Google's Bard
Google's Bard, as mysterious as it sounds, is an exciting creation from the tech giant that is designed to help us understand and navigate the complex world of digital marketing better.
In the ever-evolving landscape of digital marketing, one can't help but keep an eye on Google. Their innovative tools constantly reshape the way we approach online visibility and engagement. One such cutting-edge tool that has been creating a buzz is Google's Bard. But what exactly is it? Let's find out.
1. What is Google's Bard?
Google's Bard, as mysterious as it sounds, is an exciting creation from the tech giant that is designed to help us understand and navigate the complex world of digital marketing better. But don't let the Shakespearean name fool you — this isn't about poetic verses or sonnets.
Google's Bard is a machine learning model developed by Google whose primary function is to aid in the understanding and generation of natural language. This means it can read, comprehend, and even generate human-like text.
Now that we've briefly touched on what Google's Bard is, you might be wondering how it works, right? Well, you're in luck, because that's exactly what we'll be diving into next — the workings of Google's Bard. So, are you ready to meet your new digital bard?
2. How Google's Bard Works (and Why It’s Different)
Google's Bard operates on an advanced machine learning framework known as Transformer, which is a model architecture used for understanding language. It takes in data — in this case, text — and processes it to make sense of its structure, meaning, and context.
But here's where it gets really interesting: Google's Bard doesn't just understand language, it generates it too! It takes the text data it's provided, processes it, and then produces its own content. This means it can write blogs, social media posts, even email copy. It's like having your very own digital bard, constantly at your service, producing engaging content on demand.
Here's a simplified breakdown of how it works:
Google's Bard receives text.
It processes this text, understanding its meaning and context.
Finally, it creates new content based on its understanding.
What differentiates Bard from other Generative Text Models like ChatGPT, however, is that its underlying model is LaMDA (read: “Language Model for Dialogue Applications”). LaMDA is unique because it was trained on dialogue specifically. According to Google, during LaMDA’s training, it “picked up on several of the nuances that distinguish open-ended conversation from other forms of language.”
In other words, while models like GPT-3 and LLaMA are trained on vast amounts of text data such as Wikipedia articles and novels, LaMDA is specifically trained and fine-tuned on conversational dialogue. In theory, this should make Bard itself more conversationally oriented than its counterparts.
3. Practical Tips for Using Google's Bard
So, you're ready to start crafting content with Google's Bard? That's great, but remember, even the most advanced tool is only as good as the hands that wield it. Here are some handy tips to help you make the most of Google's Bard.
While Google's Bard is quite the whiz at understanding context, it can't read your mind. If you want it to generate content on a particular topic, make sure to feed it relevant text. Here’s a helpful guide on prompt engineering to get you started.
Lastly, practice makes perfect. Don't expect Google's Bard to deliver Pulitzer-worthy prose on its first go. Start with small tasks and gradually move on to more complex ones. With every piece of content it generates, it learns and improves.
These are just a few practical ways to optimize your use of Google's Bard. However, there's so much more to explore. Up next, we're going to dive deeper into this fascinating tool. So stick around for some in-depth insights into Google's Bard and discover how this digital bard can transform your content strategy.
4. Future Predictions for Google's Bard
Pondering about the future is always fascinating—especially when it comes to technology. Let's take this opportunity to make some educated guesses about the road ahead for Google's Bard.
On the horizon, we may see Google's Bard becoming even more flexible and versatile. Imagine being able to customize the AI to mimic specific author styles or being able to generate content in multiple languages. Wouldn't that be something?
Additionally, Google's Bard might evolve to understand the context even better. This could mean producing more nuanced and contextually rich content, making the tool even more valuable for marketers and writers.
It's also conceivable that Google could integrate the Bard with other Google services. Imagine the ease of generating fresh content for your Google Ads or enhancing your YouTube video descriptions with the help of Google's Bard.
While these are speculations, one thing is certain: the impact of Google's Bard on digital marketing and content creation will continue to grow. The tool's potential is immense, and it will be exciting to watch how it shapes our digital landscape.
Now, let's move on to some practical tips for troubleshooting Google's Bard—because even the best technology can have its hiccups.
5. Resources for Further Learning about Google's Bard
As with any tool, getting the most out of Google's Bard involves a bit of homework. Luckily, there are plenty of resources to help you in your quest for mastery.
A great starting point is Google's own documentation, including the API Documentation. While it might not be the most exciting read you'll ever pick up, it's jam-packed with technical specifications, guidelines, and best practices. Think of it as a user manual for this AI bard.
For a more interactive learning experience, try out the forums and communities that have sprung up around Google's Bard. Here, you'll find real users sharing their tips, tricks, and experiences. You can learn a lot from their triumphs and tribulations.
There are also plenty of online tutorials and courses on AI and machine learning that can give you a deeper understanding of how Google's Bard works under the hood. Knowing what's going on behind the scenes can help you troubleshoot any issues and optimize your use of the tool.
Finally, don't underestimate the power of trial and error. The best way to learn is by doing. So, get out there, get your hands dirty, and let Google's Bard inspire you. After all, every bard needs an audience, and there's no better audience than an informed and engaged one.
For more information about Bard, check out this blog, or this video.
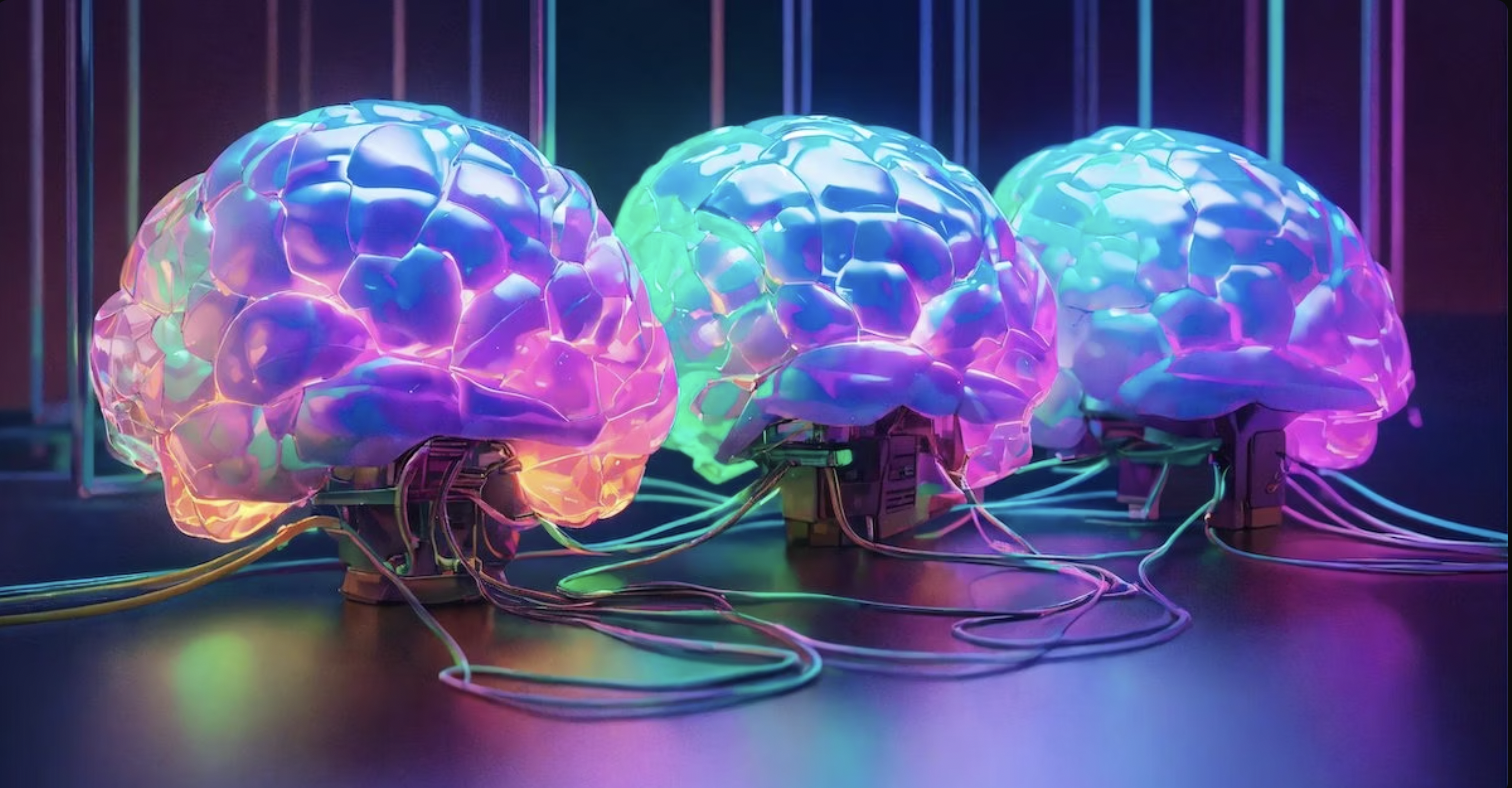
Mixture of Experts (MoE) is a method that presents an efficient approach to dramatically increasing a model’s capabilities without introducing a proportional amount of computational overhead. To learn more, check out this guide!