Affective AI
This blog post delves into the intriguing world of Affective AI, offering a comprehensive overview of its development, the core components that make it tick, and the ethical considerations that accompany its use.
Have you ever imagined a world where machines understand how you feel? It sounds like something straight out of a science fiction novel, yet, this is the reality we are stepping into with the advent of Affective AI. Each day, countless interactions with technology leave a digital footprint of our emotional states, but traditional AI systems lack the nuances to interpret these cues. Affective AI bridges this gap by integrating the sensitivity to understand human emotions, marking a significant evolution from conventional artificial intelligence systems. This blog post delves into the intriguing world of Affective AI, offering a comprehensive overview of its development, the core components that make it tick, and the ethical considerations that accompany its use. From the seminal work of Rosalind Picard in 1995 to the latest advancements in machine learning and sensor technology, we will explore how Affective AI is transforming user experiences across various industries. Ready to discover how machines are learning to empathize and what this means for the future of human-computer interaction?
Introduction to Affective AI
Affective AI stands at the forefront of a major technological revolution, where machines are not just tools but partners capable of understanding human emotions. This branch of artificial intelligence, also known as emotion AI or affective computing, seeks to develop systems that can detect, interpret, and respond to human emotions, thereby enhancing user experiences across diverse platforms. At its core, Affective AI is an interdisciplinary endeavor, merging insights from computer science, psychology, and cognitive science to create more empathetic and intuitive machines.
The journey of Affective AI began with a milestone paper by Rosalind Picard in 1995, laying the groundwork for affective computing. Since then, significant technological advancements have catalyzed its development:
Sensor technology has become more sophisticated, allowing for precise detection of emotional cues through facial expressions, voice patterns, and physiological signals.
Machine learning algorithms have evolved, enabling systems to learn from vast datasets and improve their accuracy in emotion recognition and understanding.
Data processing capabilities have expanded, supporting the complex computational needs of Affective AI systems.
The main components that constitute an Affective AI system include:
Emotion recognition: Identifying human emotions from various inputs.
Emotion understanding: Interpreting the context and significance of these emotions.
Emotion simulation: Simulating emotions to create more natural interactions.
Emotion response: Generating appropriate responses based on the emotional analysis.
Methods for emotion detection are diverse, ranging from analyzing facial expressions and voice patterns to processing physiological signals like heart rate and skin conductance. These methods underscore the versatility and adaptability of Affective AI in understanding human emotions.
However, the development and application of Affective AI are not without ethical concerns. Issues such as privacy, consent, and the potential for emotional manipulation need careful consideration. As we delve deeper into the capabilities of affective computing, these ethical considerations will play a crucial role in shaping its future and ensuring that it serves to enhance, rather than compromise, human dignity and autonomy.
The Mechanism of Affective AI
Delving into the intricate workings behind Affective AI unveils a complex interplay of technologies and methodologies designed to mimic human empathy through digital means. This section explores the foundational pillars that enable Affective AI systems to process and respond to human emotions, shedding light on the sophisticated mechanisms that allow machines to 'feel'.
The Role of Machine Learning and Deep Learning
Machine Learning (ML) and Deep Learning (DL) serve as the backbone of Affective AI, providing the framework for emotion recognition. By analyzing vast amounts of data, these systems learn to identify patterns and nuances in emotional expressions.
ML algorithms are trained on labeled datasets to recognize emotions based on facial expressions, voice tones, and body language. As the system encounters more data, its ability to categorize emotions becomes more nuanced and accurate.
DL techniques, especially Convolutional Neural Networks (CNNs), are adept at processing visual information, making them ideal for analyzing facial expressions and body language for emotion detection.
Optical Sensors and Computer Vision in Emotion Recognition
Optical sensors and computer vision algorithms are pivotal in identifying key facial features that signify emotional states. Technologies like Affectiva utilize these tools to analyze facial expressions in real-time, mapping them back to specific emotions.
The process involves capturing visual cues through cameras, followed by the extraction of facial landmarks (e.g., the corners of the mouth, the position of the eyebrows) using computer vision. These landmarks are then analyzed to infer emotional states.
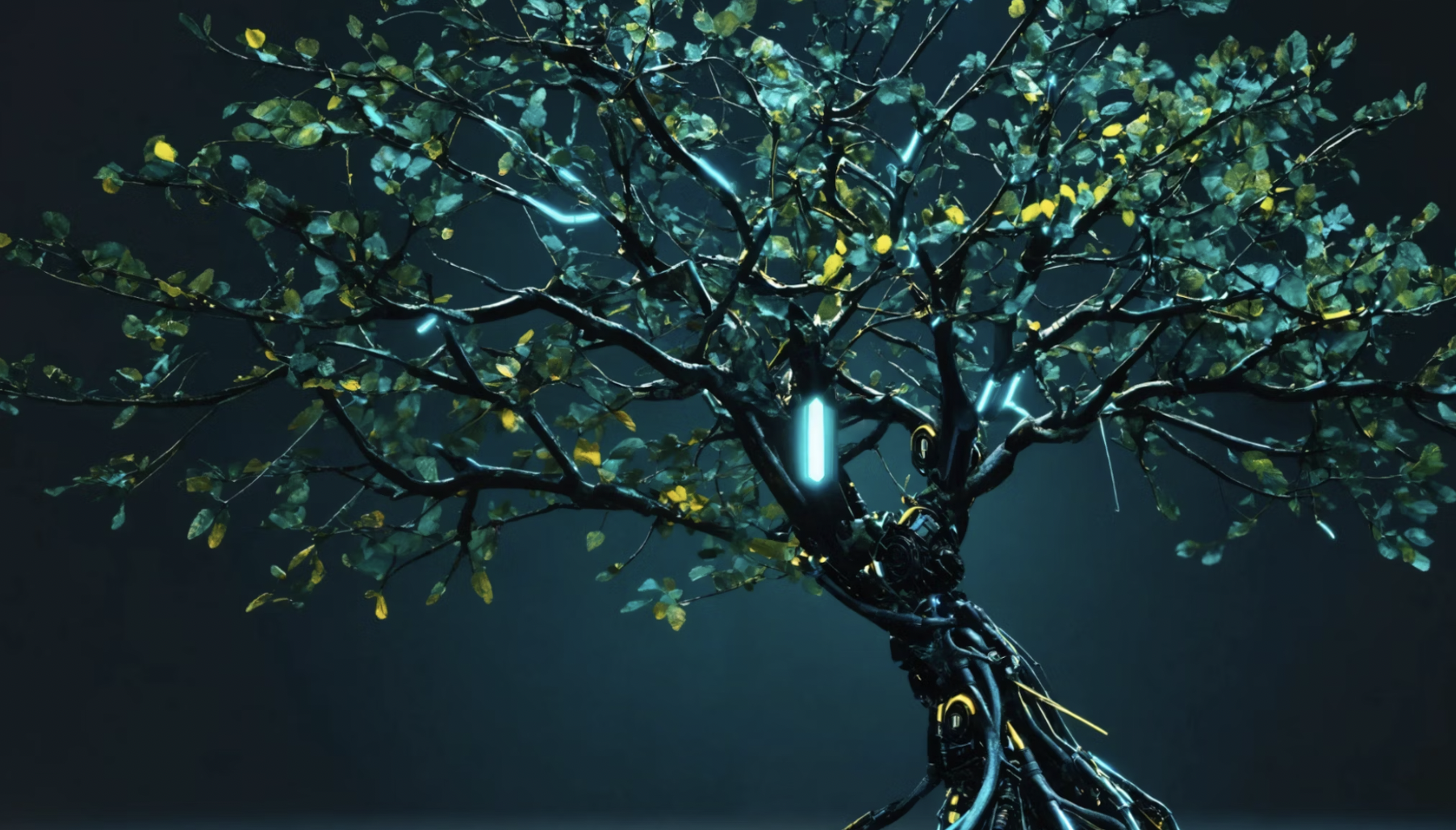
The Importance of Diverse Datasets
Large, diverse datasets are essential for training Affective AI systems with a high degree of accuracy and reliability. These datasets must encompass a wide range of emotional expressions across different demographics to avoid bias.
The diversity in the data helps the system understand and recognize emotions across different cultures, ages, and contexts, making Affective AI more universally applicable and sensitive to a broad spectrum of human emotions.
Mapping Emotions to Responses
Once an emotion is detected, Affective AI systems map these emotions to appropriate responses. This mapping is crucial for applications in digital assistants, educational software, and customer service bots, where the goal is to interact with users empathetically.
The response mechanism can range from changing the tone of voice in a digital assistant to adapting the difficulty level in educational software, depending on the user's emotional state.
Overcoming Challenges in Emotional Interpretation
Affective AI faces challenges in interpreting ambiguous or conflicting emotional signals. Human emotions are complex and can be expressed subtly, making it difficult for machines to always accurately interpret them.
Ongoing efforts to refine Affective AI's contextual understanding involve enhancing algorithms to better account for nuances in emotional expression and incorporating contextual cues to aid in the interpretation.
Integration into Conversational Agents and NLP Systems
The integration of Affective AI into conversational agents and natural language processing (NLP) systems marks a significant advancement towards creating more empathetic user interactions. By understanding and responding to user emotions, these systems can offer support, recommendations, or responses that are more aligned with the user's emotional state.
This integration is particularly impactful in customer service and mental health applications, where understanding and responding appropriately to emotions can greatly enhance the quality of interaction and support provided.
The journey of Affective AI from a concept to a transformative technology illustrates the power of machine learning, deep learning, and other computational techniques in bridging the gap between human emotions and digital experiences. By continuously refining the mechanisms behind emotion recognition and response, Affective AI paves the way for a future where technology not only understands but also empathizes with human emotions, leading to more meaningful and supportive human-computer interactions.
The Role of Affective AI in Various Industries
The transformative power of Affective AI extends far beyond its technical intricacies, revolutionizing industries by fostering deeper connections between humans and machines. This section explores how affective computing is reshaping diverse sectors, from healthcare and education to automotive and entertainment, highlighting its potential to enhance user experience and engagement profoundly.
Healthcare: Compassionate Care through Emotion Recognition
Mental Health Monitoring: Affective AI systems are pioneering new ways to support mental health by detecting subtle emotional cues indicative of depression, anxiety, or stress. Through voice and facial expression analysis, these systems offer real-time monitoring, alerting healthcare providers to changes in a patient's emotional state.
Patient Care and Therapy: Tailoring therapeutic approaches based on the emotional state of patients, Affective AI enhances the efficacy of treatments. It provides therapists and caregivers with insights into the patient's feelings, facilitating more personalized and empathetic care.
Education: Personalizing Learning Experiences
Adapting Content Delivery: Affective AI revolutionizes the learning environment by adapting educational content based on the student's emotional response. If a student shows signs of frustration or confusion, the system can modify the difficulty level or introduce alternative learning methodologies.
Enhancing Engagement: By recognizing emotions such as interest or boredom, Affective AI can adjust teaching methods to maintain student engagement, making learning more effective and enjoyable.
Business Services: Elevating Customer Service
Emotion-aware Chatbots: Integrating Affective AI into customer service, chatbots can now detect customer emotions during interactions. This capability allows for more nuanced responses, improving customer satisfaction by addressing not just the query but the emotional context as well.
Customer Feedback Analysis: Analyzing emotional responses in customer feedback, businesses can gain deeper insights into customer satisfaction and preferences, enabling more focused and effective improvements in services and products.
Media and Advertising: Tailoring Content to Emotional Responses
Audience Engagement: Affective AI analyzes emotional responses to media content, enabling creators and advertisers to tailor their strategies based on what resonates emotionally with their audience. This targeted approach enhances content engagement and effectiveness.
Personalized Advertising: By understanding emotional triggers, advertisers can craft messages that elicit the desired response, making advertising campaigns more impactful and relevant to the audience.
Automotive Industry: Enhancing Safety with Emotion Recognition
Monitoring Driver State: Emotion recognition technology in vehicles can monitor signs of fatigue, stress, or distraction, contributing significantly to driver safety. By alerting drivers or taking corrective actions, such as activating autonomous safety features, the risk of accidents can be reduced.
Entertainment and Gaming: Creating Immersive Experiences
Interactive Gaming: Affective AI introduces a new dimension to gaming, where games respond to the player's emotions, tailoring challenges and narratives to create a deeply personalized and immersive experience.
Enhanced Storytelling: In entertainment media, emotion recognition can adapt storylines in real-time, providing viewers with a unique experience that resonates with their current emotional state, making content more engaging and memorable.
As Affective AI continues to evolve, its integration across various industries promises not only to enhance user experience but also to foster a more empathetic interaction between humans and machines. Through its ability to understand and respond to human emotions, Affective AI is set to redefine the landscape of technological innovation, making our digital interactions more human, more understanding, and ultimately, more connected.
Companies Pioneering in Affective AI
The realm of Affective AI has seen remarkable contributions from both established tech giants and innovative startups. These entities are not just shaping the future of emotional intelligence in machines; they are redefining how humans interact with technology across various sectors. Let’s delve into the pioneering work of these companies.
IBM and Microsoft: Leaders in Emotion Recognition Systems
IBM has been at the forefront, leveraging its Watson platform to analyze tones and emotions in text. This system aids businesses in understanding customer sentiments, enabling personalized customer service experiences. IBM's research into emotional intelligence extends to healthcare, where its AI can detect emotional well-being through speech patterns.
Microsoft, with its Cognitive Services, offers an Emotion API that developers use to create applications that can recognize a range of emotions from facial expressions. This technology enhances user experience in gaming, where players’ emotions can influence the game narrative, and in customer service, where chatbots can respond empathetically to customer inquiries.
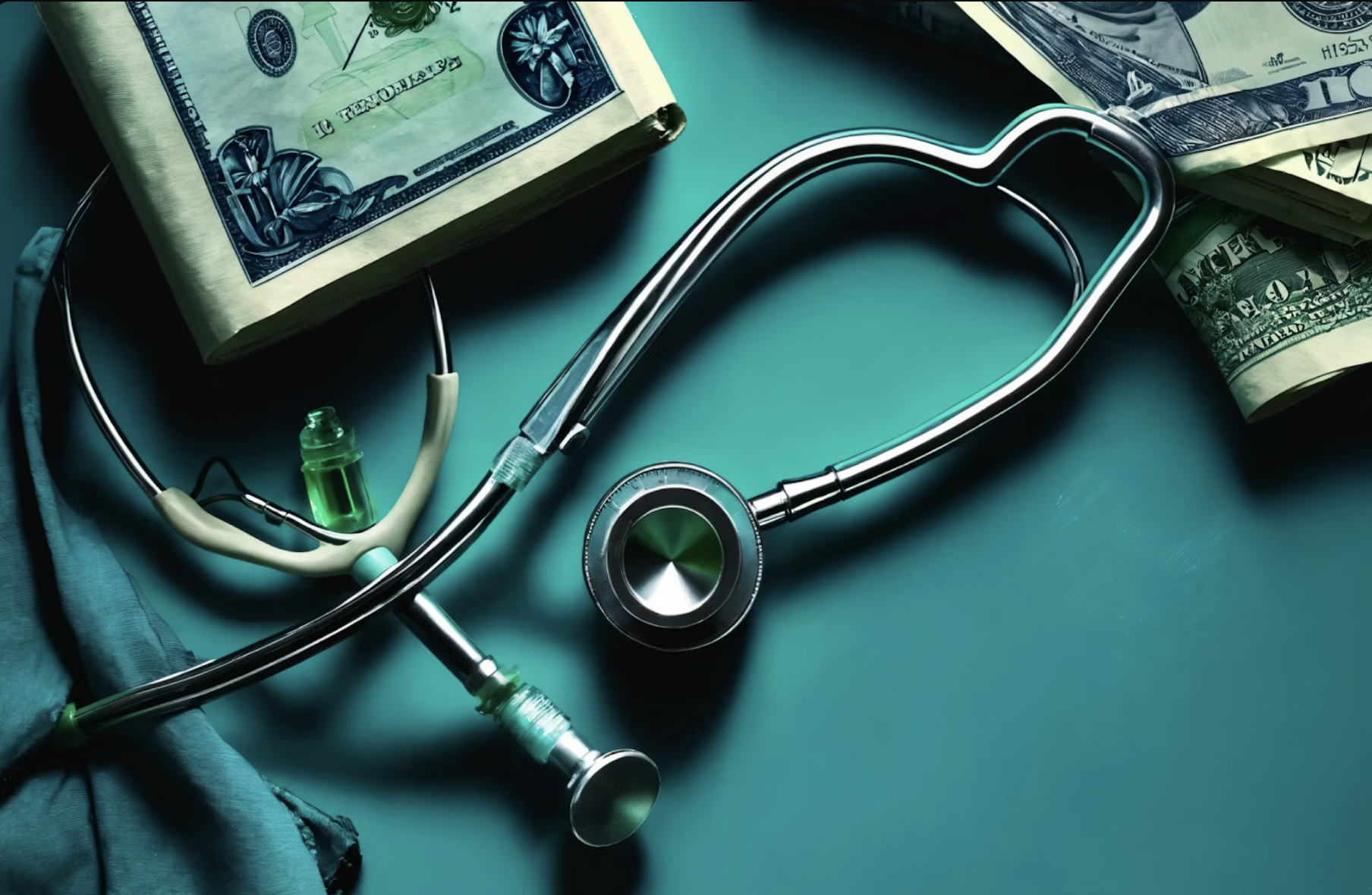
Affectiva and Beyond Verbal: Specializing in Emotion Recognition
Affectiva, derived from MIT’s Media Lab, excels in emotion recognition through facial expressions and voice analysis. Its automotive AI measures drivers’ emotional states to improve safety. In market research, Affectiva’s technology provides insights into consumer emotional engagement with advertisements, enhancing marketing strategies.
Beyond Verbal focuses on voice-driven emotions analytics, exploring the emotional undertones in vocal intonations. This technology finds its application in healthcare for monitoring patient wellness and in customer service platforms to adjust responses based on the customer’s emotional state.
Kairos: Advancing Facial Recognition for Emotion Analysis
Kairos offers facial recognition technology that identifies and analyzes emotional expressions, offering insights into how people interact with digital content, products, and services. Its applications span from security, by enhancing verification processes, to retail, where customer reactions to products can offer valuable feedback.
NuraLogix: Innovating with Transdermal Optical Imaging Technology
NuraLogix employs transdermal optical imaging technology to detect emotions. This non-invasive method analyzes facial blood flow changes captured on standard video to infer emotional states. This groundbreaking approach finds applications in health assessments, mental health monitoring, and even in banking, for stress detection during critical decision-making.
Gestigon GmbH: Enhancing Gesture-Based Interaction Systems
Gestigon GmbH contributes to the development of gesture-based interaction systems that include emotion recognition capabilities. This technology enhances user interfaces in automotive and consumer electronics by allowing natural, intuitive interactions and adjusting system responses to the user’s emotional cues.
These companies are not just developing technology; they are cultivating a future where machines understand and react to human emotions, fostering a world where technology enhances human experience in profound and meaningful ways. Through their innovations, Affective AI is set to transform industries, making our interactions with machines more natural, intuitive, and, importantly, more human.
The Potential and Limitations of Affective AI
Affective AI, a transformative technology at the intersection of artificial intelligence and emotional intelligence, promises to redefine human-computer interaction. Its potential to understand and respond to human emotions paves the way for advancements in various sectors, including mental health care, education, and customer service. However, this groundbreaking technology also encounters significant challenges and ethical dilemmas.
Enhancing Human-Computer Interaction
Improvements in Mental Health Care: Affective AI can detect subtle emotional cues in speech or facial expressions, offering groundbreaking tools for monitoring mental health conditions such as depression and anxiety. This technology can provide real-time feedback to therapists and patients, facilitating more effective interventions.
Personalized Education: By understanding and responding to the emotional state of students, affective AI can tailor educational content to keep learners engaged and motivated. This personalization can address learning challenges, making education more accessible and effective for students with diverse needs.
Revolutionizing Customer Service: Emotion-aware chatbots and virtual assistants, powered by affective AI, can provide customers with empathetic and personalized experiences. This enhances customer satisfaction and loyalty, as interactions become more understanding and human-like.
Confronting Limitations and Ethical Concerns
Accuracy and Cultural Biases: Current affective AI technologies face challenges in accurately recognizing emotions across different cultures due to variations in emotional expression. This raises concerns about cultural bias, where the technology might misinterpret emotions in multicultural contexts.
Complexity of Human Emotions: The nuanced nature of human emotions, often ambiguous and context-dependent, poses a significant challenge for affective AI systems. Developing algorithms that can understand these subtleties and respond appropriately remains a daunting task.
Privacy and Consent: The collection and analysis of emotional data raise critical privacy concerns. Ensuring that individuals' consent is informed and voluntary is paramount to maintaining trust and respecting personal boundaries.
Navigating Ethical and Social Implications
Risk of Emotional Manipulation: The ability of affective AI to understand and influence emotions introduces the potential for manipulation. Safeguards must be in place to prevent the misuse of this technology in ways that could exploit individuals' emotional vulnerabilities.
Maintaining the Human Element: Relying on machines to interpret and respond to human emotions carries the risk of dehumanizing social interactions. It's crucial to strike a balance, ensuring that affective AI complements rather than replaces the richness of human connection.
Creating Universally Applicable Systems: The subjective nature of emotions and cultural differences in expression pose challenges in developing affective AI systems that are universally applicable. Embracing diversity and inclusivity in the design and training of these systems is essential to their success and acceptance.
Affective AI stands at the forefront of a revolution in human-computer interaction, promising to make our interactions with technology more intuitive and empathetic. As we navigate its potentials and limitations, the ethical considerations and societal implications of affective AI demand thoughtful exploration and responsible innovation. Ensuring that this technology enhances rather than diminishes the human experience remains a paramount concern as we shape the future of affective AI.
The Future of Affective AI
The trajectory of Affective AI is on an exciting path, with potential breakthroughs and broader implications that promise to reshape our society and technology landscape. As we delve into the future, several key aspects emerge, highlighting the transformative power and potential challenges of Affective AI.
Ongoing Research and Technological Advancements
Multimodal Emotion Recognition Systems: Efforts are underway to enhance the accuracy of Affective AI by integrating multimodal emotion recognition systems. These systems combine visual cues, auditory signals, and physiological data to interpret human emotions more accurately. This holistic approach aims to address current limitations in recognizing subtle emotional states across different individuals and cultures.
Improving Contextual Understanding: Researchers are focusing on improving Affective AI's ability to understand the context behind emotional expressions. This includes taking into account the environment, social situation, and cultural background, which are crucial for accurately interpreting human emotions.
Expansion into New Industries and Domains
Public Safety: Affective AI is finding applications in public safety, helping to detect distress or anxiety in crowded spaces or during emergency situations. This could enable quicker response times and more tailored interventions in critical moments.
Social Media: On social media platforms, Affective AI can enhance user experience by detecting emotional responses to content, enabling platforms to deliver more personalized and emotionally resonant experiences.
Smart Homes: In smart homes, Affective AI can make living spaces more intuitive by adjusting lighting, temperature, and music based on the occupants' mood, creating a more comfortable and supportive environment.
The Role in Human-Machine Collaboration
Understanding and Responding to Human Emotions: As machines become more adept at understanding human emotional states, we can expect more empathetic responses from AI systems. This could redefine human-machine collaboration, with AI systems acting as more than just tools, but as entities that can offer support and understanding.
Advancing Collaborative Workspaces: In collaborative workspaces, Affective AI can enhance teamwork by sensing group emotions and adjusting tasks or suggesting breaks to maintain a positive and productive atmosphere.
Societal Implications
Job Roles Transformation: The widespread adoption of Affective AI will inevitably lead to changes in job roles, with new skills in demand for managing and interpreting emotional data. Emotional intelligence will become a key skill, alongside technical expertise in AI and machine learning.
Privacy and Ethical Considerations: As Affective AI becomes more integrated into our lives, privacy concerns will come to the forefront. Ensuring that emotional data is collected and used ethically will be crucial. Public engagement and clear ethical guidelines will be necessary to navigate these challenges.
Deepening Human Empathy: Interestingly, Affective AI has the potential to deepen human empathy by encouraging designers, developers, and users to consider and understand emotional states more deeply. This could lead to a more empathetic society overall.
The Importance of Interdisciplinary Collaboration
Bringing Together Diverse Expertise: The development of Affective AI requires the collaboration of experts from technology, psychology, ethics, and other fields. Such interdisciplinary collaboration can ensure that Affective AI systems are designed with a deep understanding of human emotions, ethics, and social implications.
Shaping the Future with Responsible Innovation: As we move forward, it's crucial to foster responsible innovation in the field of Affective AI. This includes developing ethical guidelines, engaging the public in conversations about the technology's impact, and ensuring that Affective AI serves to enhance human well-being.
The journey of Affective AI is just beginning, and its potential to transform our interactions with technology and each other is immense. As we venture into this future, the need for thoughtful, ethical, and collaborative approaches to development and implementation cannot be overstated. The promise of Affective AI lies not just in its technological advancements, but in its potential to foster a more empathetic and understanding society.
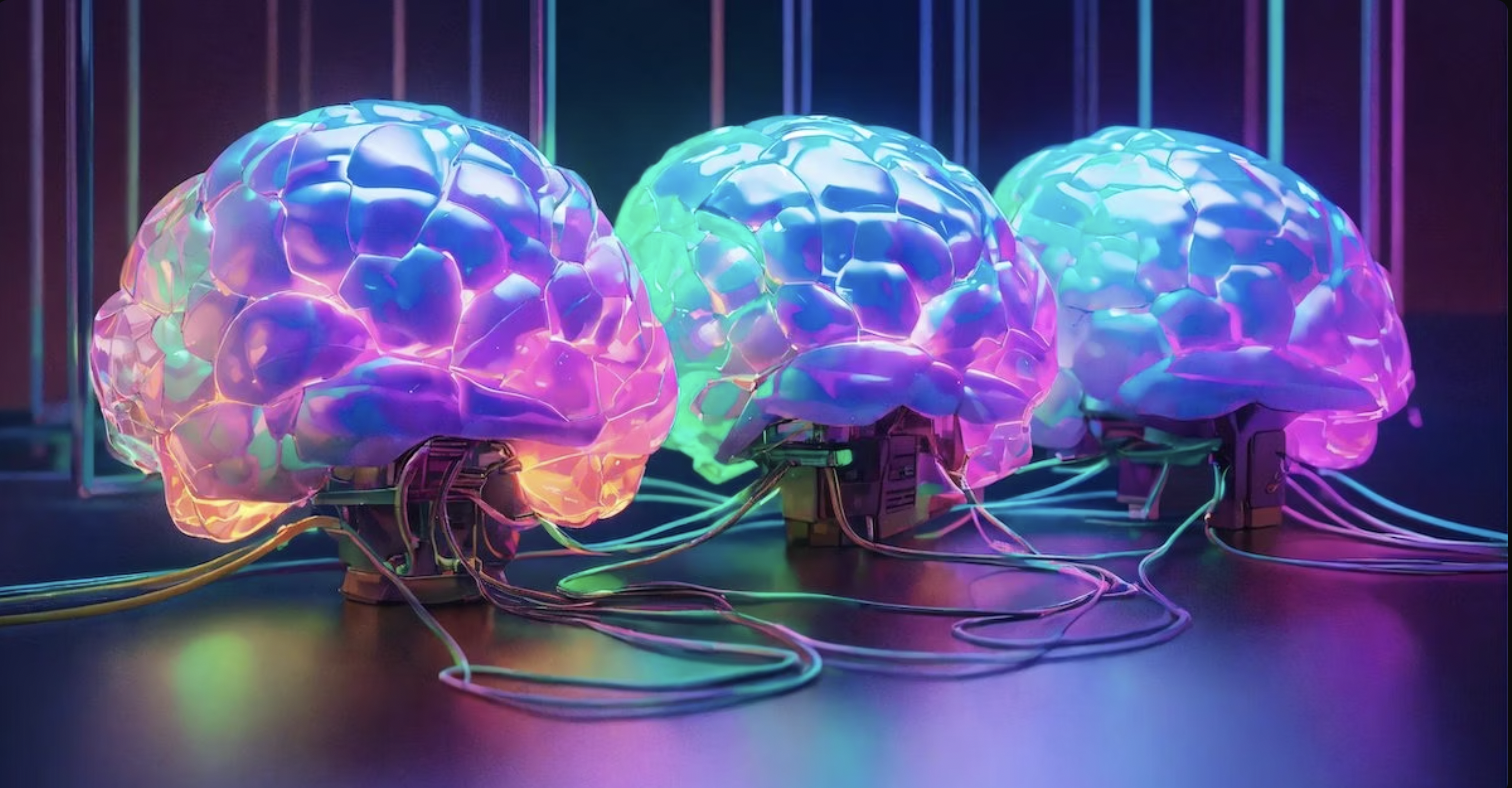