Eco-friendly AI
In a world where technology's appetite for energy seems insatiable, the quest for sustainable solutions has never been more critical. Did you know that the carbon footprint of training a single AI model can exceed that of five cars over their lifetimes? This startling statistic underscores a pressing challenge: the environmental impact of burgeoning AI technologies. Enter the realm of Eco-friendly AI, a pioneering approach that promises not just innovation but sustainability. This article delves into the essence of Eco-friendly AI, distinguishing it from traditional AI practices by its commitment to minimizing environmental impact without sacrificing performance. From energy-efficient algorithms to the utilization of renewable energy in data centers, we uncover the principles and practices defining this green revolution. Whether you're an industry professional or an eco-conscious enthusiast, understanding Eco-friendly AI's significance and application offers a pathway to contributing to a more sustainable future. Ready to explore how technology and ecology converge in the quest for a greener planet?
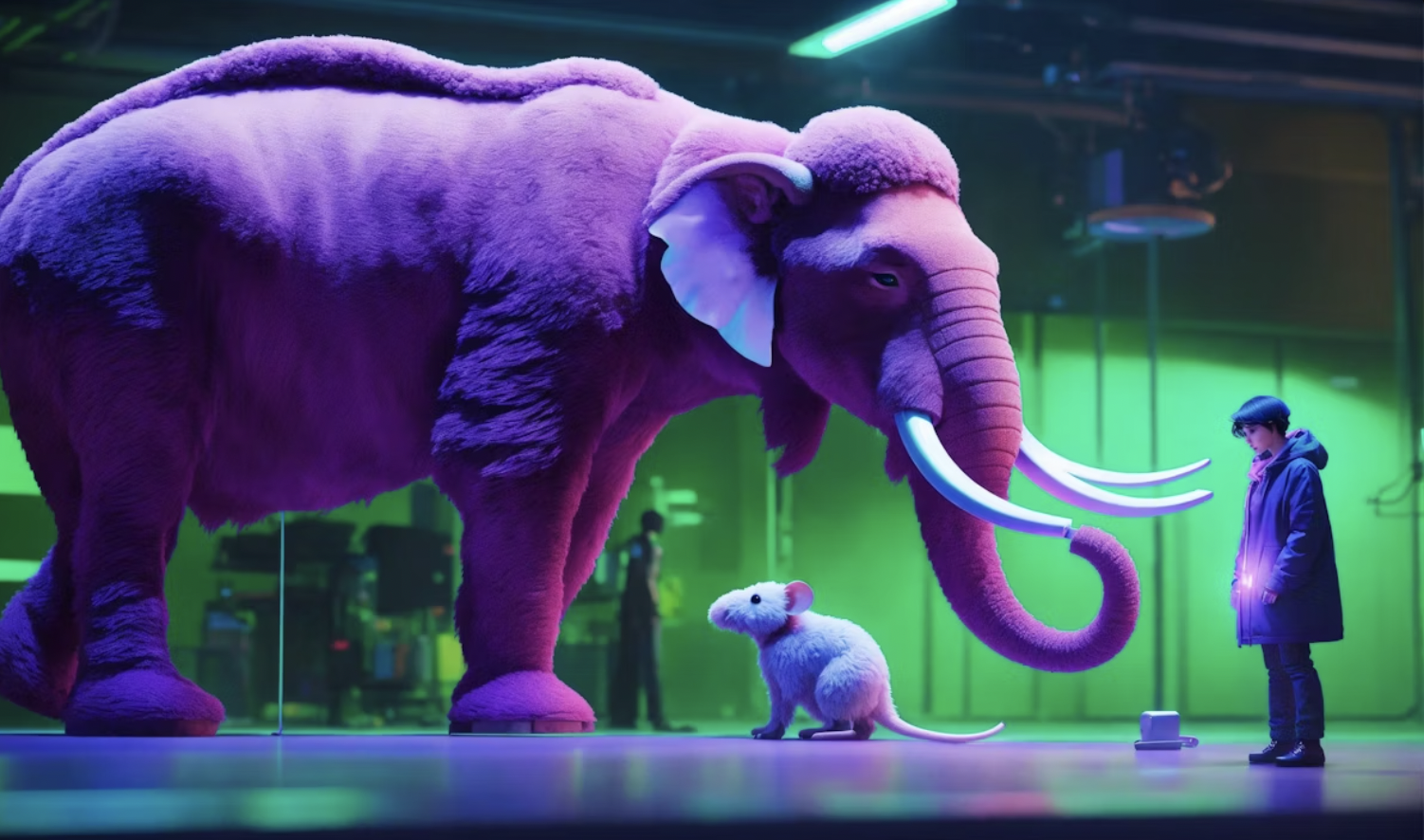
What is Eco-friendly AI
Eco-friendly AI, also known as Green AI, symbolizes a shift towards more sustainable artificial intelligence practices. At its core, this movement prioritizes the development of AI technologies that have a minimal environmental footprint. Here's a closer look at its foundational principles:
Energy-Efficient Algorithms: The heart of Green AI lies in designing algorithms that require less power to operate, thereby reducing the overall energy consumption of AI systems.
Renewable Energy Utilization in Data Centers: A significant aspect of Eco-friendly AI involves powering data centers with renewable energy sources, such as solar or wind, to diminish the carbon emissions associated with AI computations.
Sustainable Hardware Design: This principle focuses on creating hardware that not only meets computational demands but also emphasizes durability and energy efficiency.
An insightful piece from UBC IT outlines tangible solutions for optimizing AI's energy consumption, serving as a practical guide for organizations looking to adopt Green AI practices.
The distinction between Green AI and traditional AI is stark; while the latter often overlooks the environmental costs of advancements, Green AI champions a balance between innovation and sustainability. It stresses the importance of reducing carbon footprints and energy usage without compromising performance or accuracy.
The emergence of Green AI comes as a direct response to the environmental concerns tied to the rapid expansion of AI technologies. Notably, the systematic review by Verdecchia (2023) and contributions from leading organizations and researchers, such as Roy Schwartz and the AI2 Blog, highlight ongoing research and initiatives in this field.
Moreover, the role of Green IT in conjunction with Green AI underscores a comprehensive approach to reducing technology's environmental impacts. Both movements are integral to fostering a sustainable future, where technological progress does not come at the expense of our planet's health.
How Eco-friendly AI Works
The journey towards Eco-friendly AI is a multifaceted endeavor, involving various strategies and technologies aimed at reducing the environmental impact of artificial intelligence. By dissecting the mechanisms that make AI green, we uncover a blueprint for a sustainable digital future.
Optimization of AI Training Processes
The training phase of AI models is notoriously energy-intensive. However, advancements in algorithm efficiency have paved the way for more sustainable practices:
Energy-Efficient Training: Methods such as pruning and knowledge distillation enable AI models to learn from less data or transfer knowledge from larger models without the need for extensive computing resources.
Dynamic Scaling: Adjusting the computing power in real-time, based on the workload, prevents unnecessary energy consumption.
Reference: The Towards Data Science article highlights innovative methods to reduce AI's carbon footprint through smarter training techniques, showcasing the industry's commitment to sustainability.
Renewable Energy Sources and Hardware Efficiency
The backbone of eco-friendly AI lies in its reliance on renewable energy sources and the development of energy-efficient hardware:
Renewable-Powered Data Centers: Solar and wind energy are increasingly powering data centers, significantly cutting down the carbon emissions associated with AI operations.
Energy-Efficient Hardware Design: The creation of processors and hardware specifically designed for AI tasks can drastically reduce energy consumption. Techniques like ASIC (Application-Specific Integrated Circuit) and FPGA (Field-Programmable Gate Array) chips offer tailored computing that's both powerful and efficient.
Software Optimization and Model Quantization
Software plays a crucial role in the green AI ecosystem, with optimization and model quantization leading the charge:
Software Optimization: Techniques such as algorithmic improvements and the use of efficient coding practices ensure that AI software requires fewer computational resources.
Model Quantization: This process reduces the precision of the numbers used in computations, allowing AI models to run on less energy without a significant loss in accuracy or performance.
Efficient Data Management and Cloud Technologies
At the heart of eco-friendly AI lies the efficient management of data and the strategic use of cloud computing:
Efficient Data Storage: Optimizing data storage not only reduces the space needed but also the energy required for data retrieval and processing.
Cloud Computing and Virtualization: These technologies allow for the dynamic allocation of resources, ensuring that energy is consumed judiciously, only as needed, and often powered by renewable energy sources.
Eco-friendly AI Applications
Real-world applications of eco-friendly AI are already making a significant impact:
Permutable's Support for Renewables: The AI think tank Permutable has produced a report highlighting how AI is being used to promote renewable energy sources, showcasing the potential of AI to drive the adoption of clean energy solutions.
By exploring these mechanisms, it becomes evident that eco-friendly AI is not just a concept but a practical pathway to reducing the carbon footprint of one of the 21st century's most transformative technologies. The commitment to green principles in AI development and application opens up new vistas for sustainable innovation, proving that it is possible to harness the power of AI in a manner that respects and preserves our environment.
Applications of Eco-friendly AI
Eco-friendly AI, or Green AI, is revolutionizing industries by providing sustainable solutions that reduce environmental impacts while enhancing efficiency and productivity. Below, we delve into how this innovative technology is making strides across various sectors.
Environmental Monitoring and Conservation
Deforestation and Ocean Changes: Eco-friendly AI plays a crucial role in monitoring deforestation rates and oceanic changes, offering data-driven insights to inform climate action decisions. The technology employs satellite imagery and machine learning algorithms to detect changes in land use and marine environments, enabling timely interventions.
Ivy Partners article: Highlights how AI technologies contribute to understanding and combatting environmental challenges, showcasing projects where AI has mapped deforestation and tracked oceanic temperature changes to support conservation efforts.
Smart Grids and Renewable Energy Systems
Optimizing Energy Consumption: AI optimizes the distribution and consumption of energy in smart grids by predicting demand and adjusting supply accordingly. This reduces waste and ensures renewable energy sources are used efficiently.
Renewable Energy Integration: AI algorithms analyze weather patterns to predict solar and wind energy production, facilitating the integration of these unpredictable energy sources into the grid, thus enhancing the reliability of renewable energy.
Sustainable Agriculture
Precision Farming: By leveraging AI, farmers implement precision farming techniques that optimize resource use—water, fertilizers, and pesticides—thereby minimizing environmental impact while maximizing crop yields.
Crop Monitoring and Soil Health: AI-driven drones and sensors monitor crop health and soil conditions, enabling targeted interventions that conserve resources and improve farm productivity.
Waste Management and Recycling
Efficient Waste Sorting: AI-powered robots automate the sorting of recyclable materials from waste, improving recycling rates and reducing the amount of waste sent to landfills.
Predictive Waste Collection: AI systems predict when waste bins will be full, optimizing collection routes and schedules to reduce fuel consumption and emissions associated with waste collection vehicles.
Sustainable Urban Planning and Smart City Initiatives
Traffic Flow Optimization: AI algorithms analyze traffic patterns to optimize light signals and reduce congestion, leading to lower vehicle emissions and improved air quality.
Energy-Efficient Buildings: AI is used to monitor and control heating, ventilation, and air conditioning (HVAC) systems in buildings, significantly reducing energy consumption and carbon footprint.
Enhancing the Sustainability of Supply Chains and Logistics
Route Optimization: AI optimizes delivery routes, reducing fuel consumption and emissions in logistics operations.
Supply Chain Transparency: AI tools track and verify the sustainability of products throughout the supply chain, promoting ethical and environmentally friendly practices.
Case Studies and Examples
AI in Conservation: Projects highlighted in the Ivy Partners article, where AI has been employed to track deforestation and ocean changes, underscore the potential of eco-friendly AI in preserving natural habitats and biodiversity.
Smart Agriculture Practices: Precision farming techniques powered by AI are revolutionizing agriculture, enabling farmers to reduce resource use and environmental footprint.
Waste Management Innovations: AI-driven recycling initiatives have demonstrated significant improvements in recycling rates, showcasing the potential for technology to support a more sustainable waste management ecosystem.
Eco-friendly AI is not just a buzzword but a tangible solution driving positive environmental changes across industries. From conserving natural resources to optimizing energy use and enhancing sustainability in supply chains, the applications of eco-friendly AI are vast and varied. As technology continues to evolve, so too will its capabilities to address the pressing environmental challenges of our time, paving the way for a more sustainable future.
Implementing Eco-friendly AI: Practical Insights for Organizations
The transition towards eco-friendly AI presents a transformative opportunity for organizations committed to sustainability. Implementing this technology, however, requires strategic planning, overcoming challenges, and fostering collaborations. Here, we explore the practical aspects of integrating eco-friendly AI into business operations, underscoring the initial steps, challenges, and strategies for successful adoption.
Setting Clear Sustainability Goals
Initial Assessment: Conduct an environmental impact assessment of current operations to identify areas where AI can reduce carbon footprint and improve efficiency.
Goal Setting: Define clear, measurable sustainability goals that align with the organization's overall environmental strategy, leveraging eco-friendly AI as a key enabler.
Stakeholder Engagement: Involve stakeholders across the organization in goal setting to ensure buy-in and align eco-friendly AI initiatives with broader business objectives.
Overcoming Transition Challenges
Technological Barriers: Invest in training for staff to bridge the knowledge gap in eco-friendly AI technologies.
Financial Hurdles: Seek out government grants, subsidies, or partnerships with eco-friendly AI tech providers to offset initial costs.
Cultural Shifts: Foster a culture of innovation and sustainability, emphasizing the long-term benefits of eco-friendly AI over short-term costs.
The Role of Interdisciplinary Collaboration
Cross-functional Teams: Create interdisciplinary teams combining AI experts, sustainability officers, and operational staff to develop and implement eco-friendly AI solutions.
External Partnerships: Collaborate with academic institutions, industry consortia, and technology providers to stay at the forefront of eco-friendly AI developments.
Community Engagement: Engage with local communities and stakeholders to understand their concerns and expectations regarding eco-friendly AI initiatives.
Measuring and Evaluating Impact
Carbon Metrics in MLOps: Incorporate carbon footprint metrics into AI model development and deployment workflows to continuously monitor and optimize energy consumption.
Performance Benchmarks: Set benchmarks for eco-friendly AI initiatives against industry standards and best practices to evaluate performance and impact.
Continuous Improvement: Use the insights gained from monitoring and evaluation to refine strategies and technologies for better environmental outcomes.
Transparency and ethical considerations
Disclosure Practices: Maintain transparency about the environmental impact of AI systems, including energy consumption and carbon emissions.
Ethical AI Use: Ensure that eco-friendly AI applications respect privacy, security, and ethical standards, avoiding harm to individuals and communities.
Stakeholder Communication: Keep stakeholders informed about eco-friendly AI initiatives, progress, and impacts, fostering trust and support.
Funding and Support Mechanisms
Government Incentives: Explore government incentives, tax breaks, and funding opportunities designed to support green technologies and sustainable business practices.
Venture Capital and Grants: Pursue venture capital funding and grants specifically earmarked for sustainable innovation and eco-friendly AI projects.
Public-Private Partnerships: Engage in public-private partnerships to leverage shared resources, expertise, and funding for large-scale eco-friendly AI initiatives.
Call to Action for Industries and Policymakers
To truly capitalize on the potential of eco-friendly AI, industries and policymakers must prioritize the development and deployment of these technologies in their digital and environmental strategies. By setting ambitious sustainability goals, overcoming the inherent challenges of technological adoption, and fostering an ecosystem of collaboration and innovation, organizations can lead the way towards a more sustainable and efficient future powered by eco-friendly AI. This collective effort will not only drive environmental benefits but also unlock new avenues for economic growth and competitive advantage in the green economy.
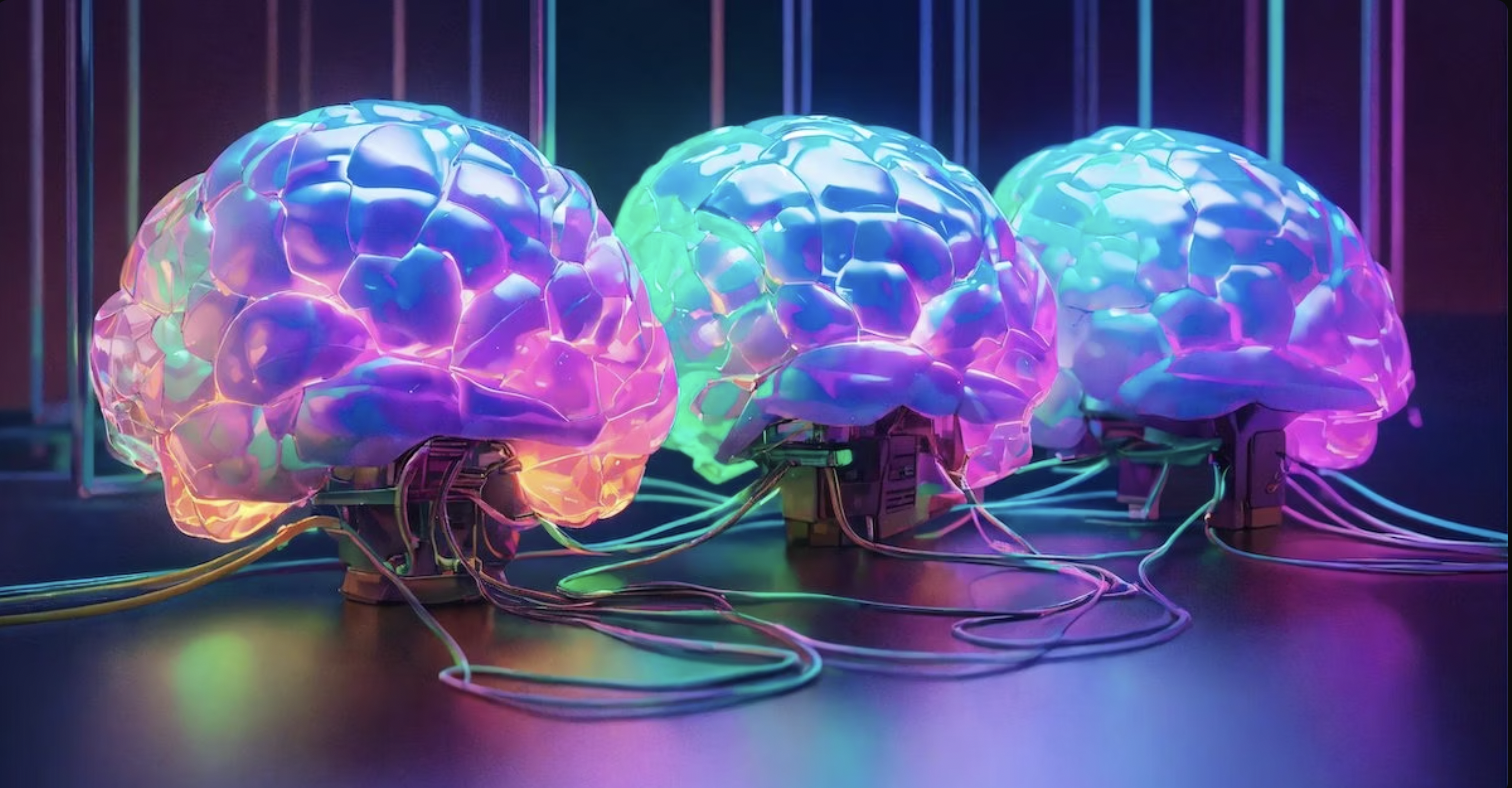