AI and Medicine
he impact of AI on medicine extends far beyond the realms of efficiency. With staggering advancements in AI, the healthcare industry is on the cusp of a paradigm shift, moving from a reactive to a proactive approach in patient treatment and health maintenance.
In the rapidly evolving landscape of healthcare, the fusion of technology and medicine heralds a new era of innovation. Have you considered how artificial intelligence (AI) is not just reshaping, but fundamentally revolutionizing healthcare management and communication? The impact of AI on medicine extends far beyond the realms of efficiency; it's about augmenting human capabilities and transforming patient care. With staggering advancements in AI, the healthcare industry is on the cusp of a paradigm shift, moving from a reactive to a proactive approach in patient treatment and health maintenance.
But what exactly does this mean for medical professionals, patients, and the broader healthcare ecosystem? This article delves into the intricate relationship between AI and medicine, exploring how AI propels healthcare into the future, while also addressing the critical ethical considerations that accompany this technological leap.
Section 1: How AI and Medicine relate
The relationship between AI and medicine is intricate and multifaceted, with AI acting as a catalyst for enhanced healthcare delivery, patient outcomes, and medical research. To understand AI in the context of healthcare, it's important to recognize its role as an enabler of more informed decision-making and precision in treatment. AI's capabilities, ranging from data analysis to machine learning, equip healthcare professionals with tools to make faster, more accurate diagnoses and tailor treatments to individual patient needs.
One of the most significant impacts of AI on healthcare management lies in its ability to streamline operations and patient care. By leveraging solutions (detailed here, for example), AI in Healthcare Management, medical institutions can optimize their workflows, reduce administrative burdens, and allocate resources more effectively, ultimately leading to improved patient experiences.
Moreover, AI's predictive abilities in preventive care and chronic disease management are groundbreaking. Concepts from pll.harvard.edu expound on how AI can analyze vast data sets to predict health risks and customize preventive measures for patients, thereby minimizing the prevalence and impact of chronic conditions.
The transformation of healthcare through AI is evident in the shift from traditional reactive treatment to proactive health maintenance. This shift is not just a theoretical concept; it's being realized as AI applications become more integrated into everyday healthcare practices.
A pivotal role of AI is in the management of healthcare data sets. The efficiency of care delivery and resource allocation improves dramatically when healthcare providers can quickly analyze and interpret complex data. AI's integration into electronic health records (EHRs) is a testament to this, as it facilitates personalized patient care by offering insights tailored to individual health profiles.
While the benefits of AI in medicine are profound, ethical considerations remain at the forefront. Ensuring data privacy and maintaining human oversight in decision-making processes are paramount to the responsible application of AI. It's essential to navigate these challenges carefully to maintain trust in the healthcare system and protect patient rights.
The journey of integrating AI into healthcare is one of balance—balancing technological innovation with ethical responsibility, balancing predictive analytics with personal touch, and balancing the potential of AI with the timeless values of patient care.
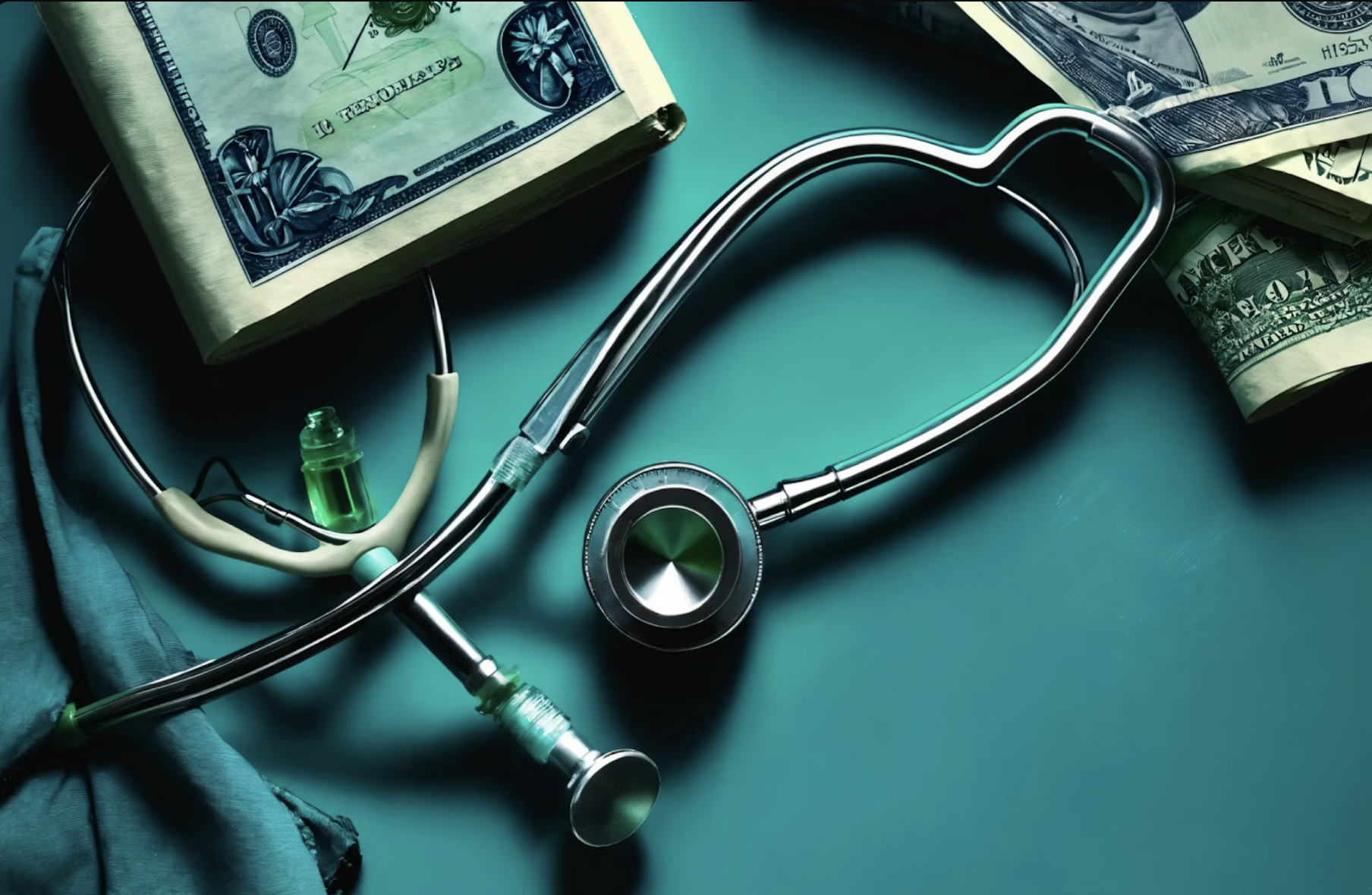
There's one AI technique that can improve healthcare and even predict the stock market. Click here to find out what it is!
Section 2: Current Medical AI models
The current landscape of AI models and algorithms in healthcare settings is nothing short of revolutionary. Each model serves as an intricate piece of a larger mosaic, where technology meets patient care, enhancing outcomes and streamlining processes. From lifestyle management to drug discovery, AI is the touchstone of modern medicine's evolution.
AI's Contribution to Lifestyle Management and Monitoring
Predictive health tracking: AI enhances the ability to monitor chronic conditions, leveraging predictive analytics to foresee and mitigate potential health events.
Behavioral data insights: By analyzing lifestyle data, AI identifies patterns and provides personalized recommendations for healthier living practices.
Remote patient monitoring: Wearables and mobile apps, powered by AI, track vital signs and activity levels, ensuring patients receive timely attention and advice.
AI Applications in Medical Imaging and Diagnostics
Enhanced radiology accuracy: AI algorithms assist radiologists in detecting subtle changes in imaging, leading to early and more accurate diagnoses.
Pathology precision: Machine learning models analyze biopsy images, identifying disease markers that may elude even the most experienced pathologists.
Speed in diagnostics: AI reduces the time taken to interpret medical images, thereby accelerating the patient care journey.
AI's Role in Mental Health
Virtual therapy assistants: AI-powered chatbots provide round-the-clock emotional support and cognitive behavioral therapy, extending the reach of mental health services.
Predictive analytics: Machine learning models identify patterns in behavior and speech that may signal the onset of mental health issues, enabling early intervention.
AI in Emergency Room and Hospital Management
Optimizing patient flow: AI systems predict peak times and patient influx, allowing hospitals to allocate resources and staff effectively.
Resource utilization: Through intelligent analysis, AI helps in the distribution and rationing of equipment and beds, leading to reduced wait times and enhanced patient care.
AI's Influence on Drug Discovery
Predicting drug efficacy: Machine learning models simulate and predict how new drugs will interact with biological systems, potentially speeding up the approval process.
Safety profiling: AI algorithms assess potential side effects, ensuring patient safety and reducing the risk of adverse reactions.
Analyzing Market Trends and Forecasting Growth
Segment growth: AI's application in healthcare is expanding, with substantial growth in segments like medical imaging and data analytics, as per market size and revenue forecasts.
Future market potential: The trajectory for AI in healthcare points to continued expansion, with an emphasis on precision medicine and patient-centric care models.
The tapestry of AI in healthcare is rich and complex, with each advancement bringing us closer to a future where medicine is more personalized, proactive, and predictive. As we stand on the brink of this new era, the potential for AI to further enhance and transform healthcare is boundless, promising a healthier tomorrow for all.
AI and Diagnosis: Pioneering Precision Medicine
The fusion of AI and medicine is reshaping the landscape of diagnosis, with AI's sophisticated algorithms enhancing the precision and efficiency of detecting diseases. This integration marks a pivotal shift from traditional diagnostic methods to a more advanced, data-driven approach. AI's prowess in diagnosis is turning a new leaf in healthcare, demonstrating its potential to augment human expertise and revolutionize patient care.
AI-Assisted Patient Data Consolidation
Process Overview: AI streamlines the consolidation of disparate patient data into a cohesive record, as demonstrated by Reveleer's approach. Their system aggregates information from numerous sources, providing clinicians with a comprehensive view of a patient's health history.
Enhanced Data Accessibility: By centralizing patient data, AI facilitates quick access to critical information, enabling healthcare providers to make informed decisions swiftly.
Error Reduction: AI minimizes the inaccuracies that often arise from manual data entry, ensuring that doctors receive reliable and up-to-date patient information.
Advancements in AI for Stroke Detection
Stroke Emergency Course Insights: The course on Artificial Intelligence in Stroke Emergency reveals how AI algorithms have advanced stroke detection, significantly impacting emergency medicine by reducing time to diagnosis and treatment.
Rapid Analysis: AI systems swiftly interpret imaging studies, such as CT scans and MRIs, identifying signs of a stroke faster than conventional methods, which is vital during the critical window for intervention.
Outcome Improvement: Prompt and precise stroke detection through AI could potentially decrease morbidity and mortality rates, illustrating the technology's life-saving capabilities.
AI in Genetic Analysis
Personalized Medicine: AI's ability to analyze genetic data paves the way for personalized treatment plans tailored to an individual's genetic makeup, heralding a new era of customized healthcare.
Predictive Power: Machine learning models predict the risk of genetic disorders, allowing for preventive measures and early treatments that are more effective and less invasive.
Disease Correlation: AI uncovers correlations between genetic markers and certain diseases, offering insights into complex conditions that may otherwise go undiagnosed.
Case Studies: AI's Diagnostic Triumphs
Early Detection: AI has successfully identified conditions such as diabetic retinopathy and various cancers earlier than traditional diagnostic tools, showcasing its potential to change the prognosis for countless patients.
Case Example: A study where AI analyzed retinal images to detect signs of diabetic eye disease demonstrated the technology's accuracy, often matching or surpassing human experts.
AI's Role in Global Health Initiatives
Bridging Expertise Gaps: In regions with limited medical expertise, AI serves as a critical resource, offering diagnostic support to healthcare providers and improving patient outcomes.
Healthcare Access: AI-powered mobile clinics and telemedicine services extend the reach of healthcare, ensuring communities worldwide have access to quality diagnostic care.
Limitations and Challenges of AI Diagnostic Models
Integration Hurdles: Incorporating AI into existing clinical workflows poses challenges, requiring careful planning and adjustment to ensure seamless operation.
Concerns Over Accuracy: Despite AI's potential, concerns persist regarding the accuracy of AI diagnoses, emphasizing the need for continuous validation and improvement.
Ethical Considerations: The reliance on AI for diagnosis raises ethical questions about accountability and transparency, necessitating clear guidelines and regulations.
The Future of AI in Diagnosis
Ongoing Research: As research progresses, AI models are evolving to become more sophisticated, promising improvements in their diagnostic capabilities.
Potential for Growth: The continued development of AI promises to enhance its diagnostic precision, potentially transforming healthcare into a more proactive, patient-centered discipline.
Collaborative Efforts: The future might see AI working in tandem with healthcare professionals, combining the strengths of both human intuition and machine intelligence for superior patient care.
AI's integration into the diagnostic process represents a monumental stride forward in medicine. As the technology continues to evolve, its potential to refine, accelerate, and personalize diagnosis is limitless, promising a future where every patient benefits from the precision and insight that AI brings to medicine.
AI Image Recognition and Medicine
Artificial intelligence has ingratiated itself into the fabric of medical diagnostics with a particular emphasis on image recognition. The partnership between AI and medicine not only streamlines workflows but also heralds a new chapter in patient care, where early detection and accuracy are paramount. Through advanced algorithms and deep learning, AI is reshaping the way medical images are analyzed, interpreted, and leveraged for better healthcare outcomes.
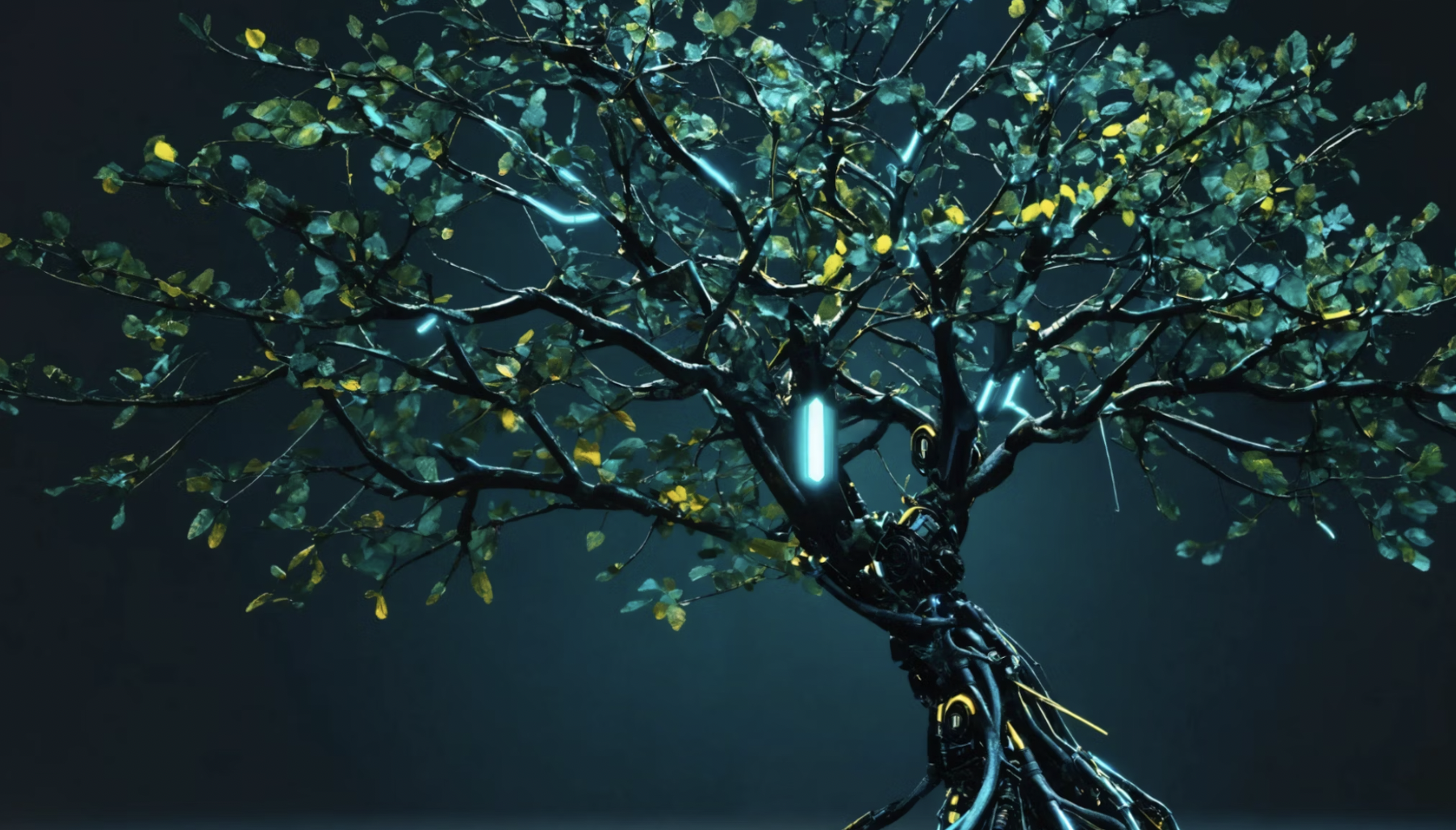
Want a glimpse into the cutting-edge of AI technology? Check out the top 10 research papers on computer vision (arXiv)!
Fundamentals of AI Image Recognition in Medical Imaging
AI image recognition technology stands at the forefront of a revolution in medical diagnostics, transforming raw data into actionable insights. Here's how:
Core Technology: At the heart of AI image recognition lies machine learning, where algorithms trained on vast datasets can recognize patterns and anomalies that may escape the human eye.
Application Across Modalities: Whether it's an X-ray, MRI, or CT scan, AI applies its learned intelligence to enhance image clarity, detect subtleties, and provide quantifiable data that supports diagnostic decisions.
Enhancing Radiologist Performance
AI does not replace radiologists; it empowers them. Here's the impact:
Precision and Speed: AI tools assist in identifying potential issues in medical images with a level of precision and speed that augments the capabilities of human radiologists.
Workload Management: With AI's help, radiologists can manage larger volumes of images, focusing their expertise on cases that require deeper analysis.
Collaborative Growth: AI Firms and Medical Institutions
Innovative partnerships are the catalysts for progress in AI and medicine:
Case in Point: Collaborations between AI companies and healthcare providers have led to the development of advanced diagnostic tools, such as AI platforms that enhance the detection of diseases like cancer, directly impacting patient survival rates.
Shared Expertise: These partnerships are a testament to the symbiotic relationship between AI developers and medical professionals, ensuring that technology evolves in line with clinical needs.
Deep Learning: The Next Frontier in Medical Image Interpretation
Deep learning is the engine driving the future of medical imaging:
Algorithm Evolution: Through deep learning, AI algorithms self-improve, becoming more adept at interpreting complex medical images and providing increasingly accurate diagnostic support.
Patient Outcomes: The direct beneficiary of this technological advancement is the patient, whose diagnosis and subsequent treatment rest on the clarity and accuracy of their medical images.
AI and Human Expertise: Striking the Right Balance
The marriage of AI and human judgment is critical to maintaining the integrity of medical diagnostics:
Quality Control: While AI provides a high throughput of analyzed images, radiologists bring their critical eye and experience to ensure the highest quality of diagnostic reporting.
Feedback Loop: Radiologists provide essential feedback to AI developers, facilitating the refinement of image recognition algorithms.
Remote Diagnostics: AI's Role in Expanding Access to Care
AI has a particularly transformative role in remote and underserved regions:
Access to Expertise: In areas with a dearth of radiologists, AI serves as an extension of the limited medical staff, providing diagnostic support that was previously unavailable.
Telemedicine Integration: AI-enhanced imaging tools integrated into telemedicine platforms can bring specialist-level diagnostics to remote corners of the globe.
Pioneering Research and the Road Ahead for AI in Image Recognition
As research intensifies, the horizon for AI in medical imaging expands:
Sophisticated Algorithms: The development of more nuanced algorithms promises to push the boundaries of what AI can recognize and interpret in medical images.
Predictive Analytics: Looking beyond diagnosis, AI's future lies in predictive analytics, where it can anticipate health issues before they manifest, thereby shifting the focus from treatment to prevention.
In the dynamic interplay between AI and medicine, image recognition technology is a standout example of how artificial intelligence can enhance, rather than replace, human expertise. As algorithms grow more sophisticated and integrations with healthcare providers deepen, the promise of AI in medicine continues to unfold, bringing with it a future where diagnostics are more accurate, accessible, and predictive than ever before.
AI Notetakers for Doctors
With the integration of AI into healthcare, an intriguing development is the rise of AI notetakers. These technological assistants are redefining the landscape of medical documentation, offering a striking balance between efficiency and accuracy that is reshaping the roles of healthcare providers and the care they offer to patients.
Functionality and Integration into Clinical Settings
AI notetakers serve as a bridge between healthcare professionals and the ever-growing demands of documentation:
Operational Mechanics: AI notetakers utilize advanced algorithms to transcribe spoken language into text, capturing patient consultations and medical observations with remarkable accuracy.
Seamless Integration: These tools are designed to meld with existing clinical workflows, often running in the background during patient interactions to minimize disruptions.
Efficiency Boost: By automating the process of note-taking, AI notetakers free up doctors to focus their attention where it's most needed — on the patient.
Accuracy and Reliability in Medical Terminology
The precision with which AI notetakers handle medical jargon is a testament to their potential:
Terminology Mastery: These systems are tailored to comprehend the complex lexicon of medicine, from pharmacological terms to surgical procedures.
Patient Interaction Nuance: Beyond terms, AI notetakers adeptly capture the nuances of patient-doctor interactions, ensuring that subtle yet critical information doesn't get lost in translation.
Impact on Healthcare Documentation
AI notetakers are poised to revolutionize medical record-keeping:
Error Reduction: With AI's attention to detail, the potential for human error in documentation diminishes, leading to more accurate medical records.
Compliance Adherence: These tools help maintain records that are compliant with healthcare regulations, which is crucial for both legal integrity and patient care.
Time-Saving Benefits
The implementation of AI notetakers heralds significant time savings for medical professionals:
Shift in Focus: Doctors can reclaim hours previously spent on paperwork, redirecting that time towards patient care and other critical tasks.
Burnout Mitigation: The reduction in administrative workload contributes to lower rates of physician burnout, promoting a healthier work-life balance.
Interoperability with Existing EHR Systems
The value of AI notetakers is amplified by their ability to work in tandem with electronic health record systems:
Data Synchronization: AI notetakers can synchronize with EHRs, ensuring that all patient information is updated in real-time and is readily accessible.
Integration Challenges: Despite the benefits, integrating AI notetakers with diverse EHR systems presents challenges, including compatibility issues and learning curves for medical staff.
Feedback from Healthcare Professionals
Insights from those on the front lines underscore the transformative nature of AI notetakers:
Positive Reception: Many healthcare professionals have embraced AI notetakers, citing improvements in workflow and patient engagement.
Areas for Improvement: Feedback also points to the need for enhancements in understanding diverse accents and dialects, as well as the handling of multiple speakers in a single session.
Future Developments in AI Notetaking Technology
The horizon for AI notetaking technology is brimming with possibilities:
Voice Recognition Advancements: The integration of more sophisticated voice recognition capabilities will further streamline the documentation process.
Natural Language Processing: Improvements in natural language processing will enable AI notetakers to better understand context, sentiment, and the subtleties of human speech, paving the way for richer, more accurate medical documentation.
In essence, AI notetakers are carving a niche in healthcare management, simultaneously addressing the twin imperatives of efficiency and care quality. As technology advances, these tools are set to become an indispensable part of the medical ecosystem, enabling healthcare professionals to devote their expertise to what truly matters—the well-being of their patients.
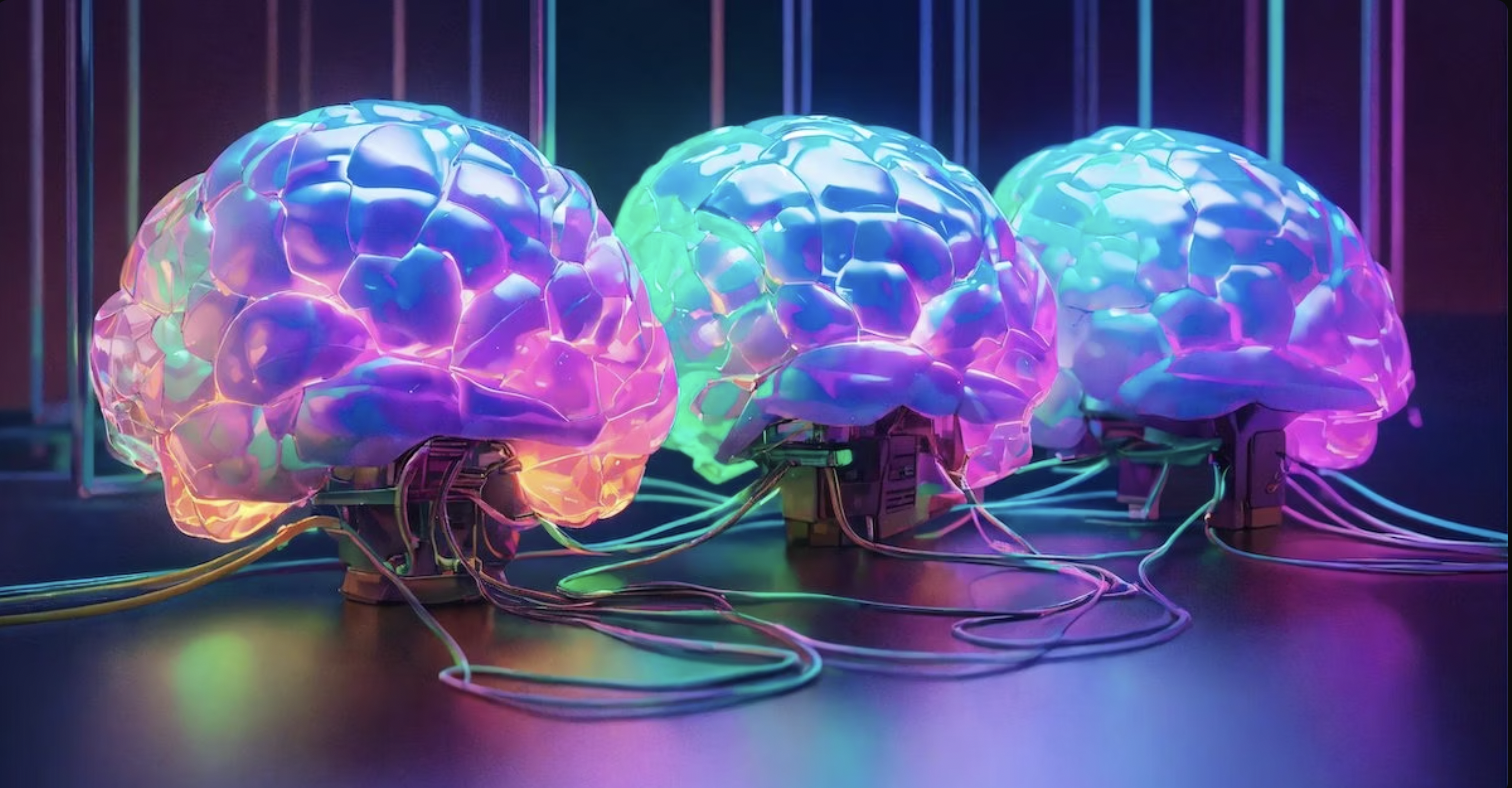
Mixture of Experts (MoE) is a method that presents an efficient approach to dramatically increasing a model’s capabilities without introducing a proportional amount of computational overhead. To learn more, check out this guide!