Learning To Rank
Learning to Rank transforms the way digital platforms interact with user queries, leveraging machine learning models to rank a list of items for relevance.
Did you know that the effectiveness of your digital platform could hinge on something as intricate yet pivotal as the order in which search results appear? The digital realm thrives on relevancy—whether it's a search engine, an e-commerce site, or a recommendation system, the quest to deliver the most relevant information to the user is relentless. This is where the concept of "learning to rank" (LTR) comes into play, revolutionizing how systems evaluate and present data. LTR stands as a cornerstone in enhancing site search relevancy by effectively ordering query results. But what sets this approach apart from traditional ranking algorithms, and why does it matter to you?
What is Learning to Rank
Learning to Rank transforms the way digital platforms interact with user queries, leveraging machine learning models to rank a list of items for relevance. Unlike traditional ranking algorithms that follow predefined rules, LTR employs supervised machine learning to dynamically order search results or recommendations based on historical data and user interactions. Let's delve deeper:
Fundamental Concept: At its core, LTR applies machine learning techniques to rank items in order of relevance to a query. This is not just about finding relevant items; it's about ordering them in a way that maximizes user satisfaction and engagement.
Improving Site Search Relevancy: Referencing insights from Lucidworks, the purpose of LTR extends beyond mere ranking—it enhances user experience by ensuring that the most relevant results top the list. This is critical for businesses and platforms that rely on precision and personalization to retain users.
Supervised Machine Learning: The distinction between LTR and traditional ranking algorithms lies in the application of supervised machine learning. LTR models learn from past queries and their outcomes, continuously improving their ability to predict and rank future queries more accurately.
Broad Applications: The impact of LTR spans various contexts, from search engines and recommendation systems to online advertising. It's a versatile approach that caters to diverse ranking problems across digital platforms, making it a valuable tool in the arsenal of data scientists and engineers.
Ranking Problems: Central to many digital platforms, ranking problems involve ordering a list of items or content based on certain criteria. LTR addresses these problems by learning from data, offering a dynamic and adaptive solution that traditional algorithms cannot match.
Learning to Rank stands as a beacon of progress in the quest for relevancy and user satisfaction on digital platforms. By understanding its fundamental principles and applications, we can appreciate the complexity and elegance of modern information retrieval systems. Are you ready to explore how LTR can revolutionize your platform's approach to data ranking and relevancy?
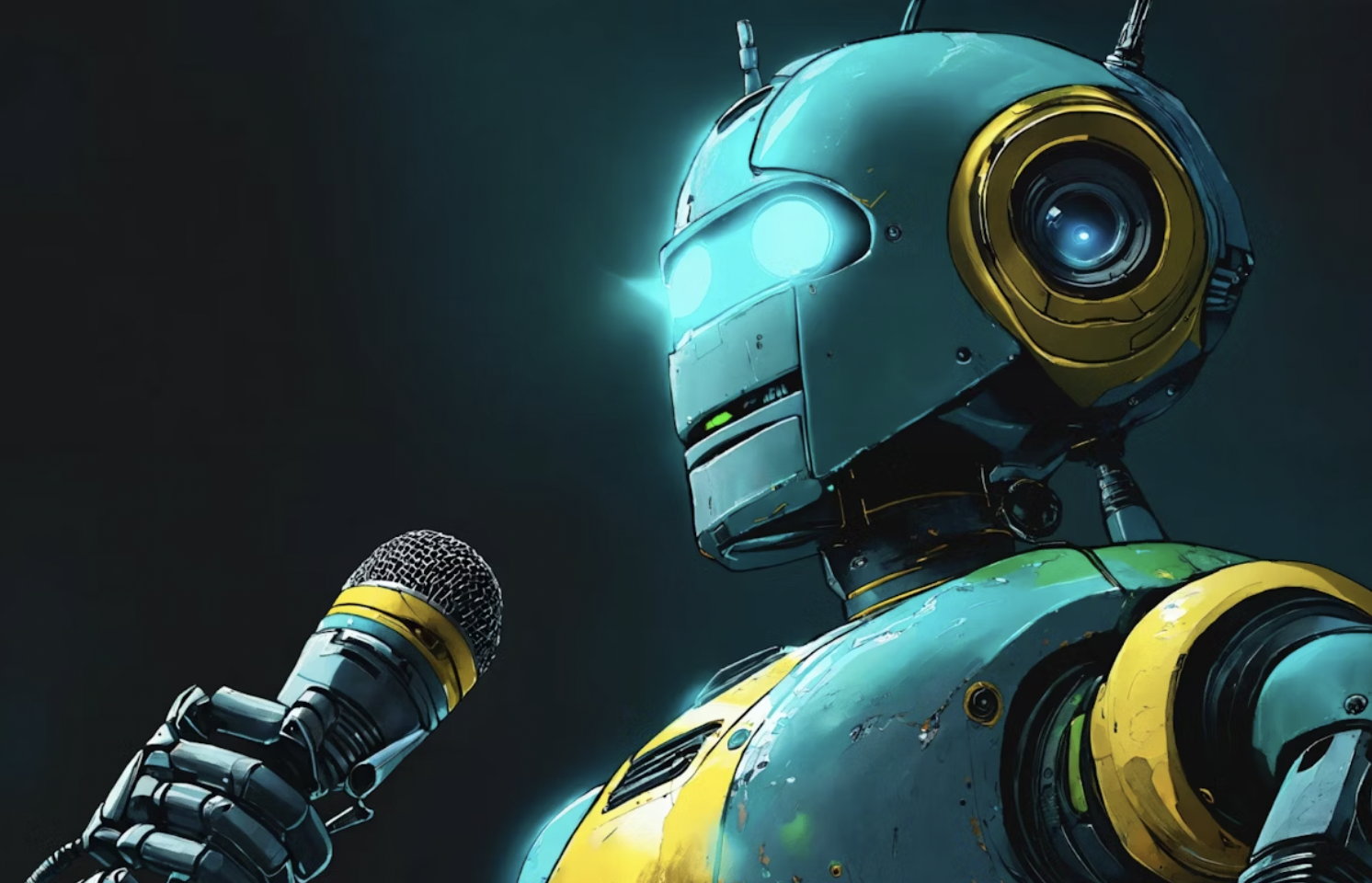
How Learning to Rank Works
Delving into the mechanics of Learning to Rank (LTR) unveils the intricate process of how machine learning models prioritize and sequence information, ensuring the highest relevance and value to the user's query. This exploration begins with understanding the foundational elements — training data, feature engineering, the training phase, evaluation measures, and the iterative nature of LTR models.
Training Data in LTR
Training data serves as the cornerstone for LTR models, consisting of three critical components:
Features: These are the attributes or characteristics of items that could influence their relevance to a query. Features could range from textual content, such as keywords or tags, to user interaction metrics like click-through rates or time spent on a page.
Queries: Representing the user's search intent, queries are what tie features to relevance judgments. They provide context to the LTR model, helping it understand what users are looking for.
Relevance Judgments: These are assessments of how well an item meets the search query's intent. Typically graded on a scale (e.g., from not relevant to highly relevant), these judgments train the model to discern the relevance of items to queries.
Feature Engineering and Selection
Feature engineering involves the creation and optimization of features that improve the model's ability to predict item relevance. This process is critical for effective LTR models:
Textual Features: Including keyword density, topic distribution, and metadata such as author or publication date. These features help the model understand the content's relevance to the query.
User Interaction Metrics: Click-through rates, time on page, and bounce rates are invaluable for gauging user satisfaction, offering indirect signals of relevance.
Selection: Not all features contribute equally. The selection process involves identifying which features are most predictive of relevance, often through techniques like feature importance analysis.
The Training Phase
During the training phase, LTR models learn to predict the relevance of items based on historical data:
Models are fed with training data that includes features of items, user queries, and relevance judgments.
Machine learning algorithms analyze this data, learning patterns and relationships between features and their relevance to queries.
The goal is to develop a predictive model that can accurately assign relevance scores to items for new, unseen queries.
Evaluation Measures in LTR
To ensure models accurately predict relevance, LTR relies on specific evaluation measures:
Precision and Recall: Precision measures the relevance of the retrieved documents, while recall assesses how many relevant documents are retrieved. High precision and recall indicate a model effectively ranks relevant items higher.
Normalized Discounted Cumulative Gain (NDCG): This metric accounts for the position of relevant items in the search results, emphasizing higher ranks for more relevant items. It's particularly useful in scenarios where the order of results is paramount.
Iterative Nature of LTR
LTR models are not static; they evolve through continuous refinement:
Retraining: New data, user feedback, and shifting user behaviors necessitate regular model updates to maintain and enhance performance.
Refinement: Ongoing analysis identifies areas for improvement, whether in feature engineering, model architecture, or evaluation measures.
Iterative cycles ensure that LTR models adapt to the dynamic nature of user queries and preferences, maintaining their effectiveness over time.
Through these stages, Learning to Rank emerges as a sophisticated approach to sorting and presenting data, driven by machine learning's power to adapt and learn from vast amounts of information. This process ensures that users encounter the most relevant, valuable content first, enhancing their digital experiences across platforms.
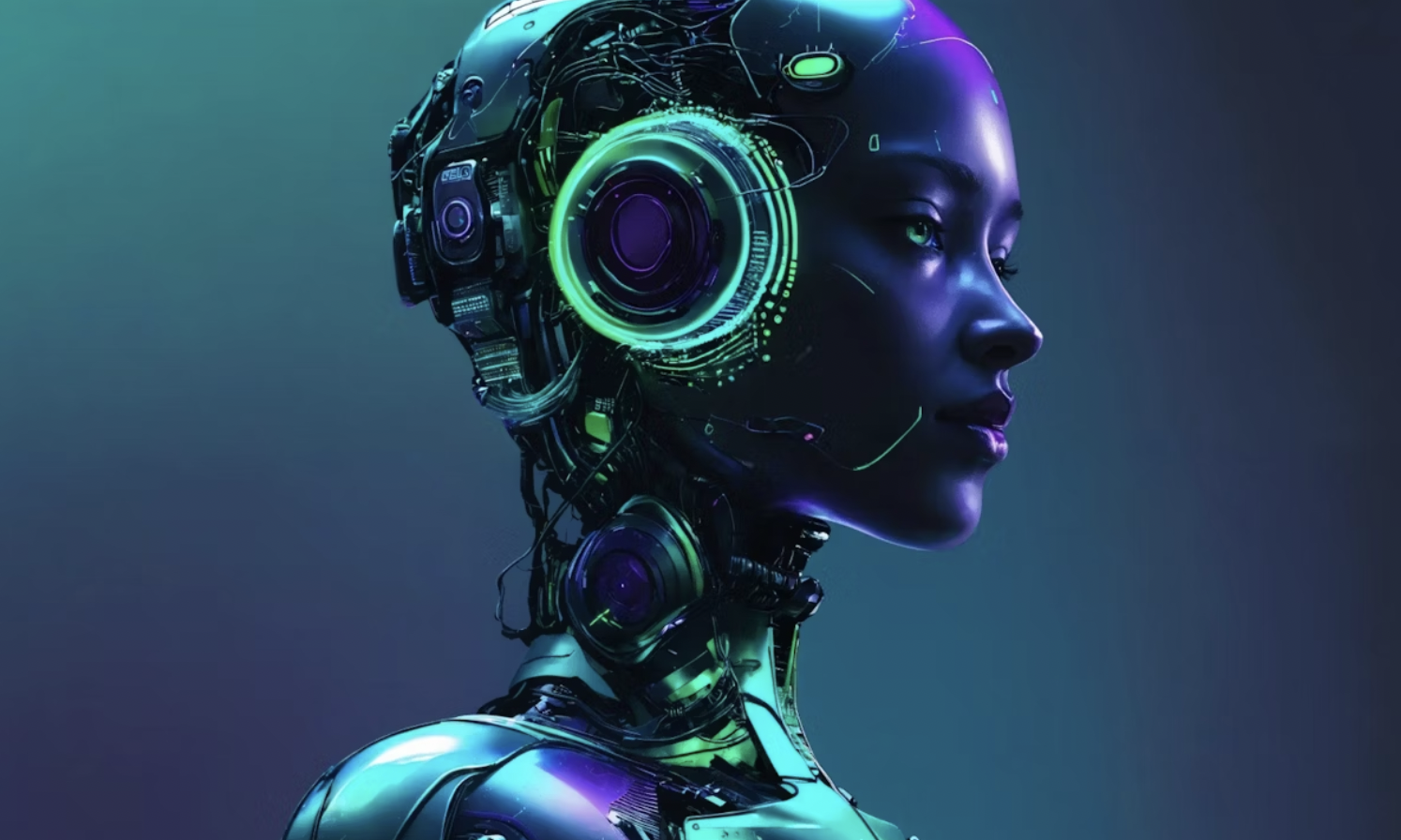
Approaches Used in Learning to Rank
Learning to Rank (LTR) algorithms revolutionize how systems process and present data by leveraging machine learning to prioritize information. These methodologies are not only foundational to enhancing search engine performance but also pivotal in optimizing recommendation systems and online advertising. Understanding the three primary LTR approaches—pointwise, pairwise, and listwise—reveals the intricacies and effectiveness of each method in tackling ranking challenges.
Pointwise Approaches
Pointwise approaches in LTR focus on evaluating individual items based on their relevance to a query. This method simplifies the ranking problem to a regression or classification task.
Relevance Scoring: Each item receives a relevance score that indicates its applicability to the user's query. These scores are often derived from features like keyword matches, site engagement metrics, or user preferences.
Ranking Generation: The system then uses these scores to order the items, with higher-scoring items appearing first. It's a straightforward approach that prioritizes direct relevancy.
Trade-offs: While pointwise methods are simpler and computationally less intensive, they might not fully capture the complexities of ranking items relative to each other. This approach works well for scenarios where the goal is to filter out irrelevant items rather than to fine-tune the ordering of highly relevant items.
Pairwise Approaches
Pairwise approaches elevate the LTR process by comparing pairs of items to judge which one is more relevant to a given query. This method shifts the focus from scoring individual items to evaluating item pairs.
Comparison-Based Ranking: By determining the preference between two items, pairwise methods can infer an item's rank relative to others in the dataset. This process is akin to a tournament where items are pitted against each other to establish a hierarchy of relevance.
Advantages and Challenges: Pairwise approaches better capture the relative preferences inherent in ranking tasks. However, they can be computationally demanding since the number of possible item pairs grows exponentially with the size of the dataset.
Applicability: These methods are particularly useful in scenarios where the precise ordering of items matters more than just their individual relevance scores.
Listwise Approaches
Listwise approaches consider the entire set of items as a single entity for optimization. This perspective aligns closely with the ultimate goal of LTR—optimizing the order of a list of items to match the user's intent.
Optimizing the Entire List: Unlike pointwise and pairwise methods, listwise approaches directly optimize the final ranking metric, whether it's NDCG, Precision@K, or another relevant measure. This holistic view allows for a more nuanced understanding of item interrelations.
Complexity and Performance: These methods can provide superior ranking quality by considering the list as a whole but at the cost of increased complexity and computational resources.
Recent Advancements: The introduction of deep learning models into listwise LTR has significantly enhanced its ability to handle complex ranking problems. Neural networks, with their capacity to model intricate patterns and relationships, have become instrumental in pushing the boundaries of what's possible with listwise LTR.
Trade-offs and Recent Advancements
Choosing between pointwise, pairwise, and listwise approaches involves balancing complexity, performance, and the specific requirements of the ranking problem at hand.
Complexity vs. Performance: While pointwise methods are less complex, they might not capture the nuances of item rankings as effectively as the more sophisticated pairwise and listwise approaches.
Recent Advancements: The emergence of neural networks and deep learning models has introduced new possibilities for LTR. These technologies offer powerful ways to model the intricate relationships between items and queries, enhancing the effectiveness of all three LTR approaches.
Deep Learning in LTR: By leveraging deep learning, practitioners can tackle more complex ranking tasks with unprecedented accuracy and efficiency. These models excel in environments where the relationships between items and their relevance to queries are deeply nuanced and highly dynamic.
The evolution of LTR through these methodologies underscores the field's ongoing commitment to refining how information is structured and presented. As machine learning continues to advance, so too will the sophistication and effectiveness of learning to rank algorithms, further enhancing our digital interactions and experiences.
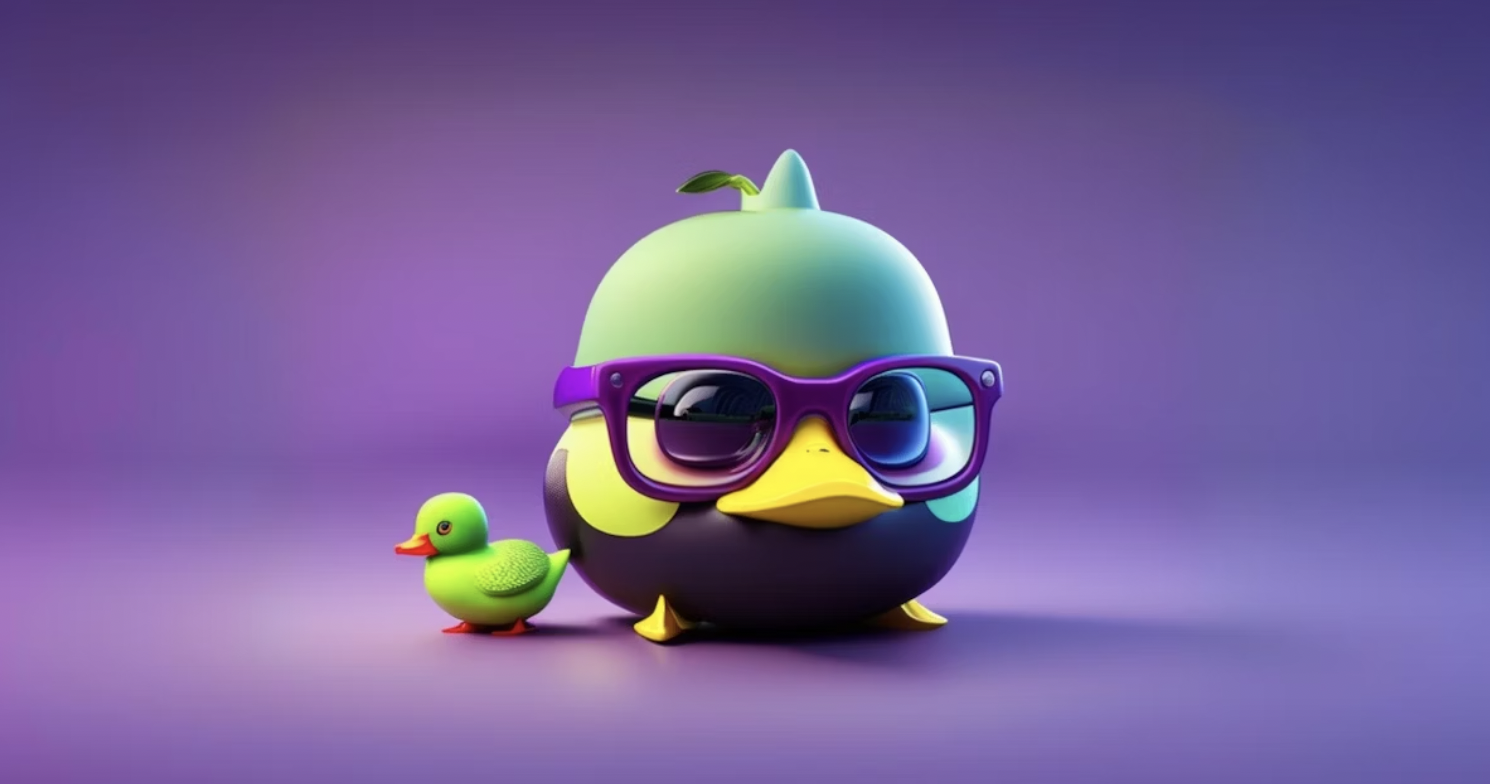
Implementing Learning to Rank
Learning to Rank (LTR) has evolved from a theoretical concept to a practical tool transforming the digital landscape, from enhancing search engine accuracy to refining recommendation systems. The journey from concept to implementation involves several critical steps, each requiring careful consideration and strategic planning. The experiences of industry leaders, as shared through platforms like the GitHub blog and insights from QTravel.ai, provide valuable blueprints for navigating the LTR implementation process.
Identifying a Ranking Problem
The first step in deploying LTR involves recognizing a ranking problem that affects user experience or business outcomes. Whether it's improving the relevancy of search results or enhancing the accuracy of recommendations, identifying the core issue is crucial.
Enhancing Search Functionality: For platforms with extensive content, making search results more relevant to user queries stands as a common ranking problem.
Improving Recommendations: In e-commerce or content platforms, tailoring recommendations to fit user preferences can significantly enhance user engagement.
Assessment of Current Systems: Understanding the limitations of existing ranking algorithms or systems helps in pinpointing areas for LTR application.
Gathering and Preparing Training Data
The foundation of any LTR system is the quality and diversity of its training data, which guides the model's learning process.
Data Diversity: Collecting a broad dataset that covers various user interactions and preferences ensures a more versatile model.
Relevance Judgments: Labeling data with relevance judgments, either manually or through user feedback, provides the ground truth for training LTR models.
Feature Engineering: Identifying and extracting meaningful features from the data, such as user behavior metrics or content attributes, is critical for model effectiveness.
Selecting an LTR Approach and Model
The choice of an LTR approach and the specific model to use depends on several factors, including the nature of the ranking problem and the computational resources available.
Approach Selection: Deciding between pointwise, pairwise, and listwise approaches based on the specific ranking task and desired outcomes.
Model Selection: Considering factors like data characteristics and computational resources when choosing between simpler models or more complex ones like neural networks.
Best Practices for Training LTR Models
Training LTR models requires attention to detail and adherence to best practices to ensure models are effective and robust.
Avoiding Overfitting: Implementing techniques such as cross-validation and regularization to ensure the model generalizes well to unseen data.
Feature Selection: Carefully selecting and periodically reviewing the features used for training to maintain model relevance and efficiency.
Continuous Evaluation and Model Updates
The digital environment is always changing, necessitating ongoing evaluation and updates to LTR models to maintain and improve ranking quality.
Regular Evaluation: Using metrics like Precision, Recall, and NDCG to assess model performance and identify areas for improvement.
Iterative Refinement: Continuously refining and retraining models with new data to adapt to changing user behaviors and preferences.
Case Studies of Successful LTR Implementations
The tangible benefits of LTR can be seen in numerous real-world applications, from search engines to recommendation systems and beyond.
GitHub: Leveraged LTR for improving issue recommendations, helping users find relevant issues more efficiently.
QTravel.ai: Applied LTR algorithms to enhance the relevance of travel recommendations, significantly improving user satisfaction and engagement.
Each of these examples underscores the transformative potential of LTR when applied thoughtfully and strategically. The challenges encountered—such as data collection, model selection, and ongoing optimization—highlight the importance of a methodical approach to LTR implementation. Yet, the benefits, including improved relevancy, enhanced user experience, and increased engagement, affirm the value of investing in LTR technologies. As the digital landscape continues to evolve, LTR stands as a critical tool for those seeking to enhance the precision and personalization of digital platforms.