Grounding
Whether you're a tech enthusiast, a professional in the field, or simply curious about the future of AI, understanding the fundamentals of grounding in AI offers a glimpse into the advancements shaping our technological landscape.
Whether you're a tech enthusiast, a professional in the field, or simply curious about the future of AI, understanding the fundamentals of grounding in AI offers a glimpse into the advancements shaping our technological landscape.
What is Grounding in AI?
Grounding in artificial intelligence (AI) represents a crucial bridge between the abstract computations of AI systems and the real world's rich tapestry. This connection ensures AI applications produce outcomes that are not only relevant but also accurate, reflecting the real-world knowledge and context. Here's a closer look at the multifaceted nature of grounding in AI:
Definition and Significance: At its core, grounding in AI involves linking AI models' internal representations with the real-world context. This connection is vital for AI applications to generate outputs that are contextually relevant and accurate, as highlighted in discussions from the Moveworks blog on improving AI context relevance.
Cognitive Science Perspective: Grounding gains a deeper dimension when viewed through the lens of cognitive science, emphasizing its role in facilitating successful communication between AI and humans. The cognitive science definition of grounding in natural language processing (NLP) sheds light on the mutual information required for this interaction.
Large Language Models (LLMs): The importance of grounding extends to large language models (LLMs), where it significantly enhances the quality, accuracy, and relevance of their generated outputs. The Microsoft Community Hub offers insights into grounding LLMs, showcasing its impact.
Preventing AI Hallucinations: A critical role of grounding is in preventing AI hallucinations, ensuring responses are data-driven and contextually relevant. Best practices to prevent these hallucinations have been discussed extensively, including on platforms like Copy.ai.
Dynamic Nature: Grounding in AI is not static; it continuously evolves to adapt to new and specific use cases over time. This dynamic nature allows AI systems to remain relevant and effective across various applications.
Versatile Importance Across AI Domains: The application of grounding spans across different AI domains, including generative AI, deep learning, and NLP. Its versatile importance is underscored in articles discussing its application in these areas.
Symbol Grounding Problem: A philosophical and cognitive challenge, the symbol grounding problem, highlights the complexity of associating abstract symbols with real-world entities. This issue remains a significant hurdle in the field of AI.
Through these lenses, grounding in AI emerges as a multifaceted concept that is pivotal for bridging the gap between AI's computational abilities and the real-world context. Its applications across various domains underscore its critical role in enhancing the interaction between humans and AI systems, paving the way for more accurate, reliable, and context-aware AI technologies.
Importance of Grounding
The importance of grounding in artificial intelligence transcends mere technical necessity; it forms the bedrock upon which the future of AI's interaction with humanity rests. By anchoring AI systems in the realities of our world, grounding ensures that these technologies evolve from mere computational marvels to entities capable of understanding and navigating the complexities of human contexts. This section delves into the multifaceted benefits and challenges of grounding in AI, offering a comprehensive overview of its pivotal role in the development, accuracy, and ethical deployment of AI systems.
Enhancing AI's Understanding of Real-World Context
Critical for Effective Operation: Grounding significantly boosts AI's ability to interpret and interact with real-world situations accurately. This is especially crucial in applications where context changes dynamically, requiring AI to adapt swiftly.
Accuracy and Relevance: By linking AI's internal representations to real-world contexts, grounding ensures the responses are not just accurate but also relevant to the user's current situation or query.
Improving Reliability of AI Systems
Minimizing Errors: Grounding plays a vital role in reducing errors and inaccuracies in AI-generated content (see overfitting and underfitting). Moveworks highlights how grounding AI ensures that outputs are closely linked to the real-world context, thereby maximizing relevance and minimizing errors.
Enhancement of Trust: As errors decrease, trust in AI systems naturally increases, encouraging wider adoption and reliance on these technologies for critical tasks.
Impact on User Experience
Contextually Appropriate Results: A grounded AI system can provide results that are not just accurate but also meaningful and appropriate to the user's specific situation, as discussed in a LinkedIn article on machine learning solutions.
Personalization: Grounding allows AI to offer personalized experiences, understanding and adapting to individual user preferences and needs over time.
Ethical Implications and Prevention of Hallucinations
Bias and Incorrect Responses: Grounding helps in mitigating biases and preventing the generation of incorrect or hallucinated responses, thereby ensuring fair and unbiased AI operations.
Promoting Ethical AI Use: By ensuring AI systems are well-grounded, developers and users can prevent misuse and promote ethical applications of AI technologies.
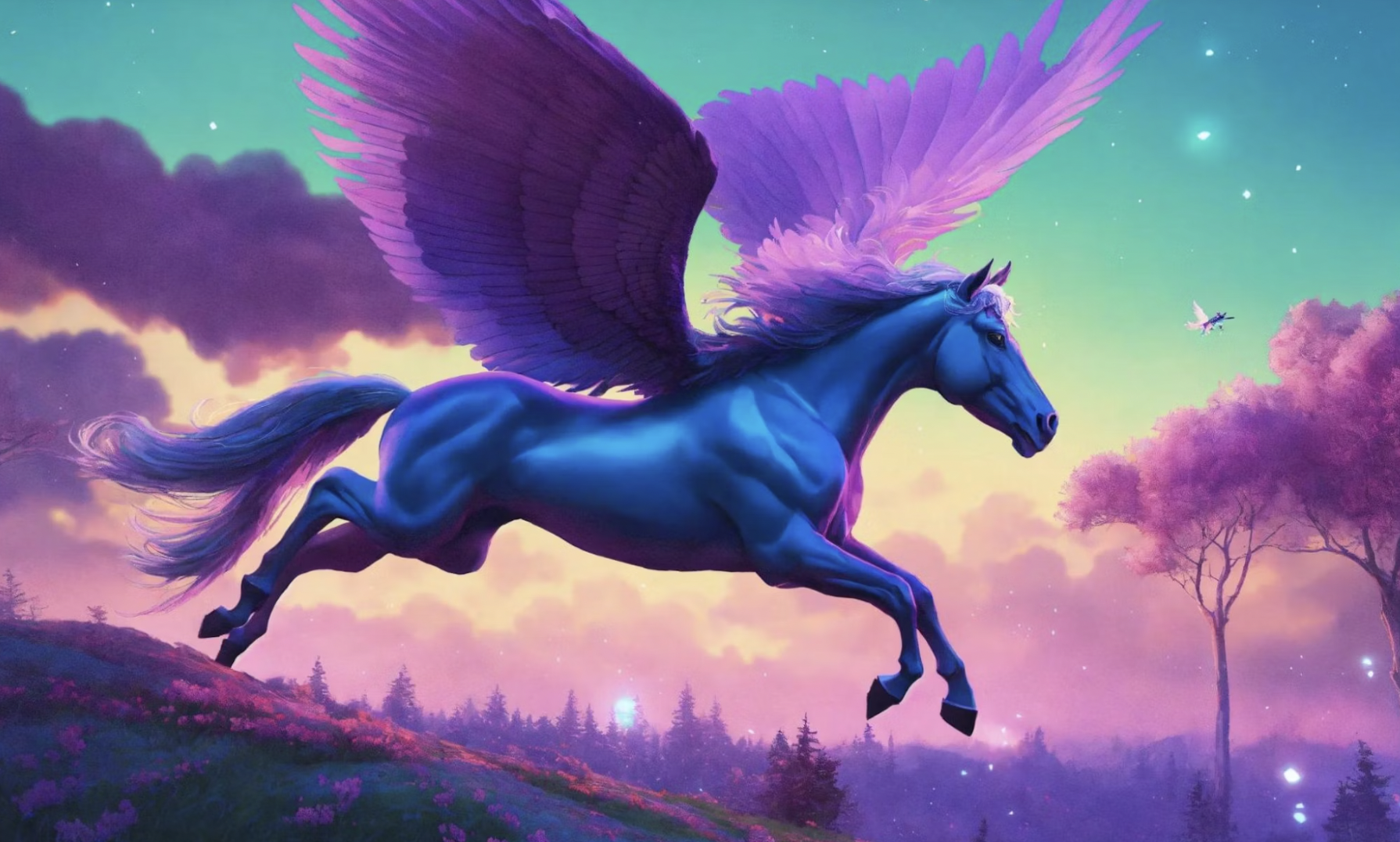
Facilitating Successful Communication
Mutual Understanding: Grounding ensures that AI systems and users share a mutual understanding, crucial for effective communication. The definition of grounding in NLP by Cognitive Science stresses the importance of mutual information for successful interactions.
Language and Semantics: Proper grounding enhances AI's grasp of language nuances and semantics, enabling more natural and effective communication.
Addressing the Symbol Grounding Problem
Complexity of Abstract Symbols: The symbol grounding problem illustrates the inherent challenge in linking abstract symbols and concepts with real-world entities and contexts.
Ongoing Research and Development: Despite its challenges, continuous research and development efforts aim to overcome the symbol grounding problem, paving the way for more sophisticated and capable AI systems.
Case Studies and Examples of Effective Grounding
Tangible Benefits: Various AI advancements, attributed to improved grounding techniques, showcase the tangible benefits of properly grounding AI systems. These include enhanced decision-making capabilities, more accurate predictive analytics, and improved user engagement.
Real-World Applications: From healthcare diagnostics to autonomous vehicle navigation, effective grounding has been instrumental in advancing AI applications, demonstrating its critical role in the evolution of AI technology.
As AI continues to integrate into every facet of our lives, the importance of grounding these systems in the real-world context cannot be overstated. Not only does grounding enhance the accuracy, reliability, and ethical considerations of AI, but it also ensures that AI systems can effectively communicate and interact with humans, understanding the nuances of our world. The journey towards fully grounded AI is fraught with challenges, including the symbol grounding problem, yet it remains a crucial endeavor for the future of AI technology.
How to Ground an AI Model
Grounding AI models effectively connects their computational prowess with the tangible realities of our world, ensuring their outputs are not just accurate but also contextually relevant. This process is pivotal across various AI applications, from chatbots to predictive analytics. Here, we explore the multifaceted approaches to grounding AI models, highlighting practical strategies and emerging trends that promise to enhance their real-world applicability.
Using Large Language Models (LLMs) with Use-Case Specific Information
Selection of Relevant Data Sources: The cornerstone of grounding LLMs involves curating and utilizing data sources that are directly relevant to the specific use case at hand. For instance, we emphasize the importance of integrating use-case specific information to ensure the quality, accuracy, and relevance of LLM outputs.
Incorporation of Real-World Context: Integrating real-world knowledge and context into LLMs allows for a more nuanced understanding and generation of responses. This may involve leveraging databases, the internet, and user interactions to feed the model with the most current and relevant information.
Enhancing Grounding through Databases, the Internet, and User Interactions
Real-Time Data Integration: ChatGPT's integration with the Bing search engine exemplifies how AI models can incorporate real-time data from the internet to stay updated and grounded in the current context.
User Interaction as a Data Source: Interactions with users provide invaluable context that can further ground AI responses, making them more personalized and relevant.
Continuous Learning and Updating
Staying Relevant Over Time: The landscape of information and context is ever-changing. Continuous learning and updating mechanisms, as seen in ChatGPT's use of Bing for current information, are crucial in ensuring AI models remain relevant and grounded in the latest data.
Adaptive Learning: Implementing systems that can learn from new data and user feedback continuously allows AI models to adapt to new contexts and use cases over time.
Grounding Techniques Across AI Applications
NLP and Generative Models: Grounding techniques find their application across a spectrum of AI domains, including natural language processing and generative models. Each domain requires a unique approach to grounding, tailored to the specific challenges and needs of the application.
Diverse Applications: From generating realistic and contextually relevant text in chatbots to producing accurate predictive models in analytics, grounding techniques enhance the utility and effectiveness of AI across various fields.
Ethical Considerations in Grounding AI
Ensuring Diversity and Avoiding Biases: Ethical grounding practices involve careful consideration of the diversity of data sources and active measures to avoid biases. This ensures that AI models do not perpetuate existing stereotypes or inequalities but rather contribute to fair and unbiased outcomes.
Transparency and Accountability: Ethical grounding also necessitates transparency in the data sources used and the mechanisms by which AI models are grounded, ensuring accountability for the outputs generated.
Implementing Grounding Techniques: A Step-by-Step Guide
Data Selection: Begin by identifying and selecting relevant, diverse, and unbiased data sources that reflect the real-world context of the AI application.
Model Training: Train the AI model using the selected data, ensuring it learns to understand and interpret the context accurately.
Continuous Updates: Implement mechanisms for the continuous updating of the model with new data and user feedback to keep it relevant over time.
Evaluation and Refinement: Regularly evaluate the model's outputs for accuracy and relevance, refining the grounding process as needed.
The Future of Grounding in AI
Dynamic Grounding: Emerging trends in AI point towards more dynamic grounding processes, where models can adapt in real-time to new data and contexts.
Real-Time Information Integration: Innovations in integrating real-time information from diverse sources promise to enhance the grounding process, making AI models even more responsive and context-aware.
By meticulously implementing these grounding techniques, AI models can achieve a deeper understanding and interaction with the real-world, paving the way for more accurate, relevant, and ethically grounded AI applications.
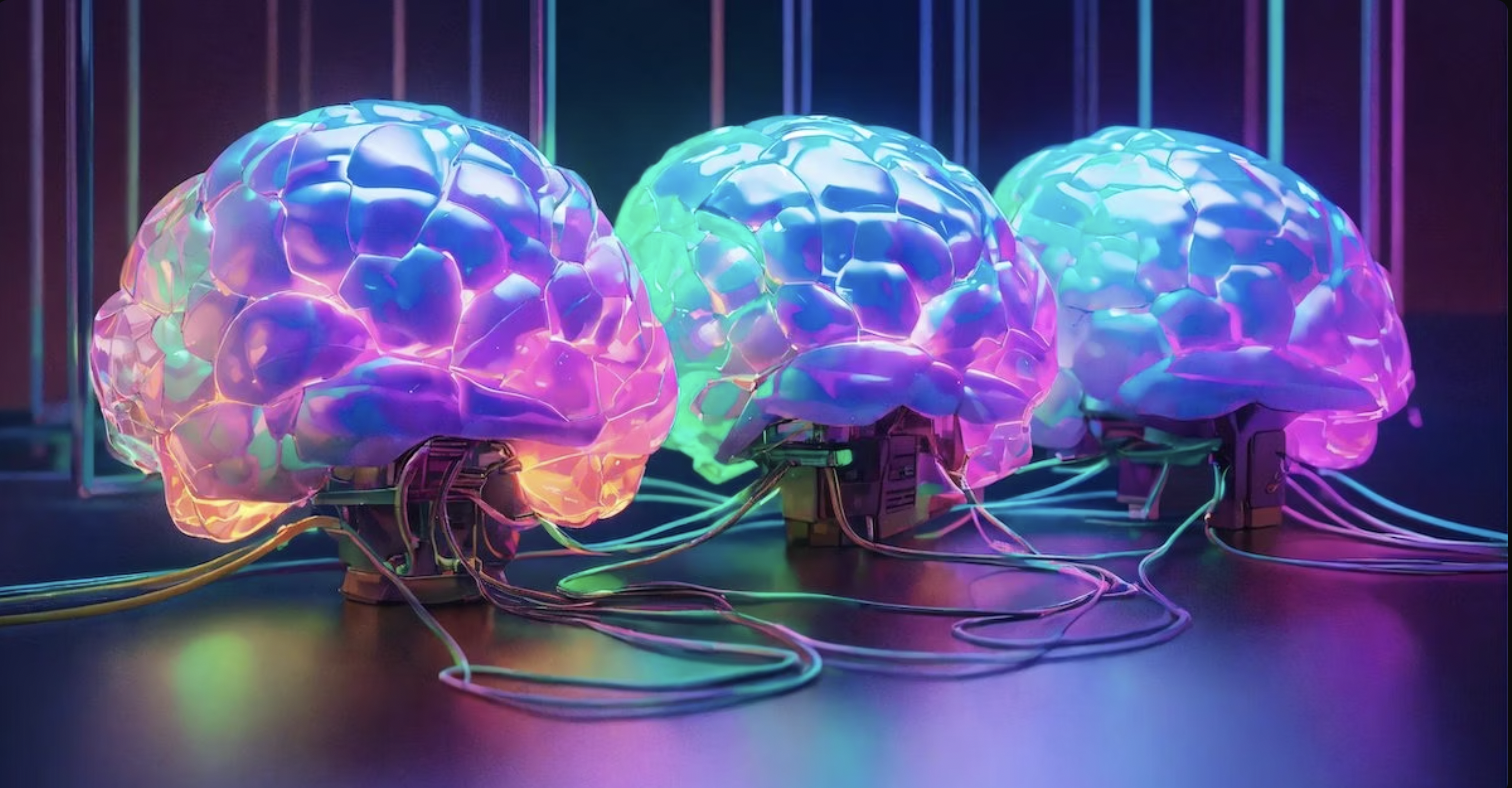