Pandas
This article covers the origins and functionalities of the Pandas library, its core data structures, and how it simplifies data manipulation and analysis tasks.
Have you ever found yourself drowning in data, struggling to extract meaningful insights or simply organize it in a comprehensible manner? You're not alone. In today's digital age, data is akin to a double-edged sword—vastly available yet overwhelmingly complex to navigate. It's a common challenge faced by many, from data scientists to business analysts. Enter the Pandas Python Library, a beacon of hope in the tumultuous sea of data. This article serves as your compass, guiding you through the ins and outs of this powerful tool. Expect to uncover the origins and functionalities of the Pandas library, learn about its core data structures, and discover how it simplifies data manipulation and analysis tasks. With this knowledge, you'll be well-equipped to tackle any data challenge that comes your way. Ready to transform your data handling capabilities?
What is `Pandas`?
The Pandas Python Library stands as a cornerstone in the realm of data manipulation and analysis, providing a robust framework for dealing with structured data. Created by Wes McKinney in 2008, its inception was motivated by the need for a high-level tool to clean, aggregate, analyze, and visualize datasets efficiently. But what's in a name? Pandas, an acronym for Panel Data and Python Data Analysis, aptly reflects its prowess in handling multi-dimensional data and its roots in Python data analysis.
At the heart of Pandas is its reliance on Numpy, another pivotal Python package. Numpy lays the foundation for Pandas' ability to support multi-dimensional arrays, which in turn underpins the library's versatile data structures: Series and DataFrames. A quick look at these structures reveals:
Series: A one-dimensional, array-like object that can hold diverse data types, acting as a column in a spreadsheet.
DataFrame: A two-dimensional, table-like structure capable of holding multiple data types across columns, akin to an entire spreadsheet.
Pandas' adaptability shines in its handling of various data formats. Whether it's CSV files, Excel spreadsheets, or SQL databases, Pandas navigates through them with ease, showcasing its utility in real-world data analysis scenarios. Moreover, its extensive functionality for reshaping, merging, and filtering datasets streamlines the preparation process for in-depth analysis.
Behind Pandas' success lies a robust community and documentation support system. This open-source library thrives on continuous updates and improvements, thanks to the collective efforts of data scientists and developers worldwide. With extensive documentation catering to users from beginner to advanced levels, Pandas ensures that anyone embarking on a data analysis journey has the necessary resources at their fingertips.
How is Pandas used in Machine Learning?
Data Cleaning
The Pandas library shines in data cleaning, offering tools to handle missing data through methods like fillna, dropna, allowing for either filling in blank spaces with predetermined values or eliminating them altogether.
Removing duplicates becomes a straightforward task with the drop_duplicates method, ensuring data integrity and reliability.
Converting data types is essential in preparing data for analysis. Pandas provides methods like astype to convert column data types, facilitating a seamless transition to the analysis phase.
Data Exploration
With Pandas, data exploration becomes an intuitive process. Functions for sorting data (sort_values), summarizing datasets (describe), and grouping data (groupby) empower analysts to discern patterns and characteristics within the data.
This suite of functionalities allows for a thorough understanding of the dataset's structure and underlying trends, setting the stage for deeper analytical work.
Data Analysis
Pandas is equipped with built-in functions for statistical analysis, eliminating the need for external libraries for basic descriptive statistics and correlation analysis.
These functionalities facilitate not just the exploration of data but also enable complex computations and analyses, streamlining the process from data cleaning to insightful analytics.
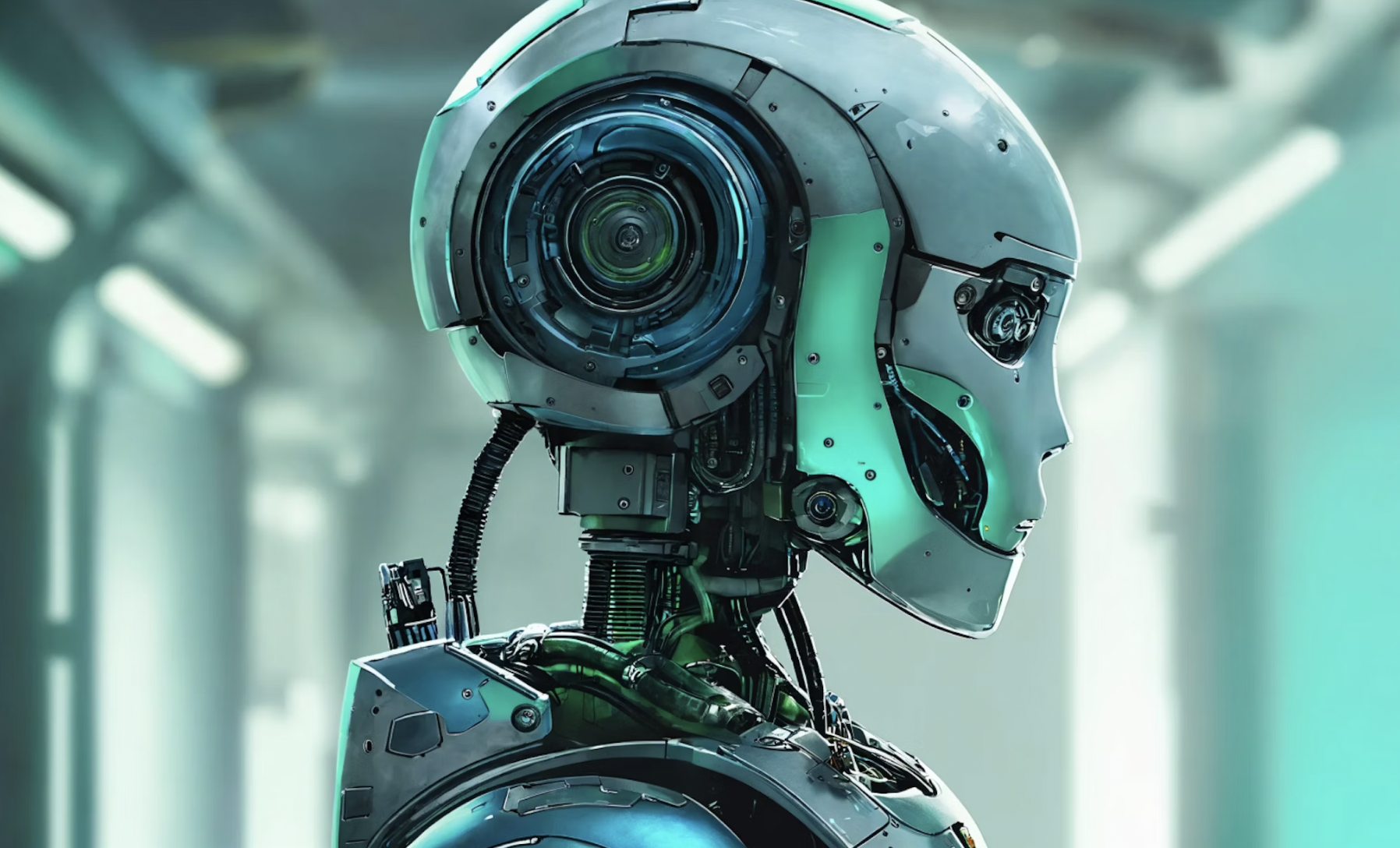
Integration with Visualization Libraries
The integration of Pandas with Matplotlib and Seaborn for data visualization opens up avenues for creating insightful charts and graphs directly from DataFrames.
This capability enhances data presentations, allowing for the visualization of complex data relationships and trends in a digestible format.
Time-Series Data Analysis
Specializing in time-series data analysis, Pandas handles date and time data types proficiently, supporting operations like date range generation and frequency conversion.
Its functionality extends to performing complex window functions for calculating moving averages, crucial for time-series forecasting and analysis.
Real-World Applications
In real-world scenarios such as financial modeling, scientific computing, and engineering, Pandas proves invaluable. Its ability to handle and analyze large datasets is paramount in these fields, where data-driven decisions are critical.
The versatility and robustness of Pandas facilitate a wide range of data manipulation and analysis tasks, underscoring its importance in real-world data applications.
Typical Workflow in Python using Pandas
Data Loading: Importing data from various formats into Pandas DataFrames.
Data Cleaning: Utilizing Pandas' tools to clean and prepare data for analysis.
Exploratory Data Analysis (EDA): Analyzing the data to identify patterns, relationships, and insights.
Visualization: Creating visual representations of the analysis to communicate findings effectively.
Statistical Analysis: Applying statistical methods to interpret data and draw conclusions.
This workflow exemplifies how Pandas serves as the backbone of the data science toolkit, centralizing the data manipulation and analysis process within Python. Its comprehensive suite of functionalities ensures that from the moment data is loaded to the final stages of analysis and visualization, Pandas remains an indispensable tool for data scientists and analysts.
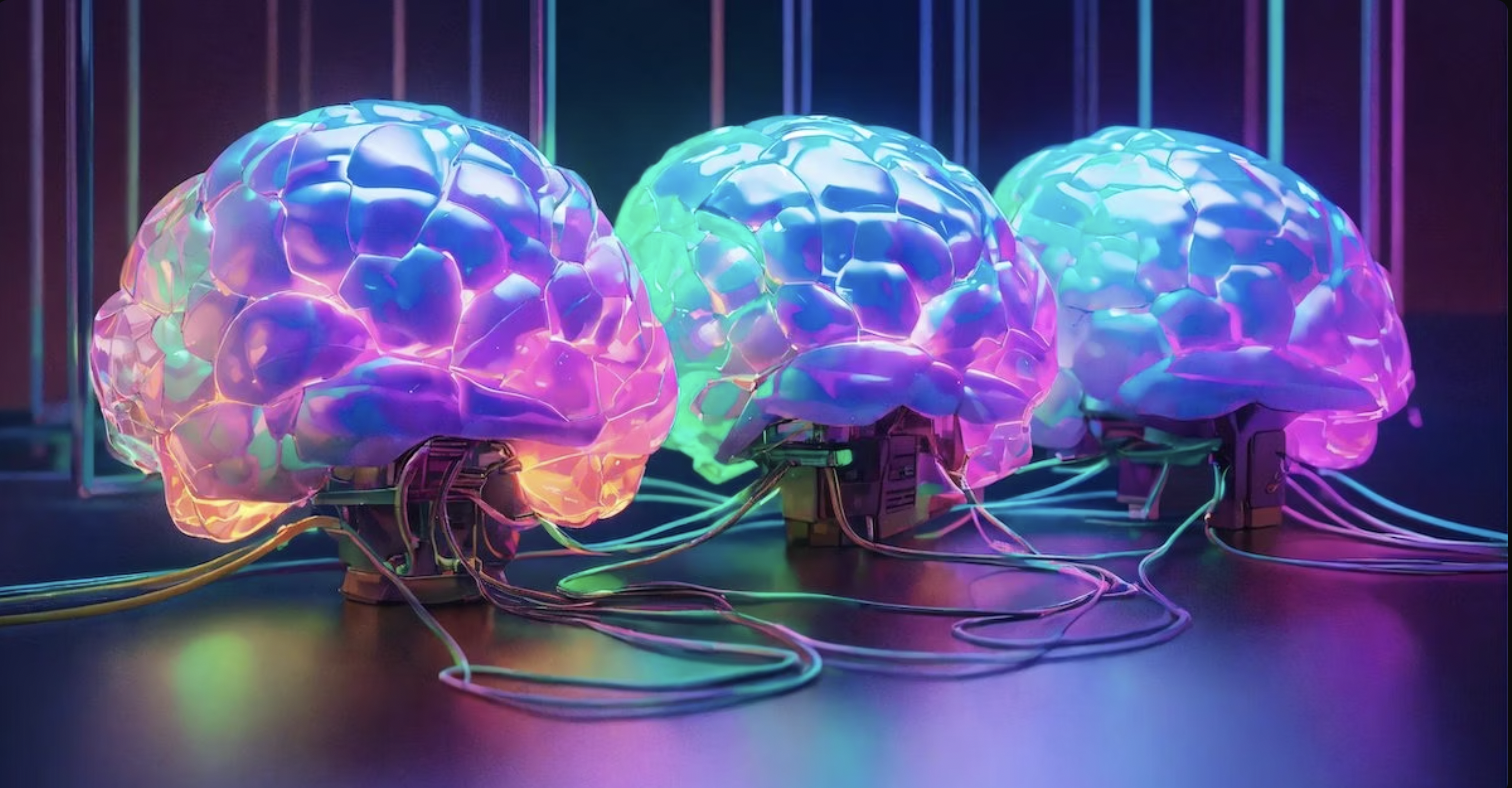