AI and Education
With AI's potential to personalize learning, improve educational outcomes, and prepare students for a digital future, this article serves as a comprehensive guide to understanding and embracing AI's transformative role in education.
Welcome to the age of digital transformation, where artificial intelligence (AI) is not just reshaping industries but revolutionizing education. Ever wondered how the relentless march of technology could enhance learning for our future generations? AI in education is no longer a distant dream; it’s a rapidly unfolding reality that promises to tackle some of the most persistent challenges in our classrooms. With AI's potential to personalize learning, improve educational outcomes, and prepare students for a digital future, this article serves as a comprehensive guide to understanding and embracing AI's transformative role in education. As we delve into the multifaceted benefits of AI, how ready are you to discover the ways in which this technology can bridge the learning divide and create a more inclusive, efficient, and engaging educational landscape?
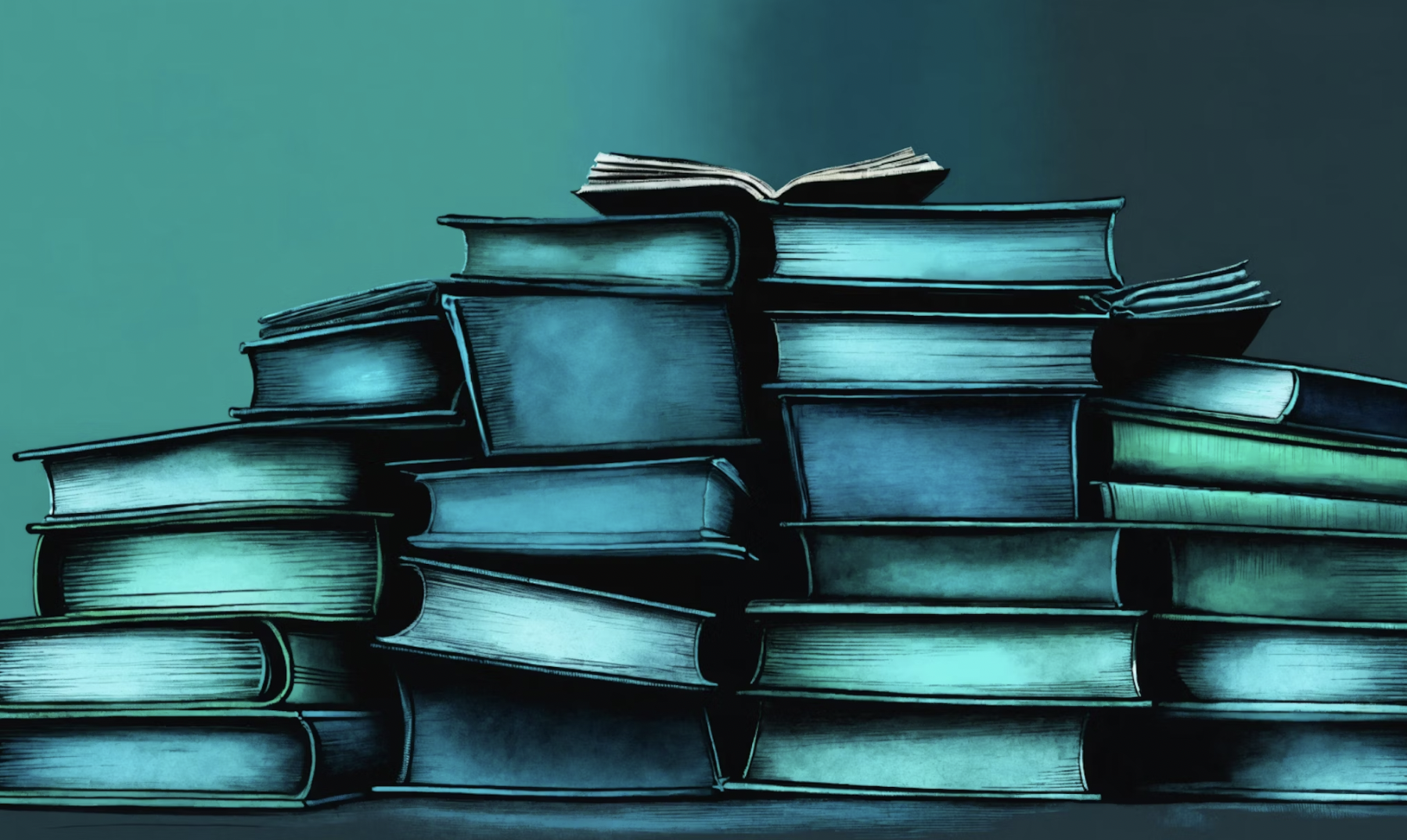
From virtual TAs to accessibility expansion, this article showcases how AI is revolutionizing the world of education.
Section 1: How AI Can Help Schools and Educators
Artificial intelligence stands at the forefront of educational innovation, offering a spectrum of benefits that can transform the way schools operate and educators teach. Let's explore the remarkable ways AI is making an impact:
Streamlining Administrative Tasks: AI can significantly reduce the burden of administrative duties. For example, AI can take over time-consuming tasks like grading and attendance tracking, freeing teachers to concentrate on student interaction and teaching quality.
Scalable Personalized Learning: One of the most exciting aspects of AI in education is its ability to deliver personalized learning experiences at scale. Traditional classroom constraints can impede addressing individual student needs, but AI provides a tailored approach to learning, adapting to the pace and style of each student.
Immediate Feedback on Assignments: The timely nature of automated feedback can drastically improve learning outcomes. AI enables immediate and personalized responses to student work, enhancing the learning process through prompt guidance and support.
Preparing for a Tech-Driven Job Market: AI in education also plays a critical role in equipping students with the skills necessary for the evolving job market. As technology permeates every industry, familiarity with AI and its applications becomes an invaluable asset for students.
Creating Equitable Educational Opportunities: AI has the potential to level the playing field by offering quality resources to students from diverse backgrounds. This technology can make high-quality education accessible to all, irrespective of socioeconomic status.
Reducing Teachers' Workload: The impact of AI on educators is profound. According to Quizlet's State of AI in Education survey, AI can alleviate teachers' workloads, enabling them to focus on enhancing the quality of instruction and student engagement.
Furthering edu-tainment content: YouTubers like VSauce, Numberphile, 3Blue1Brown, and Veritasium all create interesting educational content for the insatiably curious. AI can make it easier not only for such content-creators to find new and exciting ways to connect their audience, but also for viewers to consume that content in an expansive way.
As AI continues to integrate into the educational space, it brings a promise of a more inclusive, efficient, and individualized approach to learning. The journey into the future of education with AI is just beginning.
Section 2: Educational Applications of LLMs (Large Language Models)
Large Language Models (LLMs) like GPT-3 and Aura have ushered in a new era of possibilities in the realm of education. These advanced AI tools are not just enhancing the learning experience; they are revolutionizing it by providing adaptive learning environments, personalizing instruction, and offering innovative ways to engage with content. Here's a closer look at how LLMs are contributing to the educational landscape.
Dynamic Curriculum Content
Adaptive Learning Materials: Keymakr and similar companies are at the forefront of leveraging LLMs to create curriculum content that adapts in real-time to students' learning progress. This ensures that each student receives a learning experience tailored to their understanding and pace.
Responsive Educational Resources: As students interact with the curriculum, LLMs analyze their responses and dynamically adjust the difficulty and type of content delivered, ensuring an optimal challenge level for effective learning.
Language Learning Assistance
Immersive Experiences: LLMs serve as the backbone for interactive language learning platforms, simulating conversations and providing practice that mirrors natural language acquisition.
Contextual Understanding: These models comprehend and respond to a wide array of linguistic inputs, allowing learners to immerse themselves in the language and culture, thereby deepening their understanding.
Simplifying Complex Concepts
Natural Language Explanations: LLMs excel at breaking down complicated ideas into straightforward explanations, particularly in subjects like math and science where conceptual clarity is crucial.
Enhanced Comprehension: By using LLMs, educators can offer students a variety of explanations and analogies, catering to different learning styles and improving overall comprehension.
Summarizing Research
Condensing Information: Platforms like ChatGPT utilize LLMs to summarize extensive research, making it more accessible and digestible for students.
Quick Reference: This capability not only saves time but also allows students to quickly grasp key points and themes from a broad range of sources.
Creative Classroom Activities
Idea Generation: LLMs can propose a plethora of creative ideas for classroom activities, thereby enriching the educational experience and fostering a more engaging learning environment.
Diverse Learning Scenarios: Educators can use these AI-generated suggestions to design lessons that accommodate various learning preferences and keep students motivated.
Research Assistance
Deep Dives into Topics: LLMs empower students to explore subjects in depth, promoting critical thinking and research skills.
Guided Inquiry: With LLMs as research assistants, students can formulate more sophisticated questions and receive detailed responses that enhance their understanding.
Personal Tutor Experience
Immediate Query Responses: The ability of LLMs to provide instant feedback to student inquiries simulates the experience of having a personal tutor, available any time for academic support.
Customized Learning Support: The specificity and relevance of the feedback from LLMs can greatly influence a student's ability to grasp and master new concepts.
As we navigate the complexities of integrating LLMs into educational settings, it's clear that these models hold tremendous potential for individualizing and enhancing the learning journey. While challenges such as ensuring the accuracy of information and the ethical use of AI persist, the advantages they bring to education — from elementary schools to universities — signal a transformative shift in how we teach and learn. The future of education with LLMs promises a more personalized, accessible, and engaging experience for all students, preparing them for a world where AI is an integral part of life and work.
Section 3: Current Educational AI Models
The integration of AI into the educational sphere has led to the development of various AI models, each with unique features designed to enhance learning and teaching. These models are not just tools; they're reshaping the educational landscape, creating new opportunities for personalized learning and data-driven insights.
Generative AI Models
Generative AI models like the Llama or GPT series have become a cornerstone for producing educational content that is both diverse and tailored to individual needs. Their capabilities extend to:
Content Generation: They craft lesson plans, quizzes, and interactive content that react to student input, making learning more engaging.
Assessment Creation: These models can design assessments that align with learning objectives and adapt based on student performance.
Flexibility and Scalability: LLMs’ advanced algorithms allow for the creation of materials that scale across different subjects and grade levels, ensuring a wide applicability.
Student Engagement and Retention
Studies, such as the one by Popenici and Kerr, have examined AI models dedicated to student engagement and retention, particularly in online learning environments. These models are proving to be effective by:
Monitoring Engagement: They track student interaction with course materials to identify engagement levels and adjust content accordingly.
Predictive Analytics: AI identifies patterns that may signal a drop in student retention, enabling proactive measures to re-engage learners.
Tailored Interventions: By understanding a student's unique challenges, AI can suggest personalized strategies to enhance their learning journey.
AI-Driven Analytics Platforms
The power of AI to transform raw data into actionable insights is revolutionizing educational strategies through:
Performance Insights: AI analytics platforms dissect performance data to uncover strengths and learning gaps among students.
Curriculum Design: Educators can use these insights to refine teaching methods and curriculum design, ensuring they meet the evolving needs of their students.
Learning Patterns: Understanding how students learn allows for the development of strategies that cater to various learning styles and paces.
AI in Career Guidance
AI tools are increasingly being utilized to support students in navigating their educational and career paths by:
Personalized Suggestions: AI analyzes student interests, strengths, and market trends to provide personalized career guidance.
Educational Pathways: Students receive recommendations for courses and extracurricular activities that align with their desired career trajectory.
Professional Development: AI can suggest resources and networks to help students gain the skills and connections needed for their future professions.
Ethical Considerations
The deployment of AI in education raises important ethical questions that must be addressed:
Bias Mitigation: Steps are being taken to eliminate biases in AI models to ensure they serve all students equitably.
Transparency: It's crucial to maintain transparency about how AI models operate and the data they use.
Privacy Protection: Safeguarding student data is paramount, with measures in place to prevent misuse.
AI Detection Tools
With the rise of AI assistance, maintaining academic integrity has become a pressing concern, leading to the development of:
Integrity Measures: Tools like those discussed in the Futurism article are emerging to detect AI-generated content, ensuring students maintain honesty in their work.
Continual Development: These tools are in a constant state of evolution, refining their algorithms to stay ahead of advancements in AI.
The landscape of AI in education is one of continual innovation and refinement. As educators and institutions adapt to these technologies, the potential for creating a more effective, inclusive, and personalized learning experience becomes ever more apparent. With the right ethical framework and ongoing development, AI stands to bridge educational divides and foster a future where every student has the tools to succeed.
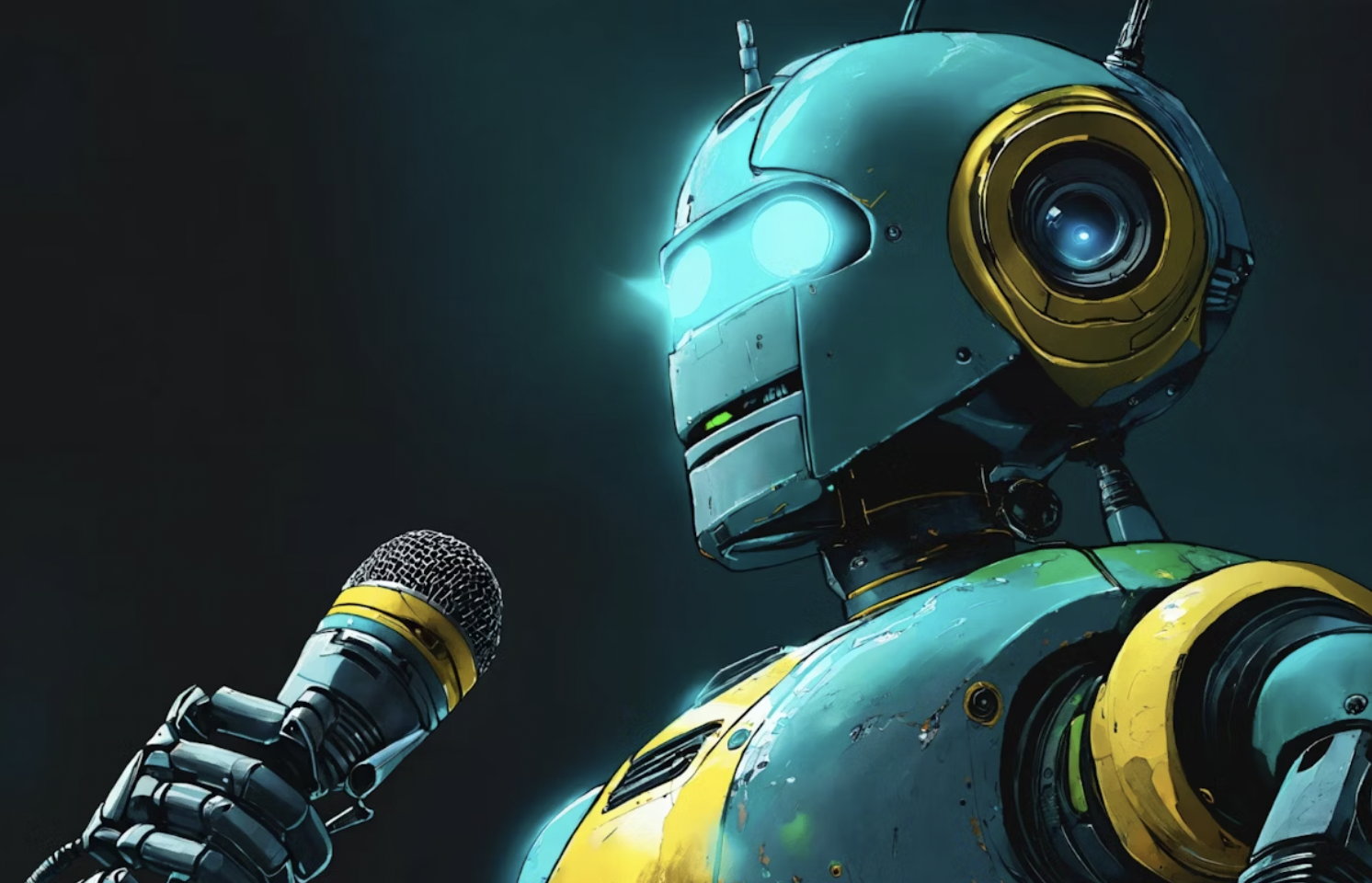
Do you know how to spot a deepfake? Or how to tell when a voice has been cloned? Learn expert detection techniques in this article.
AI and Neurodiversity in Education
The realm of education stands on the brink of a revolutionary shift as AI begins to intersect with neurodiversity. This integration promises to nurture an inclusive learning environment where every student can thrive, regardless of their unique neurological makeup. As we delve into this exciting frontier, it's essential to recognize the transformative potential AI holds for students who learn differently.
Defining Neurodiversity and Inclusive Education
Neurodiversity acknowledges the diverse spectrum of neurological differences among individuals. It's a perspective that views conditions like ADHD, dyslexia, and autism not as deficits but as variations in the human genome. The importance of inclusive education lies in its core principle—valuing every student's learning style and providing equitable access to educational resources. By tailoring educational experiences to accommodate these variations, we step closer to an education system that truly caters to all.
Recognition of Different Learning Styles: Embracing the concept of neurodiversity ensures that educational methods are not one-size-fits-all but are instead as varied as the students themselves.
Educational Equity: Providing equal opportunities to all students, including those who are neurodiverse, is not just a moral imperative but also a legal one in many jurisdictions.
Benefits for All: Inclusive education benefits neurotypical students as well by fostering an environment of diversity and empathy.
AI-Driven Tools for Personalized Learning
The advent of AI-driven tools offers a beacon of hope for neurodiverse students who might struggle with traditional learning methods. These tools are designed to adapt to each student's unique learning pace, style, and challenges, thus offering a truly personalized experience.
Customized Learning Paths: For students with ADHD, AI can provide engaging, interactive sessions that maintain focus and interest.
Literacy Support: Dyslexic learners can benefit from AI systems that tailor reading experiences to their processing style, making text more approachable.
Structured Learning Environments: AI can help create predictable and structured learning environments that are beneficial for students on the autism spectrum.
Alternative Communication Methods
AI's potential extends to providing a voice for non-verbal students or those with speech impairments. The technology can facilitate alternative communication methods, enabling these students to express themselves and participate fully in their educational journey.
Speech Generation: AI can convert text or symbols into natural-sounding speech, allowing non-verbal students to communicate their thoughts and needs.
Sign Language Recognition: Advanced AI can interpret sign language, translating it into text or speech in real-time, thus breaking down barriers for students with hearing impairments.
Adapting to Sensory Processing Needs
AI systems can be tailored to accommodate different sensory processing needs, ensuring that the learning environment is accessible to all students, including those with sensory sensitivities.
Customizable Interfaces: AI can adjust the visual and auditory elements of educational software to suit individual sensory preferences.
Sensory Modulation: For those who are easily overwhelmed by sensory input, AI can modulate the amount and type of stimuli presented during learning activities.
Early Detection and Intervention
One of the most profound impacts of AI in education could be its ability to detect and address learning disabilities early on. By identifying patterns that may indicate a learning disability, AI enables timely interventions that can significantly alter a student's educational trajectory.
Predictive Analytics: AI can analyze student performance data to predict who might be at risk for certain learning disabilities.
Targeted Support: With early detection, educators can provide targeted support and resources to address specific learning challenges.
Ethical and Privacy Considerations
While AI offers promising benefits, it also raises concerns regarding ethics and privacy. It's crucial to use these technologies responsibly, ensuring that they do not compromise the privacy or agency of neurodiverse students.
Data Protection: Strict data privacy measures must protect sensitive information about students' learning needs and styles.
Informed Consent: Transparency and consent are vital when implementing AI tools in the educational setting.
Case Studies of Success
Real-world implementations of AI to support neurodiverse learners have already begun to demonstrate the positive impact of this technology.
Customized Learning Programs: Schools that have adopted AI-driven learning programs report improved engagement and achievement among neurodiverse students.
Best Practices: These case studies serve as blueprints for best practices in implementing AI to support neurodiversity in education.
As AI continues to evolve and integrate into the educational landscape, the promise it holds for neurodiverse learners is immense. By embracing these technologies, educators can create a more inclusive, effective, and nurturing learning environment for all students, ensuring that each one has the opportunity to reach their full potential.
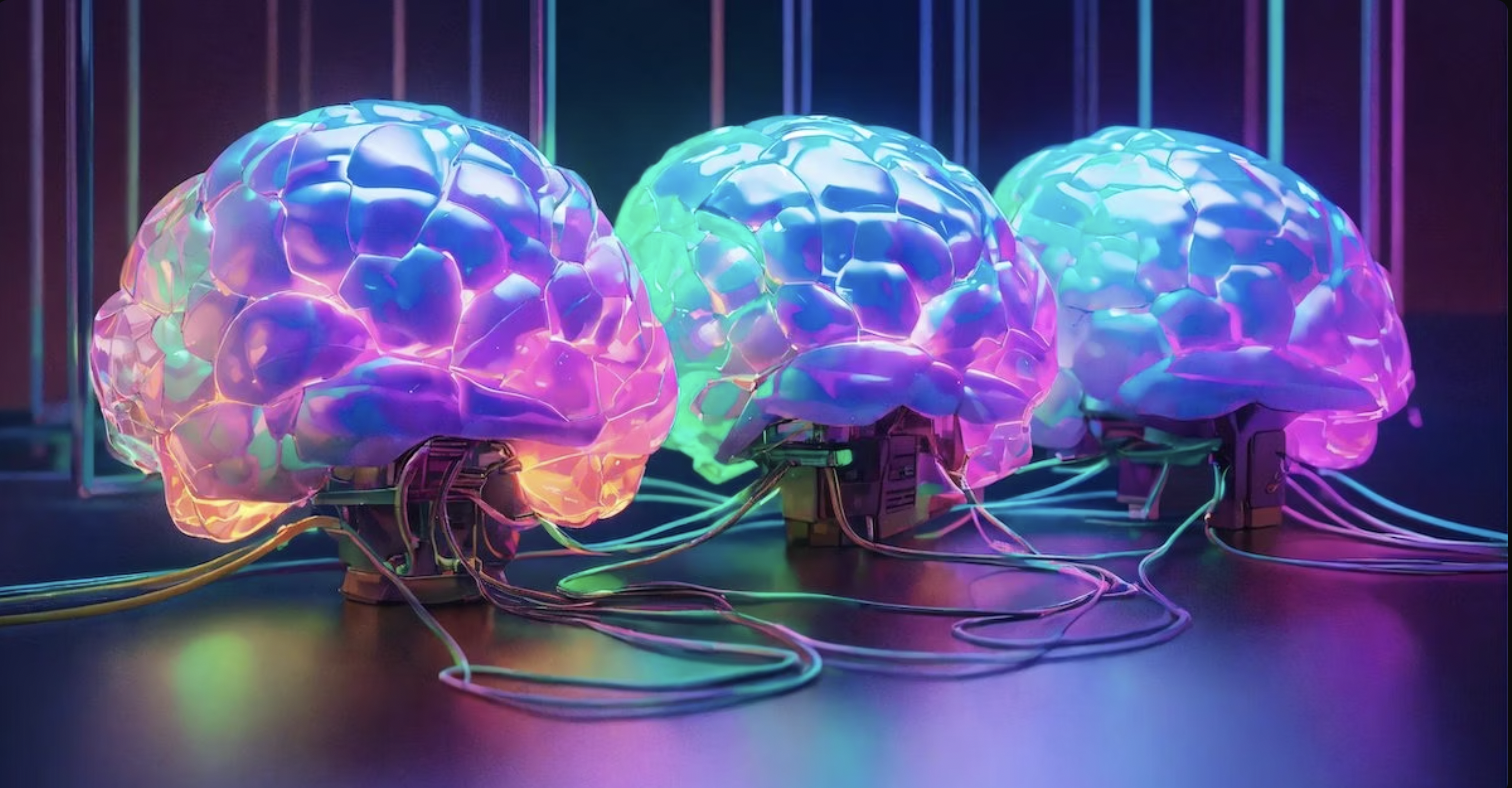
Mixture of Experts (MoE) is a method that presents an efficient approach to dramatically increasing a model’s capabilities without introducing a proportional amount of computational overhead. To learn more, check out this guide!