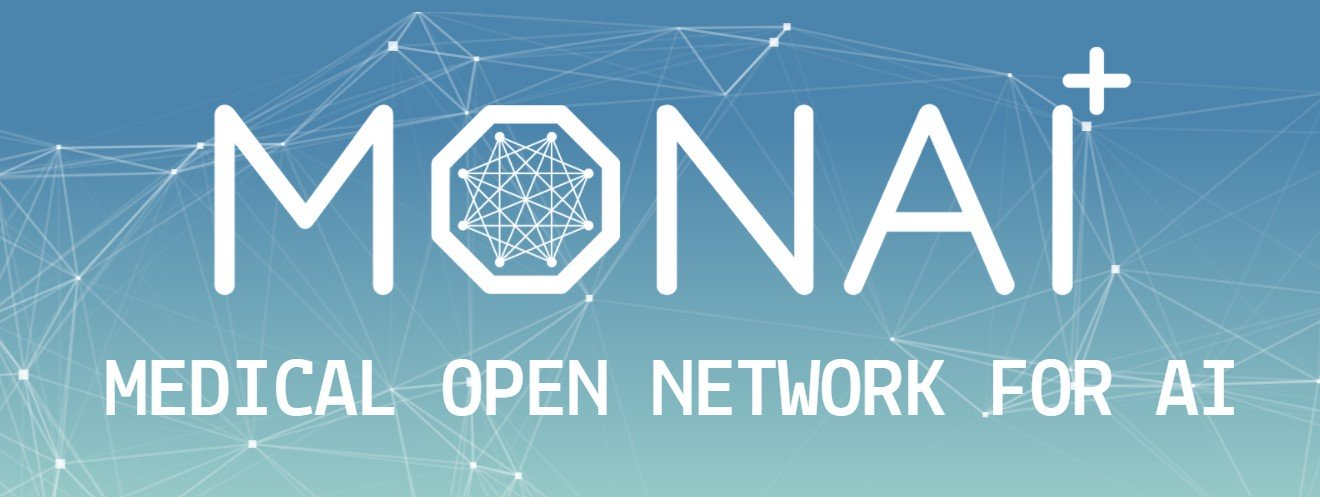
MONAI (Medical Open Network for AI): This framework, discussed in the paper "Generative AI for medical imaging: extending the MONAI framework" by Pinaya et al., allows for the development and fine-tuning of AI models specifically for medical imaging applications.
MONAI represents a significant advancement in the intersection of AI and medical imaging. Medical imaging is crucial for diagnosing and monitoring various health conditions, from cancer to neurological disorders. However, interpreting these images requires a high level of expertise and can be prone to human error. By fine-tuning AI models within the MONAI framework, researchers can develop tools that assist radiologists in identifying anomalies with greater accuracy and speed. These AI-enhanced imaging techniques can lead to earlier detection of diseases, more personalized treatment plans, and ultimately better patient outcomes.
6. Deepgram Nova-2 Medical Transcription
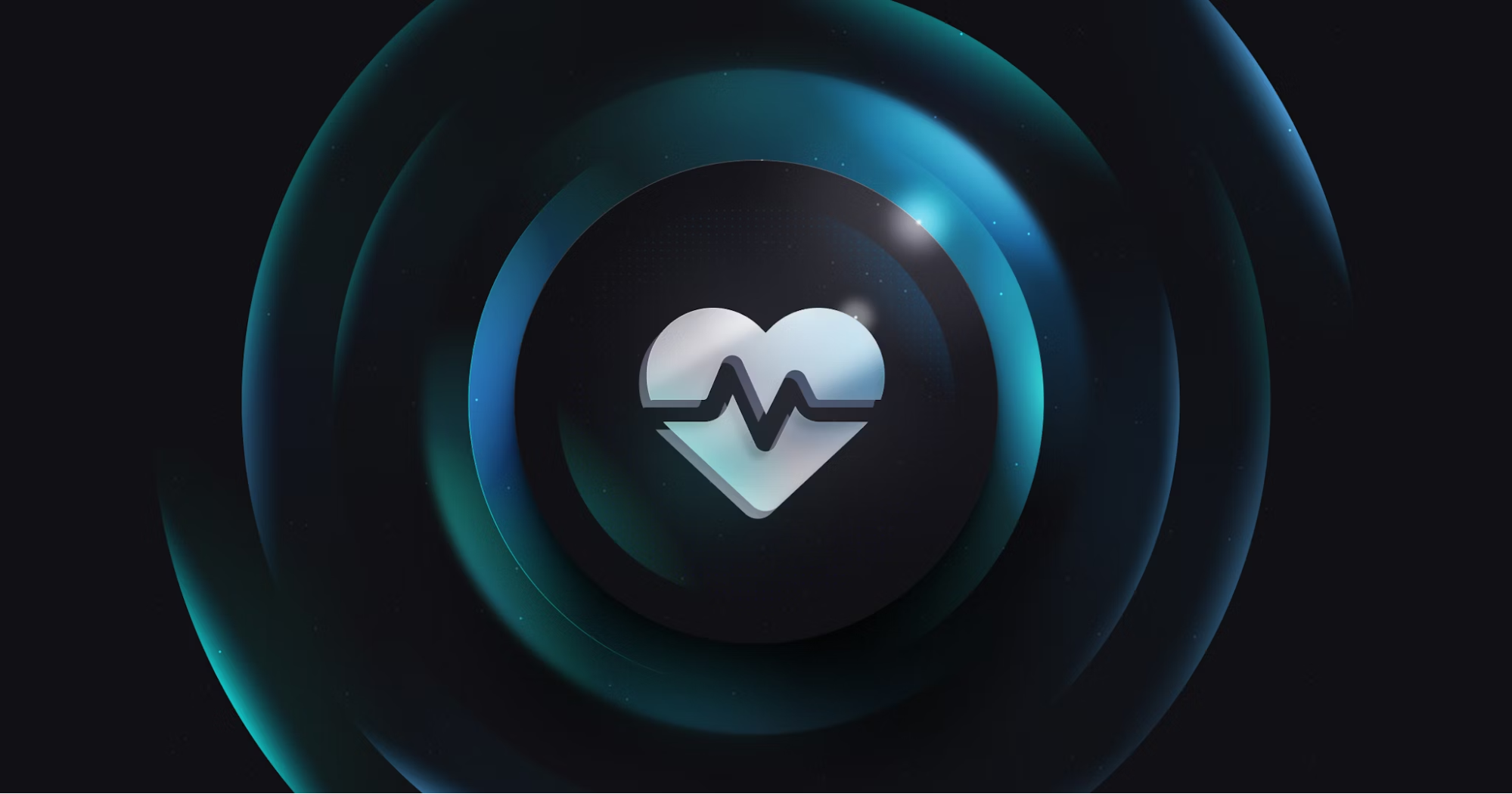
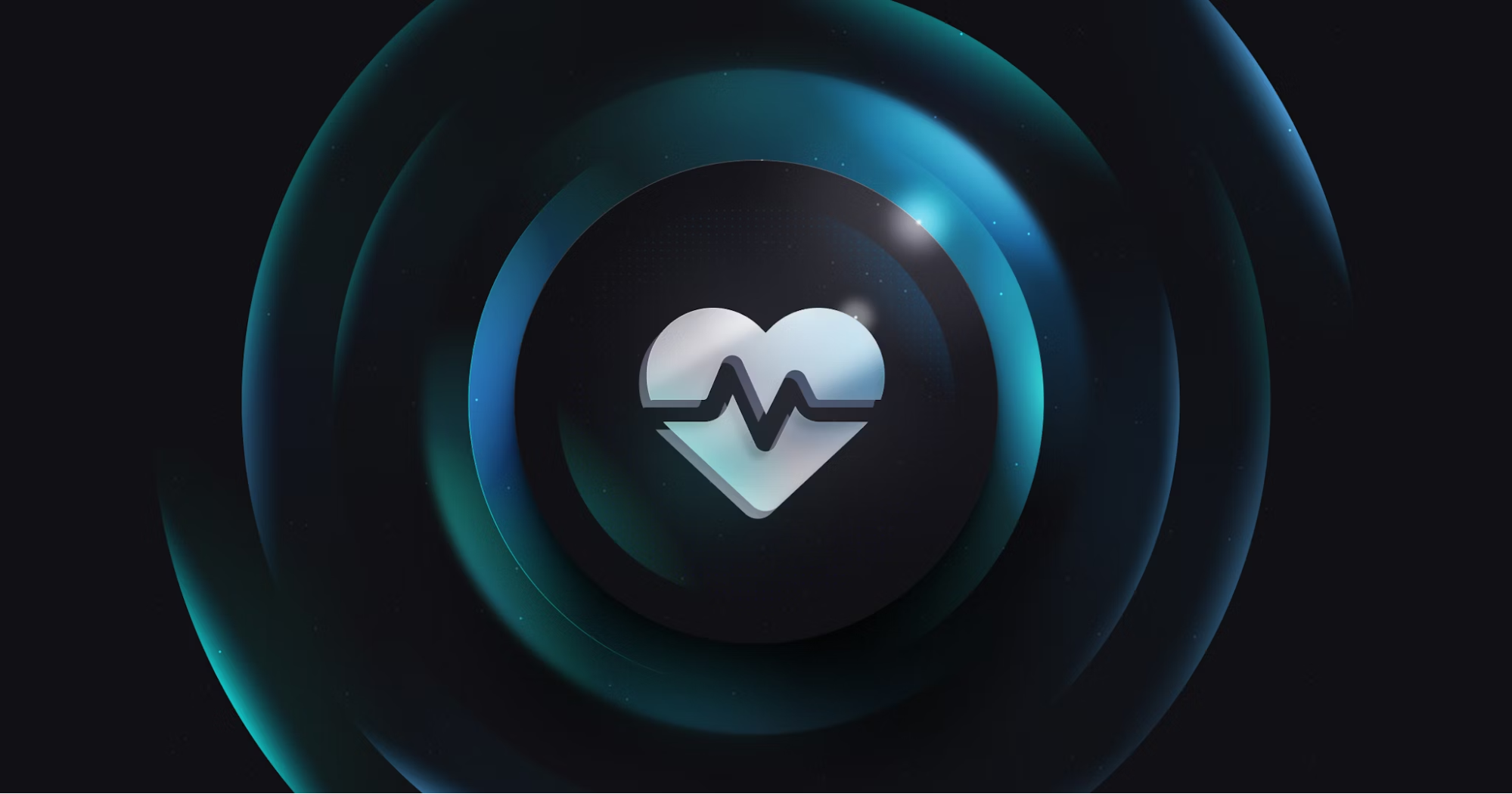
Nova-2 Medical Model - Deepgram released a Speech-to-Text (STT) model specialized for medical use cases. That is, words like drug names and disease names that regular STT models struggle with are no problem for Nova-2.
You can automate the conversion of clinical notes and doctor-patient dialogues into Electronic Health Records affordably and efficiently with this model—the industry’s best speech-to-text and language understanding API.
To learn more, click here!
Conclusion
These examples demonstrate how pre-trained AI models are being customized and fine-tuned for specific biotech and medical applications, ranging from drug discovery and protein engineering to medical imaging. The fine-tuning process allows these models to leverage domain-specific data, improving their performance in specialized tasks within the life sciences and healthcare sectors.
The fine-tuning of pre-trained AI models for biotech and medical applications is a testament to the adaptability and robustness of these technologies. By integrating domain-specific data, AI models can cater to the unique challenges and nuances of different fields. In the context of life sciences and healthcare, this means more precise and personalized solutions, whether it's in the development of new drugs, the engineering of proteins, or the interpretation of medical images. As AI continues to evolve, its integration into these fields will likely lead to more groundbreaking discoveries and innovations, ultimately enhancing human health and wellbeing.
Unlock language AI at scale with an API call.
Get conversational intelligence with transcription and understanding on the world's best speech AI platform.